forked from EvanZ/myvagrant
-
Notifications
You must be signed in to change notification settings - Fork 0
/
lab4_machine_learning_student.py
680 lines (514 loc) · 48.5 KB
/
lab4_machine_learning_student.py
1
2
3
4
5
6
7
8
9
10
11
12
13
14
15
16
17
18
19
20
21
22
23
24
25
26
27
28
29
30
31
32
33
34
35
36
37
38
39
40
41
42
43
44
45
46
47
48
49
50
51
52
53
54
55
56
57
58
59
60
61
62
63
64
65
66
67
68
69
70
71
72
73
74
75
76
77
78
79
80
81
82
83
84
85
86
87
88
89
90
91
92
93
94
95
96
97
98
99
100
101
102
103
104
105
106
107
108
109
110
111
112
113
114
115
116
117
118
119
120
121
122
123
124
125
126
127
128
129
130
131
132
133
134
135
136
137
138
139
140
141
142
143
144
145
146
147
148
149
150
151
152
153
154
155
156
157
158
159
160
161
162
163
164
165
166
167
168
169
170
171
172
173
174
175
176
177
178
179
180
181
182
183
184
185
186
187
188
189
190
191
192
193
194
195
196
197
198
199
200
201
202
203
204
205
206
207
208
209
210
211
212
213
214
215
216
217
218
219
220
221
222
223
224
225
226
227
228
229
230
231
232
233
234
235
236
237
238
239
240
241
242
243
244
245
246
247
248
249
250
251
252
253
254
255
256
257
258
259
260
261
262
263
264
265
266
267
268
269
270
271
272
273
274
275
276
277
278
279
280
281
282
283
284
285
286
287
288
289
290
291
292
293
294
295
296
297
298
299
300
301
302
303
304
305
306
307
308
309
310
311
312
313
314
315
316
317
318
319
320
321
322
323
324
325
326
327
328
329
330
331
332
333
334
335
336
337
338
339
340
341
342
343
344
345
346
347
348
349
350
351
352
353
354
355
356
357
358
359
360
361
362
363
364
365
366
367
368
369
370
371
372
373
374
375
376
377
378
379
380
381
382
383
384
385
386
387
388
389
390
391
392
393
394
395
396
397
398
399
400
401
402
403
404
405
406
407
408
409
410
411
412
413
414
415
416
417
418
419
420
421
422
423
424
425
426
427
428
429
430
431
432
433
434
435
436
437
438
439
440
441
442
443
444
445
446
447
448
449
450
451
452
453
454
455
456
457
458
459
460
461
462
463
464
465
466
467
468
469
470
471
472
473
474
475
476
477
478
479
480
481
482
483
484
485
486
487
488
489
490
491
492
493
494
495
496
497
498
499
500
501
502
503
504
505
506
507
508
509
510
511
512
513
514
515
516
517
518
519
520
521
522
523
524
525
526
527
528
529
530
531
532
533
534
535
536
537
538
539
540
541
542
543
544
545
546
547
548
549
550
551
552
553
554
555
556
557
558
559
560
561
562
563
564
565
566
567
568
569
570
571
572
573
574
575
576
577
578
579
580
581
582
583
584
585
586
587
588
589
590
591
592
593
594
595
596
597
598
599
600
601
602
603
604
605
606
607
608
609
610
611
612
613
614
615
616
617
618
619
620
621
622
623
624
625
626
627
628
629
630
631
632
633
634
635
636
637
638
639
640
641
642
643
644
645
646
647
648
649
650
651
652
653
654
655
656
657
658
659
660
661
662
663
664
665
666
667
668
669
670
671
672
673
674
675
676
677
678
679
# coding: utf-8
# version 1.0.1
# #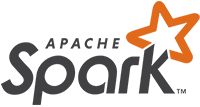 + 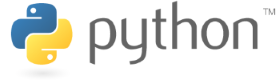
# # **Introduction to Machine Learning with Apache Spark**
# ## **Predicting Movie Ratings**
# #### One of the most common uses of big data is to predict what users want. This allows Google to show you relevant ads, Amazon to recommend relevant products, and Netflix to recommend movies that you might like. This lab will demonstrate how we can use Apache Spark to recommend movies to a user. We will start with some basic techniques, and then use the [Spark MLlib][mllib] library's Alternating Least Squares method to make more sophisticated predictions.
# #### For this lab, we will use a subset dataset of 500,000 ratings we have included for you into your VM (and on Databricks) from the [movielens 10M stable benchmark rating dataset](http://grouplens.org/datasets/movielens/). However, the same code you write will work for the full dataset, or their latest dataset of 21 million ratings.
# #### In this lab:
# #### *Part 0*: Preliminaries
# #### *Part 1*: Basic Recommendations
# #### *Part 2*: Collaborative Filtering
# #### *Part 3*: Predictions for Yourself
# #### As mentioned during the first Learning Spark lab, think carefully before calling `collect()` on any datasets. When you are using a small dataset, calling `collect()` and then using Python to get a sense for the data locally (in the driver program) will work fine, but this will not work when you are using a large dataset that doesn't fit in memory on one machine. Solutions that call `collect()` and do local analysis that could have been done with Spark will likely fail in the autograder and not receive full credit.
# [mllib]: https://spark.apache.org/mllib/
# ### Code
# #### This assignment can be completed using basic Python and pySpark Transformations and Actions. Libraries other than math are not necessary. With the exception of the ML functions that we introduce in this assignment, you should be able to complete all parts of this homework using only the Spark functions you have used in prior lab exercises (although you are welcome to use more features of Spark if you like!).
# In[2]:
import sys
import os
from test_helper import Test
baseDir = os.path.join('data')
inputPath = os.path.join('cs100', 'lab4', 'small')
ratingsFilename = os.path.join(baseDir, inputPath, 'ratings.dat.gz')
moviesFilename = os.path.join(baseDir, inputPath, 'movies.dat')
# ### **Part 0: Preliminaries**
# #### We read in each of the files and create an RDD consisting of parsed lines.
# #### Each line in the ratings dataset (`ratings.dat.gz`) is formatted as:
# #### `UserID::MovieID::Rating::Timestamp`
# #### Each line in the movies (`movies.dat`) dataset is formatted as:
# #### `MovieID::Title::Genres`
# #### The `Genres` field has the format
# #### `Genres1|Genres2|Genres3|...`
# #### The format of these files is uniform and simple, so we can use Python [`split()`](https://docs.python.org/2/library/stdtypes.html#str.split) to parse their lines.
# #### Parsing the two files yields two RDDS
# * #### For each line in the ratings dataset, we create a tuple of (UserID, MovieID, Rating). We drop the timestamp because we do not need it for this exercise.
# * #### For each line in the movies dataset, we create a tuple of (MovieID, Title). We drop the Genres because we do not need them for this exercise.
# In[3]:
numPartitions = 2
rawRatings = sc.textFile(ratingsFilename).repartition(numPartitions)
rawMovies = sc.textFile(moviesFilename)
def get_ratings_tuple(entry):
""" Parse a line in the ratings dataset
Args:
entry (str): a line in the ratings dataset in the form of UserID::MovieID::Rating::Timestamp
Returns:
tuple: (UserID, MovieID, Rating)
"""
items = entry.split('::')
return int(items[0]), int(items[1]), float(items[2])
def get_movie_tuple(entry):
""" Parse a line in the movies dataset
Args:
entry (str): a line in the movies dataset in the form of MovieID::Title::Genres
Returns:
tuple: (MovieID, Title)
"""
items = entry.split('::')
return int(items[0]), items[1]
ratingsRDD = rawRatings.map(get_ratings_tuple).cache()
moviesRDD = rawMovies.map(get_movie_tuple).cache()
ratingsCount = ratingsRDD.count()
moviesCount = moviesRDD.count()
print 'There are %s ratings and %s movies in the datasets' % (ratingsCount, moviesCount)
print 'Ratings: %s' % ratingsRDD.take(3)
print 'Movies: %s' % moviesRDD.take(3)
assert ratingsCount == 487650
assert moviesCount == 3883
assert moviesRDD.filter(lambda (id, title): title == 'Toy Story (1995)').count() == 1
assert (ratingsRDD.takeOrdered(1, key=lambda (user, movie, rating): movie)
== [(1, 1, 5.0)])
# #### In this lab we will be examining subsets of the tuples we create (e.g., the top rated movies by users). Whenever we examine only a subset of a large dataset, there is the potential that the result will depend on the order we perform operations, such as joins, or how the data is partitioned across the workers. What we want to guarantee is that we always see the same results for a subset, independent of how we manipulate or store the data.
# #### We can do that by sorting before we examine a subset. You might think that the most obvious choice when dealing with an RDD of tuples would be to use the [`sortByKey()` method][sortbykey]. However this choice is problematic, as we can still end up with different results if the key is not unique.
# #### Note: It is important to use the [`unicode` type](https://docs.python.org/2/howto/unicode.html#the-unicode-type) instead of the `string` type as the titles are in unicode characters.
# #### Consider the following example, and note that while the sets are equal, the printed lists are usually in different order by value, *although they may randomly match up from time to time.*
# #### You can try running this multiple times. If the last assertion fails, don't worry about it: that was just the luck of the draw. And note that in some environments the results may be more deterministic.
# [sortbykey]: https://spark.apache.org/docs/latest/api/python/pyspark.html#pyspark.RDD.sortByKey
# In[4]:
tmp1 = [(1, u'alpha'), (2, u'alpha'), (2, u'beta'), (3, u'alpha'), (1, u'epsilon'), (1, u'delta')]
tmp2 = [(1, u'delta'), (2, u'alpha'), (2, u'beta'), (3, u'alpha'), (1, u'epsilon'), (1, u'alpha')]
oneRDD = sc.parallelize(tmp1)
twoRDD = sc.parallelize(tmp2)
oneSorted = oneRDD.sortByKey(True).collect()
twoSorted = twoRDD.sortByKey(True).collect()
print oneSorted
print twoSorted
assert set(oneSorted) == set(twoSorted) # Note that both lists have the same elements
assert twoSorted[0][0] < twoSorted.pop()[0] # Check that it is sorted by the keys
assert oneSorted[0:2] != twoSorted[0:2] # Note that the subset consisting of the first two elements does not match
# #### Even though the two lists contain identical tuples, the difference in ordering *sometimes* yields a different ordering for the sorted RDD (try running the cell repeatedly and see if the results change or the assertion fails). If we only examined the first two elements of the RDD (e.g., using `take(2)`), then we would observe different answers - **that is a really bad outcome as we want identical input data to always yield identical output**. A better technique is to sort the RDD by *both the key and value*, which we can do by combining the key and value into a single string and then sorting on that string. Since the key is an integer and the value is a unicode string, we can use a function to combine them into a single unicode string (e.g., `unicode('%.3f' % key) + ' ' + value`) before sorting the RDD using [sortBy()][sortby].
# [sortby]: https://spark.apache.org/docs/latest/api/python/pyspark.html#pyspark.RDD.sortBy
# In[5]:
def sortFunction(tuple):
""" Construct the sort string (does not perform actual sorting)
Args:
tuple: (rating, MovieName)
Returns:
sortString: the value to sort with, 'rating MovieName'
"""
key = unicode('%.3f' % tuple[0])
value = tuple[1]
return (key + ' ' + value)
print oneRDD.sortBy(sortFunction, True).collect()
print twoRDD.sortBy(sortFunction, True).collect()
# #### If we just want to look at the first few elements of the RDD in sorted order, we can use the [takeOrdered][takeordered] method with the `sortFunction` we defined.
# [takeordered]: https://spark.apache.org/docs/latest/api/python/pyspark.html#pyspark.RDD.takeOrdered
# In[6]:
oneSorted1 = oneRDD.takeOrdered(oneRDD.count(),key=sortFunction)
twoSorted1 = twoRDD.takeOrdered(twoRDD.count(),key=sortFunction)
print 'one is %s' % oneSorted1
print 'two is %s' % twoSorted1
assert oneSorted1 == twoSorted1
# ### **Part 1: Basic Recommendations**
# #### One way to recommend movies is to always recommend the movies with the highest average rating. In this part, we will use Spark to find the name, number of ratings, and the average rating of the 20 movies with the highest average rating and more than 500 reviews. We want to filter our movies with high ratings but fewer than or equal to 500 reviews because movies with few reviews may not have broad appeal to everyone.
# #### **(1a) Number of Ratings and Average Ratings for a Movie**
# #### Using only Python, implement a helper function `getCountsAndAverages()` that takes a single tuple of (MovieID, (Rating1, Rating2, Rating3, ...)) and returns a tuple of (MovieID, (number of ratings, averageRating)). For example, given the tuple `(100, (10.0, 20.0, 30.0))`, your function should return `(100, (3, 20.0))`
# In[7]:
# TODO: Replace <FILL IN> with appropriate code
# First, implement a helper function `getCountsAndAverages` using only Python
def getCountsAndAverages(IDandRatingsTuple):
""" Calculate average rating
Args:
IDandRatingsTuple: a single tuple of (MovieID, (Rating1, Rating2, Rating3, ...))
Returns:
tuple: a tuple of (MovieID, (number of ratings, averageRating))
"""
list_tuples = [1.0*rating for rating in IDandRatingsTuple[1]]
return (IDandRatingsTuple[0], (len(list_tuples), sum(list_tuples)/len(list_tuples)))
# In[8]:
# TEST Number of Ratings and Average Ratings for a Movie (1a)
Test.assertEquals(getCountsAndAverages((1, (1, 2, 3, 4))), (1, (4, 2.5)),
'incorrect getCountsAndAverages() with integer list')
Test.assertEquals(getCountsAndAverages((100, (10.0, 20.0, 30.0))), (100, (3, 20.0)),
'incorrect getCountsAndAverages() with float list')
Test.assertEquals(getCountsAndAverages((110, xrange(20))), (110, (20, 9.5)),
'incorrect getCountsAndAverages() with xrange')
# #### **(1b) Movies with Highest Average Ratings**
# #### Now that we have a way to calculate the average ratings, we will use the `getCountsAndAverages()` helper function with Spark to determine movies with highest average ratings.
# #### The steps you should perform are:
# * #### Recall that the `ratingsRDD` contains tuples of the form (UserID, MovieID, Rating). From `ratingsRDD` create an RDD with tuples of the form (MovieID, Python iterable of Ratings for that MovieID). This transformation will yield an RDD of the form: `[(1, <pyspark.resultiterable.ResultIterable object at 0x7f16d50e7c90>), (2, <pyspark.resultiterable.ResultIterable object at 0x7f16d50e79d0>), (3, <pyspark.resultiterable.ResultIterable object at 0x7f16d50e7610>)]`. Note that you will only need to perform two Spark transformations to do this step.
# * #### Using `movieIDsWithRatingsRDD` and your `getCountsAndAverages()` helper function, compute the number of ratings and average rating for each movie to yield tuples of the form (MovieID, (number of ratings, average rating)). This transformation will yield an RDD of the form: `[(1, (993, 4.145015105740181)), (2, (332, 3.174698795180723)), (3, (299, 3.0468227424749164))]`. You can do this step with one Spark transformation
# * #### We want to see movie names, instead of movie IDs. To `moviesRDD`, apply RDD transformations that use `movieIDsWithAvgRatingsRDD` to get the movie names for `movieIDsWithAvgRatingsRDD`, yielding tuples of the form (average rating, movie name, number of ratings). This set of transformations will yield an RDD of the form: `[(1.0, u'Autopsy (Macchie Solari) (1975)', 1), (1.0, u'Better Living (1998)', 1), (1.0, u'Big Squeeze, The (1996)', 3)]`. You will need to do two Spark transformations to complete this step: first use the `moviesRDD` with `movieIDsWithAvgRatingsRDD` to create a new RDD with Movie names matched to Movie IDs, then convert that RDD into the form of (average rating, movie name, number of ratings). These transformations will yield an RDD that looks like: `[(3.6818181818181817, u'Happiest Millionaire, The (1967)', 22), (3.0468227424749164, u'Grumpier Old Men (1995)', 299), (2.882978723404255, u'Hocus Pocus (1993)', 94)]`
# In[13]:
# TODO: Replace <FILL IN> with appropriate code
# From ratingsRDD with tuples of (UserID, MovieID, Rating) create an RDD with tuples of
# the (MovieID, iterable of Ratings for that MovieID)
movieIDsWithRatingsRDD = (ratingsRDD
.map(lambda (userid, movieid, rating): (movieid, rating))
.groupByKey())
print 'movieIDsWithRatingsRDD: %s\n' % movieIDsWithRatingsRDD.take(3)
# Using `movieIDsWithRatingsRDD`, compute the number of ratings and average rating for each movie to
# yield tuples of the form (MovieID, (number of ratings, average rating))
movieIDsWithAvgRatingsRDD = movieIDsWithRatingsRDD.map(lambda rec: getCountsAndAverages(rec))
print 'movieIDsWithAvgRatingsRDD: %s\n' % movieIDsWithAvgRatingsRDD.take(3)
# To `movieIDsWithAvgRatingsRDD`, apply RDD transformations that use `moviesRDD` to get the movie
# names for `movieIDsWithAvgRatingsRDD`, yielding tuples of the form
# (average rating, movie name, number of ratings)
movieNameWithAvgRatingsRDD = (moviesRDD
.join(movieIDsWithAvgRatingsRDD)
.map(lambda (movieID, (movieName, (numRatings, avgRating))): (avgRating, movieName, numRatings)))
print 'movieNameWithAvgRatingsRDD: %s\n' % movieNameWithAvgRatingsRDD.take(3)
# In[14]:
# TEST Movies with Highest Average Ratings (1b)
Test.assertEquals(movieIDsWithRatingsRDD.count(), 3615,
'incorrect movieIDsWithRatingsRDD.count() (expected 3615)')
movieIDsWithRatingsTakeOrdered = movieIDsWithRatingsRDD.takeOrdered(3)
Test.assertTrue(movieIDsWithRatingsTakeOrdered[0][0] == 1 and
len(list(movieIDsWithRatingsTakeOrdered[0][1])) == 993,
'incorrect count of ratings for movieIDsWithRatingsTakeOrdered[0] (expected 993)')
Test.assertTrue(movieIDsWithRatingsTakeOrdered[1][0] == 2 and
len(list(movieIDsWithRatingsTakeOrdered[1][1])) == 332,
'incorrect count of ratings for movieIDsWithRatingsTakeOrdered[1] (expected 332)')
Test.assertTrue(movieIDsWithRatingsTakeOrdered[2][0] == 3 and
len(list(movieIDsWithRatingsTakeOrdered[2][1])) == 299,
'incorrect count of ratings for movieIDsWithRatingsTakeOrdered[2] (expected 299)')
Test.assertEquals(movieIDsWithAvgRatingsRDD.count(), 3615,
'incorrect movieIDsWithAvgRatingsRDD.count() (expected 3615)')
Test.assertEquals(movieIDsWithAvgRatingsRDD.takeOrdered(3),
[(1, (993, 4.145015105740181)), (2, (332, 3.174698795180723)),
(3, (299, 3.0468227424749164))],
'incorrect movieIDsWithAvgRatingsRDD.takeOrdered(3)')
Test.assertEquals(movieNameWithAvgRatingsRDD.count(), 3615,
'incorrect movieNameWithAvgRatingsRDD.count() (expected 3615)')
Test.assertEquals(movieNameWithAvgRatingsRDD.takeOrdered(3),
[(1.0, u'Autopsy (Macchie Solari) (1975)', 1), (1.0, u'Better Living (1998)', 1),
(1.0, u'Big Squeeze, The (1996)', 3)],
'incorrect movieNameWithAvgRatingsRDD.takeOrdered(3)')
# #### **(1c) Movies with Highest Average Ratings and more than 500 reviews**
# #### Now that we have an RDD of the movies with highest averge ratings, we can use Spark to determine the 20 movies with highest average ratings and more than 500 reviews.
# #### Apply a single RDD transformation to `movieNameWithAvgRatingsRDD` to limit the results to movies with ratings from more than 500 people. We then use the `sortFunction()` helper function to sort by the average rating to get the movies in order of their rating (highest rating first). You will end up with an RDD of the form: `[(4.5349264705882355, u'Shawshank Redemption, The (1994)', 1088), (4.515798462852263, u"Schindler's List (1993)", 1171), (4.512893982808023, u'Godfather, The (1972)', 1047)]`
# In[16]:
# TODO: Replace <FILL IN> with appropriate code
# Apply an RDD transformation to `movieNameWithAvgRatingsRDD` to limit the results to movies with
# ratings from more than 500 people. We then use the `sortFunction()` helper function to sort by the
# average rating to get the movies in order of their rating (highest rating first)
movieLimitedAndSortedByRatingRDD = (movieNameWithAvgRatingsRDD
.filter(lambda (_, __, numRatings): numRatings > 500)
.sortBy(sortFunction, False))
print 'Movies with highest ratings: %s' % movieLimitedAndSortedByRatingRDD.take(20)
# In[17]:
# TEST Movies with Highest Average Ratings and more than 500 Reviews (1c)
Test.assertEquals(movieLimitedAndSortedByRatingRDD.count(), 194,
'incorrect movieLimitedAndSortedByRatingRDD.count()')
Test.assertEquals(movieLimitedAndSortedByRatingRDD.take(20),
[(4.5349264705882355, u'Shawshank Redemption, The (1994)', 1088),
(4.515798462852263, u"Schindler's List (1993)", 1171),
(4.512893982808023, u'Godfather, The (1972)', 1047),
(4.510460251046025, u'Raiders of the Lost Ark (1981)', 1195),
(4.505415162454874, u'Usual Suspects, The (1995)', 831),
(4.457256461232604, u'Rear Window (1954)', 503),
(4.45468509984639, u'Dr. Strangelove or: How I Learned to Stop Worrying and Love the Bomb (1963)', 651),
(4.43953006219765, u'Star Wars: Episode IV - A New Hope (1977)', 1447),
(4.4, u'Sixth Sense, The (1999)', 1110), (4.394285714285714, u'North by Northwest (1959)', 700),
(4.379506641366224, u'Citizen Kane (1941)', 527), (4.375, u'Casablanca (1942)', 776),
(4.363975155279503, u'Godfather: Part II, The (1974)', 805),
(4.358816276202219, u"One Flew Over the Cuckoo's Nest (1975)", 811),
(4.358173076923077, u'Silence of the Lambs, The (1991)', 1248),
(4.335826477187734, u'Saving Private Ryan (1998)', 1337),
(4.326241134751773, u'Chinatown (1974)', 564),
(4.325383304940375, u'Life Is Beautiful (La Vita \ufffd bella) (1997)', 587),
(4.324110671936759, u'Monty Python and the Holy Grail (1974)', 759),
(4.3096, u'Matrix, The (1999)', 1250)], 'incorrect sortedByRatingRDD.take(20)')
# #### Using a threshold on the number of reviews is one way to improve the recommendations, but there are many other good ways to improve quality. For example, you could weight ratings by the number of ratings.
# ## **Part 2: Collaborative Filtering**
# #### In this course, you have learned about many of the basic transformations and actions that Spark allows us to apply to distributed datasets. Spark also exposes some higher level functionality; in particular, Machine Learning using a component of Spark called [MLlib][mllib]. In this part, you will learn how to use MLlib to make personalized movie recommendations using the movie data we have been analyzing.
# #### We are going to use a technique called [collaborative filtering][collab]. Collaborative filtering is a method of making automatic predictions (filtering) about the interests of a user by collecting preferences or taste information from many users (collaborating). The underlying assumption of the collaborative filtering approach is that if a person A has the same opinion as a person B on an issue, A is more likely to have B's opinion on a different issue x than to have the opinion on x of a person chosen randomly. You can read more about collaborative filtering [here][collab2].
# #### The image below (from [Wikipedia][collab]) shows an example of predicting of the user's rating using collaborative filtering. At first, people rate different items (like videos, images, games). After that, the system is making predictions about a user's rating for an item, which the user has not rated yet. These predictions are built upon the existing ratings of other users, who have similar ratings with the active user. For instance, in the image below the system has made a prediction, that the active user will not like the video.
# 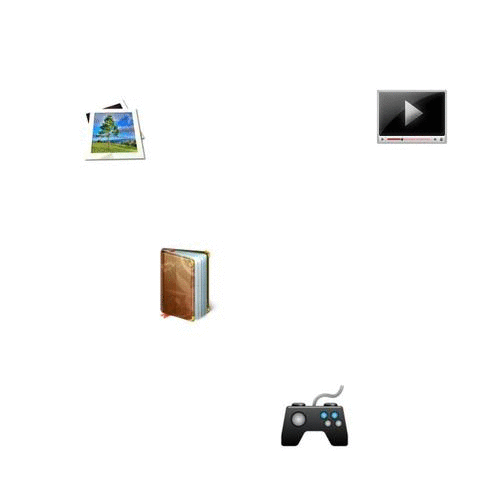
# [mllib]: https://spark.apache.org/mllib/
# [collab]: https://en.wikipedia.org/?title=Collaborative_filtering
# [collab2]: http://recommender-systems.org/collaborative-filtering/
# #### For movie recommendations, we start with a matrix whose entries are movie ratings by users (shown in red in the diagram below). Each column represents a user (shown in green) and each row represents a particular movie (shown in blue).
# #### Since not all users have rated all movies, we do not know all of the entries in this matrix, which is precisely why we need collaborative filtering. For each user, we have ratings for only a subset of the movies. With collaborative filtering, the idea is to approximate the ratings matrix by factorizing it as the product of two matrices: one that describes properties of each user (shown in green), and one that describes properties of each movie (shown in blue).
# 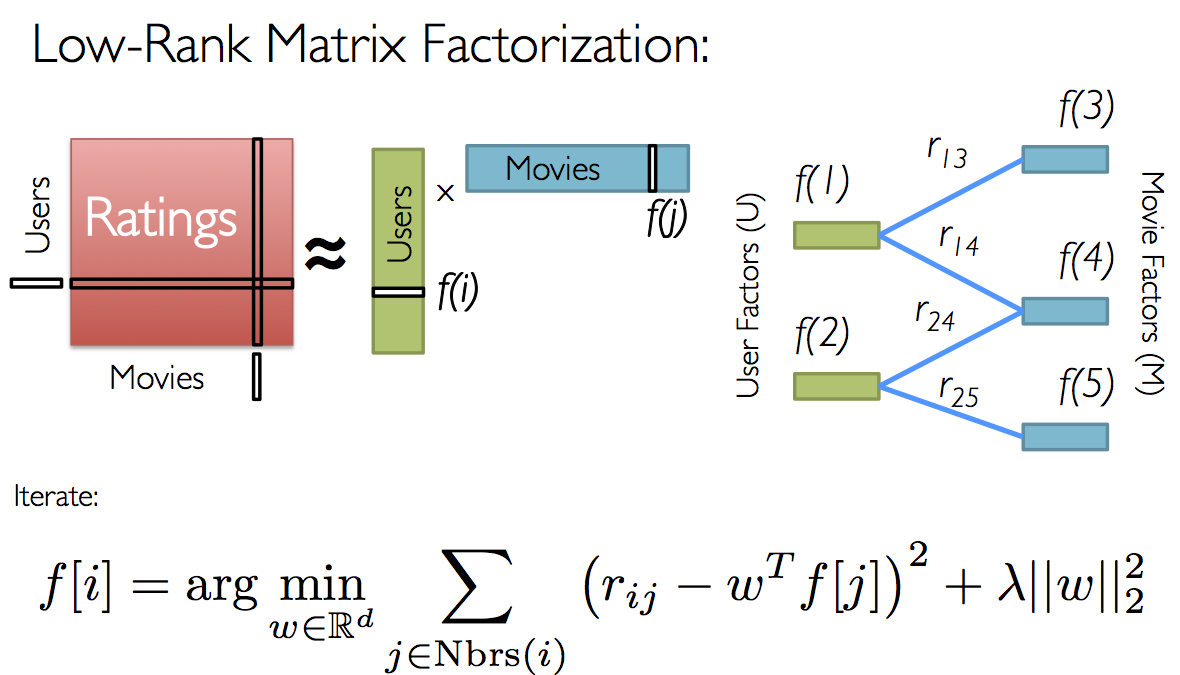
# #### We want to select these two matrices such that the error for the users/movie pairs where we know the correct ratings is minimized. The [Alternating Least Squares][als] algorithm does this by first randomly filling the users matrix with values and then optimizing the value of the movies such that the error is minimized. Then, it holds the movies matrix constrant and optimizes the value of the user's matrix. This alternation between which matrix to optimize is the reason for the "alternating" in the name.
# #### This optimization is what's being shown on the right in the image above. Given a fixed set of user factors (i.e., values in the users matrix), we use the known ratings to find the best values for the movie factors using the optimization written at the bottom of the figure. Then we "alternate" and pick the best user factors given fixed movie factors.
# #### For a simple example of what the users and movies matrices might look like, check out the [videos from Lecture 8][videos] or the [slides from Lecture 8][slides]
# [videos]: https://courses.edx.org/courses/BerkeleyX/CS100.1x/1T2015/courseware/00eb8b17939b4889a41a6d8d2f35db83/3bd3bba368be4102b40780550d3d8da6/
# [slides]: https://courses.edx.org/c4x/BerkeleyX/CS100.1x/asset/Week4Lec8.pdf
# [als]: https://en.wikiversity.org/wiki/Least-Squares_Method
# #### **(2a) Creating a Training Set**
# #### Before we jump into using machine learning, we need to break up the `ratingsRDD` dataset into three pieces:
# * #### A training set (RDD), which we will use to train models
# * #### A validation set (RDD), which we will use to choose the best model
# * #### A test set (RDD), which we will use for our experiments
# #### To randomly split the dataset into the multiple groups, we can use the pySpark [randomSplit()](https://spark.apache.org/docs/latest/api/python/pyspark.html#pyspark.RDD.randomSplit) transformation. `randomSplit()` takes a set of splits and and seed and returns multiple RDDs.
# In[18]:
trainingRDD, validationRDD, testRDD = ratingsRDD.randomSplit([6, 2, 2], seed=0L)
print 'Training: %s, validation: %s, test: %s\n' % (trainingRDD.count(),
validationRDD.count(),
testRDD.count())
print trainingRDD.take(3)
print validationRDD.take(3)
print testRDD.take(3)
assert trainingRDD.count() == 292716
assert validationRDD.count() == 96902
assert testRDD.count() == 98032
assert trainingRDD.filter(lambda t: t == (1, 914, 3.0)).count() == 1
assert trainingRDD.filter(lambda t: t == (1, 2355, 5.0)).count() == 1
assert trainingRDD.filter(lambda t: t == (1, 595, 5.0)).count() == 1
assert validationRDD.filter(lambda t: t == (1, 1287, 5.0)).count() == 1
assert validationRDD.filter(lambda t: t == (1, 594, 4.0)).count() == 1
assert validationRDD.filter(lambda t: t == (1, 1270, 5.0)).count() == 1
assert testRDD.filter(lambda t: t == (1, 1193, 5.0)).count() == 1
assert testRDD.filter(lambda t: t == (1, 2398, 4.0)).count() == 1
assert testRDD.filter(lambda t: t == (1, 1035, 5.0)).count() == 1
# #### After splitting the dataset, your training set has about 293,000 entries and the validation and test sets each have about 97,000 entries (the exact number of entries in each dataset varies slightly due to the random nature of the `randomSplit()` transformation.
# #### **(2b) Root Mean Square Error (RMSE)**
# #### In the next part, you will generate a few different models, and will need a way to decide which model is best. We will use the [Root Mean Square Error](https://en.wikipedia.org/wiki/Root-mean-square_deviation) (RMSE) or Root Mean Square Deviation (RMSD) to compute the error of each model. RMSE is a frequently used measure of the differences between values (sample and population values) predicted by a model or an estimator and the values actually observed. The RMSD represents the sample standard deviation of the differences between predicted values and observed values. These individual differences are called residuals when the calculations are performed over the data sample that was used for estimation, and are called prediction errors when computed out-of-sample. The RMSE serves to aggregate the magnitudes of the errors in predictions for various times into a single measure of predictive power. RMSE is a good measure of accuracy, but only to compare forecasting errors of different models for a particular variable and not between variables, as it is scale-dependent.
# #### The RMSE is the square root of the average value of the square of `(actual rating - predicted rating)` for all users and movies for which we have the actual rating. Versions of Spark MLlib beginning with Spark 1.4 include a [RegressionMetrics](https://spark.apache.org/docs/latest/api/python/pyspark.mllib.html#pyspark.mllib.evaluation.RegressionMetrics) modiule that can be used to compute the RMSE. However, since we are using Spark 1.3.1, we will write our own function.
# #### Write a function to compute the sum of squared error given `predictedRDD` and `actualRDD` RDDs. Both RDDs consist of tuples of the form (UserID, MovieID, Rating)
# #### Given two ratings RDDs, *x* and *y* of size *n*, we define RSME as follows: $ RMSE = \sqrt{\frac{\sum_{i = 1}^{n} (x_i - y_i)^2}{n}}$
# #### To calculate RSME, the steps you should perform are:
# * #### Transform `predictedRDD` into the tuples of the form ((UserID, MovieID), Rating). For example, tuples like `[((1, 1), 5), ((1, 2), 3), ((1, 3), 4), ((2, 1), 3), ((2, 2), 2), ((2, 3), 4)]`. You can perform this step with a single Spark transformation.
# * #### Transform `actualRDD` into the tuples of the form ((UserID, MovieID), Rating). For example, tuples like `[((1, 2), 3), ((1, 3), 5), ((2, 1), 5), ((2, 2), 1)]`. You can perform this step with a single Spark transformation.
# * #### Using only RDD transformations (you only need to perform two transformations), compute the squared error for each *matching* entry (i.e., the same (UserID, MovieID) in each RDD) in the reformatted RDDs - do *not* use `collect()` to perform this step. Note that not every (UserID, MovieID) pair will appear in both RDDs - if a pair does not appear in both RDDs, then it does not contribute to the RMSE. You will end up with an RDD with entries of the form $ (x_i - y_i)^2$ You might want to check out Python's [math](https://docs.python.org/2/library/math.html) module to see how to compute these values
# * #### Using an RDD action (but **not** `collect()`), compute the total squared error: $ SE = \sum_{i = 1}^{n} (x_i - y_i)^2 $
# * #### Compute *n* by using an RDD action (but **not** `collect()`), to count the number of pairs for which you computed the total squared error
# * #### Using the total squared error and the number of pairs, compute the RSME. Make sure you compute this value as a [float](https://docs.python.org/2/library/stdtypes.html#numeric-types-int-float-long-complex).
# #### Note: Your solution must only use transformations and actions on RDDs. Do _not_ call `collect()` on either RDD.
# In[46]:
# TODO: Replace <FILL IN> with appropriate code
import math
def computeError(predictedRDD, actualRDD):
""" Compute the root mean squared error between predicted and actual
Args:
predictedRDD: predicted ratings for each movie and each user where each entry is in the form
(UserID, MovieID, Rating)
actualRDD: actual ratings where each entry is in the form (UserID, MovieID, Rating)
Returns:
RSME (float): computed RSME value
"""
# Transform predictedRDD into the tuples of the form ((UserID, MovieID), Rating)
predictedReformattedRDD = predictedRDD.map(lambda (UserID, MovieID, Rating): ((UserID, MovieID), Rating))
# Transform actualRDD into the tuples of the form ((UserID, MovieID), Rating)
actualReformattedRDD = actualRDD.map(lambda (UserID, MovieID, Rating): ((UserID, MovieID), Rating))
# Compute the squared error for each matching entry (i.e., the same (User ID, Movie ID) in each
# RDD) in the reformatted RDDs using RDD transformtions - do not use collect()
squaredErrorsRDD = (predictedReformattedRDD
.join(actualReformattedRDD)
.map(lambda ((UserID, MovieID), (PredRating, ActRating)): (PredRating - ActRating)**2))
# Compute the total squared error - do not use collect()
totalError = squaredErrorsRDD.reduce(lambda a,b: a+b)
# Count the number of entries for which you computed the total squared error
numRatings = squaredErrorsRDD.count()
# Using the total squared error and the number of entries, compute the RSME
return math.sqrt(1.0*totalError/numRatings)
# sc.parallelize turns a Python list into a Spark RDD.
testPredicted = sc.parallelize([
(1, 1, 5),
(1, 2, 3),
(1, 3, 4),
(2, 1, 3),
(2, 2, 2),
(2, 3, 4)])
testActual = sc.parallelize([
(1, 2, 3),
(1, 3, 5),
(2, 1, 5),
(2, 2, 1)])
testPredicted2 = sc.parallelize([
(2, 2, 5),
(1, 2, 5)])
testError = computeError(testPredicted, testActual)
print 'Error for test dataset (should be 1.22474487139): %s' % testError
testError2 = computeError(testPredicted2, testActual)
print 'Error for test dataset2 (should be 3.16227766017): %s' % testError2
testError3 = computeError(testActual, testActual)
print 'Error for testActual dataset (should be 0.0): %s' % testError3
# In[47]:
# TEST Root Mean Square Error (2b)
Test.assertTrue(abs(testError - 1.22474487139) < 0.00000001,
'incorrect testError (expected 1.22474487139)')
Test.assertTrue(abs(testError2 - 3.16227766017) < 0.00000001,
'incorrect testError2 result (expected 3.16227766017)')
Test.assertTrue(abs(testError3 - 0.0) < 0.00000001,
'incorrect testActual result (expected 0.0)')
# #### **(2c) Using ALS.train()**
# #### In this part, we will use the MLlib implementation of Alternating Least Squares, [ALS.train()](https://spark.apache.org/docs/latest/api/python/pyspark.mllib.html#pyspark.mllib.recommendation.ALS). ALS takes a training dataset (RDD) and several parameters that control the model creation process. To determine the best values for the parameters, we will use ALS to train several models, and then we will select the best model and use the parameters from that model in the rest of this lab exercise.
# #### The process we will use for determining the best model is as follows:
# * #### Pick a set of model parameters. The most important parameter to `ALS.train()` is the *rank*, which is the number of rows in the Users matrix (green in the diagram above) or the number of columns in the Movies matrix (blue in the diagram above). (In general, a lower rank will mean higher error on the training dataset, but a high rank may lead to [overfitting](https://en.wikipedia.org/wiki/Overfitting).) We will train models with ranks of 4, 8, and 12 using the `trainingRDD` dataset.
# * #### Create a model using `ALS.train(trainingRDD, rank, seed=seed, iterations=iterations, lambda_=regularizationParameter)` with three parameters: an RDD consisting of tuples of the form (UserID, MovieID, rating) used to train the model, an integer rank (4, 8, or 12), a number of iterations to execute (we will use 5 for the `iterations` parameter), and a regularization coefficient (we will use 0.1 for the `regularizationParameter`).
# * #### For the prediction step, create an input RDD, `validationForPredictRDD`, consisting of (UserID, MovieID) pairs that you extract from `validationRDD`. You will end up with an RDD of the form: `[(1, 1287), (1, 594), (1, 1270)]`
# * #### Using the model and `validationForPredictRDD`, we can predict rating values by calling [model.predictAll()](https://spark.apache.org/docs/latest/api/python/pyspark.mllib.html#pyspark.mllib.recommendation.MatrixFactorizationModel.predictAll) with the `validationForPredictRDD` dataset, where `model` is the model we generated with ALS.train(). `predictAll` accepts an RDD with each entry in the format (userID, movieID) and outputs an RDD with each entry in the format (userID, movieID, rating).
# * #### Evaluate the quality of the model by using the `computeError()` function you wrote in part (2b) to compute the error between the predicted ratings and the actual ratings in `validationRDD`.
# #### Which rank produces the best model, based on the RMSE with the `validationRDD` dataset?
# #### Note: It is likely that this operation will take a noticeable amount of time (around a minute in our VM); you can observe its progress on the [Spark Web UI](http://localhost:4040). Probably most of the time will be spent running your `computeError()` function, since, unlike the Spark ALS implementation (and the Spark 1.4 [RegressionMetrics](https://spark.apache.org/docs/latest/api/python/pyspark.mllib.html#pyspark.mllib.evaluation.RegressionMetrics) module), this does not use a fast linear algebra library and needs to run some Python code for all 100k entries.
# In[49]:
# TODO: Replace <FILL IN> with appropriate code
from pyspark.mllib.recommendation import ALS
validationForPredictRDD = validationRDD.map(lambda (UserID, MovieID, Rating): (UserID, MovieID))
seed = 5L
iterations = 5
regularizationParameter = 0.1
ranks = [4, 8, 12]
errors = [0, 0, 0]
err = 0
tolerance = 0.02
minError = float('inf')
bestRank = -1
bestIteration = -1
for rank in ranks:
model = ALS.train(trainingRDD, rank, seed=seed, iterations=iterations,
lambda_=regularizationParameter)
predictedRatingsRDD = model.predictAll(validationForPredictRDD)
error = computeError(predictedRatingsRDD, validationRDD)
errors[err] = error
err += 1
print 'For rank %s the RMSE is %s' % (rank, error)
if error < minError:
minError = error
bestRank = rank
print 'The best model was trained with rank %s' % bestRank
# In[50]:
# TEST Using ALS.train (2c)
Test.assertEquals(trainingRDD.getNumPartitions(), 2,
'incorrect number of partitions for trainingRDD (expected 2)')
Test.assertEquals(validationForPredictRDD.count(), 96902,
'incorrect size for validationForPredictRDD (expected 96902)')
Test.assertEquals(validationForPredictRDD.filter(lambda t: t == (1, 1907)).count(), 1,
'incorrect content for validationForPredictRDD')
Test.assertTrue(abs(errors[0] - 0.883710109497) < tolerance, 'incorrect errors[0]')
Test.assertTrue(abs(errors[1] - 0.878486305621) < tolerance, 'incorrect errors[1]')
Test.assertTrue(abs(errors[2] - 0.876832795659) < tolerance, 'incorrect errors[2]')
# #### **(2d) Testing Your Model**
# #### So far, we used the `trainingRDD` and `validationRDD` datasets to select the best model. Since we used these two datasets to determine what model is best, we cannot use them to test how good the model is - otherwise we would be very vulnerable to [overfitting](https://en.wikipedia.org/wiki/Overfitting). To decide how good our model is, we need to use the `testRDD` dataset. We will use the `bestRank` you determined in part (2c) to create a model for predicting the ratings for the test dataset and then we will compute the RMSE.
# #### The steps you should perform are:
# * #### Train a model, using the `trainingRDD`, `bestRank` from part (2c), and the parameters you used in in part (2c): `seed=seed`, `iterations=iterations`, and `lambda_=regularizationParameter` - make sure you include **all** of the parameters.
# * #### For the prediction step, create an input RDD, `testForPredictingRDD`, consisting of (UserID, MovieID) pairs that you extract from `testRDD`. You will end up with an RDD of the form: `[(1, 1287), (1, 594), (1, 1270)]`
# * #### Use [myModel.predictAll()](https://spark.apache.org/docs/latest/api/python/pyspark.mllib.html#pyspark.mllib.recommendation.MatrixFactorizationModel.predictAll) to predict rating values for the test dataset.
# * #### For validation, use the `testRDD`and your `computeError` function to compute the RMSE between `testRDD` and the `predictedTestRDD` from the model.
# * #### Evaluate the quality of the model by using the `computeError()` function you wrote in part (2b) to compute the error between the predicted ratings and the actual ratings in `testRDD`.
# In[52]:
# TODO: Replace <FILL IN> with appropriate code
myModel = ALS.train(trainingRDD, bestRank, seed=seed, iterations=iterations,
lambda_=regularizationParameter)
testForPredictingRDD = testRDD.map(lambda (UserID, MovieID, Rating): (UserID, MovieID))
predictedTestRDD = myModel.predictAll(testForPredictingRDD)
testRMSE = computeError(testRDD, predictedTestRDD)
print 'The model had a RMSE on the test set of %s' % testRMSE
# In[53]:
# TEST Testing Your Model (2d)
Test.assertTrue(abs(testRMSE - 0.87809838344) < tolerance, 'incorrect testRMSE')
# #### **(2e) Comparing Your Model**
# #### Looking at the RMSE for the results predicted by the model versus the values in the test set is one way to evalute the quality of our model. Another way to evaluate the model is to evaluate the error from a test set where every rating is the average rating for the training set.
# #### The steps you should perform are:
# * #### Use the `trainingRDD` to compute the average rating across all movies in that training dataset.
# * #### Use the average rating that you just determined and the `testRDD` to create an RDD with entries of the form (userID, movieID, average rating).
# * #### Use your `computeError` function to compute the RMSE between the `testRDD` validation RDD that you just created and the `testForAvgRDD`.
# In[55]:
# TODO: Replace <FILL IN> with appropriate code
trainingAvgRating = trainingRDD.map(lambda (UserID, MovieID, Rating): Rating).mean()
print 'The average rating for movies in the training set is %s' % trainingAvgRating
testForAvgRDD = testRDD.map(lambda (UserID, MovieID, Rating): (UserID, MovieID, trainingAvgRating))
testAvgRMSE = computeError(testRDD, testForAvgRDD)
print 'The RMSE on the average set is %s' % testAvgRMSE
# In[56]:
# TEST Comparing Your Model (2e)
Test.assertTrue(abs(trainingAvgRating - 3.57409571052) < 0.000001,
'incorrect trainingAvgRating (expected 3.57409571052)')
Test.assertTrue(abs(testAvgRMSE - 1.12036693569) < 0.000001,
'incorrect testAvgRMSE (expected 1.12036693569)')
# #### You now have code to predict how users will rate movies!
# ## **Part 3: Predictions for Yourself**
# #### The ultimate goal of this lab exercise is to predict what movies to recommend to yourself. In order to do that, you will first need to add ratings for yourself to the `ratingsRDD` dataset.
# #### **(3a) Your Movie Ratings**
# #### To help you provide ratings for yourself, we have included the following code to list the names and movie IDs of the 50 highest-rated movies from `movieLimitedAndSortedByRatingRDD` which we created in part 1 the lab.
# In[57]:
print 'Most rated movies:'
print '(average rating, movie name, number of reviews)'
for ratingsTuple in movieLimitedAndSortedByRatingRDD.take(50):
print ratingsTuple
# #### The user ID 0 is unassigned, so we will use it for your ratings. We set the variable `myUserID` to 0 for you. Next, create a new RDD `myRatingsRDD` with your ratings for at least 10 movie ratings. Each entry should be formatted as `(myUserID, movieID, rating)` (i.e., each entry should be formatted in the same way as `trainingRDD`). As in the original dataset, ratings should be between 1 and 5 (inclusive). If you have not seen at least 10 of these movies, you can increase the parameter passed to `take()` in the above cell until there are 10 movies that you have seen (or you can also guess what your rating would be for movies you have not seen).
# In[59]:
# TODO: Replace <FILL IN> with appropriate code
myUserID = 0
# Note that the movie IDs are the *last* number on each line. A common error was to use the number of ratings as the movie ID.
myRatedMovies = [
(myUserID, 1088, 5),
(myUserID, 1171, 5),
(myUserID, 941, 5),
(myUserID, 1083, 4),
(myUserID, 789, 5),
(myUserID, 993, 4),
(myUserID, 662, 4),
(myUserID, 817, 4),
(myUserID, 564, 5),
(myUserID, 633, 3)
# The format of each line is (myUserID, movie ID, your rating)
# For example, to give the movie "Star Wars: Episode IV - A New Hope (1977)" a five rating, you would add the following line:
# (myUserID, 260, 5),
]
myRatingsRDD = sc.parallelize(myRatedMovies)
print 'My movie ratings: %s' % myRatingsRDD.take(10)
# #### **(3b) Add Your Movies to Training Dataset**
# #### Now that you have ratings for yourself, you need to add your ratings to the `training` dataset so that the model you train will incorporate your preferences. Spark's [union()](http://spark.apache.org/docs/latest/api/python/pyspark.rdd.RDD-class.html#union) transformation combines two RDDs; use `union()` to create a new training dataset that includes your ratings and the data in the original training dataset.
# In[60]:
# TODO: Replace <FILL IN> with appropriate code
trainingWithMyRatingsRDD = trainingRDD.union(myRatingsRDD)
print ('The training dataset now has %s more entries than the original training dataset' %
(trainingWithMyRatingsRDD.count() - trainingRDD.count()))
assert (trainingWithMyRatingsRDD.count() - trainingRDD.count()) == myRatingsRDD.count()
# #### **(3c) Train a Model with Your Ratings**
# #### Now, train a model with your ratings added and the parameters you used in in part (2c): `bestRank`, `seed=seed`, `iterations=iterations`, and `lambda_=regularizationParameter` - make sure you include **all** of the parameters.
# In[62]:
# TODO: Replace <FILL IN> with appropriate code
myRatingsModel = ALS.train(trainingWithMyRatingsRDD, bestRank, seed=seed, iterations=iterations,
lambda_=regularizationParameter)
# #### **(3d) Check RMSE for the New Model with Your Ratings**
# #### Compute the RMSE for this new model on the test set.
# * #### For the prediction step, we reuse `testForPredictingRDD`, consisting of (UserID, MovieID) pairs that you extracted from `testRDD`. The RDD has the form: `[(1, 1287), (1, 594), (1, 1270)]`
# * #### Use `myRatingsModel.predictAll()` to predict rating values for the `testForPredictingRDD` test dataset, set this as `predictedTestMyRatingsRDD`
# * #### For validation, use the `testRDD`and your `computeError` function to compute the RMSE between `testRDD` and the `predictedTestMyRatingsRDD` from the model.
# In[63]:
# TODO: Replace <FILL IN> with appropriate code
predictedTestMyRatingsRDD = myRatingsModel.predictAll(testForPredictingRDD)
testRMSEMyRatings = computeError(testRDD, predictedTestMyRatingsRDD)
print 'The model had a RMSE on the test set of %s' % testRMSEMyRatings
# #### **(3e) Predict Your Ratings**
# #### So far, we have only used the `predictAll` method to compute the error of the model. Here, use the `predictAll` to predict what ratings you would give to the movies that you did not already provide ratings for.
# #### The steps you should perform are:
# * #### Use the Python list `myRatedMovies` to transform the `moviesRDD` into an RDD with entries that are pairs of the form (myUserID, Movie ID) and that does not contain any movies that you have rated. This transformation will yield an RDD of the form: `[(0, 1), (0, 2), (0, 3), (0, 4)]`. Note that you can do this step with one RDD transformation.
# * #### For the prediction step, use the input RDD, `myUnratedMoviesRDD`, with myRatingsModel.predictAll() to predict your ratings for the movies.
# In[75]:
# TODO: Replace <FILL IN> with appropriate code
# Use the Python list myRatedMovies to transform the moviesRDD into an RDD with entries that are pairs of the form (myUserID, Movie ID) and that does not contain any movies that you have rated.
myUnratedMoviesRDD = (moviesRDD
.map(lambda (MovieID, Title): (myUserID, MovieID))
.filter(lambda (myUserID, MovieID): (myUserID, MovieID) not in [(user, movie) for (user,movie,rating) in myRatedMovies]))
# Use the input RDD, myUnratedMoviesRDD, with myRatingsModel.predictAll() to predict your ratings for the movies
predictedRatingsRDD = myRatingsModel.predictAll(myUnratedMoviesRDD)
# #### **(3f) Predict Your Ratings**
# #### We have our predicted ratings. Now we can print out the 25 movies with the highest predicted ratings.
# #### The steps you should perform are:
# * #### From Parts (1b) and (1c), we know that we should look at movies with a reasonable number of reviews (e.g., more than 75 reviews). You can experiment with a lower threshold, but fewer ratings for a movie may yield higher prediction errors. Transform `movieIDsWithAvgRatingsRDD` from Part (1b), which has the form (MovieID, (number of ratings, average rating)), into an RDD of the form (MovieID, number of ratings): `[(2, 332), (4, 71), (6, 442)]`
# * #### We want to see movie names, instead of movie IDs. Transform `predictedRatingsRDD` into an RDD with entries that are pairs of the form (Movie ID, Predicted Rating): `[(3456, -0.5501005376936687), (1080, 1.5885892024487962), (320, -3.7952255522487865)]`
# * #### Use RDD transformations with `predictedRDD` and `movieCountsRDD` to yield an RDD with tuples of the form (Movie ID, (Predicted Rating, number of ratings)): `[(2050, (0.6694097486155939, 44)), (10, (5.29762541533513, 418)), (2060, (0.5055259373841172, 97))]`
# * #### Use RDD transformations with `predictedWithCountsRDD` and `moviesRDD` to yield an RDD with tuples of the form (Predicted Rating, Movie Name, number of ratings), _for movies with more than 75 ratings._ For example: `[(7.983121900375243, u'Under Siege (1992)'), (7.9769201864261285, u'Fifth Element, The (1997)')]`
# In[80]:
# TODO: Replace <FILL IN> with appropriate code
# Transform movieIDsWithAvgRatingsRDD from part (1b), which has the form (MovieID, (number of ratings, average rating)), into and RDD of the form (MovieID, number of ratings)
movieCountsRDD = movieIDsWithAvgRatingsRDD.map(lambda (MovieID, (numRatings, avgRating)): (MovieID, numRatings))
# Transform predictedRatingsRDD into an RDD with entries that are pairs of the form (Movie ID, Predicted Rating)
predictedRDD = predictedRatingsRDD.map(lambda (UserID, MovieID, Rating): (MovieID, Rating))
# Use RDD transformations with predictedRDD and movieCountsRDD to yield an RDD with tuples of the form (Movie ID, (Predicted Rating, number of ratings))
predictedWithCountsRDD = (predictedRDD
.join(movieCountsRDD))
# Use RDD transformations with PredictedWithCountsRDD and moviesRDD to yield an RDD with tuples of the form (Predicted Rating, Movie Name, number of ratings), for movies with more than 75 ratings
ratingsWithNamesRDD = (predictedWithCountsRDD
.join(moviesRDD)
.map(lambda (MovieID, ((rating, numRatings), MovieName)): (rating, MovieName, numRatings))
.filter(lambda (rating, MovieName, numRatings): numRatings > 75))
predictedHighestRatedMovies = ratingsWithNamesRDD.takeOrdered(20, key=lambda x: -x[0])
print ('My highest rated movies as predicted (for movies with more than 75 reviews):\n%s' %
'\n'.join(map(str, predictedHighestRatedMovies)))