diff --git a/.all-contributorsrc b/.all-contributorsrc
index bcd2d6ed..3a0faf45 100644
--- a/.all-contributorsrc
+++ b/.all-contributorsrc
@@ -7,181 +7,184 @@
],
"contributors": [
{
- "login": "mpstewart1",
- "name": "Matthew Stewart",
- "avatar_url": "https://avatars.githubusercontent.com/mpstewart1",
- "profile": "https://github.com/mpstewart1",
- "contributions": [
- "doc"
- ]
+ "login": "michael-schnebly",
+ "name": "Michael Schnebly",
+ "avatar_url": "https://avatars.githubusercontent.com/michael-schnebly",
+ "profile": "https://github.com/michael-schnebly",
+ "contributions": []
},
{
"login": "oishib",
"name": "oishib",
"avatar_url": "https://avatars.githubusercontent.com/oishib",
"profile": "https://github.com/oishib",
- "contributions": [
- "doc"
- ]
- },
- {
- "login": "DivyaAmirtharaj",
- "name": "Divya",
- "avatar_url": "https://avatars.githubusercontent.com/DivyaAmirtharaj",
- "profile": "https://github.com/DivyaAmirtharaj",
- "contributions": [
- "doc"
- ]
+ "contributions": []
},
{
- "login": "jessicaquaye",
- "name": "Jessica Quaye",
- "avatar_url": "https://avatars.githubusercontent.com/jessicaquaye",
- "profile": "https://github.com/jessicaquaye",
- "contributions": [
- "doc"
- ]
+ "login": "sophiacho1",
+ "name": "sophiacho1",
+ "avatar_url": "https://avatars.githubusercontent.com/sophiacho1",
+ "profile": "https://github.com/sophiacho1",
+ "contributions": []
},
{
"login": "colbybanbury",
"name": "Colby Banbury",
"avatar_url": "https://avatars.githubusercontent.com/colbybanbury",
"profile": "https://github.com/colbybanbury",
- "contributions": [
- "doc"
- ]
+ "contributions": []
},
{
- "login": "profvjreddi",
- "name": "Vijay Janapa Reddi",
- "avatar_url": "https://avatars.githubusercontent.com/profvjreddi",
- "profile": "https://github.com/profvjreddi",
- "contributions": [
- "doc"
- ]
+ "login": "happyappledog",
+ "name": "happyappledog",
+ "avatar_url": "https://avatars.githubusercontent.com/happyappledog",
+ "profile": "https://github.com/happyappledog",
+ "contributions": []
},
{
- "login": "ShvetankPrakash",
- "name": "Shvetank Prakash",
- "avatar_url": "https://avatars.githubusercontent.com/ShvetankPrakash",
- "profile": "https://github.com/ShvetankPrakash",
- "contributions": [
- "doc"
- ]
+ "login": "jared-ni",
+ "name": "Jared Ni",
+ "avatar_url": "https://avatars.githubusercontent.com/jared-ni",
+ "profile": "https://github.com/jared-ni",
+ "contributions": []
},
{
- "login": "uchendui",
- "name": "Ikechukwu Uchendu",
- "avatar_url": "https://avatars.githubusercontent.com/uchendui",
- "profile": "https://github.com/uchendui",
- "contributions": [
- "doc"
- ]
+ "login": "mpstewart1",
+ "name": "Matthew Stewart",
+ "avatar_url": "https://avatars.githubusercontent.com/mpstewart1",
+ "profile": "https://github.com/mpstewart1",
+ "contributions": []
},
{
- "login": "Mjrovai",
- "name": "Marcelo Rovai",
- "avatar_url": "https://avatars.githubusercontent.com/Mjrovai",
- "profile": "https://github.com/Mjrovai",
- "contributions": [
- "doc"
- ]
+ "login": "marcozennaro",
+ "name": "Marco Zennaro",
+ "avatar_url": "https://avatars.githubusercontent.com/marcozennaro",
+ "profile": "https://github.com/marcozennaro",
+ "contributions": []
},
{
- "login": "BaeHenryS",
- "name": "Henry Bae",
- "avatar_url": "https://avatars.githubusercontent.com/BaeHenryS",
- "profile": "https://github.com/BaeHenryS",
- "contributions": [
- "doc"
- ]
+ "login": "18jeffreyma",
+ "name": "Jeffrey Ma",
+ "avatar_url": "https://avatars.githubusercontent.com/18jeffreyma",
+ "profile": "https://github.com/18jeffreyma",
+ "contributions": []
},
{
- "login": "mmaz",
- "name": "Mark Mazumder",
- "avatar_url": "https://avatars.githubusercontent.com/mmaz",
- "profile": "https://github.com/mmaz",
- "contributions": [
- "doc"
- ]
+ "login": "sjohri20",
+ "name": "sjohri20",
+ "avatar_url": "https://avatars.githubusercontent.com/sjohri20",
+ "profile": "https://github.com/sjohri20",
+ "contributions": []
},
{
"login": "Naeemkh",
"name": "naeemkh",
"avatar_url": "https://avatars.githubusercontent.com/Naeemkh",
"profile": "https://github.com/Naeemkh",
- "contributions": [
- "doc"
- ]
+ "contributions": []
},
{
- "login": "ishapira1",
- "name": "ishapira",
- "avatar_url": "https://avatars.githubusercontent.com/ishapira1",
- "profile": "https://github.com/ishapira1",
- "contributions": [
- "doc"
- ]
+ "login": "AditiR-42",
+ "name": "AditiR_42",
+ "avatar_url": "https://avatars.githubusercontent.com/AditiR-42",
+ "profile": "https://github.com/AditiR-42",
+ "contributions": []
},
{
- "login": "sophiacho1",
- "name": "sophiacho1",
- "avatar_url": "https://avatars.githubusercontent.com/sophiacho1",
- "profile": "https://github.com/sophiacho1",
- "contributions": [
- "doc"
- ]
+ "login": "jessicaquaye",
+ "name": "Jessica Quaye",
+ "avatar_url": "https://avatars.githubusercontent.com/jessicaquaye",
+ "profile": "https://github.com/jessicaquaye",
+ "contributions": []
},
{
- "login": "18jeffreyma",
- "name": "Jeffrey Ma",
- "avatar_url": "https://avatars.githubusercontent.com/18jeffreyma",
- "profile": "https://github.com/18jeffreyma",
- "contributions": [
- "doc"
- ]
+ "login": "ShvetankPrakash",
+ "name": "Shvetank Prakash",
+ "avatar_url": "https://avatars.githubusercontent.com/ShvetankPrakash",
+ "profile": "https://github.com/ShvetankPrakash",
+ "contributions": []
},
{
- "login": "marcozennaro",
- "name": "Marco Zennaro",
- "avatar_url": "https://avatars.githubusercontent.com/marcozennaro",
- "profile": "https://github.com/marcozennaro",
- "contributions": [
- "doc"
- ]
+ "login": "profvjreddi",
+ "name": "Vijay Janapa Reddi",
+ "avatar_url": "https://avatars.githubusercontent.com/profvjreddi",
+ "profile": "https://github.com/profvjreddi",
+ "contributions": []
},
{
- "login": "sjohri20",
- "name": "sjohri20",
- "avatar_url": "https://avatars.githubusercontent.com/sjohri20",
- "profile": "https://github.com/sjohri20",
- "contributions": [
- "doc"
- ]
+ "login": "mmaz",
+ "name": "Mark Mazumder",
+ "avatar_url": "https://avatars.githubusercontent.com/mmaz",
+ "profile": "https://github.com/mmaz",
+ "contributions": []
},
{
"login": "aptl26",
"name": "aptl26",
"avatar_url": "https://avatars.githubusercontent.com/aptl26",
"profile": "https://github.com/aptl26",
- "contributions": [
- "doc"
- ]
+ "contributions": []
+ },
+ {
+ "login": "alxrod",
+ "name": "alxrod",
+ "avatar_url": "https://avatars.githubusercontent.com/alxrod",
+ "profile": "https://github.com/alxrod",
+ "contributions": []
+ },
+ {
+ "login": "BaeHenryS",
+ "name": "Henry Bae",
+ "avatar_url": "https://avatars.githubusercontent.com/BaeHenryS",
+ "profile": "https://github.com/BaeHenryS",
+ "contributions": []
+ },
+ {
+ "login": "DivyaAmirtharaj",
+ "name": "Divya",
+ "avatar_url": "https://avatars.githubusercontent.com/DivyaAmirtharaj",
+ "profile": "https://github.com/DivyaAmirtharaj",
+ "contributions": []
+ },
+ {
+ "login": "uchendui",
+ "name": "Ikechukwu Uchendu",
+ "avatar_url": "https://avatars.githubusercontent.com/uchendui",
+ "profile": "https://github.com/uchendui",
+ "contributions": []
+ },
+ {
+ "login": "Ekhao",
+ "name": "Emil Njor",
+ "avatar_url": "https://avatars.githubusercontent.com/Ekhao",
+ "profile": "https://github.com/Ekhao",
+ "contributions": []
+ },
+ {
+ "login": "Mjrovai",
+ "name": "Marcelo Rovai",
+ "avatar_url": "https://avatars.githubusercontent.com/Mjrovai",
+ "profile": "https://github.com/Mjrovai",
+ "contributions": []
},
{
"login": "jaysonzlin",
"name": "Jayson Lin",
"avatar_url": "https://avatars.githubusercontent.com/jaysonzlin",
"profile": "https://github.com/jaysonzlin",
- "contributions": [
- "doc"
- ]
+ "contributions": []
+ },
+ {
+ "login": "ishapira1",
+ "name": "ishapira",
+ "avatar_url": "https://avatars.githubusercontent.com/ishapira1",
+ "profile": "https://github.com/ishapira1",
+ "contributions": []
}
],
"repoType": "github",
"contributorsPerLine": 5,
"repoHost": "https=//github.com",
"commitConvention": "angular",
- "skipCi": true,
- "commitType": "docs"
+ "skipCi": true
}
\ No newline at end of file
diff --git a/.github/workflows/contributors/update_contributors.py b/.github/workflows/contributors/update_contributors.py
index e9f19509..fd5b0418 100644
--- a/.github/workflows/contributors/update_contributors.py
+++ b/.github/workflows/contributors/update_contributors.py
@@ -5,9 +5,9 @@
from absl import app
import requests
-CONTRIBUTORS_FILE = '.all-contributorsrc'
+CONTRIBUTORS_FILE = ".all-contributorsrc"
-EXCLUDED_USERS = {'web-flow', 'github-actions[bot]', 'mrdragonbear', 'jveejay'}
+EXCLUDED_USERS = {"web-flow", "github-actions[bot]", "mrdragonbear", "jveejay"}
OWNER = "harvard-edge"
REPO = "cs249r_book"
@@ -17,19 +17,19 @@
def main(_):
token = os.environ["GH_TOKEN"]
- headers = {
- "Authorization": f"token {token}"
- }
+ headers = {"Authorization": f"token {token}"}
data = []
- next_page = f'https://api.github.com/repos/{OWNER}/{REPO}/commits?sha={BRANCH}&per_page=100'
+ next_page = (
+ f"https://api.github.com/repos/{OWNER}/{REPO}/commits?sha={BRANCH}&per_page=100"
+ )
last_page = None
while next_page != last_page:
- print(f'Fetching page: {next_page}')
+ print(f"Fetching page: {next_page}")
res = requests.get(next_page, headers=headers)
data.extend(res.json())
- next_page = res.links.get('next', {}).get('url', None)
- last_page = res.links.get('last', {}).get('url', None)
+ next_page = res.links.get("next", {}).get("url", None)
+ last_page = res.links.get("last", {}).get("url", None)
user_to_name_dict = dict()
name_to_user_dict = dict()
@@ -37,24 +37,26 @@ def main(_):
user_full_names_from_api = set()
for node in data:
- commit_info = node.get('commit', None)
- commit_author_info = commit_info.get('author', None)
- commit_commiter_info = commit_info.get('committer', None)
- author_info = node.get('author', None)
- committer_info = node.get('committer', None)
- committer_login_info = committer_info.get('login', None) if committer_info else None
+ commit_info = node.get("commit", None)
+ commit_author_info = commit_info.get("author", None)
+ commit_commiter_info = commit_info.get("committer", None)
+ author_info = node.get("author", None)
+ committer_info = node.get("committer", None)
+ committer_login_info = (
+ committer_info.get("login", None) if committer_info else None
+ )
user_full_name = None
username = None
if commit_author_info:
- user_full_name = commit_author_info['name']
+ user_full_name = commit_author_info["name"]
elif commit_commiter_info:
- user_full_name = commit_commiter_info['name']
+ user_full_name = commit_commiter_info["name"]
if author_info:
- username = author_info['login']
+ username = author_info["login"]
elif committer_login_info:
- username = committer_login_info['login']
+ username = committer_login_info["login"]
if user_full_name:
name_to_user_dict[user_full_name] = username if username else None
@@ -63,25 +65,28 @@ def main(_):
user_to_name_dict[username] = user_full_name if user_full_name else None
users_from_api.add(username)
- print('Users pulled from API: ', users_from_api)
+ print("Users pulled from API: ", users_from_api)
- with open(CONTRIBUTORS_FILE, 'r') as contrib_file:
+ with open(CONTRIBUTORS_FILE, "r") as contrib_file:
existing_contributor_data = json.load(contrib_file)
- existing_contributors = existing_contributor_data['contributors']
+ existing_contributors = existing_contributor_data["contributors"]
existing_contributor_logins = []
for existing_contributor in existing_contributors:
- user_to_name_dict[existing_contributor['login']] = existing_contributor['name']
- existing_contributor_logins.append(existing_contributor['login'])
+ user_to_name_dict[existing_contributor["login"]] = existing_contributor[
+ "name"
+ ]
+ existing_contributor_logins.append(existing_contributor["login"])
existing_contributor_logins_set = set(existing_contributor_logins)
- print('Existing contributors: ', existing_contributor_logins_set)
+ print("Existing contributors: ", existing_contributor_logins_set)
existing_contributor_logins_set -= EXCLUDED_USERS
# All contributors in the file should be in the API
assert existing_contributor_logins_set.issubset(
- users_from_api), 'All contributors in the .all-contributorsrc file should be pulled using the API'
+ users_from_api
+ ), "All contributors in the .all-contributorsrc file should be pulled using the API"
new_contributor_logins = users_from_api - existing_contributor_logins_set
- print('New contributors: ', new_contributor_logins - EXCLUDED_USERS)
+ print("New contributors: ", new_contributor_logins - EXCLUDED_USERS)
result = users_from_api - EXCLUDED_USERS
@@ -89,30 +94,35 @@ def main(_):
projectName=REPO,
projectOwner=OWNER,
files=["contributors.qmd", "README.md"],
- contributors=[dict(login=user,
- name=user_to_name_dict[user] or user,
- # If the user has no full name listed, use their username
- avatar_url=f'https://avatars.githubusercontent.com/{user}',
- profile=f'https://github.com/{user}',
- contributions=['doc'], ) for
- user in result],
-
- repoType='github',
+ contributors=[
+ dict(
+ login=user,
+ name=user_to_name_dict[user] or user,
+ # If the user has no full name listed, use their username
+ avatar_url=f"https://avatars.githubusercontent.com/{user}",
+ profile=f"https://github.com/{user}",
+ # contributions=["doc"],
+ contributions=[],
+ )
+ for user in result
+ ],
+ repoType="github",
contributorsPerLine=5,
repoHost="https=//github.com",
- commitConvention='angular',
+ commitConvention="angular",
skipCi=True,
- commitType="docs"
+ # commitType="docs"
)
print(final_result)
- json_string = json.dumps(final_result,
- indent=4) # The indent parameter is optional, but it formats the output to be more readable
+ json_string = json.dumps(
+ final_result, indent=4
+ ) # The indent parameter is optional, but it formats the output to be more readable
print(json_string)
- with open(CONTRIBUTORS_FILE, 'w') as contrib_file:
+ with open(CONTRIBUTORS_FILE, "w") as contrib_file:
contrib_file.write(json_string)
-if __name__ == '__main__':
+if __name__ == "__main__":
app.run(main)
diff --git a/README.md b/README.md
index d671a594..92334977 100644
--- a/README.md
+++ b/README.md
@@ -88,31 +88,39 @@ quarto render
diff --git a/_quarto.yml b/_quarto.yml
index 42d399fc..cc9504e7 100644
--- a/_quarto.yml
+++ b/_quarto.yml
@@ -81,6 +81,7 @@ book:
- object_detection_fomo.qmd
- kws_feature_eng.qmd
- kws_nicla.qmd
+ - dsp_spectral_features_block.qmd
- motion_classify_ad.qmd
references: references.qmd
diff --git a/ai_for_good.qmd b/ai_for_good.qmd
index 33b946ac..dec411e1 100644
--- a/ai_for_good.qmd
+++ b/ai_for_good.qmd
@@ -100,6 +100,14 @@ A collaborative research team from the University of Khartoum and the ICTP is ex
This portable, self-contained system shows great promise for entomology. The researchers suggest it could revolutionize insect monitoring and vector control strategies in remote areas. By providing cheaper, easier mosquito analytics, TinyML could significantly bolster malaria eradication efforts. Its versatility and minimal power needs make it ideal for field use in isolated, off-grid regions with scarce resources but high disease burden.
+### TinyML Design Contest in Healthcare
+
+The first TinyML contest in healthcare, TDC’22 [@jia2023life], was held in 2022 to motivate participating teams to design AI/ML algorithms for detecting life-threatening ventricular arrhythmias (VAs) and deploy them on Implantable Cardioverter Defibrillators (ICDs). VAs are the main cause of sudden cardiac death (SCD). People at high risk of SCD rely on the ICD to deliver proper and timely defibrillation treatment (i.e., shocking the heart back into normal rhythm) when experiencing life-threatening VAs.
+
+An on-device algorithm for early and timely life-threatening VA detection will increase the chances of survival. The proposed AI/ML algorithm needed to be deployed and executed on an extremely low-power and resource-constrained microcontroller (MCU) (a $10 development board with an ARM Cortex-M4 core at 80 MHz, 256 kB of flash memory and 64 kB of SRAM). The submitted designs were evaluated by metrics measured on the MCU for (1) detection performance; (2) inference latency; and (3) memory occupation by the program of AI/ML algorithms.
+
+The champion, GaTech EIC Lab, obtained 0.972 in $F_\beta$ (F1 score with a higher weight to recall), 1.747 ms in latency and 26.39 kB in memory footprint with a deep neural network. An ICD with an on-device VA detection algorithm was [implanted in a clinical trial](https://youtu.be/vx2gWzAr85A?t=2359).
+
## Science
In many scientific fields, researchers are limited by the quality and resolution of data they can collect. They often must infer the true parameters of interest indirectly, using approximate correlations and models built on sparse data points. This constrains the accuracy of scientific understanding and predictions.
diff --git a/benchmarking.qmd b/benchmarking.qmd
index 6f11a146..294e8a89 100644
--- a/benchmarking.qmd
+++ b/benchmarking.qmd
@@ -35,17 +35,17 @@ When benchmarks are maintained over time, they become instrumental in capturing
Benchmarking has several important goals and objectives that guide its implementation for machine learning systems.
-- **Performance assessment.** This involves evaluating key metrics like the speed, accuracy, and efficiency of a given model. For instance, in a TinyML context, it is crucial to benchmark how quickly a voice assistant can recognize commands, as this evaluates real-time performance.
+* **Performance assessment.** This involves evaluating key metrics like the speed, accuracy, and efficiency of a given model. For instance, in a TinyML context, it is crucial to benchmark how quickly a voice assistant can recognize commands, as this evaluates real-time performance.
-- **Resource evaluation.** This means assessing the model's impact on critical system resources including battery life, memory usage, and computational overhead. A relevant example is comparing the battery drain of two different image recognition algorithms running on a wearable device.
+* **Resource evaluation.** This means assessing the model's impact on critical system resources including battery life, memory usage, and computational overhead. A relevant example is comparing the battery drain of two different image recognition algorithms running on a wearable device.
-- **Validation and verification.** Benchmarking helps ensure the system functions correctly and meets specified requirements. One way is by checking the accuracy of an algorithm, like a heart rate monitor on a smartwatch, against readings from medical-grade equipment as a form of clinical validation.
+* **Validation and verification.** Benchmarking helps ensure the system functions correctly and meets specified requirements. One way is by checking the accuracy of an algorithm, like a heart rate monitor on a smartwatch, against readings from medical-grade equipment as a form of clinical validation.
-- **Competitive analysis.** This enables comparing solutions against competing offerings in the market. For example, benchmarking a custom object detection model versus common tinyML benchmarks like MobileNet and Tiny-YOLO.
+* **Competitive analysis.** This enables comparing solutions against competing offerings in the market. For example, benchmarking a custom object detection model versus common tinyML benchmarks like MobileNet and Tiny-YOLO.
-- **Credibility.** Accurate benchmarks uphold the credibility of AI solutions and the organizations that develop them. They demonstrate a commitment to transparency, honesty, and quality, which is essential in building trust with users and stakeholders.
+* **Credibility.** Accurate benchmarks uphold the credibility of AI solutions and the organizations that develop them. They demonstrate a commitment to transparency, honesty, and quality, which is essential in building trust with users and stakeholders.
-- **Regulation and Standardization**. As the AI industry continues to grow, there is an increasing need for regulation and standardization to ensure that AI solutions are safe, ethical, and effective. Accurate and reliable benchmarks are an essential component of this regulatory framework, as they provide the data and evidence needed to assess compliance with industry standards and legal requirements.
+* **Regulation and Standardization**. As the AI industry continues to grow, there is an increasing need for regulation and standardization to ensure that AI solutions are safe, ethical, and effective. Accurate and reliable benchmarks are an essential component of this regulatory framework, as they provide the data and evidence needed to assess compliance with industry standards and legal requirements.
This chapter will cover the 3 types of benchmarks in AI, the standard metrics, tools, and techniques designers use to optimize their systems, and the challenges and trends in benchmarking.
@@ -115,11 +115,9 @@ Micro-benchmarks in AI are specialized, focusing on the evaluation of distinct c
These types of microbenchmarks include that zoom into very specific operations or components of the AI pipeline, such as the following:
-- Tensor Operations: Libraries like [cuDNN](https://developer.nvidia.com/cudnn) (by NVIDIA) often have benchmarks to measure the performance of individual tensor operations, such as convolutions or matrix multiplications, which are foundational to deep learning computations.
-
-- Activation Functions: Benchmarks that measure the speed and efficiency of various activation functions like ReLU, Sigmoid, or Tanh in isolation.
-
-- Layer Benchmarks: Evaluations of the computational efficiency of distinct neural network layers, such as a LSTM layer or a Transformer block, when operating on standardized input sizes.
+* Tensor Operations: Libraries like [cuDNN](https://developer.nvidia.com/cudnn) (by NVIDIA) often have benchmarks to measure the performance of individual tensor operations, such as convolutions or matrix multiplications, which are foundational to deep learning computations.
+* Activation Functions: Benchmarks that measure the speed and efficiency of various activation functions like ReLU, Sigmoid, or Tanh in isolation.
+* Layer Benchmarks: Evaluations of the computational efficiency of distinct neural network layers, such as a LSTM layer or a Transformer block, when operating on standardized input sizes.
Example: [DeepBench](https://github.com/baidu-research/DeepBench), introduced by Baidu, is a good example of something that asseses the above. DeepBench assesses the performance of basic operations in deep learning models, providing insights into how different hardware platforms handle neural network training and inference.
@@ -129,11 +127,11 @@ Macro-benchmarks provide a holistic view, assessing the end-to-end performance o
Examples: These benchmarks evaluate the AI model:
-- [MLPerf Inference](https://github.com/mlcommons/inference)[@reddi2020mlperf]: An industry-standard set of benchmarks for measuring the performance of machine learning software and hardware. MLPerf has a suite of dedicated benchmarks for specific scales, such as [MLPerf Mobile](https://github.com/mlcommons/mobile_app_open) for mobile class devices and [MLPerf Tiny](https://github.com/mlcommons/tiny), which focuses on microcontrollers and other resource-constrained devices.
+* [MLPerf Inference](https://github.com/mlcommons/inference)[@reddi2020mlperf]: An industry-standard set of benchmarks for measuring the performance of machine learning software and hardware. MLPerf has a suite of dedicated benchmarks for specific scales, such as [MLPerf Mobile](https://github.com/mlcommons/mobile_app_open) for mobile class devices and [MLPerf Tiny](https://github.com/mlcommons/tiny), which focuses on microcontrollers and other resource-constrained devices.
-- [EEMBC's MLMark](https://github.com/eembc/mlmark): A benchmarking suite for evaluating the performance and power efficiency of embedded devices running machine learning workloads. This benchmark provides insights into how different hardware platforms handle tasks like image recognition or audio processing.
+* [EEMBC's MLMark](https://github.com/eembc/mlmark): A benchmarking suite for evaluating the performance and power efficiency of embedded devices running machine learning workloads. This benchmark provides insights into how different hardware platforms handle tasks like image recognition or audio processing.
-- [AI-Benchmark](https://ai-benchmark.com/)[@ignatov2018ai]: A benchmarking tool designed for Android devices, it valuates the performance of AI tasks on mobile devices, encompassing various real-world scenarios like image recognition, face parsing, and optical character recognition.
+* [AI-Benchmark](https://ai-benchmark.com/)[@ignatov2018ai]: A benchmarking tool designed for Android devices, it valuates the performance of AI tasks on mobile devices, encompassing various real-world scenarios like image recognition, face parsing, and optical character recognition.
#### End-to-end Benchmarks
@@ -237,25 +235,25 @@ Training metrics, when viewed from a systems perspective, offer insights that tr
The following metrics are often considered important:
-1. Training Time: The time taken to train a model from scratch until it reaches a satisfactory performance level. It is a direct measure of the computational resources required to train a model. For example, [Google's BERT](https://arxiv.org/abs/1810.04805)[@devlin2018bert] model is a natural language processing model that requires several days to train on a massive corpus of text data using multiple GPUs. The long training time is a significant challenge in terms of resource consumption and cost.
+1. **Training Time:** The time taken to train a model from scratch until it reaches a satisfactory performance level. It is a direct measure of the computational resources required to train a model. For example, [Google's BERT](https://arxiv.org/abs/1810.04805)[@devlin2018bert] model is a natural language processing model that requires several days to train on a massive corpus of text data using multiple GPUs. The long training time is a significant challenge in terms of resource consumption and cost.
-2. Scalability: How well the training process can handle increases in data size or model complexity. Scalability can be assessed by measuring training time, memory usage, and other resource consumption as data size or model complexity increases. [OpenAI's GPT-3](https://arxiv.org/abs/2005.14165)[@brown2020language] model has 175 billion parameters, making it one of the largest language models in existence. Training GPT-3 required extensive engineering efforts to scale up the training process to handle the massive model size. This involved the use of specialized hardware, distributed training, and other techniques to ensure that the model could be trained efficiently.
+2. **Scalability:** How well the training process can handle increases in data size or model complexity. Scalability can be assessed by measuring training time, memory usage, and other resource consumption as data size or model complexity increases. [OpenAI's GPT-3](https://arxiv.org/abs/2005.14165)[@brown2020language] model has 175 billion parameters, making it one of the largest language models in existence. Training GPT-3 required extensive engineering efforts to scale up the training process to handle the massive model size. This involved the use of specialized hardware, distributed training, and other techniques to ensure that the model could be trained efficiently.
-3. Resource Utilization: The extent to which the training process utilizes available computational resources such as CPU, GPU, memory, and disk I/O. High resource utilization can indicate an efficient training process, while low utilization can suggest bottlenecks or inefficiencies. For instance, training a convolutional neural network (CNN) for image classification requires significant GPU resources. Utilizing multi-GPU setups and optimizing the training code for GPU acceleration can greatly improve resource utilization and training efficiency.
+3. **Resource Utilization:** The extent to which the training process utilizes available computational resources such as CPU, GPU, memory, and disk I/O. High resource utilization can indicate an efficient training process, while low utilization can suggest bottlenecks or inefficiencies. For instance, training a convolutional neural network (CNN) for image classification requires significant GPU resources. Utilizing multi-GPU setups and optimizing the training code for GPU acceleration can greatly improve resource utilization and training efficiency.
-4. Memory Consumption: The amount of memory used by the training process. Memory consumption can be a limiting factor for training large models or datasets. As an example, Google researchers faced significant memory consumption challenges when training BERT. The model has hundreds of millions of parameters, which require large amounts of memory to store. The researchers had to develop techniques to reduce memory consumption, such as gradient checkpointing and model parallelism.
+4. **Memory Consumption:** The amount of memory used by the training process. Memory consumption can be a limiting factor for training large models or datasets. As an example, Google researchers faced significant memory consumption challenges when training BERT. The model has hundreds of millions of parameters, which require large amounts of memory to store. The researchers had to develop techniques to reduce memory consumption, such as gradient checkpointing and model parallelism.
-5. Energy Consumption: The amount of energy consumed during the training process. As machine learning models become larger and more complex, energy consumption has become an important consideration. Training large machine learning models can consume significant amounts of energy, leading to a large carbon footprint. For instance, the training of OpenAI's GPT-3 was estimated to have a carbon footprint equivalent to traveling by car for 700,000 kilometers.
+5. **Energy Consumption:** The amount of energy consumed during the training process. As machine learning models become larger and more complex, energy consumption has become an important consideration. Training large machine learning models can consume significant amounts of energy, leading to a large carbon footprint. For instance, the training of OpenAI's GPT-3 was estimated to have a carbon footprint equivalent to traveling by car for 700,000 kilometers.
-6. Throughput: The number of training samples processed per unit time. Higher throughput generally indicates a more efficient training process. When training a recommendation system for an e-commerce platform, the throughput is an important metric to consider. A high throughput ensures that the model can process large volumes of user interaction data in a timely manner, which is crucial for maintaining the relevance and accuracy of the recommendations. But it's also important to understand how to balance throughput with latency bounds. Therefore, often there is a latency-bounded throughput constraint that's imposed on service-level agreements for datacenter application deployments.
+6. **Throughput:** The number of training samples processed per unit time. Higher throughput generally indicates a more efficient training process. When training a recommendation system for an e-commerce platform, the throughput is an important metric to consider. A high throughput ensures that the model can process large volumes of user interaction data in a timely manner, which is crucial for maintaining the relevance and accuracy of the recommendations. But it's also important to understand how to balance throughput with latency bounds. Therefore, often there is a latency-bounded throughput constraint that's imposed on service-level agreements for datacenter application deployments.
-7. Cost: The cost of training a model, which can include both computational and human resources. Cost is an important factor when considering the practicality and feasibility of training large or complex models. The cost of training large language models like GPT-3 is estimated to be in the range of millions of dollars. This cost includes computational resources, electricity, and human resources required for model development and training.
+7. **Cost:** The cost of training a model, which can include both computational and human resources. Cost is an important factor when considering the practicality and feasibility of training large or complex models. The cost of training large language models like GPT-3 is estimated to be in the range of millions of dollars. This cost includes computational resources, electricity, and human resources required for model development and training.
-8. Fault Tolerance and Robustness: The ability of the training process to handle failures or errors without crashing or producing incorrect results. This is important for ensuring the reliability of the training process. In a real-world scenario, where a machine learning model is being trained on a distributed system, network failures or hardware malfunctions can occur. In recent years, for instance, it has become abundantly clear that faults that arise from silent data corruption have emerged as a major issue. A fault-tolerant and robust training process can recover from such failures without compromising the integrity of the model.
+8. **Fault Tolerance and Robustness:** The ability of the training process to handle failures or errors without crashing or producing incorrect results. This is important for ensuring the reliability of the training process. In a real-world scenario, where a machine learning model is being trained on a distributed system, network failures or hardware malfunctions can occur. In recent years, for instance, it has become abundantly clear that faults that arise from silent data corruption have emerged as a major issue. A fault-tolerant and robust training process can recover from such failures without compromising the integrity of the model.
-9. Ease of Use and Flexibility: The ease with which the training process can be set up and used, as well as its flexibility in handling different types of data and models. In companies like Google, efficiency can sometimes be measured in terms of the number of Software Engineer (SWE) years saved since that translates directly to impact. Ease of use and flexibility can reduce the time and effort required to train a model. TensorFlow and PyTorch are popular machine learning frameworks that provide user-friendly interfaces and flexible APIs for building and training machine learning models. These frameworks support a wide range of model architectures and are equipped with tools that simplify the training process.
+9. **Ease of Use and Flexibility:** The ease with which the training process can be set up and used, as well as its flexibility in handling different types of data and models. In companies like Google, efficiency can sometimes be measured in terms of the number of Software Engineer (SWE) years saved since that translates directly to impact. Ease of use and flexibility can reduce the time and effort required to train a model. TensorFlow and PyTorch are popular machine learning frameworks that provide user-friendly interfaces and flexible APIs for building and training machine learning models. These frameworks support a wide range of model architectures and are equipped with tools that simplify the training process.
-10. Reproducibility: The ability to reproduce the results of the training process. Reproducibility is important for verifying the correctness and validity of a model. However, there are often variations due to stochastic network characteristics and this makes it hard to reproduce the precise behavior of applications being trained, and this can present a challenge for benchmarking.
+10. **Reproducibility:** The ability to reproduce the results of the training process. Reproducibility is important for verifying the correctness and validity of a model. However, there are often variations due to stochastic network characteristics and this makes it hard to reproduce the precise behavior of applications being trained, and this can present a challenge for benchmarking.
By benchmarking for these types of metrics, we can obtain a comprehensive view of the performance and efficiency of the training process from a systems perspective, which can help identify areas for improvement and ensure that resources are used effectively.
@@ -263,20 +261,14 @@ By benchmarking for these types of metrics, we can obtain a comprehensive view o
Selecting a handful of representative tasks for benchmarking machine learning systems is challenging because machine learning is applied to a diverse range of domains, each with its own unique characteristics and requirements. Here are some of the challenges faced in selecting representative tasks:
-1. Diversity of Applications: Machine learning is used in numerous fields such as healthcare, finance, natural language processing, computer vision, and many more. Each field has specific tasks that may not be representative of other fields. For example, image classification tasks in computer vision may not be relevant to financial fraud detection.
-
-2. Variability in Data Types and Quality: Different tasks require different types of data, such as text, images, videos, or numerical data. The quality and availability of data can vary greatly between tasks, making it difficult to select tasks that are representative of the general challenges faced in machine learning.
-
-3. Task Complexity and Difficulty: The complexity of tasks varies greatly, with some tasks being relatively straightforward, while others are highly complex and require sophisticated models and techniques. Selecting representative tasks that cover the range of complexities encountered in machine learning is a challenge.
-
-4. Ethical and Privacy Concerns: Some tasks may involve sensitive or private data, such as medical records or personal information. These tasks may have ethical and privacy concerns that need to be addressed, which can make them less suitable as representative tasks for benchmarking.
-
-5. Scalability and Resource Requirements: Different tasks may have different scalability and resource requirements. Some tasks may require extensive computational resources, while others can be performed with minimal resources. Selecting tasks that are representative of the general resource requirements in machine learning is difficult.
-
-6. Evaluation Metrics: The metrics used to evaluate the performance of machine learning models vary between tasks. Some tasks may have well-established evaluation metrics, while others may lack clear or standardized metrics. This can make it challenging to compare performance across different tasks.
-
-7. Generalizability of Results: The results obtained from benchmarking on a specific task may not be generalizable to other tasks. This means that the performance of a machine learning system on a selected task may not be indicative of its performance on other tasks.
-
+1. **Diversity of Applications:** Machine learning is used in numerous fields such as healthcare, finance, natural language processing, computer vision, and many more. Each field has specific tasks that may not be representative of other fields. For example, image classification tasks in computer vision may not be relevant to financial fraud detection.
+2. **Variability in Data Types and Quality:** Different tasks require different types of data, such as text, images, videos, or numerical data. The quality and availability of data can vary greatly between tasks, making it difficult to select tasks that are representative of the general challenges faced in machine learning.
+3. **Task Complexity and Difficulty:** The complexity of tasks varies greatly, with some tasks being relatively straightforward, while others are highly complex and require sophisticated models and techniques. Selecting representative tasks that cover the range of complexities encountered in machine learning is a challenge.
+4. **Ethical and Privacy Concerns:** Some tasks may involve sensitive or private data, such as medical records or personal information. These tasks may have ethical and privacy concerns that need to be addressed, which can make them less suitable as representative tasks for benchmarking.
+5. **Scalability and Resource Requirements:** Different tasks may have different scalability and resource requirements. Some tasks may require extensive computational resources, while others can be performed with minimal resources. Selecting tasks that are representative of the general resource requirements in machine learning is difficult.
+6. **Evaluation Metrics:** The metrics used to evaluate the performance of machine learning models vary between tasks. Some tasks may have well-established evaluation metrics, while others may lack clear or standardized metrics. This can make it challenging to compare performance across different tasks.
+7. **Generalizability of Results:** The results obtained from benchmarking on a specific task may not be generalizable to other tasks. This means that the performance of a machine learning system on a selected task may not be indicative of its performance on other tasks.
+8.
It is important to carefully consider these factors when designing benchmarks to ensure that they are meaningful and relevant to the diverse range of tasks encountered in machine learning.
#### Benchmarks
@@ -285,55 +277,45 @@ Here are some original works that laid the fundamental groundwork for developing
*[MLPerf Training Benchmark](https://github.com/mlcommons/training)*
-MLPerf is a suite of benchmarks designed to measure the performance of machine learning hardware, software, and services. The MLPerf Training benchmark[@mattson2020mlperf] focuses on the time it takes to train models to a target quality metric. It includes a diverse set of workloads, such as image classification, object detection, translation, and reinforcement learning.
+MLPerf is a suite of benchmarks designed to measure the performance of machine learning hardware, software, and services. The MLPerf Training benchmark [@mattson2020mlperf] focuses on the time it takes to train models to a target quality metric. It includes a diverse set of workloads, such as image classification, object detection, translation, and reinforcement learning.
Metrics:
-- Training time to target quality
-
-- Throughput (examples per second)
-
-- Resource utilization (CPU, GPU, memory, disk I/O)
+* Training time to target quality
+* Throughput (examples per second)
+* Resource utilization (CPU, GPU, memory, disk I/O)
*[DAWNBench](https://dawn.cs.stanford.edu/benchmark/)*
-DAWNBench[@coleman2017dawnbench] is a benchmark suite that focuses on end-to-end deep learning training time and inference performance. It includes common tasks such as image classification and question answering.
+DAWNBench [@coleman2017dawnbench] is a benchmark suite that focuses on end-to-end deep learning training time and inference performance. It includes common tasks such as image classification and question answering.
Metrics:
-- Time to train to target accuracy
-
-- Inference latency
-
-- Cost (in terms of cloud compute and storage resources)
+* Time to train to target accuracy
+* Inference latency
+* Cost (in terms of cloud compute and storage resources)
*[Fathom](https://github.com/rdadolf/fathom)*
-Fathom[@adolf2016fathom] is a benchmark from Harvard University that includes a diverse set of workloads to evaluate the performance of deep learning models. It includes common tasks such as image classification, speech recognition, and language modeling.
+Fathom [@adolf2016fathom] is a benchmark from Harvard University that includes a diverse set of workloads to evaluate the performance of deep learning models. It includes common tasks such as image classification, speech recognition, and language modeling.
Metrics:
-- Operations per second (to measure computational efficiency)
-
-- Time to completion for each workload
-
-- Memory bandwidth
+* Operations per second (to measure computational efficiency)
+* Time to completion for each workload
+* Memory bandwidth
*Example Use Case*
Consider a scenario where we want to benchmark the training of an image classification model on a specific hardware platform.
-1. Task: The task is to train a convolutional neural network (CNN) for image classification on the CIFAR-10 dataset.
-
-2. Benchmark: We can use the MLPerf Training benchmark for this task. It includes an image classification workload that is relevant to our task.
-
-3. Metrics: We will measure the following metrics:
+1. ** Task:** The task is to train a convolutional neural network (CNN) for image classification on the CIFAR-10 dataset.
+2. ** Benchmark:** We can use the MLPerf Training benchmark for this task. It includes an image classification workload that is relevant to our task.
+3. ** Metrics:** We will measure the following metrics:
-- Training time to reach a target accuracy of 90%.
-
-- Throughput in terms of images processed per second.
-
-- GPU and CPU utilization during training.
+* Training time to reach a target accuracy of 90%.
+* Throughput in terms of images processed per second.
+* GPU and CPU utilization during training.
By measuring these metrics, we can assess the performance and efficiency of the training process on the selected hardware platform. This information can then be used to identify potential bottlenecks or areas for improvement.
@@ -353,39 +335,39 @@ Finally, ensuring that the model's predictions are not only accurate but also co
#### Metrics
-1. Accuracy: Accuracy is one of the most vital metrics when benchmarking machine learning models, quantifying the proportion of correct predictions made by the model compared to the true values or labels. For example, in the case of a spam detection model that can correctly classify 95 out of 100 email messages as spam or not spam, the accuracy of this model would be calculated as 95%.
+1. **Accuracy:** Accuracy is one of the most vital metrics when benchmarking machine learning models, quantifying the proportion of correct predictions made by the model compared to the true values or labels. For example, in the case of a spam detection model that can correctly classify 95 out of 100 email messages as spam or not spam, the accuracy of this model would be calculated as 95%.
-2. Latency: Latency is a performance metric that calculates the time lag or delay occurring between the receipt of an input and the production of the corresponding output by the machine learning system. An example that clearly depicts latency is a real-time translation application; if there exists a half-second delay from the moment a user inputs a sentence to the time the translated text is displayed by the app, then the system's latency is 0.5 seconds.
+2. **Latency:** Latency is a performance metric that calculates the time lag or delay occurring between the receipt of an input and the production of the corresponding output by the machine learning system. An example that clearly depicts latency is a real-time translation application; if there exists a half-second delay from the moment a user inputs a sentence to the time the translated text is displayed by the app, then the system's latency is 0.5 seconds.
-3. Latency-Bounded Throughput: Latency-bounded throughput is a valuable metric that combines the aspects of latency and throughput, measuring the maximum throughput of a system while still meeting a specified latency constraint. For example, in a video streaming application that utilizes a machine learning model to automatically generate and display subtitles, latency-bounded throughput would measure how many video frames the system can process per second (throughput) while ensuring that the subtitles are displayed with no more than a 1-second delay (latency). This metric is particularly important in real-time applications where meeting latency requirements is crucial to the user experience.
+3. **Latency-Bounded Throughput:** Latency-bounded throughput is a valuable metric that combines the aspects of latency and throughput, measuring the maximum throughput of a system while still meeting a specified latency constraint. For example, in a video streaming application that utilizes a machine learning model to automatically generate and display subtitles, latency-bounded throughput would measure how many video frames the system can process per second (throughput) while ensuring that the subtitles are displayed with no more than a 1-second delay (latency). This metric is particularly important in real-time applications where meeting latency requirements is crucial to the user experience.
-4. Throughput: Throughput assesses the system's capacity by measuring the total number of inferences or predictions a machine learning model can handle within a specific unit of time. Consider a speech recognition system that employs a Recurrent Neural Network (RNN) as its underlying model; if this system is capable of processing and understanding 50 different audio clips in a minute, then its throughput rate stands at 50 clips per minute.
+4. **Throughput:** Throughput assesses the system's capacity by measuring the total number of inferences or predictions a machine learning model can handle within a specific unit of time. Consider a speech recognition system that employs a Recurrent Neural Network (RNN) as its underlying model; if this system is capable of processing and understanding 50 different audio clips in a minute, then its throughput rate stands at 50 clips per minute.
-5. Inference Time: Inference time is a crucial metric that measures the duration a machine learning system, such as a Convolutional Neural Network (CNN) used in image recognition tasks, takes to process an input and generate a prediction or output. For instance, if a CNN takes approximately 2 milliseconds to accurately identify and label a cat within a given photo, then its inference time is said to be 2 milliseconds.
+5. **Inference Time:** Inference time is a crucial metric that measures the duration a machine learning system, such as a Convolutional Neural Network (CNN) used in image recognition tasks, takes to process an input and generate a prediction or output. For instance, if a CNN takes approximately 2 milliseconds to accurately identify and label a cat within a given photo, then its inference time is said to be 2 milliseconds.
-6. Energy Efficiency: Energy efficiency is a metric that determines the amount of energy consumed by the machine learning model to perform a single inference. A prime example of this would be a natural language processing model built on a Transformer network architecture; if it utilizes 0.1 Joules of energy to translate a sentence from English to French, its energy efficiency is measured at 0.1 Joules per inference.
+6. **Energy Efficiency:** Energy efficiency is a metric that determines the amount of energy consumed by the machine learning model to perform a single inference. A prime example of this would be a natural language processing model built on a Transformer network architecture; if it utilizes 0.1 Joules of energy to translate a sentence from English to French, its energy efficiency is measured at 0.1 Joules per inference.
-7. Memory Usage: Memory usage quantifies the volume of RAM needed by a machine learning model to carry out inference tasks. A relevant example to illustrate this would be a face recognition system that is based on a CNN; if such a system requires 150 MB of RAM to process and recognize faces within an image, then its memory usage is 150 MB.
+7. **Memory Usage:** Memory usage quantifies the volume of RAM needed by a machine learning model to carry out inference tasks. A relevant example to illustrate this would be a face recognition system that is based on a CNN; if such a system requires 150 MB of RAM to process and recognize faces within an image, then its memory usage is 150 MB.
#### Tasks
By and large, the challenges in picking representative tasks for benchmarking inference machine learning systems are somewhat of the same taxonomy as what we have provided for training. Nevertheless, to be pedantic, let's discuss those in the context of inference machine learning systems.
-1. Diversity of Applications: Inference machine learning is employed across numerous domains such as healthcare, finance, entertainment, security, and more. Each domain has its unique tasks, and what's representative in one domain might not be in another. For example, an inference task for predicting stock prices in the financial domain might not be representative of image recognition tasks in the medical domain.
+1. **Diversity of Applications:** Inference machine learning is employed across numerous domains such as healthcare, finance, entertainment, security, and more. Each domain has its unique tasks, and what's representative in one domain might not be in another. For example, an inference task for predicting stock prices in the financial domain might not be representative of image recognition tasks in the medical domain.
-2. Variability in Data Types: Different inference tasks require different types of data -- text, images, videos, numerical data, etc. Ensuring that benchmarks address the wide variety of data types used in real-world applications is challenging. For example, voice recognition systems process audio data, which is vastly different from the visual data processed by facial recognition systems.
+2. **Variability in Data Types:** Different inference tasks require different types of data -- text, images, videos, numerical data, etc. Ensuring that benchmarks address the wide variety of data types used in real-world applications is challenging. For example, voice recognition systems process audio data, which is vastly different from the visual data processed by facial recognition systems.
-3. Task Complexity: The complexity of inference tasks can differ immensely, from basic classification tasks to intricate tasks requiring state-of-the-art models. For example, differentiating between two categories (binary classification) is typically simpler than detecting hundreds of object types in a crowded scene.
+3. **Task Complexity:** The complexity of inference tasks can differ immensely, from basic classification tasks to intricate tasks requiring state-of-the-art models. For example, differentiating between two categories (binary classification) is typically simpler than detecting hundreds of object types in a crowded scene.
-4. Real-time Requirements: Some applications demand immediate or real-time responses, while others may allow for some delay. In autonomous driving, real-time object detection and decision-making are paramount, whereas a recommendation engine for a shopping website might tolerate slight delays.
+4. **Real-time Requirements:** Some applications demand immediate or real-time responses, while others may allow for some delay. In autonomous driving, real-time object detection and decision-making are paramount, whereas a recommendation engine for a shopping website might tolerate slight delays.
-5. Scalability Concerns: Given the varied scale of applications, from edge devices to cloud-based servers, tasks must represent the diverse computational environments where inference occurs. For example, an inference task running on a smartphone's limited resources is quite different from one running on a powerful cloud server.
+5. **Scalability Concerns:** Given the varied scale of applications, from edge devices to cloud-based servers, tasks must represent the diverse computational environments where inference occurs. For example, an inference task running on a smartphone's limited resources is quite different from one running on a powerful cloud server.
-6. Evaluation Metrics Diversity: Depending on the task, the metrics to evaluate performance can differ significantly. Finding a common ground or universally accepted metric for diverse tasks is a challenge. For example, precision and recall might be vital for a medical diagnosis task, whereas throughput (inferences per second) might be more crucial for video processing tasks.
+6. **Evaluation Metrics Diversity:** Depending on the task, the metrics to evaluate performance can differ significantly. Finding a common ground or universally accepted metric for diverse tasks is a challenge. For example, precision and recall might be vital for a medical diagnosis task, whereas throughput (inferences per second) might be more crucial for video processing tasks.
-7. Ethical and Privacy Concerns: Especially in sensitive areas like facial recognition or personal data processing, there are concerns related to ethics and privacy. These concerns can impact the selection and nature of tasks used for benchmarking. For example, using real-world facial data for benchmarking can raise privacy issues, whereas synthetic data might not replicate real-world challenges.
+7. **Ethical and Privacy Concerns:** Especially in sensitive areas like facial recognition or personal data processing, there are concerns related to ethics and privacy. These concerns can impact the selection and nature of tasks used for benchmarking. For example, using real-world facial data for benchmarking can raise privacy issues, whereas synthetic data might not replicate real-world challenges.
-8. Hardware Diversity: With a wide range of devices from GPUs, CPUs, TPUs, to custom ASICs used for inference, ensuring that tasks are representative across varied hardware is challenging. For example, a task optimized for inference on a GPU might perform sub-optimally on an edge device.
+8. **Hardware Diversity:** With a wide range of devices from GPUs, CPUs, TPUs, to custom ASICs used for inference, ensuring that tasks are representative across varied hardware is challenging. For example, a task optimized for inference on a GPU might perform sub-optimally on an edge device.
#### Benchmarks
@@ -397,15 +379,11 @@ MLPerf Inference is a comprehensive suite of benchmarks that assess the performa
Metrics:
-- Inference time
-
-- Latency
-
-- Throughput
-
-- Accuracy
-
-- Energy consumption
+* Inference time
+* Latency
+* Throughput
+* Accuracy
+* Energy consumption
*[AI Benchmark](https://ai-benchmark.com/)*
@@ -413,15 +391,11 @@ AI Benchmark is a benchmarking tool that evaluates the performance of AI and mac
Metrics:
-- Inference time
-
-- Latency
-
-- Energy consumption
-
-- Memory usage
-
-- Throughput
+* Inference time
+* Latency
+* Energy consumption
+* Memory usage
+* Throughput
*[OpenVINO™ toolkit](https://www.intel.com/content/www/us/en/developer/tools/openvino-toolkit/overview.html)*
@@ -429,13 +403,10 @@ OpenVINO™ toolkit provides a benchmark tool to measure the performance of deep
Metrics:
-- Inference time
-
-- Throughput
-
-- Latency
-
-- CPU and GPU utilization
+* Inference time
+* Throughput
+* Latency
+* CPU and GPU utilization
*Example Use Case*
@@ -447,13 +418,10 @@ Benchmark: We can use the AI Benchmark for this task as it focuses on evaluating
Metrics: We will measure the following metrics:
-- Inference time to process each video frame
-
-- Latency to generate the bounding boxes for detected objects
-
-- Energy consumption during the inference process
-
-- Throughput in terms of video frames processed per second
+* Inference time to process each video frame
+* Latency to generate the bounding boxes for detected objects
+* Energy consumption during the inference process
+* Throughput in terms of video frames processed per second
By measuring these metrics, we can assess the performance of the object detection model on the edge device and identify any potential bottlenecks or areas for optimization to enhance real-time processing capabilities.
@@ -489,21 +457,17 @@ Baseline submissions are critical for contextualizing results and acting as a re
While benchmarking provides a structured methodology for performance evaluation in complex domains like artificial intelligence and computing, the process also poses several challenges. If not properly addressed, these challenges can undermine the credibility and accuracy of benchmarking results. Some of the predominant difficulties faced in benchmarking include the following:
-- Incomplete problem coverage - Benchmark tasks may not fully represent the problem space. For instance, common image classification datasets like [CIFAR-10](https://www.cs.toronto.edu/~kriz/cifar.html) have limited diversity in image types. Algorithms tuned for such benchmarks may fail to generalize well to real-world datasets.
-
-- Statistical insignificance - Benchmarks must have enough trials and data samples to produce statistically significant results. For example, benchmarking an OCR model on only a few text scans may not adequately capture its true error rates.
-
-- Limited reproducibility - Varying hardware, software versions, codebases and other factors can reduce reproducibility of benchmark results. MLPerf addresses this by providing reference implementations and environment specification.
-
-- Misalignment with end goals - Benchmarks focusing only on speed or accuracy metrics may misalign with real-world objectives like cost and power efficiency. Benchmarks must reflect all critical performance axes.
-
-- Rapid staleness - Due to the fast pace of advancements in AI and computing, benchmarks and their datasets can become outdated quickly. Maintaining up-to-date benchmarks is thus a persistent challenge.
-
+* Incomplete problem coverage - Benchmark tasks may not fully represent the problem space. For instance, common image classification datasets like [CIFAR-10](https://www.cs.toronto.edu/~kriz/cifar.html) have limited diversity in image types. Algorithms tuned for such benchmarks may fail to generalize well to real-world datasets.
+* Statistical insignificance - Benchmarks must have enough trials and data samples to produce statistically significant results. For example, benchmarking an OCR model on only a few text scans may not adequately capture its true error rates.
+* Limited reproducibility - Varying hardware, software versions, codebases and other factors can reduce reproducibility of benchmark results. MLPerf addresses this by providing reference implementations and environment specification.
+* Misalignment with end goals - Benchmarks focusing only on speed or accuracy metrics may misalign with real-world objectives like cost and power efficiency. Benchmarks must reflect all critical performance axes.
+* Rapid staleness - Due to the fast pace of advancements in AI and computing, benchmarks and their datasets can become outdated quickly. Maintaining up-to-date benchmarks is thus a persistent challenge.
+*
But of all these, perhaps the most important challenge is dealing with benchmark engineering.
#### Hardware Lottery
-The ["hardware lottery"](https://arxiv.org/abs/2009.06489) in benchmarking machine learning systems refers to the situation where the success or efficiency of a machine learning model is significantly influenced by the compatibility of the model with the underlying hardware[@chu2021discovering]. In other words, some models perform exceptionally well because they are a good fit for the particular characteristics or capabilities of the hardware on which they are run, rather than because they are intrinsically superior models. Unfortunately, the hardware used is often omitted from papers or given only brief mentions, making reproducing results difficult if not impossible.
+The ["hardware lottery"](https://arxiv.org/abs/2009.06489) in benchmarking machine learning systems refers to the situation where the success or efficiency of a machine learning model is significantly influenced by the compatibility of the model with the underlying hardware [@chu2021discovering]. In other words, some models perform exceptionally well because they are a good fit for the particular characteristics or capabilities of the hardware on which they are run, rather than because they are intrinsically superior models. Unfortunately, the hardware used is often omitted from papers or given only brief mentions, making reproducing results difficult if not impossible.
For instance, certain machine learning models may be designed and optimized to take advantage of parallel processing capabilities of specific hardware accelerators, such as Graphics Processing Units (GPUs) or Tensor Processing Units (TPUs). As a result, these models might show superior performance when benchmarked on such hardware, compared to other models that are not optimized for the hardware.
@@ -511,7 +475,7 @@ For example, a 2018 paper introduced a new convolutional neural network architec
The "hardware lottery" can introduce challenges and biases in benchmarking machine learning systems, as the performance of the model is not solely dependent on the model's architecture or algorithm, but also on the compatibility and synergies with the underlying hardware. This can make it difficult to fairly compare different models and to identify the best model based on its intrinsic merits. It can also lead to a situation where the community converges on models that are a good fit for the popular hardware of the day, potentially overlooking other models that might be superior but are not compatible with the current hardware trends.
-[Hardware Lottery](./images/benchmarking/hardware_lottery.png)
+
#### Benchmark Engineering
@@ -598,15 +562,15 @@ While the above examples primarily focus on image datasets, there have been sign
Today, we have a plethora of datasets spanning various domains, including healthcare, finance, social sciences, and more. The following characteristics are how we can taxonomiize the space and growth of machine learning datasets that fuel model development.
-1. Diversity of Data Sets: The variety of data sets available to researchers and engineers has expanded dramatically over the years, covering a wide range of fields, including natural language processing, image recognition, and more. This diversity has fueled the development of specialized machine learning models tailored to specific tasks, such as translation, speech recognition, and facial recognition.
+1. **Diversity of Data Sets:** The variety of data sets available to researchers and engineers has expanded dramatically over the years, covering a wide range of fields, including natural language processing, image recognition, and more. This diversity has fueled the development of specialized machine learning models tailored to specific tasks, such as translation, speech recognition, and facial recognition.
-2. Volume of Data: The sheer volume of data that has become available in the digital age has also played a crucial role in advancing machine learning models. Large data sets enable models to capture the complexity and nuances of real-world phenomena, leading to more accurate and reliable predictions.
+2. **Volume of Data:** The sheer volume of data that has become available in the digital age has also played a crucial role in advancing machine learning models. Large data sets enable models to capture the complexity and nuances of real-world phenomena, leading to more accurate and reliable predictions.
-3. Quality and Cleanliness of Data: The quality of data is another critical factor that influences the performance of machine learning models. Clean, well-labeled, and unbiased data sets are essential for training models that are robust and fair.
+3. **Quality and Cleanliness of Data:** The quality of data is another critical factor that influences the performance of machine learning models. Clean, well-labeled, and unbiased data sets are essential for training models that are robust and fair.
-4. Open Access to Data: The availability of open-access data sets has also contributed significantly to the progress in machine learning. Open data allows researchers from around the world to collaborate, share insights, and build upon each other's work, leading to faster innovation and development of more advanced models.
+4. **Open Access to Data:** The availability of open-access data sets has also contributed significantly to the progress in machine learning. Open data allows researchers from around the world to collaborate, share insights, and build upon each other's work, leading to faster innovation and development of more advanced models.
-5. Ethics and Privacy Concerns: As data sets continue to grow in size and complexity, ethical considerations and privacy concerns become increasingly important. There is an ongoing debate about the balance between leveraging data for machine learning advancements and protecting individuals' privacy rights.
+5. **Ethics and Privacy Concerns:** As data sets continue to grow in size and complexity, ethical considerations and privacy concerns become increasingly important. There is an ongoing debate about the balance between leveraging data for machine learning advancements and protecting individuals' privacy rights.
The development of machine learning models is heavily reliant on the availability of diverse, large, high-quality, and open-access data sets. As we move forward, addressing the ethical considerations and privacy concerns associated with the use of large data sets is crucial to ensure that machine learning technologies benefit society as a whole. There is a growing awareness that data acts as the rocket fuel for machine learning, driving and fueling the development of machine learning models. Consequently, an increasing amount of focus is being placed on the development of the data sets themselves. We will explore this in further detail in the data benchmarking section.
@@ -690,11 +654,11 @@ Lastly, the contributions of academic and research institutions cannot be overst
As machine learning models become more sophisticated, so do the benchmarks required to accurately assess them. There are several emerging benchmarks and datasets that are gaining popularity due to their ability to evaluate models in more complex and realistic scenarios:
-**Multimodal Datasets:** These datasets contain multiple types of data, such as text, images, and audio, to better represent real-world situations. An example is the VQA (Visual Question Answering) dataset[@antol2015vqa], where models are tested on their ability to answer text-based questions about images.
+**Multimodal Datasets:** These datasets contain multiple types of data, such as text, images, and audio, to better represent real-world situations. An example is the VQA (Visual Question Answering) dataset [@antol2015vqa], where models are tested on their ability to answer text-based questions about images.
**Fairness and Bias Evaluation:** There is an increasing focus on creating benchmarks that assess the fairness and bias of machine learning models. Examples include the [AI Fairness 360](https://ai-fairness-360.org/) toolkit, which offers a comprehensive set of metrics and datasets for evaluating bias in models.
-**Out-of-Distribution Generalization**: Testing how well models perform on data that is different from the original training distribution. This evaluates the model's ability to generalize to new, unseen data. Example benchmarks are Wilds[@koh2021wilds], RxRx, and ANC-Bench.
+**Out-of-Distribution Generalization**: Testing how well models perform on data that is different from the original training distribution. This evaluates the model's ability to generalize to new, unseen data. Example benchmarks are Wilds [@koh2021wilds], RxRx, and ANC-Bench.
**Adversarial Robustness:** Evaluating model performance under adversarial attacks or perturbations to the input data. This tests the model's robustness. Example benchmarks are ImageNet-A[@hendrycks2021natural], ImageNet-C[@xie2020adversarial], and CIFAR-10.1.
@@ -758,7 +722,7 @@ The shift towards data-centric AI represents a significant paradigm shift. By pr
### Benchmarking Data
-Data benchmarking aims to evaluate common issues in datasets, such as identifying label errors, noisy features, representation imbalance (for example, out of the 1000 classes in Imagenet-1K, there are over 100 categories which are just types of dogs), class imbalance (where some classes have many more samples than others), whether models trained on a given dataset can generalize to out-of-distribution features, or what types of biases might exist in a given dataset[@gaviria2022dollar]. In its simplest form, data benchmarking aims to improve accuracy on a test set by removing noisy or mislabeled training samples while keeping the model architecture fixed. Recent competitions in data benchmarking have invited participants to submit novel augmentation strategies and active learning techniques.
+Data benchmarking aims to evaluate common issues in datasets, such as identifying label errors, noisy features, representation imbalance (for example, out of the 1000 classes in Imagenet-1K, there are over 100 categories which are just types of dogs), class imbalance (where some classes have many more samples than others), whether models trained on a given dataset can generalize to out-of-distribution features, or what types of biases might exist in a given dataset [@gaviria2022dollar]. In its simplest form, data benchmarking aims to improve accuracy on a test set by removing noisy or mislabeled training samples while keeping the model architecture fixed. Recent competitions in data benchmarking have invited participants to submit novel augmentation strategies and active learning techniques.
Data-centric techniques continue to gain attention in benchmarking, especially as foundation models are increasingly trained on self-supervised objectives. Compared to smaller datasets like Imagenet-1K, massive datasets commonly used in self-supervised learning such as Common Crawl, OpenImages, and LAION-5B contain an order of magnitude higher amounts of noise, duplicates, bias, and potentially offensive data.
@@ -774,17 +738,12 @@ Improving data quality can be a useful method to significantly impact machine le
There are several approaches that can be taken to improve data quality. These methods include and are not limited to the following:
-- Data Cleaning: This involves handling missing values, correcting errors, and removing outliers. Clean data ensures that the model is not learning from noise or inaccuracies.
-
-- Data Interpretability and Explainability: Common techniques include LIME [@ribeiro2016should] which provides insight into the decision boundaries of classifiers, and Shapley values [@lundberg2017unified] which estimate the importance of individual samples in contributing to a model's predictions.
-
-- Feature Engineering: Transforming or creating new features can significantly improve model performance by providing more relevant information for learning.
-
-- Data Augmentation: Augmenting data by creating new samples through various transformations can help improve model robustness and generalization.
-
-- Active Learning: This is a semi-supervised learning approach where the model actively queries a human oracle to label the most informative samples [@coleman2022similarity]. This ensures that the model is trained on the most relevant data.
-
-- Dimensionality Reduction: Techniques like PCA can be used to reduce the number of features in a dataset, thereby reducing complexity and training time.
+* **Data Cleaning:** This involves handling missing values, correcting errors, and removing outliers. Clean data ensures that the model is not learning from noise or inaccuracies.
+* **Data Interpretability and Explainability:** Common techniques include LIME [@ribeiro2016should] which provides insight into the decision boundaries of classifiers, and Shapley values [@lundberg2017unified] which estimate the importance of individual samples in contributing to a model's predictions.
+* **Feature Engineering:** Transforming or creating new features can significantly improve model performance by providing more relevant information for learning.
+* **Data Augmentation:** Augmenting data by creating new samples through various transformations can help improve model robustness and generalization.
+* **Active Learning:** This is a semi-supervised learning approach where the model actively queries a human oracle to label the most informative samples [@coleman2022similarity]. This ensures that the model is trained on the most relevant data.
+* Dimensionality Reduction: Techniques like PCA can be used to reduce the number of features in a dataset, thereby reducing complexity and training time.
There are many other methods in the wild. But the goal is the same. By refining the dataset and ensuring it is of the highest quality, we can directly reduce the training time required for models to converge. However, achieving this requires the development and implementation of sophisticated methods, algorithms, and techniques that can clean, preprocess, and augment data while retaining the most informative samples. This is an ongoing challenge that will require continued research and innovation in the field of machine learning.
diff --git a/contributors.qmd b/contributors.qmd
index cd599f9e..ca001269 100644
--- a/contributors.qmd
+++ b/contributors.qmd
@@ -2,37 +2,106 @@
We extend our sincere thanks to the diverse group of individuals who have generously contributed their expertise, insights, and time to enhance both the content and codebase of this project. Below you will find a list of all contributors. If you would like to contribute to this project, please see our [GitHub](https://github.com/harvard-edge/cs249r_book) page.
+
+
+
+
@@ -40,4 +109,18 @@ We extend our sincere thanks to the diverse group of individuals who have genero
-
\ No newline at end of file
+
+
+
+
\ No newline at end of file
diff --git a/dsp_spectral_features_block.qmd b/dsp_spectral_features_block.qmd
new file mode 100644
index 00000000..64126027
--- /dev/null
+++ b/dsp_spectral_features_block.qmd
@@ -0,0 +1,613 @@
+# DSP - Spectral Features {.unnumbered}
+
+## Introduction
+
+TinyML projects related to motion (or vibration) involve data from IMUs (usually **accelerometers** and **gyroscopes**). These time-series type datasets should be preprocessed before inputting them into a Machine Learning model training, which is a challenging area for embedded machine learning. Still, Edge Impulse helps overcome this complexity with its digital signal processing (DSP) preprocessing step and, more specifically, the [Spectral Features Block](https://docs.edgeimpulse.com/docs/edge-impulse-studio/processing-blocks/spectral-features) for Inertial sensors.
+
+But how does it work under the hood? Let's dig into it.
+
+## Extracting Features Review
+
+Extracting features from a dataset captured with inertial sensors, such as accelerometers, involves processing and analyzing the raw data. Accelerometers measure the acceleration of an object along one or more axes (typically three, denoted as X, Y, and Z). These measurements can be used to understand various aspects of the object's motion, such as movement patterns and vibrations. Here's a high-level overview of the process:
+
+**Data collection**: First, we need to gather data from the accelerometers. Depending on the application, data may be collected at different sampling rates. It's essential to ensure that the sampling rate is high enough to capture the relevant dynamics of the studied motion (the sampling rate should be at least double the maximum relevant frequency present in the signal).
+
+**Data preprocessing**: Raw accelerometer data can be noisy and contain errors or irrelevant information. Preprocessing steps, such as filtering and normalization, can help clean and standardize the data, making it more suitable for feature extraction.
+
+> The Studio does not perform normalization or standardization, so sometimes, when working with Sensor Fusion, it could be necessary to perform this step before uploading data to the Studio. This is particularly crucial in sensor fusion projects, as seen in this tutorial, [Sensor Data Fusion with Spresense and CommonSense](https://docs.edgeimpulse.com/experts/air-quality-and-environmental-projects/environmental-sensor-fusion-commonsense).
+
+**Segmentation**: Depending on the nature of the data and the application, dividing the data into smaller segments or **windows** may be necessary. This can help focus on specific events or activities within the dataset, making feature extraction more manageable and meaningful. The **window size** and overlap (**window span**) choice depend on the application and the frequency of the events of interest. As a rule of thumb, we should try to capture a couple of "data cycles."
+
+**Feature extraction**: Once the data is preprocessed and segmented, you can extract features that describe the motion's characteristics. Some typical features extracted from accelerometer data include:
+
+- **Time-domain** features describe the data's [statistical properties](https://www.mdpi.com/1424-8220/22/5/2012) within each segment, such as mean, median, standard deviation, skewness, kurtosis, and zero-crossing rate.
+- **Frequency-domain** features are obtained by transforming the data into the frequency domain using techniques like the [Fast Fourier Transform (FFT)](https://en.wikipedia.org/wiki/Fast_Fourier_transform). Some typical frequency-domain features include the power spectrum, spectral energy, dominant frequencies (amplitude and frequency), and spectral entropy.
+- **Time-frequency** domain features combine the time and frequency domain information, such as the [Short-Time Fourier Transform (STFT)](https://en.wikipedia.org/wiki/Short-time_Fourier_transform) or the [Discrete Wavelet Transform (DWT)](https://en.wikipedia.org/wiki/Discrete_wavelet_transform). They can provide a more detailed understanding of how the signal's frequency content changes over time.
+
+In many cases, the number of extracted features can be large, which may lead to overfitting or increased computational complexity. Feature selection techniques, such as mutual information, correlation-based methods, or principal component analysis (PCA), can help identify the most relevant features for a given application and reduce the dimensionality of the dataset. The Studio can help with such feature-relevant calculations.
+
+Let's explore in more detail a typical TinyML Motion Classification project covered in this series of Hands-Ons.
+
+## A TinyML Motion Classification project
+
+{fig-align="center" width="6.5in"}
+
+In the hands-on project, *Motion Classification and Anomaly Detection*, we simulated mechanical stresses in transport, where our problem was to classify four classes of movement:
+
+- **Maritime** (pallets in boats)
+- **Terrestrial** (pallets in a Truck or Train)
+- **Lift** (pallets being handled by Fork-Lift)
+- **Idle** (pallets in Storage houses)
+
+The accelerometers provided the data on the pallet (or container).
+
+{fig-align="center" width="6.5in"}
+
+Below is one sample (raw data) of 10 seconds, captured with a sampling frequency of 50Hz:
+
+{fig-align="center" width="6.5in"}
+
+> The result is similar when this analysis is done over another dataset with the same principle, using a different sampling frequency, 62.5Hz instead of 50Hz.
+
+## Data Pre-Processing
+
+The raw data captured by the accelerometer (a "time series" data) should be converted to "tabular data" using one of the typical Feature Extraction methods described in the last section.
+
+We should segment the data using a sliding window over the sample data for feature extraction. The project captured accelerometer data every 10 seconds with a sample rate of 62.5 Hz. A 2-second window captures 375 data points (3 axis x 2 seconds x 62.5 samples). The window is slid every 80ms, creating a larger dataset where each instance has 375 "raw features."
+
+{fig-align="center" width="6.5in"}
+
+On the Studio, the previous version (V1) of the **Spectral Analysis Block** extracted as time-domain features only the RMS, and for the frequency-domain, the peaks and frequency (using FFT) and the power characteristics (PSD) of the signal over time resulting in a fixed tabular dataset of 33 features (11 per each axis),
+
+{fig-align="center" width="6.5in"}
+
+Those 33 features were the Input tensor of a Neural Network Classifier.
+
+In 2022, Edge Impulse released version 2 of the Spectral Analysis block, which we will explore here.
+
+### Edge Impulse - Spectral Analysis Block V.2 under the hood
+
+In Version 2, Time Domain Statistical features per axis/channel are:
+
+- RMS
+- Skewness
+- Kurtosis
+
+And the Frequency Domain Spectral features per axis/channel are:
+
+- Spectral Power
+- Skewness (in the next version)
+- Kurtosis (in the next version)
+
+In this [link,](https://docs.edgeimpulse.com/docs/edge-impulse-studio/processing-blocks/spectral-features) we can have more details about the feature extraction.
+
+> Clone the [public project](https://studio.edgeimpulse.com/public/198358/latest). You can also follow the explanation, playing with the code using my Google CoLab Notebook: [Edge Impulse Spectral Analysis Block Notebook](https://colab.research.google.com/github/Mjrovai/TinyML4D/blob/main/SciTinyM-2023/Edge_Impulse-Spectral_Analysis_Block/Edge_Impulse_Spectral_Analysis_Block_V3.ipynb).
+
+Start importing the libraries:
+
+``` python
+import numpy as np
+import matplotlib.pyplot as plt
+import seaborn as sns
+import math
+from scipy.stats import skew, kurtosis
+from scipy import signal
+from scipy.signal import welch
+from scipy.stats import entropy
+from sklearn import preprocessing
+import pywt
+
+plt.rcParams['figure.figsize'] = (12, 6)
+plt.rcParams['lines.linewidth'] = 3
+```
+
+From the studied project, let's choose a data sample from accelerometers as below:
+
+- Window size of 2 seconds: `[2,000]` ms
+- Sample frequency: `[62.5]` Hz
+- We will choose the `[None]` filter (for simplicity) and a
+- FFT length: `[16]`.
+
+``` python
+f = 62.5 # Hertz
+wind_sec = 2 # seconds
+FFT_Lenght = 16
+axis = ['accX', 'accY', 'accZ']
+n_sensors = len(axis)
+```
+
+{fig-align="center" width="5.6in"}
+
+Selecting the *Raw Features* on the Studio Spectral Analysis tab, we can copy all 375 data points of a particular 2-second window to the clipboard.
+
+{fig-align="center" width="6.5in"}
+
+Paste the data points to a new variable *data*:
+
+``` python
+data=[-5.6330, 0.2376, 9.8701, -5.9442, 0.4830, 9.8701, -5.4217, ...]
+No_raw_features = len(data)
+N = int(No_raw_features/n_sensors)
+```
+
+The total raw features are 375, but we will work with each axis individually, where N= 125 (number of samples per axis).
+
+We aim to understand how Edge Impulse gets the processed features.
+
+{fig-align="center" width="4.57in"}
+
+So, you should also past the processed features on a variable (to compare the calculated features in Python with the ones provided by the Studio) :
+
+``` python
+features = [2.7322, -0.0978, -0.3813, 2.3980, 3.8924, 24.6841, 9.6303, ...]
+N_feat = len(features)
+N_feat_axis = int(N_feat/n_sensors)
+```
+
+The total number of processed features is 39, which means 13 features/axis.
+
+Looking at those 13 features closely, we will find 3 for the time domain (RMS, Skewness, and Kurtosis):
+
+- `[rms] [skew] [kurtosis]`
+
+and 10 for the frequency domain (we will return to this later).
+
+- `[spectral skew][spectral kurtosis][Spectral Power 1] ... [Spectral Power 8]`
+
+**Splitting raw data per sensor**
+
+The data has samples from all axes; let's split and plot them separately:
+
+``` python
+def plot_data(sensors, axis, title):
+ [plt.plot(x, label=y) for x,y in zip(sensors, axis)]
+ plt.legend(loc='lower right')
+ plt.title(title)
+ plt.xlabel('#Sample')
+ plt.ylabel('Value')
+ plt.box(False)
+ plt.grid()
+ plt.show()
+
+accX = data[0::3]
+accY = data[1::3]
+accZ = data[2::3]
+sensors = [accX, accY, accZ]
+plot_data(sensors, axis, 'Raw Features')
+```
+
+{fig-align="center" width="6.5in"}
+
+**Subtracting the mean**
+
+Next, we should subtract the mean from the *data*. Subtracting the mean from a data set is a common data pre-processing step in statistics and machine learning. The purpose of subtracting the mean from the data is to center the data around zero. This is important because it can reveal patterns and relationships that might be hidden if the data is not centered.
+
+Here are some specific reasons why subtracting the mean can be helpful:
+
+- It simplifies analysis: By centering the data, the mean becomes zero, making some calculations simpler and easier to interpret.
+- It removes bias: If the data is biased, subtracting the mean can remove it and allow for a more accurate analysis.
+- It can reveal patterns: Centering the data can help uncover patterns that might be hidden if the data is not centered. For example, centering the data can help you identify trends over time if you analyze a time series dataset.
+- It can improve performance: In some machine learning algorithms, centering the data can improve performance by reducing the influence of outliers and making the data more easily comparable. Overall, subtracting the mean is a simple but powerful technique that can be used to improve the analysis and interpretation of data.
+
+``` python
+dtmean = [(sum(x)/len(x)) for x in sensors]
+[print('mean_'+x+'= ', round(y, 4)) for x,y in zip(axis, dtmean)][0]
+
+accX = [(x - dtmean[0]) for x in accX]
+accY = [(x - dtmean[1]) for x in accY]
+accZ = [(x - dtmean[2]) for x in accZ]
+sensors = [accX, accY, accZ]
+
+plot_data(sensors, axis, 'Raw Features - Subctract the Mean')
+```
+
+{fig-align="center" width="6.5in"}
+
+## Time Domain Statistical features
+
+**RMS Calculation**
+
+The RMS value of a set of values (or a continuous-time waveform) is the square root of the arithmetic mean of the squares of the values or the square of the function that defines the continuous waveform. In physics, the RMS value of an electrical current is defined as the "value of the direct current that dissipates the same power in a resistor."
+
+In the case of a set of n values {𝑥1, 𝑥2, ..., 𝑥𝑛}, the RMS is:
+
+{fig-align="center"}
+
+> NOTE that the RMS value is different for the original raw data, and after subtracting the mean
+
+``` py
+# Using numpy and standartized data (subtracting mean)
+rms = [np.sqrt(np.mean(np.square(x))) for x in sensors]
+```
+
+We can compare the calculated RMS values here with the ones presented by Edge Impulse:
+
+``` python
+[print('rms_'+x+'= ', round(y, 4)) for x,y in zip(axis, rms)][0]
+print("\nCompare with Edge Impulse result features")
+print(features[0:N_feat:N_feat_axis])
+```
+
+`rms_accX= 2.7322`
+
+`rms_accY= 0.7833`
+
+`rms_accZ= 0.1383`
+
+Compared with Edge Impulse result features:
+
+`[2.7322, 0.7833, 0.1383]`
+
+**Skewness and kurtosis calculation**
+
+In statistics, skewness and kurtosis are two ways to measure the **shape of a distribution**.
+
+Here, we can see the sensor values distribution:
+
+``` python
+fig, axes = plt.subplots(nrows=1, ncols=3, figsize=(13, 4))
+sns.kdeplot(accX, fill=True, ax=axes[0])
+sns.kdeplot(accY, fill=True, ax=axes[1])
+sns.kdeplot(accZ, fill=True, ax=axes[2])
+axes[0].set_title('accX')
+axes[1].set_title('accY')
+axes[2].set_title('accZ')
+plt.suptitle('IMU Sensors distribution', fontsize=16, y=1.02)
+plt.show()
+```
+
+{fig-align="center" width="6.5in"}
+
+[**Skewness**](https://en.wikipedia.org/wiki/Skewness) is a measure of the asymmetry of a distribution. This value can be positive or negative.
+
+{fig-align="center" width="4.65in"}
+
+- A negative skew indicates that the tail is on the left side of the distribution, which extends towards more negative values.
+- A positive skew indicates that the tail is on the right side of the distribution, which extends towards more positive values.
+- A zero value indicates no skewness in the distribution at all, meaning the distribution is perfectly symmetrical.
+
+``` python
+skew = [skew(x, bias=False) for x in sensors]
+[print('skew_'+x+'= ', round(y, 4)) for x,y in zip(axis, skew)][0]
+print("\nCompare with Edge Impulse result features")
+features[1:N_feat:N_feat_axis]
+```
+
+`skew_accX= -0.099`
+
+`skew_accY= 0.1756`
+
+`skew_accZ= 6.9463`
+
+Compared with Edge Impulse result features:
+
+`[-0.0978, 0.1735, 6.8629]`
+
+[**Kurtosis**](https://en.wikipedia.org/wiki/Kurtosis) is a measure of whether or not a distribution is heavy-tailed or light-tailed relative to a normal distribution.
+
+{fig-align="center"}
+
+- The kurtosis of a normal distribution is zero.
+- If a given distribution has a negative kurtosis, it is said to be playkurtic, which means it tends to produce fewer and less extreme outliers than the normal distribution.
+- If a given distribution has a positive kurtosis , it is said to be leptokurtic, which means it tends to produce more outliers than the normal distribution.
+
+``` python
+kurt = [kurtosis(x, bias=False) for x in sensors]
+[print('kurt_'+x+'= ', round(y, 4)) for x,y in zip(axis, kurt)][0]
+print("\nCompare with Edge Impulse result features")
+features[2:N_feat:N_feat_axis]
+```
+
+`kurt_accX= -0.3475`
+
+`kurt_accY= 1.2673`
+
+`kurt_accZ= 68.1123`
+
+Compared with Edge Impulse result features:
+
+`[-0.3813, 1.1696, 65.3726]`
+
+## Spectral features
+
+The filtered signal is passed to the Spectral power section, which computes the **FFT** to generate the spectral features.
+
+Since the sampled window is usually larger than the FFT size, the window will be broken into frames (or "sub-windows"), and the FFT is calculated over each frame.
+
+**FFT length** - The FFT size. This determines the number of FFT bins and the resolution of frequency peaks that can be separated. A low number means more signals will average together in the same FFT bin, but it also reduces the number of features and model size. A high number will separate more signals into separate bins, generating a larger model.
+
+- The total number of Spectral Power features will vary depending on how you set the filter and FFT parameters. With No filtering, the number of features is 1/2 of the FFT Length.
+
+**Spectral Power - Welch's method**
+
+We should use [Welch's method](https://docs.scipy.org/doc/scipy-0.14.0/reference/generated/scipy.signal.welch.html) to split the signal on the frequency domain in bins and calculate the power spectrum for each bin. This method divides the signal into overlapping segments, applies a window function to each segment, computes the periodogram of each segment using DFT, and averages them to obtain a smoother estimate of the power spectrum.
+
+``` python
+# Function used by Edge Impulse instead of scipy.signal.welch().
+def welch_max_hold(fx, sampling_freq, nfft, n_overlap):
+ n_overlap = int(n_overlap)
+ spec_powers = [0 for _ in range(nfft//2+1)]
+ ix = 0
+ while ix <= len(fx):
+ # Slicing truncates if end_idx > len, and rfft will auto-zero pad
+ fft_out = np.abs(np.fft.rfft(fx[ix:ix+nfft], nfft))
+ spec_powers = np.maximum(spec_powers, fft_out**2/nfft)
+ ix = ix + (nfft-n_overlap)
+ return np.fft.rfftfreq(nfft, 1/sampling_freq), spec_powers
+```
+
+Applying the above function to 3 signals:
+
+``` python
+fax,Pax = welch_max_hold(accX, fs, FFT_Lenght, 0)
+fay,Pay = welch_max_hold(accY, fs, FFT_Lenght, 0)
+faz,Paz = welch_max_hold(accZ, fs, FFT_Lenght, 0)
+specs = [Pax, Pay, Paz ]
+```
+
+We can plot the Power Spectrum P(f):
+
+``` python
+plt.plot(fax,Pax, label='accX')
+plt.plot(fay,Pay, label='accY')
+plt.plot(faz,Paz, label='accZ')
+plt.legend(loc='upper right')
+plt.xlabel('Frequency (Hz)')
+#plt.ylabel('PSD [V**2/Hz]')
+plt.ylabel('Power')
+plt.title('Power spectrum P(f) using Welch\'s method')
+plt.grid()
+plt.box(False)
+plt.show()
+```
+
+{fig-align="center" width="6.5in"}
+
+Besides the Power Spectrum, we can also include the skewness and kurtosis of the features in the frequency domain (should be available on a new version):
+
+``` python
+spec_skew = [skew(x, bias=False) for x in specs]
+spec_kurtosis = [kurtosis(x, bias=False) for x in specs]
+```
+
+Let's now list all Spectral features per axis and compare them with EI:
+
+``` python
+print("EI Processed Spectral features (accX): ")
+print(features[3:N_feat_axis][0:])
+print("\nCalculated features:")
+print (round(spec_skew[0],4))
+print (round(spec_kurtosis[0],4))
+[print(round(x, 4)) for x in Pax[1:]][0]
+```
+
+EI Processed Spectral features (accX):
+
+2.398, 3.8924, 24.6841, 9.6303, 8.4867, 7.7793, 2.9963, 5.6242, 3.4198, 4.2735
+
+Calculated features:
+
+2.9069 8.5569 24.6844 9.6304 8.4865 7.7794 2.9964 5.6242 3.4198 4.2736
+
+``` python
+print("EI Processed Spectral features (accY): ")
+print(features[16:26][0:]) #13: 3+N_feat_axis; 26 = 2x N_feat_axis
+print("\nCalculated features:")
+print (round(spec_skew[1],4))
+print (round(spec_kurtosis[1],4))
+[print(round(x, 4)) for x in Pay[1:]][0]
+```
+
+EI Processed Spectral features (accY):
+
+0.9426, -0.8039, 5.429, 0.999, 1.0315, 0.9459, 1.8117, 0.9088, 1.3302, 3.112
+
+Calculated features:
+
+1.1426 -0.3886 5.4289 0.999 1.0315 0.9458 1.8116 0.9088 1.3301 3.1121
+
+``` python
+print("EI Processed Spectral features (accZ): ")
+print(features[29:][0:]) #29: 3+(2*N_feat_axis);
+print("\nCalculated features:")
+print (round(spec_skew[2],4))
+print (round(spec_kurtosis[2],4))
+[print(round(x, 4)) for x in Paz[1:]][0]
+```
+
+EI Processed Spectral features (accZ):
+
+0.3117, -1.3812, 0.0606, 0.057, 0.0567, 0.0976, 0.194, 0.2574, 0.2083, 0.166
+
+Calculated features:
+
+0.3781 -1.4874 0.0606 0.057 0.0567 0.0976 0.194 0.2574 0.2083 0.166
+
+## Time-frequency domain
+
+### Wavelets
+
+[Wavelet](https://en.wikipedia.org/wiki/Wavelet) is a powerful technique for analyzing signals with transient features or abrupt changes, such as spikes or edges, which are difficult to interpret with traditional Fourier-based methods.
+
+Wavelet transforms work by breaking down a signal into different frequency components and analyzing them individually. The transformation is achieved by convolving the signal with a **wavelet function**, a small waveform centered at a specific time and frequency. This process effectively decomposes the signal into different frequency bands, each of which can be analyzed separately.
+
+One of the critical benefits of wavelet transforms is that they allow for time-frequency analysis, which means that they can reveal the frequency content of a signal as it changes over time. This makes them particularly useful for analyzing non-stationary signals, which vary over time.
+
+Wavelets have many practical applications, including signal and image compression, denoising, feature extraction, and image processing.
+
+Let's select Wavelet on the Spectral Features block in the same project:
+
+- Type: Wavelet
+- Wavelet Decomposition Level: 1
+- Wavelet: bior1.3
+
+{fig-align="center"}
+
+**The Wavelet Function**
+
+``` python
+wavelet_name='bior1.3'
+num_layer = 1
+
+wavelet = pywt.Wavelet(wavelet_name)
+[phi_d,psi_d,phi_r,psi_r,x] = wavelet.wavefun(level=5)
+plt.plot(x, psi_d, color='red')
+plt.title('Wavelet Function')
+plt.ylabel('Value')
+plt.xlabel('Time')
+plt.grid()
+plt.box(False)
+plt.show()
+```
+
+{fig-align="center" width="6.5in"}
+
+As we did before, let's copy and past the Processed Features:
+
+{fig-align="center" width="6.5in"}
+
+``` python
+features = [3.6251, 0.0615, 0.0615, -7.3517, -2.7641, 2.8462, 5.0924, ...]
+N_feat = len(features)
+N_feat_axis = int(N_feat/n_sensors)
+```
+
+Edge Impulse computes the [Discrete Wavelet Transform (DWT)](https://pywavelets.readthedocs.io/en/latest/ref/dwt-discrete-wavelet-transform.html) for each one of the Wavelet Decomposition levels selected. After that, the features will be extracted.
+
+In the case of **Wavelets**, the extracted features are *basic statistical values*, *crossing values*, and *entropy.* There are, in total, 14 features per layer as below:
+
+- \[11\] Statiscal Features: **n5, n25, n75, n95, mean, median,** standard deviation **(std)**, variance **(var)** root mean square **(rms), kurtosis**, and skewness **(skew)**.
+- \[2\] Crossing Features: Zero crossing rate **(zcross)** and mean crossing rate **(mcross)** are the times that the signal passes through the baseline (y = 0) and the average level (y = u) per unit of time, respectively
+- \[1\] Complexity Feature: **Entropy** is a characteristic measure of the complexity of the signal
+
+All the above 14 values are calculated for each Layer (including L0, the original signal)
+
+- The total number of features varies depending on how you set the filter and the number of layers. For example, with \[None\] filtering and Level\[1\], the number of features per axis will be 14 x 2 (L0 and L1) = 28. For the three axes, we will have a total of 84 features.
+
+### Wavelet Analysis
+
+Wavelet analysis decomposes the signal (**accX, accY**, **and accZ**) into different frequency components using a set of filters, which separate these components into low-frequency (slowly varying parts of the signal containing long-term patterns), such as **accX_l1, accY_l1, accZ_l1** and, high-frequency (rapidly varying parts of the signal containing short-term patterns) components, such as **accX_d1, accY_d1, accZ_d1**, permitting the extraction of features for further analysis or classification.
+
+Only the low-frequency components (approximation coefficients, or cA) will be used. In this example, we assume only one level (Single-level Discrete Wavelet Transform), where the function will return a tuple. With a multilevel decomposition, the "Multilevel 1D Discrete Wavelet Transform", the result will be a list (for detail, please see: [Discrete Wavelet Transform (DWT)](https://pywavelets.readthedocs.io/en/latest/ref/dwt-discrete-wavelet-transform.html) )
+
+``` python
+(accX_l1, accX_d1) = pywt.dwt(accX, wavelet_name)
+(accY_l1, accY_d1) = pywt.dwt(accY, wavelet_name)
+(accZ_l1, accZ_d1) = pywt.dwt(accZ, wavelet_name)
+sensors_l1 = [accX_l1, accY_l1, accZ_l1]
+
+# Plot power spectrum versus frequency
+plt.plot(accX_l1, label='accX')
+plt.plot(accY_l1, label='accY')
+plt.plot(accZ_l1, label='accZ')
+plt.legend(loc='lower right')
+plt.xlabel('Time')
+plt.ylabel('Value')
+plt.title('Wavelet Approximation')
+plt.grid()
+plt.box(False)
+plt.show()
+```
+
+{fig-align="center" width="6.5in"}
+
+### Feature Extraction
+
+Let's start with the basic statistical features. Note that we apply the function for both the original signals and the resultant cAs from the DWT:
+
+``` python
+def calculate_statistics(signal):
+ n5 = np.percentile(signal, 5)
+ n25 = np.percentile(signal, 25)
+ n75 = np.percentile(signal, 75)
+ n95 = np.percentile(signal, 95)
+ median = np.percentile(signal, 50)
+ mean = np.mean(signal)
+ std = np.std(signal)
+ var = np.var(signal)
+ rms = np.sqrt(np.mean(np.square(signal)))
+ return [n5, n25, n75, n95, median, mean, std, var, rms]
+
+stat_feat_l0 = [calculate_statistics(x) for x in sensors]
+stat_feat_l1 = [calculate_statistics(x) for x in sensors_l1]
+```
+
+The Skelness and Kurtosis:
+
+``` python
+skew_l0 = [skew(x, bias=False) for x in sensors]
+skew_l1 = [skew(x, bias=False) for x in sensors_l1]
+kurtosis_l0 = [kurtosis(x, bias=False) for x in sensors]
+kurtosis_l1 = [kurtosis(x, bias=False) for x in sensors_l1]
+```
+
+**Zero crossing (zcross)** is the number of times the wavelet coefficient crosses the zero axis. It can be used to measure the signal's frequency content since high-frequency signals tend to have more zero crossings than low-frequency signals.
+
+**Mean crossing (mcross)**, on the other hand, is the number of times the wavelet coefficient crosses the mean of the signal. It can be used to measure the amplitude since high-amplitude signals tend to have more mean crossings than low-amplitude signals.
+
+``` python
+def getZeroCrossingRate(arr):
+ my_array = np.array(arr)
+ zcross = float("{0:.2f}".format((((my_array[:-1] * my_array[1:]) < 0).su m())/len(arr)))
+ return zcross
+
+def getMeanCrossingRate(arr):
+ mcross = getZeroCrossingRate(np.array(arr) - np.mean(arr))
+ return mcross
+
+def calculate_crossings(list):
+ zcross=[]
+ mcross=[]
+ for i in range(len(list)):
+ zcross_i = getZeroCrossingRate(list[i])
+ zcross.append(zcross_i)
+ mcross_i = getMeanCrossingRate(list[i])
+ mcross.append(mcross_i)
+ return zcross, mcross
+
+cross_l0 = calculate_crossings(sensors)
+cross_l1 = calculate_crossings(sensors_l1)
+```
+
+In wavelet analysis, **entropy** refers to the degree of disorder or randomness in the distribution of wavelet coefficients. Here, we used Shannon entropy, which measures a signal's uncertainty or randomness. It is calculated as the negative sum of the probabilities of the different possible outcomes of the signal multiplied by their base 2 logarithm. In the context of wavelet analysis, Shannon entropy can be used to measure the complexity of the signal, with higher values indicating greater complexity.
+
+``` python
+def calculate_entropy(signal, base=None):
+ value, counts = np.unique(signal, return_counts=True)
+ return entropy(counts, base=base)
+
+entropy_l0 = [calculate_entropy(x) for x in sensors]
+entropy_l1 = [calculate_entropy(x) for x in sensors_l1]
+```
+
+Let's now list all the wavelet features and create a list by layers.
+
+``` python
+L1_features_names = ["L1-n5", "L1-n25", "L1-n75", "L1-n95", "L1-median", "L1-mean", "L1-std", "L1-var", "L1-rms", "L1-skew", "L1-Kurtosis", "L1-zcross", "L1-mcross", "L1-entropy"]
+
+L0_features_names = ["L0-n5", "L0-n25", "L0-n75", "L0-n95", "L0-median", "L0-mean", "L0-std", "L0-var", "L0-rms", "L0-skew", "L0-Kurtosis", "L0-zcross", "L0-mcross", "L0-entropy"]
+
+all_feat_l0 = []
+for i in range(len(axis)):
+ feat_l0 = stat_feat_l0[i]+[skew_l0[i]]+[kurtosis_l0[i]]+[cross_l0[0][i]]+[cross_l0[1][i]]+[entropy_l0[i]]
+ [print(axis[i]+' '+x+'= ', round(y, 4)) for x,y in zip(L0_features_names, feat_l0)][0]
+ all_feat_l0.append(feat_l0)
+all_feat_l0 = [item for sublist in all_feat_l0 for item in sublist]
+print(f"\nAll L0 Features = {len(all_feat_l0)}")
+
+all_feat_l1 = []
+for i in range(len(axis)):
+feat_l1 = stat_feat_l1[i]+[skew_l1[i]]+[kurtosis_l1[i]]+[cross_l1[0][i]]+[cross_l1[1][i]]+[entropy_l1[i]]
+[print(axis[i]+' '+x+'= ', round(y, 4)) for x,y in zip(L1_features_names, feat_l1)][0]
+all_feat_l1.append(feat_l1)
+all_feat_l1 = [item for sublist in all_feat_l1 for item in sublist]
+print(f"\nAll L1 Features = {len(all_feat_l1)}")
+```
+
+{fig-align="center" width="3.58in"}
+
+## Conclusion
+
+Edge Impulse Studio is a powerful online platform that can handle the pre-processing task for us. Still, given our engineering perspective, we want to understand what is happening under the hood. This knowledge will help us find the best options and hyper-parameters for tuning our projects.
+
+Daniel Situnayake wrote in his [blog:](https://situnayake.com/) "Raw sensor data is highly dimensional and noisy. Digital signal processing algorithms help us sift the signal from the noise. DSP is an essential part of embedded engineering, and many edge processors have on-board acceleration for DSP. As an ML engineer, learning basic DSP gives you superpowers for handling high-frequency time series data in your models." I recommend you read Dan's excellent post in its totality: [nn to cpp: What you need to know about porting deep learning models to the edge](https://situnayake.com/2023/03/21/nn-to-cpp.html).
diff --git a/efficient_ai.qmd b/efficient_ai.qmd
index 8b5b9d83..6753940f 100644
--- a/efficient_ai.qmd
+++ b/efficient_ai.qmd
@@ -5,10 +5,24 @@ Efficiency in artificial intelligence (AI) is not simply a luxury; it is a neces
::: {.callout-tip}
## Learning Objectives
-* coming soon.
+- Recognize the need for efficient AI in TinyML/edge devices.
+
+- Understand the need for efficient model architectures like MobileNets and SqueezeNet.
+
+- Understand why techniques for model compression are important.
+
+- Get an inclination for why efficient AI hardware is important.
+
+- Appreciate the significance of numerics and their representations.
+
+- Appreciate that we need to understand nuances of model comparison beyond accuracy.
+
+- Recognize efficiency encompasses technology, costs, environment, ethics.
:::
+The focus is on gaining a conceptual understanding of the motivations and significance of the various strategies for achieving efficient AI, both in terms of techniques and a holistic perspective. Subsequent chapters will dive into the nitty gritty details on these various concepts.
+
## Introduction
Training models can consume a significant amount of energy, sometimes equivalent to the carbon footprint of sizable industrial processes. We will cover some of these sustainability details in the [AI Sustainability](./sustainable_ai.qmd) chapter. On the deployment side, if these models are not optimized for efficiency, they can quickly drain device batteries, demand excessive memory, or fall short of real-time processing needs. Through this introduction, our objective is to elucidate the nuances of efficiency, setting the groundwork for a comprehensive exploration in the subsequent chapters.
diff --git a/images/cover_ml_ops.png b/images/cover_ml_ops.png
new file mode 100644
index 00000000..9b2f921b
Binary files /dev/null and b/images/cover_ml_ops.png differ
diff --git a/images/imgs_dsp_spectral_features/case_study.png b/images/imgs_dsp_spectral_features/case_study.png
new file mode 100644
index 00000000..cd435677
Binary files /dev/null and b/images/imgs_dsp_spectral_features/case_study.png differ
diff --git a/images/imgs_dsp_spectral_features/data_sample.png b/images/imgs_dsp_spectral_features/data_sample.png
new file mode 100644
index 00000000..edb86291
Binary files /dev/null and b/images/imgs_dsp_spectral_features/data_sample.png differ
diff --git a/images/imgs_dsp_spectral_features/features.png b/images/imgs_dsp_spectral_features/features.png
new file mode 100644
index 00000000..6bac98f2
Binary files /dev/null and b/images/imgs_dsp_spectral_features/features.png differ
diff --git a/images/imgs_dsp_spectral_features/fft.png b/images/imgs_dsp_spectral_features/fft.png
new file mode 100644
index 00000000..5d5cdc6c
Binary files /dev/null and b/images/imgs_dsp_spectral_features/fft.png differ
diff --git a/images/imgs_dsp_spectral_features/fft_result.png b/images/imgs_dsp_spectral_features/fft_result.png
new file mode 100644
index 00000000..501782f7
Binary files /dev/null and b/images/imgs_dsp_spectral_features/fft_result.png differ
diff --git a/images/imgs_dsp_spectral_features/impulse.png b/images/imgs_dsp_spectral_features/impulse.png
new file mode 100644
index 00000000..fde5fea7
Binary files /dev/null and b/images/imgs_dsp_spectral_features/impulse.png differ
diff --git a/images/imgs_dsp_spectral_features/kurto.png b/images/imgs_dsp_spectral_features/kurto.png
new file mode 100644
index 00000000..f9765c64
Binary files /dev/null and b/images/imgs_dsp_spectral_features/kurto.png differ
diff --git a/images/imgs_dsp_spectral_features/process_features.png b/images/imgs_dsp_spectral_features/process_features.png
new file mode 100644
index 00000000..db3ef3ac
Binary files /dev/null and b/images/imgs_dsp_spectral_features/process_features.png differ
diff --git a/images/imgs_dsp_spectral_features/rms.png b/images/imgs_dsp_spectral_features/rms.png
new file mode 100644
index 00000000..f125eabc
Binary files /dev/null and b/images/imgs_dsp_spectral_features/rms.png differ
diff --git a/images/imgs_dsp_spectral_features/sample.png b/images/imgs_dsp_spectral_features/sample.png
new file mode 100644
index 00000000..dd6a2978
Binary files /dev/null and b/images/imgs_dsp_spectral_features/sample.png differ
diff --git a/images/imgs_dsp_spectral_features/sample_no_mean.png b/images/imgs_dsp_spectral_features/sample_no_mean.png
new file mode 100644
index 00000000..64d6c6e4
Binary files /dev/null and b/images/imgs_dsp_spectral_features/sample_no_mean.png differ
diff --git a/images/imgs_dsp_spectral_features/skew.png b/images/imgs_dsp_spectral_features/skew.png
new file mode 100644
index 00000000..3473bd98
Binary files /dev/null and b/images/imgs_dsp_spectral_features/skew.png differ
diff --git a/images/imgs_dsp_spectral_features/skew_2.png b/images/imgs_dsp_spectral_features/skew_2.png
new file mode 100644
index 00000000..fee54d85
Binary files /dev/null and b/images/imgs_dsp_spectral_features/skew_2.png differ
diff --git a/images/imgs_dsp_spectral_features/spectral_block.jpeg b/images/imgs_dsp_spectral_features/spectral_block.jpeg
new file mode 100644
index 00000000..d3cd6fa2
Binary files /dev/null and b/images/imgs_dsp_spectral_features/spectral_block.jpeg differ
diff --git a/images/imgs_dsp_spectral_features/v1.png b/images/imgs_dsp_spectral_features/v1.png
new file mode 100644
index 00000000..23a0c349
Binary files /dev/null and b/images/imgs_dsp_spectral_features/v1.png differ
diff --git a/images/imgs_dsp_spectral_features/v1_features.png b/images/imgs_dsp_spectral_features/v1_features.png
new file mode 100644
index 00000000..47ab6794
Binary files /dev/null and b/images/imgs_dsp_spectral_features/v1_features.png differ
diff --git a/images/imgs_dsp_spectral_features/wav.png b/images/imgs_dsp_spectral_features/wav.png
new file mode 100644
index 00000000..ea7a8658
Binary files /dev/null and b/images/imgs_dsp_spectral_features/wav.png differ
diff --git a/images/imgs_dsp_spectral_features/wav_processed.png b/images/imgs_dsp_spectral_features/wav_processed.png
new file mode 100644
index 00000000..de1ccdb0
Binary files /dev/null and b/images/imgs_dsp_spectral_features/wav_processed.png differ
diff --git a/images/imgs_dsp_spectral_features/wav_result.png b/images/imgs_dsp_spectral_features/wav_result.png
new file mode 100644
index 00000000..8583c324
Binary files /dev/null and b/images/imgs_dsp_spectral_features/wav_result.png differ
diff --git a/images/imgs_dsp_spectral_features/wavelet_input.png b/images/imgs_dsp_spectral_features/wavelet_input.png
new file mode 100644
index 00000000..88fc2eab
Binary files /dev/null and b/images/imgs_dsp_spectral_features/wavelet_input.png differ
diff --git a/images/ondevice_fed_averaging.png b/images/ondevice_fed_averaging.png
new file mode 100644
index 00000000..27b6fca5
Binary files /dev/null and b/images/ondevice_fed_averaging.png differ
diff --git a/images/ondevice_gboard_approach.png b/images/ondevice_gboard_approach.png
new file mode 100644
index 00000000..188cc544
Binary files /dev/null and b/images/ondevice_gboard_approach.png differ
diff --git a/images/ondevice_gboard_example.png b/images/ondevice_gboard_example.png
new file mode 100644
index 00000000..d754f4dd
Binary files /dev/null and b/images/ondevice_gboard_example.png differ
diff --git a/images/ondevice_intro.png b/images/ondevice_intro.png
new file mode 100644
index 00000000..35562d03
Binary files /dev/null and b/images/ondevice_intro.png differ
diff --git a/images/ondevice_medperf.png b/images/ondevice_medperf.png
new file mode 100644
index 00000000..e2c9187e
Binary files /dev/null and b/images/ondevice_medperf.png differ
diff --git a/images/ondevice_pretraining.png b/images/ondevice_pretraining.png
new file mode 100644
index 00000000..8f55f8dd
Binary files /dev/null and b/images/ondevice_pretraining.png differ
diff --git a/images/ondevice_pruning.png b/images/ondevice_pruning.png
new file mode 100644
index 00000000..43910adc
Binary files /dev/null and b/images/ondevice_pruning.png differ
diff --git a/images/ondevice_quantization_matrix.png b/images/ondevice_quantization_matrix.png
new file mode 100644
index 00000000..5135b7d4
Binary files /dev/null and b/images/ondevice_quantization_matrix.png differ
diff --git a/images/ondevice_split_model.png b/images/ondevice_split_model.png
new file mode 100644
index 00000000..0c7f55a7
Binary files /dev/null and b/images/ondevice_split_model.png differ
diff --git a/images/ondevice_training_flow.png b/images/ondevice_training_flow.png
new file mode 100644
index 00000000..1a25fa3a
Binary files /dev/null and b/images/ondevice_training_flow.png differ
diff --git a/images/ondevice_transfer_learning_apps.png b/images/ondevice_transfer_learning_apps.png
new file mode 100644
index 00000000..32277f41
Binary files /dev/null and b/images/ondevice_transfer_learning_apps.png differ
diff --git a/images/ondevice_transfer_tinytl.png b/images/ondevice_transfer_tinytl.png
new file mode 100644
index 00000000..4312c9c1
Binary files /dev/null and b/images/ondevice_transfer_tinytl.png differ
diff --git a/ondevice_learning.qmd b/ondevice_learning.qmd
index 72e3f2ed..2898a356 100644
--- a/ondevice_learning.qmd
+++ b/ondevice_learning.qmd
@@ -1,63 +1,645 @@
# On-Device Learning
-
+
+
+On-device Learning represents a significant innovation for embedded and edge IoT devices, enabling models to train and update directly on small local devices. This contrasts with traditional methods where models are trained on expansive cloud computing resources before deployment. With On-Device Learning, devices like smart speakers, wearables, and industrial sensors can refine models in real-time based on local data, without needing to transmit data externally. For example, a voice-enabled smart speaker could learn and adapt to its owner's speech patterns and vocabulary right on the device. But there is no such thing as free lunch, therefore in this chapter, we will discuss both the benefits and the limitations of on-device learning.
::: {.callout-tip}
## Learning Objectives
-* coming soon.
+* Understand on-device learning and how it differs from cloud-based training
+
+* Recognize the benefits and limitations of on-device learning
+
+* Examine strategies to adapt models through complexity reduction, optimization, and data compression
+
+* Understand related concepts like federated learning and transfer learning
+
+* Analyze the security implications of on-device learning and mitigation strategies
:::
+
## Introduction
-Explanation: This section sets the stage for the reader, explaining why on-device learning is a critical aspect of embedded AI systems.
+On-device Learning refers to the process of training ML models directly on the device where they are deployed, as opposed to traditional methods where models are trained on powerful servers and then deployed to devices. This method is particularly relevant to TinyML, where ML systems are integrated into tiny, resource-constrained devices.
+
+An example of On-Device Learning can be seen in a smart thermostat that adapts to user behavior over time. Initially, the thermostat may have a generic model that understands basic patterns of usage. However, as it is exposed to more data, such as the times the user is home or away, preferred temperatures, and external weather conditions, the thermostat can refine its model directly on the device to provide a personalized experience for the user. This is all done without the need to send data back to a central server for processing.
+
+Another example is in predictive text on smartphones. As users type, the phone learns from the user’s language patterns and suggests words or phrases that are likely to be used next. This learning happens directly on the device, and the model updates in real-time as more data is collected. A widely used real-world example of on-device learning is Gboard. On an Android phone, Gboard learns from typing and dictation patterns to enhance the experience for all users.
+
+)](images/ondevice_intro.png)
- - Importance in Embedded AI
- - Why is On-device Learning Needed
## Advantages and Limitations
-Explanation: Understanding the pros and cons of on-device learning helps to identify the scenarios where it is most effective and the challenges that need to be addressed.
+On-Device Learning provides a number of advantages over traditional cloud-based ML. By keeping data and models on the device, it eliminates the need for costly data transmission and addresses privacy concerns. This allows for more personalized, responsive experiences as the model can adapt in real-time to user behavior.
+
+However, On-Device Learning also comes with tradeoffs. The limited compute resources on consumer devices can make it challenging to run complex models locally. Datasets are also more restricted since they consist only of user-generated data from a single device. Additionally, updating models requires pushing out new versions rather than seamless cloud updates.
+
+On-Device Learning opens up new capabilities by enabling offline AI while maintaining user privacy. But it requires carefully managing model and data complexity within the constraints of consumer devices. Finding the right balance between localization and cloud offloading is key to delivering optimized on-device experiences.
+
+### Benefits
+
+#### Privacy and Data Security
+
+One of the significant advantages of on-device learning is the enhanced privacy and security of user data. For instance, consider a smartwatch that monitors sensitive health metrics such as heart rate and blood pressure. By processing data and adapting models directly on the device, the biometric data remains localized, circumventing the need to transmit raw data to cloud servers where it could be susceptible to breaches.
+
+Server breaches are far from rare, with millions of records compromised annually. For example, the 2017 Equifax breach exposed the personal data of 147 million people. By keeping data on the device, the risk of such exposures is drastically minimized. On-device learning acts as a safeguard against unauthorized access from various threats, including malicious actors, insider threats, and accidental exposure, by eliminating reliance on centralized cloud storage.
+
+Regulations like the Health Insurance Portability and Accountability Act ([HIPAA](https://www.cdc.gov/phlp/publications/topic/hipaa.html)) and the General Data Protection Regulation ([GDPR](https://gdpr.eu/tag/gdpr/)) mandate stringent data privacy requirements that on-device learning adeptly addresses. By ensuring data remains localized and is not transferred to other systems, on-device learning facilitates [compliance with these regulations](https://www.researchgate.net/publication/321515854_The_EU_General_Data_Protection_Regulation_GDPR_A_Practical_Guide).
+
+On-device learning is not just beneficial for individual users; it has significant implications for organizations and sectors dealing with highly sensitive data. For instance, within the military, on-device learning empowers frontline systems to adapt models and function independently of connections to central servers that could potentially be compromised. By localizing data processing and learning, critical and sensitive information is staunchly protected. However, this comes with the trade-off that individual devices take on more value and may incentivize theft or destruction, as they become sole carriers of specialized AI models. Care must be taken to secure devices themselves when transitioning to on-device learning.
+
+It is also important in preserving the privacy, security, and regulatory compliance of personal and sensitive data. Training and operating models locally, as opposed to in the cloud, substantially augments privacy measures, ensuring that user data is safeguarded from potential threats.
+
+However, this is not entirely intuitive because on-device learning could instead open systems up to new privacy attacks.
+With valuable data summaries and model updates permanently stored on individual devices, it may be much harder to physically and digitally protect them compared to a large computing cluster. While on-device learning reduces the amount of data compromised in any one breach, it could also introduce new dangers by dispersing sensitive information across many decentralized endpoints. Careful security practices are still essential for on-device systems.
+
+#### Regulatory Compliance
+
+On-device learning helps address major privacy regulations like ([GDPR](https://gdpr.eu/tag/gdpr/)) and [CCPA](https://oag.ca.gov/privacy/ccpa). These regulations require data localization, restricting cross-border data transfers to approved countries with adequate controls. GDPR also mandates privacy by design and consent requirements for data collection. By keeping data processing and model training localized on-device, sensitive user data is not transferred across borders. This avoids major compliance headaches for organizations.
+
+For example, a healthcare provider monitoring patient vitals with wearables would have to ensure cross-border data transfers comply with HIPAA and GDPR if using the cloud. Determining which country's laws apply and securing approvals for international data flows introduces legal and engineering burdens. With on-device learning, no data leaves the device, simplifying compliance. The time and resources spent on compliance are reduced significantly.
+
+Industries like healthcare, finance and government with highly regulated data can benefit greatly from on-device learning. By localizing data and learning, regulatory requirements on privacy and data sovereignty are more easily met. On-device solutions provide an efficient way to build compliant AI applications.
+
+Major privacy regulations impose restrictions on cross-border data movement that on-device learning inherently addresses through localized processing. This reduces the compliance burden for organizations working with regulated data.
+
+#### Reduced Bandwidth, Costs, and Increased Efficiency
+
+One major advantage of on-device learning is the significant reduction in bandwidth usage and associated cloud infrastructure costs. By keeping data localized for model training, rather than transmitting raw data to the cloud, on-device learning can result in substantial savings in bandwidth. For instance, a network of cameras analyzing video footage can achieve up to significant reductions in data transfer by training models on-device rather than streaming all video footage to the cloud for processing.
+
+This reduction in data transmission not only saves bandwidth but also translates to lower costs for servers, networking, and data storage in the cloud. Large organizations, which might spend millions on cloud infrastructure to train models on device data, can experience dramatic cost reductions through on-device learning. In the era of Generative AI, where [costs have been escalating significantly](https://epochai.org/blog/trends-in-the-dollar-training-cost-of-machine-learning-systems), finding ways to keep expenses down has become increasingly important.
+
+Furthermore, the energy and environmental costs associated with running large server farms are also diminished. Data centers are known to consume vast amounts of energy, contributing to greenhouse gas emissions. By reducing the need for extensive cloud-based infrastructure, on-device learning plays a part in mitigating the environmental impact of data processing [@wu2022sustainable].
+
+Specifically for endpoint applications, on-device learning minimizes the number of network API calls needed to run inference through a cloud provider. For applications with millions of users, the cumulative costs associated with bandwidth and API calls can quickly escalate. In contrast, performing training and inferences locally is considerably more efficient and cost-effective. On-device learning has been shown to reduce training memory requirements, drastically improve memory efficiency, and reduce up to 20% in per-iteration latency under the state-of-the-art optimizations [@dhar2021survey].
+
+Another key benefit of on-device learning is the potential for IoT devices to continuously adapt their ML model to new data for continuous, lifelong learning. On-device models can quickly become outdated as user behavior, data patterns, and preferences change. Continuous learning enables the model to efficiently adapt to new data and improvements and maintain high model performance over time.
+
+### Limitations
+
+While traditional cloud-based ML systems have access to nearly endless computing resources, on-device learning is often restricted by the limitations in computational and storage power of the edge device that the model is trained on. By definition, an [edge device](http://arxiv.org/abs/1911.00623) is a device with restrained computing, memory, and energy resources, that cannot be easily increased or decreased. Thus, the reliance on edge devices can restrict the complexity, efficiency, and size of on-device ML models.
+
+#### Compute resources
+
+Traditional cloud-based ML systems utilize large servers with multiple high-end GPUs or TPUs that provide nearly endless computational power and memory. For example, services like Amazon Web Services (AWS) [EC2](https://docs.aws.amazon.com/AWSEC2/latest/UserGuide/concepts.html) allow configuring clusters of GPU instances for massively parallel training.
+
+In contrast, on-device learning is restricted by the hardware limitations of the edge device it runs on. Edge devices refer to endpoints like smartphones, embedded electronics, and IoT devices. By definition, these devices have highly restrained computing, memory, and energy resources compared to the cloud.
+
+For example, a typical smartphone or Raspberry Pi may only have a few CPU cores, a few GB of RAM, and a small battery. Even more resource-constrained are TinyML microcontroller devices such as the [Arduino Nano BLE Sense](https://store-usa.arduino.cc/products/arduino-nano-33-ble-sense). The resources are fixed on these devices and can't easily be increased on demand like scaling cloud infrastructure. This reliance on edge devices directly restricts the complexity, efficiency, and size of models that can be deployed for on-device training:
+
+- **Complexity**: Limits on memory, computing, and power restrict model architecture design, constraining the number of layers and parameters.
+- **Efficiency**: Models must be heavily optimized through methods like quantization and pruning to run faster and consume less energy.
+- **Size**: Actual model files must be compressed as much as possible to fit within the storage limitations of edge devices.
+
+Thus, while the cloud offers endless scalability, on-device learning must operate within the tight resource constraints of endpoint hardware. This requires careful co-design of streamlined models, training methods, and optimizations tailored specifically for edge devices.
+
+#### Dataset Size, Accuracy, and Generalization
+
+In addition to limited computing resources, on-device learning is also constrained in terms of the dataset available for training models.
+
+In the cloud, models are trained on massive, diverse datasets like ImageNet or Common Crawl. For example, ImageNet contains over 14 million images carefully categorized across thousands of classes.
+
+On-device learning instead relies on smaller, decentralized data silos unique to each device. A smartphone camera roll may contain only thousands of photos centered around a user's specific interests and environments.
+
+
+This decentralized data leads to a lack of IID (independent and identically distributed) data. For instance, two friends may take many photos of the same places and objects, meaning their data distributions are highly correlated rather than independent.
+
+Reasons data may be non-IID in on-device settings:
+
+- **User heterogeneity**: different users have different interests and environments.
+- **Device differences:** sensors, regions, and demographics affect data.
+- **Temporal effects:** time of day, seasonal impacts on data.
+
+The effectiveness of ML relies heavily on large, diverse training data. With small, localized datasets, on-device models may fail to generalize across different user populations and environments.
+
+For example, a disease detection model trained only on images from a single hospital would not generalize well to other patient demographics. Without extensive, diverse medical images, the model's real-world performance would suffer.
+
+Thus, while cloud-based learning leverages massive datasets, on-device learning relies on much smaller, decentralized data silos unique to each user.
+
+The limited data and optimizations required for on-device learning can negatively impact model accuracy and generalization:
+- Small datasets increase overfitting risk. For example, a fruit classifier trained on 100 images risks overfitting compared to one trained on 1 million diverse images.
+- Noisy user-generated data reduces quality. Sensor noise or improper data labeling by non-experts may degrade training.
+- Optimizations like pruning and quantization trade off accuracy for efficiency. An 8-bit quantized model runs faster but less accurately than a 32-bit model.
+
+So while cloud models achieve high accuracy with massive datasets and no constraints, on-device models can struggle to generalize. Some studies show that on-device training matches cloud accuracy on select tasks. However, performance on real-world workloads requires further study [@lin2022device].
+
+For instance, a cloud model can accurately detect pneumonia in chest X-rays from thousands of hospitals. However, an on-device model trained only on a small local patient population may fail to generalize.
+
+Unreliable accuracy limits the real-world applicability of on-device learning for mission-critical uses like disease diagnosis or self-driving vehicles.
+
+On-device training is also slower than the cloud due to limited resources. Even if each iteration is faster, the overall training process takes longer.
+
+For example, a real-time robotics application may require model updates within milliseconds. On-device training on small embedded hardware may take seconds or minutes per update - too slow for real-time use.
+
+Accuracy, generalization, and speed challenges pose hurdles to adopting on-device learning for real-world production systems, especially when reliability and low latency are critical.
+
+## Ondevice Adaptation
+
+In an ML task, resource consumption [mainly](http://arxiv.org/abs/1911.00623) comes from three sources:
+
+* The ML model itself;
+* The optimization process during model learning
+* Storing and processing the dataset used for learning.
+
+Correspondingly, there are three approaches to adapting existing ML algorithms onto resource-constrained devices:
+
+* Reducing the complexity of the ML model
+* Modifying optimizations to reduce training resource requirements
+* Creating new storage-efficient data representations
+
+In the following section, we will review these on-device learning adaptation methods. More details on model optimizations can be found in the [Model Optimizations](./optimizations.qmd) chapter.
+
+### Reducing Model Complexity
+
+In this section, we will briefly discuss ways to reduce model complexity to adapt ML models on-device. For details of reducing model complexity, please refer to the Model Optimization Chapter.
+
+#### Traditional ML Algorithms
+
+Due to the compute and memory limitations of edge devices, select traditional ML algorithms are great candidates for on-device learning applications due to their lightweight nature. Some example algorithms with low resource footprints include Naive Bayes Classifier, Support Vector Machines (SVMs), Linear Regression, Logistic Regression, and select Decision Tree algorithms.
- - Benefits
- - Constraints
+With some refinements, these classical ML algorithms can be adapted to specific hardware architectures and perform simple tasks, and their low performance requirements make it easy to integrate continuous learning even on edge devices.
-## Continuous Learning
+#### Pruning
+Pruning is a technique used to reduce the size and complexity of an ML model to improve their efficiency and generalization performance. This is beneficial for training models on edge devices, where we want to minimize the resource usage while maintaining competitive accuracy.
-Explanation: Continuous learning is essential for embedded systems to adapt to new data and situations without requiring frequent updates from a central server.
+The primary goal of pruning is to remove parts of the model that do not contribute significantly to its predictive power while retaining the most informative aspects. In the context of decision trees, pruning involves removing some of the branches (subtrees) from the tree, leading to a smaller and simpler tree. In the context of DNN, pruning is used to reduce the number of neurons (units) or connections in the network.
- - Incremental Algorithms
- - Adaptability
+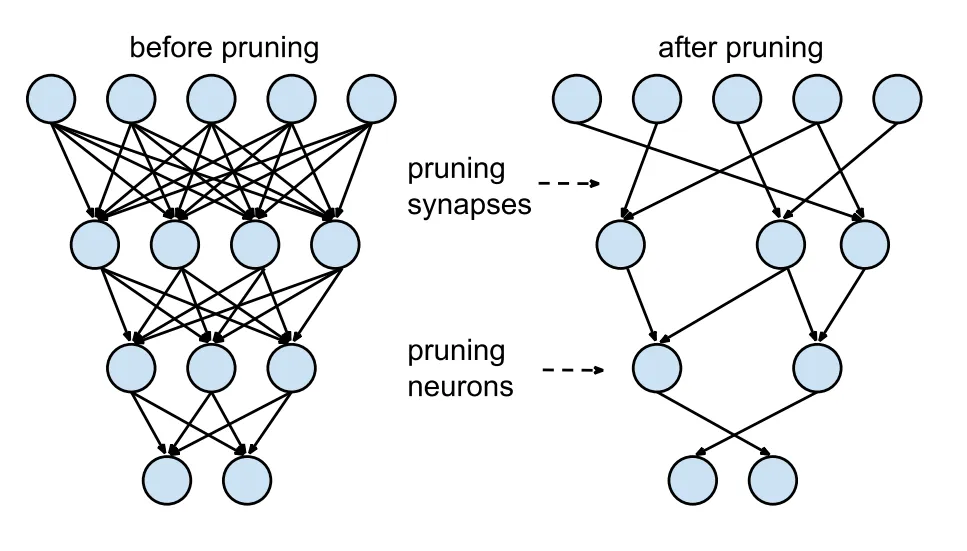)](images/ondevice_pruning.png)
-## Federated Machine Learning
+#### Reducing Complexity of Deep Learning Models
+Traditional cloud-based DNN frameworks have too much memory overhead to be used on-device. [For example](http://arxiv.org/abs/2206.15472), deep learning systems like PyTorch and TensorFlow require hundreds of megabytes of memory overhead when training models such as [MobilenetV2](https://openaccess.thecvf.com/content_cvpr_2018/html/Sandler_MobileNetV2_Inverted_Residuals_CVPR_2018_paper.html), and the overhead scales as the number of training parameters increases.
-Explanation: Federated learning allows multiple devices to collaborate in model training without sharing raw data, which is highly relevant for embedded systems concerned with data privacy.
- - Architecture
- - Optimization
+Traditional cloud-based DNN frameworks have too much memory overhead to be used on-device. For example, deep learning systems like PyTorch and TensorFlow require hundreds of megabytes of memory overhead when training models such as MobilenetV2-w0.35, and the overhead scales as the number of training parameters increases.
+
+Current research for lightweight DNNs mostly explore CNN architectures. Several bare-metal frameworks designed for running Neural Network on MCUs by keeping computational overhead and memory footprint low also exist. Some examples include MNN, TVM, and TensorFlow Lite. However, they can only perform inference during forward pass and lack support for back-propagation. While these models are designed for edge deployment, their reduction in model weights and architectural connections led to reduced resource requirements for continuous learning.
+
+The tradeoff between performance and model support is clear when adapting the most popular DNN systems. How do we adapt existing DNN models to resource-constrained settings while maintaining support for back-propagation and continuous learning? Latest research suggests algorithm and system codesign techniques that help reduce the resource consumption of ML training on edge devices. Utilizing techniques such as quantization-aware scaling (QAS), sparse updates, and other cutting edge techniques, on-device learning is possible on embedded systems with a few hundred kilobytes of RAM without additional memory while maintaining [high accuracy](http://arxiv.org/abs/2206.15472).
+
+### Modifying Optimization Processes
+
+Choosing the right optimization strategy is important for DNN training on-device, since this allows for the finding of a good local minimum. This optimization strategy must also consider limited memory and power since training occurs on-device.
+
+#### Quantization-Aware Scaling
+
+Quantization is a common method for reducing the memory footprint of DNN training. Although this could introduce new errors, these errors can be mitigated by designing a model to characterize this statistical error. For example, models could use stochastic rounding or introduce the quantization error into the gradient updates.
+
+A specific algorithmic technique is Quantization-Aware Scaling (QAS), used to improve the performance of neural networks on low-precision hardware, such as edge devices and mobile devices or TinyML systems, by adjusting the scale factors during the quantization process.
+
+As you recall from the Optimizations chapter, quantization is the process of mapping a continuous range of values to a discrete set of values. In the context of neural networks, quantization often involves reducing the precision of the weights and activations from 32-bit floating point to lower-precision formats such as 8-bit integers. This reduction in precision can significantly reduce the computational cost and memory footprint of the model, making it suitable for deployment on low-precision hardware.
+
+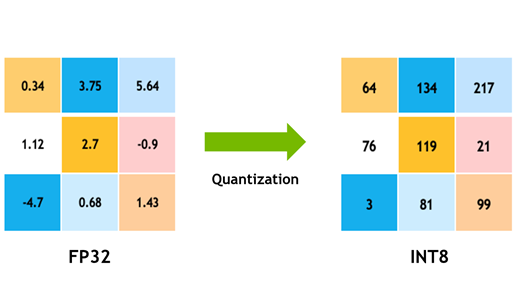)](images/ondevice_quantization_matrix.png)
+
+However, the quantization process can also introduce quantization errors that can degrade the performance of the model. Quantization-aware scaling is a technique that aims to minimize these errors by adjusting the scale factors used in the quantization process.
+
+The QAS process involves two main steps:
+
+* **Quantization-aware training:** In this step, the neural network is trained with quantization in mind, using simulated quantization to mimic the effects of quantization during the forward and backward passes. This allows the model to learn to compensate for the quantization errors and improve its performance on low-precision hardware. Refer to QAT section in model optimizations for details.
+
+)](https://raw.githubusercontent.com/matlab-deep-learning/quantization-aware-training/main/images/ste.png)
+
+* **Quantization and scaling:** After training, the model is quantized to low-precision format, and the scale factors are adjusted to minimize the quantization errors. The scale factors are chosen based on the distribution of the weights and activations in the model, and are adjusted to ensure that the quantized values are within the range of the low-precision format.
+
+QAS is used to overcome the difficulties of optimizing models on tiny devices. Without needing hyperparamter tuning. QAS automatically scales tensor gradients with various bit-precisions. This in turn stabilizes the training process and matches the accuracy of floating-point precision.
+
+#### Sparse Updates
+
+Although QAS enables optimizing a quantized model, it uses a large amount of memory that is unrealistic for on-device training. So spare update is used to reduce the memory footprint of full backward computation. Instead of pruning weights for inference, sparse update prunes the gradient during backwards propagation to update the model sparsely. In other words, sparse update skips computing gradients of less important layers and sub-tensors.
+
+However, determining the optimal sparse update scheme given a constraining memory budget can be challenging due to the large search space. For example, the MCUNet model has 43 convolutional layers and a search space of approximately 1030. One technique to address this issue is contribution analysis. Contribution analysis measures the accuracy improvement from biases (updating the last few biases compared to only updating the classifier) and weights (updating the weight of one extra layer compared to only having a bias update). By trying to maximize these improvements, contribution analysis automatically derives an optimal sparse update scheme for enabling on-device training.
+
+#### Layer-Wise Training
+
+Other methods besides quantization can help optimize routines. One such method is layer-wise training. A significant memory consumer of DNN training is the end-to-end back-propagation. This requires all intermediate feature maps to be stored so the model can calculate gradients. An alternative to this approach that reduces the memory footprint of DNN training is sequential layer-by-layer training [@chen2016training]. Instead of training end-to-end, training a single layer at a time helps avoid having to store intermediate feature maps.
+
+#### Trading Computation for Memory
+
+The strategy of trading computation for memory involves releasing some of the memory being used to store intermediate results. Instead, these results can be recomputed as needed. Reducing memory in exchange for more computation is shown to reduce the memory footprint of DNN training to fit into almost any budget while also minimizing computational cost [@gruslys2016memory].
+
+### Developing New Data Representations
+
+The dimensionality and volume of the training data can significantly impact on-device adaptation. So another technique for adapting models onto resource-cosntrained devices is to represent datasets in a more efficient way.
+
+#### Data Compression
+
+The goal of data compression is to reach high accuracies while limiting the amount of training data. One method to achieve this is prioritizing sample complexity: the amount of training data required for the algorithm to reach a target accuracy [@dhar2021survey].
+
+Other more common methods of data compression focus on reducing the dimensionality and the volume of the training data. For example, an approach could take advantage of matrix sparsity to reduce the memory footprint of storing training data. Training data can be transformed into a lower-dimensional embedding and factorized into a dictionary matrix multiplied by a block-sparse coefficient matrix [@rouhani2017tinydl]. Another example could involve representing words from a large language training dataset in a more compressed vector format [@li2016lightrnn].
## Transfer Learning
-Explanation: Transfer learning enables a pre-trained model to adapt to new tasks with less data, which is beneficial for embedded systems where data might be scarce.
+Transfer learning is a ML technique where a model developed for a particular task is reused as the starting point for a model on a second task. In the context of on-device AI, transfer learning allows us to leverage pre-trained models that have already learned useful representations from large datasets, and fine-tune them for specific tasks using smaller datasets directly on the device. This can significantly reduce the computational resources and time required for training models from scratch.
+
+Let's take the example of a smart sensor application that uses on-device AI to recognize objects in images captured by the device. Traditionally, this would require sending the image data to a server, where a large neural network model processes the data and sends back the results. With on-device AI, the model is stored and runs directly on-device, eliminating the need to send data to a server.
+
+If we want to customize the model for the on-device characteristics, training a neural network model from scratch on the device would however be impractical due to the limited computational resources and battery life. This is where transfer learning comes in. Instead of training a model from scratch, we can take a pre-trained model, such as a convolutional neural network (CNN) or a transformer network that has been trained on a large dataset of images, and fine-tune it for our specific object recognition task. This fine-tuning can be done directly on the device using a smaller dataset of images relevant to the task. By leveraging the pre-trained model, we can reduce the computational resources and time required for training, while still achieving high accuracy for the object recognition task.
+
+Transfer learning plays an important role in making on-device AI practical by allowing us to leverage pre-trained models and fine-tune them for specific tasks, thereby reducing the computational resources and time required for training. The combination of on-device AI and transfer learning opens up new possibilities for AI applications that are more privacy-conscious and responsive to user needs.
+
+Transfer learning has revolutionized the way models are developed and deployed, both in the cloud and at the edge. Transfer learning is being used in the real world. One such example is the use of transfer learning to develop AI models that can detect and diagnose diseases from medical images, such as X-rays, MRI scans, and CT scans. For example, researchers at Stanford University developed a transfer learning model that can detect cancer in skin images with an accuracy of 97% [@esteva2017dermatologist]. This model was pre-trained on 1.28 million images to classify a broad range of objects, then specialized for cancer detection by training on a dermatologist-curated dataset of skin images.
+
+Implementation in production scenarios can be broadly categorized into two stages: pre-deployment and post-deployment.
+
+)](images/ondevice_transfer_learning_apps.png)
+
+### Pre-Deployment Specialization
+
+In the pre-deployment stage, transfer learning acts as a catalyst to expedite the development process. Here's how it typically works: Imagine we are creating a system to recognize different breeds of dogs. Rather than starting from scratch, we can utilize a pre-trained model that has already mastered the broader task of recognizing animals in images.
+
+This pre-trained model serves as a solid foundation and contains a wealth of knowledge acquired from extensive data. We then fine-tune this model using a specialized dataset containing images of various dog breeds. This fine-tuning process tailors the model to our specific need – identifying dog breeds with precision. Once fine-tuned and validated to meet performance criteria, this specialized model is then ready for deployment.
+
+Here's how it works in practice:
+
+* **Start with a Pre-Trained Model:** Begin by selecting a model that has already been trained on a comprehensive dataset, usually related to a general task. This model serves as the foundation for the task at hand.
+* **Fine-Tuning:** The pre-trained model is then fine-tuned on a smaller, more specialized dataset that is specific to the desired task. This step allows the model to adapt and specialize its knowledge to the specific requirements of the application.
+* **Validation:** After fine-tuning, the model is validated to ensure it meets the performance criteria for the specialized task.
+* **Deployment:** Once validated, the specialized model is then deployed into the production environment.
+
+This method significantly reduces the time and computational resources required to train a model from scratch [@pan2009survey]. By adopting transfer learning, embedded systems can achieve high accuracy on specialized tasks without the need to gather extensive data or expend significant computational resources on training from the ground up.
+
+### Post-Deployment Adaptation
+
+Deployment to a device need not mark the culmination of a ML model's educational trajectory. With the advent of transfer learning, we open the doors to the deployment of adaptive ML models to real-world scenarios, catering to the personalized needs of users.
+
+Consider a real-world application where a parent wishes to identify their child in a collection of images from a school event on their smartphone. In this scenario, the parent is faced with the challenge of locating their child amidst images of many other children. Here, transfer learning can be employed to fine-tune an embedded system's model to this unique and specialized task. Initially, the system might use a generic model trained to recognize faces in images. However, with transfer learning, the system can adapt this model to recognize the specific features of the user's child.
+
+Here's how it works:
+
+1. **Data Collection:** The embedded system gathers images that include the child, ideally with the parent's input to ensure accuracy and relevance. This can be done directly on the device, maintaining the user's data privacy.
+2. **Model Fine-Tuning:** The pre-existing face recognition model, which has been trained on a large and diverse dataset, is then fine-tuned using the newly collected images of the child. This process adapts the model to recognize the child's specific facial features, distinguishing them from other children in the images.
+3. **Validation:** The refined model is then validated to ensure it accurately recognizes the child in various images. This can involve the parent verifying the model's performance and providing feedback for further improvements.
+4. **Deployment:** Once validated, the adapted model is deployed on the device, enabling the parent to easily identify their child in images without having to sift through them manually.
+
+This on-the-fly customization enhances the model's efficacy for the individual user, ensuring that they benefit from ML personalization. This is in part how iPhotos or Google photos works when they ask us to recognize a face and then based on that information they index all the photos by that face. Because the learning and adaptation occur on the device itself, there are no risks to personal privacy. The parent's images are not uploaded to a cloud server or shared with third parties, protecting the family's privacy while still reaping the benefits of a personalized ML model. This approach represents a significant step forward in the quest to provide users with tailored ML solutions that respect and uphold their privacy.
+
+### Benefits
+
+Transfer learning has become an important technique in the field of ML and artificial intelligence, and it is particularly valuable for several reasons.
+
+1. **Data Scarcity:** In many real-world scenarios, acquiring a sufficiently large labeled dataset for training a ML model from scratch is challenging. Transfer learning mitigates this issue by allowing the use of pre-trained models that have already learned valuable features from a vast dataset.
+2. **Computational Expense:** Training a model from scratch requires significant computational resources and time, especially for complex models like deep neural networks. By using transfer learning, we can leverage the computation that has already been done during the training of the source model, thereby saving both time and computational power.
+3. **Limited Annotated Data:** For some specific tasks, there might be ample raw data available, but the process of labeling that data for supervised learning can be costly and time-consuming. Transfer learning enables us to utilize pre-trained models that have been trained on a related task with labeled data, hence requiring less annotated data for the new task.
+
+There are advantages to reusing the features:
+
+1. **Hierarchical Feature Learning:** Deep learning models, particularly Convolutional Neural Networks (CNNs), have the ability to learn hierarchical features. Lower layers typically learn generic features like edges and shapes, while higher layers learn more complex and task-specific features. Transfer learning allows us to reuse the generic features learned by a model and fine-tune the higher layers for our specific task.
+2. **Boosting Performance:** Transfer learning has been proven to boost the performance of models on tasks with limited data. The knowledge gained from the source task can provide a valuable starting point and lead to faster convergence and improved accuracy on the target task.
+
+### Core Concepts
+Understanding the core concepts of transfer learning is essential for effectively utilizing this powerful approach in ML. Here we'll break down some of the main principles and components that underlie the process of transfer learning.
+
+#### Source and Target Tasks
+
+In transfer learning, there are two main tasks involved: the source task and the target task. The source task is the task for which the model has already been trained and has learned valuable information. The target task is the new task we want the model to perform. The goal of transfer learning is to leverage the knowledge gained from the source task to improve performance on the target task.
+
+If we have a model trained to recognize various fruits in images (source task), and we want to create a new model to recognize different vegetables in images (target task), we can use transfer learning to leverage the knowledge gained during the fruit recognition task to improve the performance of the vegetable recognition model.
+
+#### Representation Transfer
+
+Representation transfer is about transferring the learned representations (features) from the source task to the target task. There are three main types of representation transfer:
+- Instance Transfer: This involves reusing the data instances from the source task in the target task.
+- Feature-Representation Transfer: This involves transferring the learned feature representations from the source task to the target task.
+- Parameter Transfer: This involves transferring the learned parameters (weights) of the model from the source task to the target task.
+
+In natural language processing, a model trained to understand the syntax and grammar of a language (source task) can have its learned representations transferred to a new model designed to perform sentiment analysis (target task).
+
+#### Fine-Tuning
+Fine-tuning is the process of adjusting the parameters of a pre-trained model to adapt it to the target task. This typically involves updating the weights of the model's layers, especially the last few layers, to make the model more relevant for the new task. In image classification, a model pre-trained on a general dataset like ImageNet (source task) can be fine-tuned by adjusting the weights of its layers to perform well on a specific classification task, like recognizing specific animal species (target task).
+
+#### Feature Extractions
+Feature extraction involves using a pre-trained model as a fixed feature extractor, where the output of the model's intermediate layers is used as features for the target task. This approach is particularly useful when the target task has a small dataset, as the pre-trained model's learned features can significantly enhance performance. In medical image analysis, a model pre-trained on a large dataset of general medical images (source task) can be used as a feature extractor to provide valuable features for a new model designed to recognize specific types of tumors in X-ray images (target task).
+
+### Types of Transfer Learning
+
+Transfer learning can be classified into three main types based on the nature of the source and target tasks and data. Let's explore each type in detail:
+
+#### Inductive Transfer Learning
+
+In inductive transfer learning, the goal is to learn the target predictive function with the help of source data. It typically involves fine-tuning a pre-trained model on the target task with available labeled data. A common example of inductive transfer learning is image classification tasks. For instance, a model pre-trained on the ImageNet dataset (source task) can be fine-tuned to classify specific types of birds (target task) using a smaller labeled dataset of bird images.
+
+#### Transductive Transfer Learning
+
+Transductive transfer learning involves using source and target data, but only the source task. The main aim is to transfer knowledge from the source domain to the target domain, even though the tasks remain the same. Sentiment analysis for different languages can serve as an example of transductive transfer learning. A model trained to perform sentiment analysis in English (source task) can be adapted to perform sentiment analysis in another language, like French (target task), by leveraging parallel datasets of English and French sentences with the same sentiments.
+
+#### Unsupervised Transfer Learning
- - Use Cases
- - Benefits
+Unsupervised transfer learning is used when the source and target tasks are related, but there is no labeled data available for the target task. The goal is to leverage the knowledge gained from the source task to improve performance on the target task, even without labeled data. An example of unsupervised transfer learning is topic modeling in text data. A model trained to extract topics from news articles (source task) can be adapted to extract topics from social media posts (target task) without needing labeled data for the social media posts.
-## Data Augmentation
+#### Comparison and Trade-offs
-Explanation: Data augmentation can enrich the training set, improving model performance, which is particularly useful when data is limited in embedded systems.
+By leveraging these different types of transfer learning, practitioners can choose the approach that best fits the nature of their tasks and available data, ultimately leading to more effective and efficient ML models. So in summary:
+- Inductive: different source and target tasks, different domains
+- Transductive: different source and target tasks, same domain
+- Unsupervised: unlabeled source data, transfers feature representations
- - Techniques
- - Role in On-Device Learning
+Here's a matrix that outlines in a bit more detail the similarities and differences between the types of transfer learning:
+
+| | Inductive Transfer Learning | Transductive Transfer Learning | Unsupervised Transfer Learning |
+|------------------------------|-----------------------------|--------------------------------|---------------------------------|
+| **Labeled Data for Target Task** | Required | Not Required | Not Required |
+| **Source Task** | Can be different | Same | Same or Different |
+| **Target Task** | Can be different | Same | Can be different |
+| **Objective** | Improve target task performance with source data | Transfer knowledge from source to target domain | Leverage source task to improve target task performance without labeled data |
+| **Example** | ImageNet to bird classification | Sentiment analysis in different languages | Topic modeling for different text data |
+
+### Constraints and Considerations
+
+When engaging in transfer learning, there are several factors that must be considered to ensure successful knowledge transfer and model performance. Here's a breakdown of some key factors:
+
+#### Domain Similarity
+
+Domain similarity refers to how closely related the source and target domains are. The more similar the domains, the more likely the transfer learning will be successful. Transferring knowledge from a model trained on images of outdoor scenes (source domain) to a new task that involves recognizing objects in indoor scenes (target domain) might be more successful than transferring knowledge from outdoor scenes to a task involving text analysis, as the domains (images vs. text) are quite different.
+
+#### Task Similarity
+
+Task similarity refers to how closely related the source and target tasks are. Similar tasks are likely to benefit more from transfer learning. A model trained to recognize different breeds of dogs (source task) can be more easily adapted to recognize different breeds of cats (target task) than it can be adapted to perform a completely different task like language translation.
+
+#### Data Quality and Quantity
+
+The quality and quantity of data available for the target task can significantly impact the success of transfer learning. More and high-quality data can result in better model performance. If we have a large dataset with clear, well-labeled images for our target task of recognizing specific bird species, the transfer learning process is likely to be more successful than if we have a small, noisy dataset.
+
+#### Feature Space Overlap
+
+Feature space overlap refers to how well the features learned by the source model align with the features needed for the target task. Greater overlap can lead to more successful transfer learning. A model trained on high-resolution images (source task) may not transfer well to a target task that involves low-resolution images, as the feature space (high-res vs. low-res) is different.
+
+#### Model Complexity
+
+The complexity of the source model can also impact the success of transfer learning. Sometimes, a simpler model might transfer better than a complex one, as it is less likely to overfit the source task. A simple convolutional neural network (CNN) model trained on image data (source task) may transfer more successfully to a new image classification task (target task) than a complex CNN with many layers, as the simpler model is less likely to overfit the source task.
+
+By considering these factors, ML practitioners can make informed decisions about when and how to utilize transfer learning, ultimately leading to more successful model performance on the target task. The success of transfer learning hinges on the degree of similarity between the source and target domains. There is risk of overfitting, especially when fine-tuning occurs on a limited dataset. On the computational front, it is worth noting that certain pre-trained models, owing to their size, might not comfortably fit into the memory constraints of some devices or may run prohibitively slowly. Over time, as data evolves, there is potential for model drift, indicating the need for periodic re-training or ongoing adaptation.
+
+## Federated Machine Learning {#sec-fl}
+
+Federated Learning Overview
+
+The modern internet is full of large networks of connected devices. Whether it’s cell phones, thermostats, smart speakers or any number of other IOT products, countless edge devices are a goldmine for hyper-personalized, rich data. However, with that rich data comes an assortment of problems with information transfer and privacy. Constructing a training dataset in the cloud from these devices would involve high volumes of bandwidth and cost inefficient data transfer and violate user's privacy.
+
+Federated learning offers a solution to these problems: train models partially on the edge devices and only communicate model updates to the cloud. In 2016, a team from Google designed architecture for federated learning that attempts to address these problems.
+
+In their initial paper Google outlines a principle federated learning algorithm called FederatedAveraging. Specifically, FederatedAveraging performs stochastic gradient descent (SGD) over several different edge devices. In this process, each device calculates a gradient $g_k = \nabla F_k(w_t)$ which is then applied to update the server side weights as (with $\eta$ as learning rate across $k$ clients):
+$$
+w_{t+1} \rightarrow w_t - \eta \sum_{k=1}^{K} \frac{n_k}{n}g_k
+$$
+This boils down the basic algorithm for federated learning on the right. For each round of training, the server takes a random set of the client devices and calls each client to train on its local batch using the most recent server side weights. Those weights then get returned to the server where they are collected individually then averaged to update the global model weights.
+
+)](images/ondevice_fed_averaging.png)
+
+With this proposed structure, there are a few key vectors for optimizing federated learning further. We will outline each in the following subsections.
+
+### Communication Efficiency
+
+
+One of the key bottlenecks in federated learning is communication. Every time a client trains the model, they must communicate back to the server their updates. Similarly, once the server has averaged all the updates, it must send them back to the client. On large networks of millions of devices, this incurs huge bandwidth and resource cost. As the field of federated learning advances, a few optimizations have been developed to minimize this communication. To address the footprint of the model, researchers have developed model compression techniques. In the client server protocol, federated learning can also minimize communication through selective sharing of updates on clients. Finally, efficient aggregation techniques can also streamline the communication process.
+
+### Model Compression
+
+In standard federated learning, the server must communicate the entire model to each client and then the client must send back all of the updated weights. This means that the easiest way to reduce both the memory and communication footprint on the client is to minimize the size of the model needed to be communicated. To do this, we can employ all of the previously discussed model optimization strategies.
+
+In 2022, another team at Google proposed wherein each client communicates via a compressed format and decompresses the model on the fly for training [@yang2023online], allocating and deallocating the full memory for the model only for a short period while training. The model is compressed through a range of various quantization strategies elaborated upon in their paper. Meanwhile the server can update the uncompressed model, decompressing and applying updates as they come in.
+
+### Selective Update Sharing
+
+There are a breadth of methods for selectively sharing updates. The general principle is that reducing the portion of the model that the clients are training on the edge reduces the memory necessary for training and the size of communication to the server. In basic federated learning, the client trains the entire model. This means that when a client sends an update to the server it has gradients for every weight in the network.
+
+However, we cannot just reduce communication by sending pieces of those gradients to the server from each client because the gradients are part of an entire update required to improve the model. Instead, you need to architecturally design the model such that the clients each train only a small portion of the broader model, reducing the total communication while still gaining the benefit of training on client data. A paper from the University of Sheffield applies this concept to a CNN by splitting the global model into two parts: an upper and lower part as shown below [@shi2022data].
+
+)](images/ondevice_split_model.png)
+
+ The lower part is designed to focus on generic features in the dataset while the upper part trained on those generic features is designed to be more sensitive to the activation maps. This means that the lower part of the model is trained through standard federated averaging across all of the clients. Meanwhile, the upper part of the model is trained entirely on the server side from the activation maps generated by the clients. This approach drastically reduces communication for the model while still making the network robust to various types of input found in the data on the client devices.
+
+### Optimized Aggregation
+
+In addition to reducing the communication overhead, optimizing the aggregation function can improve model training speed and accuracy in certain federated learning use cases. While the standard for aggregation is just averaging, there are various other approaches which can improve model efficiency, accuracy, and security. One alternative is clipped averaging which clips the model updates within a specific range. Another strategy to preserve security is differential privacy average aggregation. This approach integrates differential privacy into the aggregations tep to protect client identities. Each client adds a layer of random noise to their updates before communicating to the server. The server then updates the server with the noisy updates, meaning that the amount of noise needs to be tuned carefully to balance privacy and accuracy.
+
+In addition to security enhancing aggregation methods, there are several modifications to the aggregation methods that can improve training speed and performance by adding client metadata along with the weight updates. Momentum aggregation is a technique which helps address the convergence problem. In federated learning, client data can be extremely heterogeneous depending on the different environments devices are in. That means that many models with heterogeneous data may struggle to converge. Each client stores a momentum term locally which tracks the pace of change over several updates. With clients communicating this momentum, the server can factor in the rate of change of each update when changing the global model to accelerate convergence. Similarly, weighted aggregation can factoro in the client performance or other parameters like device type or network connection strength to adjust the weight with which the server should incorporate the model updates. Further description of specific aggregation algorithms are described by @moshawrab2023reviewing.
+
+### Handling non-IID Data
+
+When using federated learning to train a model across many client devices, it is convenient to consider the data to be independent and identically distributed (IID) across all clients. When data is IID, the model will converge faster and perform better because each local update on any given client is more representative of the broader dataset. This makes aggregation straightforward as you can directly average all clients. However, this is not how data often appears in the real world. Consider a few of the following ways in which data may be non-IID:
+
+- If you are learning on a set of health-monitor devices, different device models could mean different sensor qualities and properties. This means that low quality sensors and devices may produce data, and therefore model updates distinctly different than high quality ones
+
+- A smart keyboard trained to perform autocorrect. If you have a disproportionate amount of devices from a certain region the slang, sentence structure, or even language they were using could skew more model updates towards a certain style of typing
+
+- If you have wildlife sensors in remote areas, connectivity may not be equally distributed causing some clients in certain regions to be able to send more model updates than others. If those regions have different wildlife activity from certain species, that could skew the updates toward those animals
+
+There are a few approaches to addressing non-IID data in federated learning. One approach would be to change the aggregation algorithm. If you use a weighted aggregation algorithm, you can adjust based on different client properties like region, sensor properties, or connectivity [@zhao2018federated].
+
+### Client Selection
+
+Considering all of the factors influencing the efficacy of federated learning like IID data and communication, client selection is key component to ensuring a system trains well. Selecting the wrong clients can skew the dataset, resulting in non-IID data. Similarly, choosing clients randomly with bad network connections can slow down communication. Therefore, when selecting the right subset of clients, several key characteristics must be considered.
+
+When selecting clients, there are three main components to consider: data heterogeneity, resource allocation, and communication cost. To address data heterogeneity, we can select for clients on the previously proposed metrics in the non-IID section. In federated learning, all devices may not have the same amount of compute, resulting in some being more inefficient at training than others. When selecting a subset of clients for training, one must consider a balance of data heterogeneity and available resources. In an ideal scenario, you can always select the subset of clients with the greatest resources. However, this may skew your dataset so a balance must be struck. Communication differences add another layer to this, you do not want to be bottlenecked by waiting for devices with poor connections to transmit their entire updates. Therefore, you must also consider choosing a subset of diverse yet well-connected devices.
+
+### An Example of Deployed Federated Learning: G board
+
+A primary example of a deployed federated learning system is Google’s Keyboard, Gboard, for android devices. In their implementation of federated learning for the keyboard, Google focused on employing differential privacy techniques to protect the user’s data and identity. Gboard leverages language models for several key features such as Next Word Prediction (NWP), Smart Compose (SC), and On-The-Fly rescoring (OTF) [@xu2023federated].
+
+NWP will anticipate the next word the user is trying to type based on the previous one. SC gives inline suggestions to speed up the typing based on each character. OTF will re-rank the proposed next words based on the active typing process. All three of these models need to run quickly on the edge and federated learning can accelerate training on the users' data. However, uploading every word a user typed to the cloud for training would be a massive privacy violation. Therefore, federated learning with an emphasis on differential privacy protects the user while still enabling a better user experience.
+
+![Examples of Google G Board Features (Credit: (Zheng et al., 2023)[https://arxiv.org/abs/2305.18465])](images/ondevice_gboard_example.png)
+
+To accomplish this goal, Google employed their algorithm DP-FTRL which provides a formal guarantee that trained models will not memorize specific user data or identities. DP-FTRL combined with secure aggregation, a strategy of encrypting model updates, provides an optimal balance of privacy and utility. Furthermore, adaptive clipping is applied in the aggregation process to limit the impact of individual users on the global model. Through a combination of all of these techniques, Google can continuously refine their keyboard while preserving user privacy in a formally provable way.
+
+![Google's System Design for Differential Privacy in G Board (Credit: (Zheng et al., 2023)[https://arxiv.org/abs/2305.18465])](images/ondevice_gboard_approach.png)
+
+### Benchmarking for Federated Learning: MedPerf
+
+One of the richest examples of data on the edge is medical devices. These devices store some of the most personal data on users but offer huge advances in personalized treatment and better accuracy in medical AI. Given these two factors, medical devices are the perfect use case for federated learning. [MedPerf](https://doi.org/10.1038/s42256-023-00652-2) is an open source platform used to benchmark models using federated evaluation [@karargyris2023federated]. Instead of just training models via federated learning, MedPerf takes the model to edge devices to test it against personalized data while preserving privacy. In this way a benchmark committee can evaluate various models in the real world on edge devices while still preserving patient anonymity.
## Security Concerns
-Explanation: Security is a significant concern for any system that performs learning on-device, as it may expose vulnerabilities.
+Performing ML model training and adaptation on end-user devices also introduces security risks that must be addressed. Some key security concerns include:
+
+- **Exposure of private data**: Training data may be leaked or stolen from devices
+- **Data poisoning**: Adversaries can manipulate training data to degrade model performance
+- **Model extraction**: Attackers may attempt to steal trained model parameters
+- **Membership inference**: Models may reveal participation of specific users' data
+- **Evasion attacks**: Specially crafted inputs can cause misclassification
+
+Any system that performs learning on-device introduces security concerns, as it may expose vulnerabilities in larger scale models. There are numerous security risks associated with any ML model, but these risks have specific consequences for on-device learning. Fortunately, there are methods to mitigate these risks to improve the real-world performance of on-device learning.
+
+### Data Poisoning
+On-device ML introduces unique data security challenges compared to traditional cloud-based training. In particular, data poisoning attacks pose a serious threat during on-device learning. Adversaries can manipulate training data to degrade model performance when deployed.
+
+Several data poisoning attack techniques exist:
+
+* **Label Flipping:** It involves applying incorrect labels to samples. For instance, in image classification, cat photos may be labeled as dogs to confuse the model. Flipping even [10% of labels](https://proceedings.mlr.press/v139/schwarzschild21a.html) can have significant consequences on the model.
+* **Data Insertion:** It introduces fake or distorted inputs into the training set. This could include pixelated images, noisy audio, or garbled text.
+* **Logic Corruption:** This alters the underlying [patterns](https://www.worldscientific.com/doi/10.1142/S0218001414600027) in data to mislead the model. In sentiment analysis, highly negative reviews may be marked positive through this technique. For this reason, recent surveys have shown that many companies are more [afraid of data poisoning](https://proceedings.mlr.press/v139/schwarzschild21a.html) than other adversarial ML concerns.
+
+What makes data poisoning alarming is how it exploits the discrepancy between curated datasets and live training data. Consider a cat photo dataset collected from the internet. In the weeks later when this data trains a model on-device, new cat photos on the web differ significantly.
+
+With data poisoning, attackers purchase domains and upload content that influences a portion of the training data. Even small data changes significantly impact the model's learned behavior. Consequently, poisoning can instill racist, sexist, or other harmful biases if unchecked.
+
+[Microsoft Tay](https://en.wikipedia.org/wiki/Tay_(chatbot)) was a chatbot launched by Microsoft in 2016. It was designed to learn from its interactions with users on social media platforms like Twitter. Unfortunately, Microsoft Tay became a prime example of data poisoning in ML models. Within 24 hours of its launch, Microsoft had to take Tay offline because it had started producing offensive and inappropriate messages, including hate speech and racist comments. This occurred because some users on social media intentionally fed Tay with harmful and offensive input, which the chatbot then learned from and incorporated into its responses.
+
+This incident is a clear example of data poisoning because malicious actors intentionally manipulated the data used to train and inform the chatbot's responses. The data poisoning resulted in the chatbot adopting harmful biases and producing output that was not intended by its developers. It demonstrates how even small amounts of maliciously crafted data can have a significant impact on the behavior of ML models, and highlights the importance of implementing robust data filtering and validation mechanisms to prevent such incidents from occurring.
+
+The real-world impacts of such biases could be dangerous. Rigorous data validation, anomaly detection, and tracking of data provenance are critical defensive measures. Adopting frameworks like Five Safes ensures models are trained on high-quality, representative data [@desai2016five].
+
+Data poisoning is a pressing concern for secure on-device learning, since data at the endpoint cannot be easily monitored in real-time and if models are allowed to adapt on their own then we run the risk of the device acting malicously. But continued research in adversarial ML aims to develop robust solutions to detect and mitigate such data attacks.
+
+### Adversarial Attacks
+
+During the training phase, attackers might inject malicious data into the training dataset, which can subtly alter the model's behavior. For example, an attacker could add images of cats that are labeled as dogs into a dataset used to train an image classification model. If done cleverly, the model's accuracy might not significantly drop, and the attack could go unnoticed. The model would then incorrectly classify some cats as dogs, which could have consequences depending on the application.
+
+In an embedded security camera system, for instance, this could allow an intruder to avoid detection by wearing a specific pattern that the model has been tricked into classifying as non-threatening.
- - Risks
- - Mitigation
+During the inference phase, attackers can use adversarial examples to fool the model. Adversarial examples are inputs that have been slightly altered in a way that causes the model to make incorrect predictions. For instance, an attacker might add a small amount of noise to an image in a way that causes a face recognition system to misidentify a person. These attacks can be particularly concerning in applications where safety is at stake, such as autonomous vehicles. In the example you mentioned, the researchers were able to cause a traffic sign recognition system to misclassify a stop sign as a speed sign. This type of misclassification could potentially lead to accidents if it occurred in a real-world autonomous driving system.
+
+To mitigate these risks, several defenses can be employed:
+
+* **Data Validation and Sanitization:** Before incorporating new data into the training dataset, it should be thoroughly validated and sanitized to ensure it is not malicious.
+* **Adversarial Training:** The model can be trained on adversarial examples to make it more robust to these types of attacks.
+* **Input Validation:** During inference, inputs should be validated to ensure they have not been manipulated to create adversarial examples.
+* **Regular Auditing and Monitoring:** Regularly auditing and monitoring the model's behavior can help to detect and mitigate adversarial attacks. In the context of tiny ML systems, this is easier said than done, because it is often hard to monitor embedded ML systems at the endpoint due to communication bandwidth limitations and so forth, which we will discuss in the MLOps chapter.
+
+By understanding the potential risks and implementing these defenses, we can help to secure on-device training at the endpoint/edge and mitigate the impact of adversarial attacks. Most people easily confuse data poisoning and adversarial attacks. So here is a table comparing data poisoning and adversarial attacks:
+
+| Aspect | Data Poisoning | Adversarial Attacks |
+|--------------------|-----------------------------------------|-------------------------------------------|
+| **Timing** | Training phase | Inference phase |
+| **Target** | Training data | Input data |
+| **Goal** | Negatively affect model's performance | Cause incorrect predictions |
+| **Method** | Insert malicious examples into training data, often with incorrect labels | Add carefully crafted noise to input data |
+| **Example** | Adding images of cats labeled as dogs to a dataset used for training an image classification model | Adding a small amount of noise to an image in a way that causes a face recognition system to misidentify a person |
+| **Potential Effects** | Model learns incorrect patterns and makes incorrect predictions | Immediate and potentially dangerous incorrect predictions |
+| **Applications Affected** | Any ML model | Autonomous vehicles, security systems, etc. |
+
+### Model Inversion
+
+Model inversion attacks are a privacy threat to on-device machine learning models trained on sensitive user data [@nguyen2023re]. Understanding this attack vector and mitigation strategies will be important for building secure and ethical on-device AI. For example, imagine an iPhone app uses on-device learning to categorize photos in your camera roll into groups like "beach", "food", or "selfies" for easier searching.
+
+The on-device model may be pretrained by Apple on a dataset of iCloud photos from consenting users. A malicious attacker could attempt to extract parts of those original iCloud training photos using model inversion. Specifically, the attacker feeds crafted synthetic inputs into the on-device photo classifier. By tweaking the synthetic inputs and observing how the model categorizes them, they can refine the inputs until they reconstruct copies of the original training data - like a beach photo from a user's iCloud. Now the attacker has breached that user's privacy by obtaining one of their personal photos without consent. This demonstrates why model inversion is dangerous - it can potentially leak highly sensitive training data.
+
+Photos are an especially high-risk data type because they often contain identifiable people, location information, and private moments. But the same attack methodology could apply to other personal data like audio recordings, text messages, or users' health data.
+
+To defend against model inversion, one would need to take precautions like adding noise to the model outputs or using privacy-preserving machine learning techniques like [federated learning](@sec-fl) to train the on-device model. The goal is to prevent attackers from being able to reconstruct the original training data.
+
+
+### On-Device Learning Security Concerns
+
+While data poisoning and adversarial attacks are common concerns for ML models in general, on-device learning introduces unique security risks. When on-device variants of large-scale models are published, adversaries can exploit these smaller models to attack their larger counterparts. Research has demonstrated that as on-device models and full-scale models become more similar, the vulnerability of the original large-scale models increases significantly. For instance, evaluations across 19 Deep Neural Networks (DNNs) revealed that exploiting on-device models could increase the vulnerability of the original large-scale models by [up to 100 times](http://arxiv.org/abs/2212.13700).
+
+There are three primary types of security risks specific to on-device learning:
+
+- **Transfer-Based Attacks**: These attacks exploit the transferability property between a surrogate model (an approximation of the target model, similar to an on-device model) and a remote target model (the original full-scale model). Attackers generate adversarial examples using the surrogate model, which can then be used to deceive the target model. For example, imagine an on-device model designed to identify spam emails. An attacker could use this model to generate a spam email that is not detected by the larger, full-scale email filtering system.
+
+- **Optimization-Based Attacks**: These attacks generate adversarial examples for transfer-based attacks using some form of objective function, and iteratively modify inputs to achieve the desired outcome. Gradient estimation attacks, for example, approximate the model’s gradient using query outputs (such as softmax confidence scores), while gradient-free attacks use the model’s final decision (the predicted class) to approximate the gradient, albeit requiring many more queries.
+
+- **Query Attacks with Transfer Priors**: These attacks combine elements of transfer-based and optimization-based attacks. They reverse engineer on-device models to serve as surrogates for the target full-scale model. In other words, attackers use the smaller on-device model to understand how the larger model works, and then use this knowledge to attack the full-scale model.
+
+By understanding these specific risks associated with on-device learning, we can develop more robust security protocols to protect both on-device and full-scale models from potential attacks.
+
+### Mitigation of On-Device Learning Risks
+
+To mitigate the numerous security risks associated with on-device learning, a variety of methods can be employed. These methods may be specific to the type of attack or serve as a general tool to bolster security.
+
+One strategy to reduce security risks is to diminish the similarity between on-device models and full-scale models, thereby reducing transferability by up to 90%. This method, known as similarity-unpairing, addresses the problem that arises when adversaries exploit the input-gradient similarity between the two models. By fine-tuning the full-scale model to create a new version with similar accuracy but different input gradients, we can then construct the on-device model by quantizing this updated full-scale model. This unpairing reduces the vulnerability of on-device models by limiting the exposure of the original full-scale model. Importantly, the order of finetuning and quantization can be varied while still achieving risk mitigation [@hong2023publishing].
+
+To tackle data poisoning, it is imperative to source datasets from trusted and reliable [vendors](https://www.eetimes.com/cybersecurity-threats-loom-over-endpoint-ai-systems/?_gl=1%2A17zgs0d%2A_ga%2AMTY0MzA1MTAyNS4xNjk4MDgyNzc1%2A_ga_ZLV02RYCZ8%2AMTY5ODA4Mjc3NS4xLjAuMTY5ODA4Mjc3NS42MC4wLjA).
+
+In combating adversarial attacks, several strategies can be employed. A proactive approach involves generating adversarial examples and incorporating them into the model's training dataset, thereby fortifying the model against such attacks. Tools like [CleverHans](http://github.com/cleverhans-lab/cleverhans), an open-source training library, are instrumental in creating adversarial examples. Defense distillation is another effective strategy, wherein the on-device model outputs probabilities of different classifications rather than definitive decisions [@hong2023publishing], making it more challenging for adversarial examples to exploit the model.
+
+The theft of intellectual property is another significant concern when deploying on-device models. Intellectual property theft is a concern when deploying on-device models, as adversaries may attempt to reverse-engineer the model to steal the underlying technology. To safeguard against intellectual property theft, the binary executable of the trained model should be stored on a microcontroller unit with encrypted software and secured physical interfaces of the chip. Furthermore, the final dataset used for training the model should be kept [private](https://www.eetimes.com/cybersecurity-threats-loom-over-endpoint-ai-systems/?_gl=1%2A17zgs0d%2A_ga%2AMTY0MzA1MTAyNS4xNjk4MDgyNzc1%2A_ga_ZLV02RYCZ8%2AMTY5ODA4Mjc3NS4xLjAuMTY5ODA4Mjc3NS42MC4wLjA).
+
+Furthermore, on-device models often utilize well-known or open-source datasets, such as MobileNet's Visual Wake Words. As such, it is important to maintain the [privacy of the final dataset](http://arxiv.org/abs/2212.13700) used for training the model. Additionally, protecting the data augmentation process and incorporating specific use cases can minimize the risk of reverse-engineering an on-device model.
+
+Lastly, the Adversarial Threat Landscape for Artificial-Intelligence Systems ([ATLAS](https://atlas.mitre.org/)) serves as a valuable matrix tool that helps assess the risk profile of on-device models, empowering developers to identify and [mitigate](https://www.eetimes.com/cybersecurity-threats-loom-over-endpoint-ai-systems/?_gl=1%2A17zgs0d%2A_ga%2AMTY0MzA1MTAyNS4xNjk4MDgyNzc1%2A_ga_ZLV02RYCZ8%2AMTY5ODA4Mjc3NS4xLjAuMTY5ODA4Mjc3NS42MC4wLjA) potential risks proactively.
+
+### Securing Training Data
+There are a variety of different ways to secure on-device training data. Each of these concepts in itself is really deep and could be worth a class by itself. So here we'll briefly allude to those concepts so you're aware about what to learn further.
+
+#### Encryption
+
+Encryption serves as the first line of defense for training data. This involves implementing end-to-end encryption for both local storage on devices and communication channels to prevent unauthorized access to raw training data. Trusted execution environments, such as [Intel SGX](https://www.intel.com/content/www/us/en/architecture-and-technology/software-guard-extensions.html) and [ARM TrustZone](https://www.arm.com/technologies/trustzone-for-cortex-a#:~:text=Arm%20TrustZone%20technology%20offers%20an,trust%20based%20on%20PSA%20guidelines.), are essential for facilitating secure training on encrypted data.
+
+Additionally, when aggregating updates from multiple devices, secure multi-party computation protocols can be employed to enhance security [@kairouz2015secure]. A practical application of this is in collaborative on-device learning, where cryptographic privacy-preserving aggregation of user model updates can be implemented. This technique effectively hides individual user data even during the aggregation phase.
+
+#### Differential Privacy
+
+Differential privacy is another crucial strategy for protecting training data. By injecting calibrated statistical noise into the data, we can mask individual records while still extracting valuable population patterns [@dwork2014algorithmic]. Managing the privacy budget across multiple training iterations and reducing noise as the model converges is also vital [@abadi2016deep]. Methods such as formally provable differential privacy, which may include adding Laplace or Gaussian noise scaled to the dataset's sensitivity, can be employed.
+
+#### Anomaly Detection
+
+Anomaly detection plays a pivotal role in identifying and mitigating potential data poisoning attacks. This can be achieved through statistical analyses like Principal Component Analysis (PCA) and clustering, which help to detect deviations in aggregated training data. Time-series methods such as [Cumulative Sum (CUSUM)](https://en.wikipedia.org/wiki/CUSUM) charts are useful for identifying shifts indicative of potential poisoning. Comparing current data distributions with previously seen clean data distributions can also help to flag anomalies. Moreover, suspected poisoned batches should be removed from the training update aggregation process. For example, spot checks on subsets of training images on devices can be conducted using photoDNA hashes to identify poisoned inputs.
+
+#### Input Data Validation
+
+Lastly, input data validation is essential for ensuring the integrity and validity of input data before it is fed into the training model, thereby protecting against adversarial payloads. Similarity measures, such as cosine distance, can be employed to catch inputs that deviate significantly from the expected distribution. Suspicious inputs that may contain adversarial payloads should be quarantined and sanitized. Furthermore, parser access to training data should be restricted to validated code paths only. Leveraging hardware security features, such as ARM Pointer Authentication, can prevent memory corruption (ARM Limited, 2023). An example of this is implementing input integrity checks on audio training data used by smart speakers before processing by the speech recognition model [@chen2023learning].
+
+## On-Device Training Frameworks
+
+Embedded inference frameworks like TF-Lite Micro [@david2021tensorflow], TVM [@chen2018tvm], and MCUNet [@lin2020mcunet] provide a slim runtime for running neural network models on microcontrollers and other resource-constrained devices. However, they don't support on-device training. Training requires its own set of specialized tools due to the impact of quantization on gradient calculation and the memory footprint of backpropagation [@lin2022device].
+
+In recent years, there are a handful of tools and frameworks that have started to emerge that enable on-device training, and these include Tiny Training Engine [@lin2022device], TinyTL [@cai2020tinytl], and TinyTrain [@kwon2023tinytrain].
+
+### Tiny Training Engine
+
+Tiny Training Engine (TTE) uses several techniques to optimize memory usage and speed up the training process. First, TTE performs graph optimization like pruning and sparse updates to reduce memory requirements and accelerate computations. Second, TTE offloads the automatic differentiation to compile time instead of runtime. This significantly reduces overhead during training.
+
+
+
+Specifically, TTE follows four main steps:
+
+- During compile time, TTE traces the forward propagation graph and derives the corresponding backward graph for backpropagation. This allows [differentiation](https://harvard-edge.github.io/cs249r_book/frameworks.html#differentiable-programming) to happen at compile time rather than runtime.
+- TTE prunes any nodes representing frozen weights from the backward graph. Frozen weights are weights that are not updated during training to reduce certain neurons' impact. Pruning their nodes saves memory.
+- TTE reorders the gradient descent operators to interleave them with the backward pass computations. This scheduling minimizes memory footprints.
+- TTE uses code generation to compile the optimized forward and backward graphs, which are then deployed for on-device training.
+
+### Tiny Transfer Learning
+
+Tiny Transfer Learning (TinyTL) enables memory-efficient on-device training through a technique called weight freezing. During training, much of the memory bottleneck comes from storing intermediate activations and updating the weights in the neural network.
+
+To reduce this memory overhead, TinyTL freezes the majority of the weights so they do not need to be updated during training. This eliminates the need to store intermediate activations for frozen parts of the network. TinyTL only fine-tunes the bias terms, which are much smaller than the weights.
+
+
+
+Freezing weights is applicable not just to fully-connected layers but also to convolutional and normalization layers. However, only adapting the biases limits the model's ability to learn and adapt to new data.
+
+To increase adaptability without much additional memory, TinyTL uses a small residual learning model. This refines the intermediate feature maps to produce better outputs, even with fixed weights. The residual model introduces minimal overhead - less than 3.8% on top of the base model.
+
+By freezing most weights TinyTL significantly cuts down memory usage during on-device training. The residual model then allows it to still adapt and learn effectively for the task. The combined approach provides memory-efficient on-device training with minimal impact on model accuracy.
+
+### Tiny Train
+
+TinyTrain significantly reduces the time required for on-device training by selectively updating only certain parts of the model. It does this using a technique called task-adaptive sparse updating.
+
+Based on the user data, memory, and compute available on the device, TinyTrain dynamically chooses which layers of the neural network to update during training. This layer selection is optimized to reduce computation and memory usage while maintaining high accuracy.
+
+
+
+More specifically, TinyTrain first does offline pretraining of the model. During pretraining, it not only trains the model on the task data but also meta-trains the model. Meta-training means training the model on metadata about the training process itself. This meta-learning improves the model's ability to adapt accurately even when limited data is available for the target task.
+
+Then, during the online adaptation stage when the model is being customized on the device, TinyTrain performs task-adaptive sparse updates. Using the criteria around the device's capabilities, it selects only certain layers to update through backpropagation. The layers are chosen to balance accuracy, memory usage, and computation time.
+
+By sparsely updating layers tailored to the device and task, TinyTrain is able to significantly reduce on-device training time and resource usage. The offline meta-training also improves accuracy when adapting with limited data. Together, these methods enable fast, efficient, and accurate on-device training.
+
+### Comparison
+
+
+Here is a table summarizing the key similarities and differences between the Tiny Training Engine, TinyTL, and TinyTrain frameworks:
+
+| Framework | Similarities | Differences |
+|-|-|-|
+| Tiny Training Engine | - On-device training
- Optimize memory & computation
- Leverage pruning, sparsity, etc. | - Traces forward & backward graphs
- Prunes frozen weights
- Interleaves backprop & gradients
- Code generation|
+| TinyTL | - On-device training
- Optimize memory & computation
- Leverage freezing, sparsity, etc. | - Freezes most weights
- Only adapts biases
- Uses residual model |
+| TinyTrain | - On-device training
- Optimize memory & computation
- Leverage sparsity, etc. | - Meta-training in pretraining
- Task-adaptive sparse updating
- Selective layer updating |
## Conclusion
- - Key Takeaways
\ No newline at end of file
+The concept of on-device learning is increasingly important for increasing the usability and scalability of TinyML. This chapter explored the intricacies of on-device learning, exploring its advantages and limitations, adaptation strategies, key related algorithms and techniques, security implications, and existing and emerging on-device training frameworks.
+
+On-device learning is, undoubtedly, a groundbreaking paradigm that brings forth numerous advantages for embedded and edge ML deployments. By performing training directly on the endpoint devices, on-device learning obviates the need for continuous cloud connectivity, making it particularly well-suited for IoT and edge computing applications. It comes with benefits such as improved privacy, ease of compliance, and resource efficiency. At the same time, on-device learning faces limitations related to hardware constraints, limited data size, and reduced model accuracy and generalization.
+
+Mechanisms such as reduced model complexity, optimization and data compression techniques, and related learning methods such as transfer learning and federated learning allow models to adapt to learn and evolve under resource constraints, thus serving as the bedrock for effective ML on edge devices.
+
+The critical security concerns in on-device learning highlighted in this chapter, ranging from data poisoning and adversarial attacks to specific risks introduced by on-device learning, must be addressed in real workloads for on-device learning to be a viable paradigm. Effective mitigation strategies, such as data validation, encryption, differential privacy, anomaly detection, and input data validation, are crucial to safeguard on-device learning systems from these threats.
+
+The emergence of specialized on-device training frameworks like Tiny Training Engine, Tiny Transfer Learning, and Tiny Train presents practical tools to enable efficient on-device training. These frameworks employ various techniques to optimize memory usage, reduce computational overhead, and streamline the on-device training process.
+
+In conclusion, on-device learning stands at the forefront of TinyML, promising a future where models can autonomously acquire knowledge and adapt to changing environments on edge devices. The application of on-device learning has the potential to revolutionize various domains, including healthcare, industrial IoT, and smart cities. However, the transformative potential of on-device learning must be balanced with robust security measures to protect against data breaches and adversarial threats. Embracing innovative on-device training frameworks and implementing stringent security protocols are key steps in unlocking the full potential of on-device learning. As this technology continues to evolve, it holds the promise of making our devices smarter, more responsive, and better integrated into our daily lives.
diff --git a/ops.qmd b/ops.qmd
index 316b1360..3c2266d8 100644
--- a/ops.qmd
+++ b/ops.qmd
@@ -1,6 +1,6 @@
# Embedded AIOps
-
+
::: {.callout-tip}
## Learning Objectives
diff --git a/references.bib b/references.bib
index 9e7bf34d..ee76ca3e 100644
--- a/references.bib
+++ b/references.bib
@@ -1,1312 +1,1970 @@
-@inproceedings{lin2014microsoft,
- title={Microsoft coco: Common objects in context},
- author={Lin, Tsung-Yi and Maire, Michael and Belongie, Serge and Hays, James and Perona, Pietro and Ramanan, Deva and Doll{\'a}r, Piotr and Zitnick, C Lawrence},
- booktitle={Computer Vision--ECCV 2014: 13th European Conference, Zurich, Switzerland, September 6-12, 2014, Proceedings, Part V 13},
- pages={740--755},
- year={2014},
- organization={Springer}
+@inproceedings{abadi2016deep,
+ title = {Deep learning with differential privacy},
+ author = {Abadi, Martin and Chu, Andy and Goodfellow, Ian and McMahan, H Brendan and Mironov, Ilya and Talwar, Kunal and Zhang, Li},
+ year = 2016,
+ booktitle = {Proceedings of the 2016 ACM SIGSAC conference on computer and communications security},
+ pages = {308--318}
}
-@article{banbury2020benchmarking,
- title={Benchmarking tinyml systems: Challenges and direction},
- author={Banbury, Colby R and Reddi, Vijay Janapa and Lam, Max and Fu, William and Fazel, Amin and Holleman, Jeremy and Huang, Xinyuan and Hurtado, Robert and Kanter, David and Lokhmotov, Anton and others},
- journal={arXiv preprint arXiv:2003.04821},
- year={2020}
+@inproceedings{abadi2016tensorflow,
+ title = {$\{$TensorFlow$\}$: a system for $\{$Large-Scale$\}$ machine learning},
+ author = {Abadi, Mart{\'\i}n and Barham, Paul and Chen, Jianmin and Chen, Zhifeng and Davis, Andy and Dean, Jeffrey and Devin, Matthieu and Ghemawat, Sanjay and Irving, Geoffrey and Isard, Michael and others},
+ year = 2016,
+ booktitle = {12th USENIX symposium on operating systems design and implementation (OSDI 16)},
+ pages = {265--283}
}
-@misc{hinton2015distilling,
- title={Distilling the Knowledge in a Neural Network},
- author={Geoffrey Hinton and Oriol Vinyals and Jeff Dean},
- year={2015},
- eprint={1503.02531},
- archivePrefix={arXiv},
- primaryClass={stat.ML}
+@inproceedings{adolf2016fathom,
+ title = {Fathom: Reference workloads for modern deep learning methods},
+ author = {Adolf, Robert and Rama, Saketh and Reagen, Brandon and Wei, Gu-Yeon and Brooks, David},
+ year = 2016,
+ booktitle = {2016 IEEE International Symposium on Workload Characterization (IISWC)},
+ pages = {1--10},
+ organization = {IEEE}
}
-@inproceedings{gordon2018morphnet,
- title={Morphnet: Fast \& simple resource-constrained structure learning of deep networks},
- author={Gordon, Ariel and Eban, Elad and Nachum, Ofir and Chen, Bo and Wu, Hao and Yang, Tien-Ju and Choi, Edward},
- booktitle={Proceedings of the IEEE conference on computer vision and pattern recognition},
- pages={1586--1595},
- year={2018}
+@misc{al2016theano,
+ title = {Theano: A Python framework for fast computation of mathematical expressions},
+ author = {The Theano Development Team and Rami Al-Rfou and Guillaume Alain and Amjad Almahairi and Christof Angermueller and Dzmitry Bahdanau and Nicolas Ballas and Frédéric Bastien and Justin Bayer and Anatoly Belikov and Alexander Belopolsky and Yoshua Bengio and Arnaud Bergeron and James Bergstra and Valentin Bisson and Josh Bleecher Snyder and Nicolas Bouchard and Nicolas Boulanger-Lewandowski and Xavier Bouthillier and Alexandre de Brébisson and Olivier Breuleux and Pierre-Luc Carrier and Kyunghyun Cho and Jan Chorowski and Paul Christiano and Tim Cooijmans and Marc-Alexandre Côté and Myriam Côté and Aaron Courville and Yann N. Dauphin and Olivier Delalleau and Julien Demouth and Guillaume Desjardins and Sander Dieleman and Laurent Dinh and Mélanie Ducoffe and Vincent Dumoulin and Samira Ebrahimi Kahou and Dumitru Erhan and Ziye Fan and Orhan Firat and Mathieu Germain and Xavier Glorot and Ian Goodfellow and Matt Graham and Caglar Gulcehre and Philippe Hamel and Iban Harlouchet and Jean-Philippe Heng and Balázs Hidasi and Sina Honari and Arjun Jain and Sébastien Jean and Kai Jia and Mikhail Korobov and Vivek Kulkarni and Alex Lamb and Pascal Lamblin and Eric Larsen and César Laurent and Sean Lee and Simon Lefrancois and Simon Lemieux and Nicholas Léonard and Zhouhan Lin and Jesse A. Livezey and Cory Lorenz and Jeremiah Lowin and Qianli Ma and Pierre-Antoine Manzagol and Olivier Mastropietro and Robert T. McGibbon and Roland Memisevic and Bart van Merriënboer and Vincent Michalski and Mehdi Mirza and Alberto Orlandi and Christopher Pal and Razvan Pascanu and Mohammad Pezeshki and Colin Raffel and Daniel Renshaw and Matthew Rocklin and Adriana Romero and Markus Roth and Peter Sadowski and John Salvatier and François Savard and Jan Schlüter and John Schulman and Gabriel Schwartz and Iulian Vlad Serban and Dmitriy Serdyuk and Samira Shabanian and Étienne Simon and Sigurd Spieckermann and S. Ramana Subramanyam and Jakub Sygnowski and Jérémie Tanguay and Gijs van Tulder and Joseph Turian and Sebastian Urban and Pascal Vincent and Francesco Visin and Harm de Vries and David Warde-Farley and Dustin J. Webb and Matthew Willson and Kelvin Xu and Lijun Xue and Li Yao and Saizheng Zhang and Ying Zhang},
+ year = 2016,
+ eprint = {1605.02688},
+ archiveprefix = {arXiv},
+ primaryclass = {cs.SC}
}
-
-@article{lin2020mcunet,
- title={Mcunet: Tiny deep learning on iot devices},
- author={Lin, Ji and Chen, Wei-Ming and Lin, Yujun and Gan, Chuang and Han, Song and others},
- journal={Advances in Neural Information Processing Systems},
- volume={33},
- pages={11711--11722},
- year={2020}
+@article{Aledhari_Razzak_Parizi_Saeed_2020,
+ title = {Federated learning: A survey on enabling technologies, Protocols, and applications},
+ author = {Aledhari, Mohammed and Razzak, Rehma and Parizi, Reza M. and Saeed, Fahad},
+ year = 2020,
+ journal = {IEEE Access},
+ volume = 8,
+ pages = {140699–140725},
+ doi = {10.1109/access.2020.3013541}
}
-@inproceedings{tan2019mnasnet,
- title={Mnasnet: Platform-aware neural architecture search for mobile},
- author={Tan, Mingxing and Chen, Bo and Pang, Ruoming and Vasudevan, Vijay and Sandler, Mark and Howard, Andrew and Le, Quoc V},
- booktitle={Proceedings of the IEEE/CVF conference on computer vision and pattern recognition},
- pages={2820--2828},
- year={2019}
+@article{aljundi_gradient_nodate,
+ title = {Gradient based sample selection for online continual learning},
+ author = {Aljundi, Rahaf and Lin, Min and Goujaud, Baptiste and Bengio, Yoshua},
+ language = {en},
+ file = {Aljundi et al. - Gradient based sample selection for online continu.pdf:/Users/alex/Zotero/storage/GPHM4KY7/Aljundi et al. - Gradient based sample selection for online continu.pdf:application/pdf}
}
-@article{cai2018proxylessnas,
- title={Proxylessnas: Direct neural architecture search on target task and hardware},
- author={Cai, Han and Zhu, Ligeng and Han, Song},
- journal={arXiv preprint arXiv:1812.00332},
- year={2018}
+@inproceedings{altayeb2022classifying,
+ title = {Classifying mosquito wingbeat sound using TinyML},
+ author = {Altayeb, Moez and Zennaro, Marco and Rovai, Marcelo},
+ year = 2022,
+ booktitle = {Proceedings of the 2022 ACM Conference on Information Technology for Social Good},
+ pages = {132--137}
}
-@inproceedings{wu2019fbnet,
- title={Fbnet: Hardware-aware efficient convnet design via differentiable neural architecture search},
- author={Wu, Bichen and Dai, Xiaoliang and Zhang, Peizhao and Wang, Yanghan and Sun, Fei and Wu, Yiming and Tian, Yuandong and Vajda, Peter and Jia, Yangqing and Keutzer, Kurt},
- booktitle={Proceedings of the IEEE/CVF conference on computer vision and pattern recognition},
- pages={10734--10742},
- year={2019}
+@misc{amodei_ai_2018,
+ title = {{AI} and {Compute}},
+ author = {Amodei, Dario and Hernandez, Danny},
+ year = 2018,
+ month = may,
+ journal = {OpenAI Blog},
+ url = {https://openai.com/research/ai-and-compute}
}
-
-@article{xu2018alternating,
- title={Alternating multi-bit quantization for recurrent neural networks},
- author={Xu, Chen and Yao, Jianqiang and Lin, Zhouchen and Ou, Wenwu and Cao, Yuanbin and Wang, Zhirong and Zha, Hongbin},
- journal={arXiv preprint arXiv:1802.00150},
- year={2018}
+@inproceedings{antol2015vqa,
+ title = {Vqa: Visual question answering},
+ author = {Antol, Stanislaw and Agrawal, Aishwarya and Lu, Jiasen and Mitchell, Margaret and Batra, Dhruv and Zitnick, C Lawrence and Parikh, Devi},
+ year = 2015,
+ booktitle = {Proceedings of the IEEE international conference on computer vision},
+ pages = {2425--2433}
+}
+
+@article{app112211073,
+ title = {Hardware/Software Co-Design for TinyML Voice-Recognition Application on Resource Frugal Edge Devices},
+ author = {Kwon, Jisu and Park, Daejin},
+ year = 2021,
+ journal = {Applied Sciences},
+ volume = 11,
+ number = 22,
+ doi = {10.3390/app112211073},
+ issn = {2076-3417},
+ url = {https://www.mdpi.com/2076-3417/11/22/11073},
+ article-number = 11073,
+ abstract = {On-device artificial intelligence has attracted attention globally, and attempts to combine the internet of things and TinyML (machine learning) applications are increasing. Although most edge devices have limited resources, time and energy costs are important when running TinyML applications. In this paper, we propose a structure in which the part that preprocesses externally input data in the TinyML application is distributed to the hardware. These processes are performed using software in the microcontroller unit of an edge device. Furthermore, resistor–transistor logic, which perform not only windowing using the Hann function, but also acquire audio raw data, is added to the inter-integrated circuit sound module that collects audio data in the voice-recognition application. As a result of the experiment, the windowing function was excluded from the TinyML application of the embedded board. When the length of the hardware-implemented Hann window is 80 and the quantization degree is 2−5, the exclusion causes a decrease in the execution time of the front-end function and energy consumption by 8.06% and 3.27%, respectively.}
+}
+
+@article{Ardila_Branson_Davis_Henretty_Kohler_Meyer_Morais_Saunders_Tyers_Weber_2020,
+ title = {Common Voice: A Massively-Multilingual Speech Corpus},
+ author = {Ardila, Rosana and Branson, Megan and Davis, Kelly and Henretty, Michael and Kohler, Michael and Meyer, Josh and Morais, Reuben and Saunders, Lindsay and Tyers, Francis M. and Weber, Gregor},
+ year = 2020,
+ month = {May},
+ journal = {Proceedings of the 12th Conference on Language Resources and Evaluation},
+ pages = {4218–4222}
}
-@article{krishnamoorthi2018quantizing,
- title={Quantizing deep convolutional networks for efficient inference: A whitepaper},
- author={Krishnamoorthi, Raghuraman},
- journal={arXiv preprint arXiv:1806.08342},
- year={2018}
+@misc{awq,
+ title = {AWQ: Activation-aware Weight Quantization for LLM Compression and Acceleration},
+ author = {Lin and Tang, Tang and Yang, Dang and Gan, Han},
+ year = 2023,
+ doi = {10.48550/arXiv.2306.00978},
+ url = {https://arxiv.org/abs/2306.00978},
+ urldate = {2023-10-03},
+ abstract = {Large language models (LLMs) have shown excellent performance on various tasks, but the astronomical model size raises the hardware barrier for serving (memory size) and slows down token generation (memory bandwidth). In this paper, we propose Activation-aware Weight Quantization (AWQ), a hardware-friendly approach for LLM low-bit weight-only quantization. Our method is based on the observation that weights are not equally important: protecting only 1% of salient weights can greatly reduce quantization error. We then propose to search for the optimal perchannel scaling that protects the salient weights by observing the activation, not weights. AWQ does not rely on any backpropagation or reconstruction, so it can well preserve LLMs’ generalization ability on different domains and modalities, without overfitting to the calibration set. AWQ outperforms existing work on various language modeling and domain-specific benchmarks. Thanks to better generalization, it achieves excellent quantization performance for instruction-tuned LMs and, for the first time, multi-modal LMs. Alongside AWQ, we implement an efficient and flexible inference framework tailored for LLMs on the edge, offering more than 3× speedup over the Huggingface FP16 implementation on both desktop and mobile GPUs. It also democratizes the deployment of the 70B Llama-2 model on mobile GPU (NVIDIA Jetson Orin 64GB).}
}
-
-@article{iandola2016squeezenet,
- title={SqueezeNet: AlexNet-level accuracy with 50x fewer parameters and< 0.5 MB model size},
- author={Iandola, Forrest N and Han, Song and Moskewicz, Matthew W and Ashraf, Khalid and Dally, William J and Keutzer, Kurt},
- journal={arXiv preprint arXiv:1602.07360},
- year={2016}
+@inproceedings{bamoumen2022tinyml,
+ title = {How TinyML Can be Leveraged to Solve Environmental Problems: A Survey},
+ author = {Bamoumen, Hatim and Temouden, Anas and Benamar, Nabil and Chtouki, Yousra},
+ year = 2022,
+ booktitle = {2022 International Conference on Innovation and Intelligence for Informatics, Computing, and Technologies (3ICT)},
+ pages = {338--343},
+ organization = {IEEE}
}
+@article{banbury2020benchmarking,
+ title = {Benchmarking tinyml systems: Challenges and direction},
+ author = {Banbury, Colby R and Reddi, Vijay Janapa and Lam, Max and Fu, William and Fazel, Amin and Holleman, Jeremy and Huang, Xinyuan and Hurtado, Robert and Kanter, David and Lokhmotov, Anton and others},
+ year = 2020,
+ journal = {arXiv preprint arXiv:2003.04821}
+}
-@misc{tan2020efficientnet,
- title={EfficientNet: Rethinking Model Scaling for Convolutional Neural Networks},
- author={Mingxing Tan and Quoc V. Le},
- year={2020},
- eprint={1905.11946},
- archivePrefix={arXiv},
- primaryClass={cs.LG}
+@article{bank2023autoencoders,
+ title = {Autoencoders},
+ author = {Bank, Dor and Koenigstein, Noam and Giryes, Raja},
+ year = 2023,
+ journal = {Machine Learning for Data Science Handbook: Data Mining and Knowledge Discovery Handbook},
+ publisher = {Springer},
+ pages = {353--374}
}
-@misc{howard2017mobilenets,
- title={MobileNets: Efficient Convolutional Neural Networks for Mobile Vision Applications},
- author={Andrew G. Howard and Menglong Zhu and Bo Chen and Dmitry Kalenichenko and Weijun Wang and Tobias Weyand and Marco Andreetto and Hartwig Adam},
- year={2017},
- eprint={1704.04861},
- archivePrefix={arXiv},
- primaryClass={cs.CV}
+@book{barroso2019datacenter,
+ title = {The datacenter as a computer: Designing warehouse-scale machines},
+ author = {Barroso, Luiz Andr{\'e} and H{\"o}lzle, Urs and Ranganathan, Parthasarathy},
+ year = 2019,
+ publisher = {Springer Nature}
}
-@inproceedings{hendrycks2021natural,
- title={Natural adversarial examples},
- author={Hendrycks, Dan and Zhao, Kevin and Basart, Steven and Steinhardt, Jacob and Song, Dawn},
- booktitle={Proceedings of the IEEE/CVF Conference on Computer Vision and Pattern Recognition},
- pages={15262--15271},
- year={2021}
+@article{Bender_Friedman_2018,
+ title = {Data statements for natural language processing: Toward mitigating system bias and enabling better science},
+ author = {Bender, Emily M. and Friedman, Batya},
+ year = 2018,
+ journal = {Transactions of the Association for Computational Linguistics},
+ volume = 6,
+ pages = {587–604},
+ doi = {10.1162/tacl_a_00041}
}
-@inproceedings{xie2020adversarial,
- title={Adversarial examples improve image recognition},
- author={Xie, Cihang and Tan, Mingxing and Gong, Boqing and Wang, Jiang and Yuille, Alan L and Le, Quoc V},
- booktitle={Proceedings of the IEEE/CVF conference on computer vision and pattern recognition},
- pages={819--828},
- year={2020}
+@article{beyer2020we,
+ title = {Are we done with imagenet?},
+ author = {Beyer, Lucas and H{\'e}naff, Olivier J and Kolesnikov, Alexander and Zhai, Xiaohua and Oord, A{\"a}ron van den},
+ year = 2020,
+ journal = {arXiv preprint arXiv:2006.07159}
}
-@inproceedings{koh2021wilds,
- title={Wilds: A benchmark of in-the-wild distribution shifts},
- author={Koh, Pang Wei and Sagawa, Shiori and Marklund, Henrik and Xie, Sang Michael and Zhang, Marvin and Balsubramani, Akshay and Hu, Weihua and Yasunaga, Michihiro and Phillips, Richard Lanas and Gao, Irena and others},
- booktitle={International Conference on Machine Learning},
- pages={5637--5664},
- year={2021},
- organization={PMLR}
+@article{biggio2014pattern,
+ title = {Pattern recognition systems under attack: Design issues and research challenges},
+ author = {Biggio, Battista and Fumera, Giorgio and Roli, Fabio},
+ year = 2014,
+ journal = {International Journal of Pattern Recognition and Artificial Intelligence},
+ publisher = {World Scientific},
+ volume = 28,
+ number = {07},
+ pages = 1460002
}
-@inproceedings{antol2015vqa,
- title={Vqa: Visual question answering},
- author={Antol, Stanislaw and Agrawal, Aishwarya and Lu, Jiasen and Mitchell, Margaret and Batra, Dhruv and Zitnick, C Lawrence and Parikh, Devi},
- booktitle={Proceedings of the IEEE international conference on computer vision},
- pages={2425--2433},
- year={2015}
+@misc{blalock_what_2020,
+ title = {What is the {State} of {Neural} {Network} {Pruning}?},
+ author = {Blalock, Davis and Ortiz, Jose Javier Gonzalez and Frankle, Jonathan and Guttag, John},
+ year = 2020,
+ month = mar,
+ publisher = {arXiv},
+ doi = {10.48550/arXiv.2003.03033},
+ url = {http://arxiv.org/abs/2003.03033},
+ urldate = {2023-10-20},
+ note = {arXiv:2003.03033 [cs, stat]},
+ abstract = {Neural network pruning---the task of reducing the size of a network by removing parameters---has been the subject of a great deal of work in recent years. We provide a meta-analysis of the literature, including an overview of approaches to pruning and consistent findings in the literature. After aggregating results across 81 papers and pruning hundreds of models in controlled conditions, our clearest finding is that the community suffers from a lack of standardized benchmarks and metrics. This deficiency is substantial enough that it is hard to compare pruning techniques to one another or determine how much progress the field has made over the past three decades. To address this situation, we identify issues with current practices, suggest concrete remedies, and introduce ShrinkBench, an open-source framework to facilitate standardized evaluations of pruning methods. We use ShrinkBench to compare various pruning techniques and show that its comprehensive evaluation can prevent common pitfalls when comparing pruning methods.},
+ keywords = {Computer Science - Machine Learning, Statistics - Machine Learning},
+ file = {arXiv Fulltext PDF:/Users/jeffreyma/Zotero/storage/MA4QGZ6E/Blalock et al. - 2020 - What is the State of Neural Network Pruning.pdf:application/pdf;arXiv.org Snapshot:/Users/jeffreyma/Zotero/storage/8DFKG4GL/2003.html:text/html}
}
-@inproceedings{chu2021discovering,
- title={Discovering multi-hardware mobile models via architecture search},
- author={Chu, Grace and Arikan, Okan and Bender, Gabriel and Wang, Weijun and Brighton, Achille and Kindermans, Pieter-Jan and Liu, Hanxiao and Akin, Berkin and Gupta, Suyog and Howard, Andrew},
- booktitle={Proceedings of the IEEE/CVF Conference on Computer Vision and Pattern Recognition},
- pages={3022--3031},
- year={2021}
+@article{brown2020language,
+ title = {Language models are few-shot learners},
+ author = {Brown, Tom and Mann, Benjamin and Ryder, Nick and Subbiah, Melanie and Kaplan, Jared D and Dhariwal, Prafulla and Neelakantan, Arvind and Shyam, Pranav and Sastry, Girish and Askell, Amanda and others},
+ year = 2020,
+ journal = {Advances in neural information processing systems},
+ volume = 33,
+ pages = {1877--1901}
+}
+
+@inproceedings{cai_online_2021,
+ title = {Online {Continual} {Learning} with {Natural} {Distribution} {Shifts}: {An} {Empirical} {Study} with {Visual} {Data}},
+ shorttitle = {Online {Continual} {Learning} with {Natural} {Distribution} {Shifts}},
+ author = {Cai, Zhipeng and Sener, Ozan and Koltun, Vladlen},
+ year = 2021,
+ month = oct,
+ booktitle = {2021 {IEEE}/{CVF} {International} {Conference} on {Computer} {Vision} ({ICCV})},
+ publisher = {IEEE},
+ address = {Montreal, QC, Canada},
+ pages = {8261--8270},
+ doi = {10.1109/ICCV48922.2021.00817},
+ isbn = {978-1-66542-812-5},
+ url = {https://ieeexplore.ieee.org/document/9710740/},
+ urldate = {2023-10-26},
+ language = {en},
+ file = {Cai et al. - 2021 - Online Continual Learning with Natural Distributio.pdf:/Users/alex/Zotero/storage/R7ZMIM4K/Cai et al. - 2021 - Online Continual Learning with Natural Distributio.pdf:application/pdf}
+}
+
+@article{cai_tinytl_nodate,
+ title = {{TinyTL}: {Reduce} {Memory}, {Not} {Parameters} for {Efficient} {On}-{Device} {Learning}},
+ author = {Cai, Han and Gan, Chuang and Zhu, Ligeng and Han, Song},
+ language = {en},
+ file = {Cai et al. - TinyTL Reduce Memory, Not Parameters for Efficient.pdf:/Users/alex/Zotero/storage/J9C8PTCX/Cai et al. - TinyTL Reduce Memory, Not Parameters for Efficient.pdf:application/pdf}
}
-@article{david2021tensorflow,
- title={Tensorflow lite micro: Embedded machine learning for tinyml systems},
- author={David, Robert and Duke, Jared and Jain, Advait and Janapa Reddi, Vijay and Jeffries, Nat and Li, Jian and Kreeger, Nick and Nappier, Ian and Natraj, Meghna and Wang, Tiezhen and others},
- journal={Proceedings of Machine Learning and Systems},
- volume={3},
- pages={800--811},
- year={2021}
+@article{cai2018proxylessnas,
+ title = {Proxylessnas: Direct neural architecture search on target task and hardware},
+ author = {Cai, Han and Zhu, Ligeng and Han, Song},
+ year = 2018,
+ journal = {arXiv preprint arXiv:1812.00332}
}
-@article{warden2018speech,
- title={Speech commands: A dataset for limited-vocabulary speech recognition},
- author={Warden, Pete},
- journal={arXiv preprint arXiv:1804.03209},
- year={2018}
+@article{cai2020tinytl,
+ title = {Tinytl: Reduce memory, not parameters for efficient on-device learning},
+ author = {Cai, Han and Gan, Chuang and Zhu, Ligeng and Han, Song},
+ year = 2020,
+ journal = {Advances in Neural Information Processing Systems},
+ volume = 33,
+ pages = {11285--11297}
}
-@inproceedings{adolf2016fathom,
- title={Fathom: Reference workloads for modern deep learning methods},
- author={Adolf, Robert and Rama, Saketh and Reagen, Brandon and Wei, Gu-Yeon and Brooks, David},
- booktitle={2016 IEEE International Symposium on Workload Characterization (IISWC)},
- pages={1--10},
- year={2016},
- organization={IEEE}
+@article{Chapelle_Scholkopf_Zien,
+ title = {Semi-supervised learning (Chapelle, O. et al., eds.; 2006) [book reviews]},
+ author = {Chapelle, O. and Scholkopf, B. and Zien, Eds., A.},
+ year = 2009,
+ journal = {IEEE Transactions on Neural Networks},
+ volume = 20,
+ number = 3,
+ pages = {542–542},
+ doi = {10.1109/tnn.2009.2015974}
}
-@article{coleman2017dawnbench,
- title={Dawnbench: An end-to-end deep learning benchmark and competition},
- author={Coleman, Cody and Narayanan, Deepak and Kang, Daniel and Zhao, Tian and Zhang, Jian and Nardi, Luigi and Bailis, Peter and Olukotun, Kunle and R{\'e}, Chris and Zaharia, Matei},
- journal={Training},
- volume={100},
- number={101},
- pages={102},
- year={2017}
+@misc{chen__inpainting_2022,
+ title = {Inpainting {Fluid} {Dynamics} with {Tensor} {Decomposition} ({NumPy})},
+ author = {Chen (陈新宇), Xinyu},
+ year = 2022,
+ month = mar,
+ journal = {Medium},
+ url = {https://medium.com/@xinyu.chen/inpainting-fluid-dynamics-with-tensor-decomposition-numpy-d84065fead4d},
+ urldate = {2023-10-20},
+ abstract = {Some simple examples for showing how to use tensor decomposition to reconstruct fluid dynamics},
+ language = {en}
+}
+
+@misc{chen_tvm_2018,
+ title = {{TVM}: {An} {Automated} {End}-to-{End} {Optimizing} {Compiler} for {Deep} {Learning}},
+ shorttitle = {{TVM}},
+ author = {Chen, Tianqi and Moreau, Thierry and Jiang, Ziheng and Zheng, Lianmin and Yan, Eddie and Cowan, Meghan and Shen, Haichen and Wang, Leyuan and Hu, Yuwei and Ceze, Luis and Guestrin, Carlos and Krishnamurthy, Arvind},
+ year = 2018,
+ month = oct,
+ publisher = {arXiv},
+ url = {http://arxiv.org/abs/1802.04799},
+ urldate = {2023-10-26},
+ note = {arXiv:1802.04799 [cs]},
+ language = {en},
+ keywords = {Computer Science - Artificial Intelligence, Computer Science - Machine Learning, Computer Science - Programming Languages},
+ annote = {Comment: Significantly improved version, add automated optimization},
+ file = {Chen et al. - 2018 - TVM An Automated End-to-End Optimizing Compiler f.pdf:/Users/alex/Zotero/storage/QR8MHJ38/Chen et al. - 2018 - TVM An Automated End-to-End Optimizing Compiler f.pdf:application/pdf}
+}
+
+@article{chen2016training,
+ title = {Training deep nets with sublinear memory cost},
+ author = {Chen, Tianqi and Xu, Bing and Zhang, Chiyuan and Guestrin, Carlos},
+ year = 2016,
+ journal = {arXiv preprint arXiv:1604.06174}
}
-@article{mattson2020mlperf,
- title={Mlperf training benchmark},
- author={Mattson, Peter and Cheng, Christine and Diamos, Gregory and Coleman, Cody and Micikevicius, Paulius and Patterson, David and Tang, Hanlin and Wei, Gu-Yeon and Bailis, Peter and Bittorf, Victor and others},
- journal={Proceedings of Machine Learning and Systems},
- volume={2},
- pages={336--349},
- year={2020}
+@inproceedings{chen2018tvm,
+ title = {$\{$TVM$\}$: An automated $\{$End-to-End$\}$ optimizing compiler for deep learning},
+ author = {Chen, Tianqi and Moreau, Thierry and Jiang, Ziheng and Zheng, Lianmin and Yan, Eddie and Shen, Haichen and Cowan, Meghan and Wang, Leyuan and Hu, Yuwei and Ceze, Luis and others},
+ year = 2018,
+ booktitle = {13th USENIX Symposium on Operating Systems Design and Implementation (OSDI 18)},
+ pages = {578--594}
}
-@article{brown2020language,
- title={Language models are few-shot learners},
- author={Brown, Tom and Mann, Benjamin and Ryder, Nick and Subbiah, Melanie and Kaplan, Jared D and Dhariwal, Prafulla and Neelakantan, Arvind and Shyam, Pranav and Sastry, Girish and Askell, Amanda and others},
- journal={Advances in neural information processing systems},
- volume={33},
- pages={1877--1901},
- year={2020}
+@article{chen2023learning,
+ title = {Learning domain-heterogeneous speaker recognition systems with personalized continual federated learning},
+ author = {Chen, Zhiyong and Xu, Shugong},
+ year = 2023,
+ journal = {EURASIP Journal on Audio, Speech, and Music Processing},
+ publisher = {Springer},
+ volume = 2023,
+ number = 1,
+ pages = 33
}
-@article{devlin2018bert,
- title={Bert: Pre-training of deep bidirectional transformers for language understanding},
- author={Devlin, Jacob and Chang, Ming-Wei and Lee, Kenton and Toutanova, Kristina},
- journal={arXiv preprint arXiv:1810.04805},
- year={2018}
+@misc{chollet2015,
+ title = {keras},
+ author = {François Chollet},
+ year = 2015,
+ journal = {GitHub repository},
+ publisher = {GitHub},
+ howpublished = {\url{https://github.com/fchollet/keras}},
+ commit = {5bcac37}
}
-@inproceedings{ignatov2018ai,
- title={Ai benchmark: Running deep neural networks on android smartphones},
- author={Ignatov, Andrey and Timofte, Radu and Chou, William and Wang, Ke and Wu, Max and Hartley, Tim and Van Gool, Luc},
- booktitle={Proceedings of the European Conference on Computer Vision (ECCV) Workshops},
- pages={0--0},
- year={2018}
+@article{chollet2018keras,
+ title = {Introduction to keras},
+ author = {Chollet, Fran{\c{c}}ois},
+ year = 2018,
+ journal = {March 9th}
}
-@inproceedings{reddi2020mlperf,
- title={Mlperf inference benchmark},
- author={Reddi, Vijay Janapa and Cheng, Christine and Kanter, David and Mattson, Peter and Schmuelling, Guenther and Wu, Carole-Jean and Anderson, Brian and Breughe, Maximilien and Charlebois, Mark and Chou, William and others},
- booktitle={2020 ACM/IEEE 47th Annual International Symposium on Computer Architecture (ISCA)},
- pages={446--459},
- year={2020},
- organization={IEEE}
+@inproceedings{chu2021discovering,
+ title = {Discovering multi-hardware mobile models via architecture search},
+ author = {Chu, Grace and Arikan, Okan and Bender, Gabriel and Wang, Weijun and Brighton, Achille and Kindermans, Pieter-Jan and Liu, Hanxiao and Akin, Berkin and Gupta, Suyog and Howard, Andrew},
+ year = 2021,
+ booktitle = {Proceedings of the IEEE/CVF Conference on Computer Vision and Pattern Recognition},
+ pages = {3022--3031},
+ eprint = {2008.08178},
+ archiveprefix = {arXiv},
+ primaryclass = {cs.CV}
}
-@misc{Thefutur92:online,
-author = {ARM.com},
-title = {The future is being built on Arm: Market diversification continues to drive strong royalty and licensing growth as ecosystem reaches quarter of a trillion chips milestone – Arm®},
-howpublished = {\url{https://www.arm.com/company/news/2023/02/arm-announces-q3-fy22-results}},
-month = {},
-year = {},
-note = {(Accessed on 09/16/2023)}
+@article{coleman2017dawnbench,
+ title = {Dawnbench: An end-to-end deep learning benchmark and competition},
+ author = {Coleman, Cody and Narayanan, Deepak and Kang, Daniel and Zhao, Tian and Zhang, Jian and Nardi, Luigi and Bailis, Peter and Olukotun, Kunle and R{\'e}, Chris and Zaharia, Matei},
+ year = 2017,
+ journal = {Training},
+ volume = 100,
+ number = 101,
+ pages = 102
}
-@inproceedings{deng2009imagenet,
- title={Imagenet: A large-scale hierarchical image database},
- author={Deng, Jia and Dong, Wei and Socher, Richard and Li, Li-Jia and Li, Kai and Fei-Fei, Li},
- booktitle={2009 IEEE conference on computer vision and pattern recognition},
- pages={248--255},
- year={2009},
- organization={Ieee}
+@inproceedings{coleman2022similarity,
+ title = {Similarity search for efficient active learning and search of rare concepts},
+ author = {Coleman, Cody and Chou, Edward and Katz-Samuels, Julian and Culatana, Sean and Bailis, Peter and Berg, Alexander C and Nowak, Robert and Sumbaly, Roshan and Zaharia, Matei and Yalniz, I Zeki},
+ year = 2022,
+ booktitle = {Proceedings of the AAAI Conference on Artificial Intelligence},
+ volume = 36,
+ number = 6,
+ pages = {6402--6410}
+}
+
+@misc{cottier_trends_2023,
+ title = {Trends in the {Dollar} {Training} {Cost} of {Machine} {Learning} {Systems}},
+ author = {Cottier, Ben},
+ year = 2023,
+ month = jan,
+ journal = {Epoch AI Report},
+ url = {https://epochai.org/blog/trends-in-the-dollar-training-cost-of-machine-learning-systems}
+}
+
+@misc{david_tensorflow_2021,
+ title = {{TensorFlow} {Lite} {Micro}: {Embedded} {Machine} {Learning} on {TinyML} {Systems}},
+ shorttitle = {{TensorFlow} {Lite} {Micro}},
+ author = {David, Robert and Duke, Jared and Jain, Advait and Reddi, Vijay Janapa and Jeffries, Nat and Li, Jian and Kreeger, Nick and Nappier, Ian and Natraj, Meghna and Regev, Shlomi and Rhodes, Rocky and Wang, Tiezhen and Warden, Pete},
+ year = 2021,
+ month = mar,
+ publisher = {arXiv},
+ url = {http://arxiv.org/abs/2010.08678},
+ urldate = {2023-10-26},
+ note = {arXiv:2010.08678 [cs]},
+ language = {en},
+ keywords = {Computer Science - Artificial Intelligence, Computer Science - Machine Learning},
+ file = {David et al. - 2021 - TensorFlow Lite Micro Embedded Machine Learning o.pdf:/Users/alex/Zotero/storage/YCFVNEVH/David et al. - 2021 - TensorFlow Lite Micro Embedded Machine Learning o.pdf:application/pdf}
}
@article{david2021tensorflow,
- title={Tensorflow lite micro: Embedded machine learning for tinyml systems},
- author={David, Robert and Duke, Jared and Jain, Advait and Janapa Reddi, Vijay and Jeffries, Nat and Li, Jian and Kreeger, Nick and Nappier, Ian and Natraj, Meghna and Wang, Tiezhen and others},
- journal={Proceedings of Machine Learning and Systems},
- volume={3},
- pages={800--811},
- year={2021}
+ title = {Tensorflow lite micro: Embedded machine learning for tinyml systems},
+ author = {David, Robert and Duke, Jared and Jain, Advait and Janapa Reddi, Vijay and Jeffries, Nat and Li, Jian and Kreeger, Nick and Nappier, Ian and Natraj, Meghna and Wang, Tiezhen and others},
+ year = 2021,
+ journal = {Proceedings of Machine Learning and Systems},
+ volume = 3,
+ pages = {800--811}
}
-
-@article{al2016theano,
- title={Theano: A Python framework for fast computation of mathematical expressions},
- author={Al-Rfou, Rami and Alain, Guillaume and Almahairi, Amjad and Angermueller, Christof and Bahdanau, Dzmitry and Ballas, Nicolas and Bastien, Fr{\'e}d{\'e}ric and Bayer, Justin and Belikov, Anatoly and Belopolsky, Alexander and others},
- journal={arXiv e-prints},
- pages={arXiv--1605},
- year={2016}
+@article{dean2012large,
+ title = {Large scale distributed deep networks},
+ author = {Dean, Jeffrey and Corrado, Greg and Monga, Rajat and Chen, Kai and Devin, Matthieu and Mao, Mark and Ranzato, Marc'aurelio and Senior, Andrew and Tucker, Paul and Yang, Ke and others},
+ year = 2012,
+ journal = {Advances in neural information processing systems},
+ volume = 25
}
+@misc{deci,
+ title = {The Ultimate Guide to Deep Learning Model Quantization and Quantization-Aware Training},
+ url = {https://deci.ai/quantization-and-quantization-aware-training/}
+}
-
-@inproceedings{jia2014caffe,
- title={Caffe: Convolutional architecture for fast feature embedding},
- author={Jia, Yangqing and Shelhamer, Evan and Donahue, Jeff and Karayev, Sergey and Long, Jonathan and Girshick, Ross and Guadarrama, Sergio and Darrell, Trevor},
- booktitle={Proceedings of the 22nd ACM international conference on Multimedia},
- pages={675--678},
- year={2014}
+@misc{deepcompress,
+ title = {Deep Compression: Compressing Deep Neural Networks with Pruning, Trained Quantization and Huffman Coding},
+ author = {Han and Mao and Dally},
+ year = 2016,
+ doi = {10.48550/arXiv.1510.00149},
+ url = {https://arxiv.org/abs/1510.00149},
+ urldate = {2016-02-15},
+ abstract = {Neural networks are both computationally intensive and memory intensive, making them difficult to deploy on embedded systems with limited hardware resources. To address this limitation, we introduce "deep compression", a three stage pipeline: pruning, trained quantization and Huffman coding, that work together to reduce the storage requirement of neural networks by 35x to 49x without affecting their accuracy. Our method first prunes the network by learning only the important connections. Next, we quantize the weights to enforce weight sharing, finally, we apply Huffman coding. After the first two steps we retrain the network to fine tune the remaining connections and the quantized centroids. Pruning, reduces the number of connections by 9x to 13x; Quantization then reduces the number of bits that represent each connection from 32 to 5. On the ImageNet dataset, our method reduced the storage required by AlexNet by 35x, from 240MB to 6.9MB, without loss of accuracy. Our method reduced the size of VGG-16 by 49x from 552MB to 11.3MB, again with no loss of accuracy. This allows fitting the model into on-chip SRAM cache rather than off-chip DRAM memory. Our compression method also facilitates the use of complex neural networks in mobile applications where application size and download bandwidth are constrained. Benchmarked on CPU, GPU and mobile GPU, compressed network has 3x to 4x layerwise speedup and 3x to 7x better energy efficiency.}
}
-@article{brown2020language,
- title={Language models are few-shot learners},
- author={Brown, Tom and Mann, Benjamin and Ryder, Nick and Subbiah, Melanie and Kaplan, Jared D and Dhariwal, Prafulla and Neelakantan, Arvind and Shyam, Pranav and Sastry, Girish and Askell, Amanda and others},
- journal={Advances in neural information processing systems},
- volume={33},
- pages={1877--1901},
- year={2020}
+@inproceedings{deng2009imagenet,
+ title = {ImageNet: A large-scale hierarchical image database},
+ author = {Deng, Jia and Socher, R. and Fei-Fei, Li and Dong, Wei and Li, Kai and Li, Li-Jia},
+ year = 2009,
+ month = {06},
+ booktitle = {2009 IEEE Conference on Computer Vision and Pattern Recognition(CVPR)},
+ volume = 00,
+ pages = {248--255},
+ doi = {10.1109/CVPR.2009.5206848},
+ url = {https://ieeexplore.ieee.org/abstract/document/5206848/},
+ added-at = {2018-09-20T15:22:39.000+0200},
+ biburl = {https://www.bibsonomy.org/bibtex/252793859f5bcbbd3f7f9e5d083160acf/analyst},
+ description = {ImageNet: A large-scale hierarchical image database},
+ interhash = {fbfae3e4fe1a81c477ba00efd0d4d977},
+ intrahash = {52793859f5bcbbd3f7f9e5d083160acf},
+ keywords = {2009 computer-vision cvpr dataset ieee paper},
+ timestamp = {2018-09-20T15:22:39.000+0200}
+}
+
+@article{desai2016five,
+ title = {Five Safes: designing data access for research},
+ author = {Desai, Tanvi and Ritchie, Felix and Welpton, Richard and others},
+ year = 2016,
+ journal = {Economics Working Paper Series},
+ volume = 1601,
+ pages = 28
+}
+
+@article{desai2020five,
+ title = {Five Safes: designing data access for research; 2016},
+ author = {Desai, Tanvi and Ritchie, Felix and Welpton, Richard},
+ year = 2020,
+ journal = {URL https://www2. uwe. ac. uk/faculties/bbs/Documents/1601. pdf}
}
-@inproceedings{he2016deep,
- title={Deep residual learning for image recognition},
- author={He, Kaiming and Zhang, Xiangyu and Ren, Shaoqing and Sun, Jian},
- booktitle={Proceedings of the IEEE conference on computer vision and pattern recognition},
- pages={770--778},
- year={2016}
+@article{devlin2018bert,
+ title = {Bert: Pre-training of deep bidirectional transformers for language understanding},
+ author = {Devlin, Jacob and Chang, Ming-Wei and Lee, Kenton and Toutanova, Kristina},
+ year = 2018,
+ journal = {arXiv preprint arXiv:1810.04805}
}
+@article{dhar2021survey,
+ title = {A survey of on-device machine learning: An algorithms and learning theory perspective},
+ author = {Dhar, Sauptik and Guo, Junyao and Liu, Jiayi and Tripathi, Samarth and Kurup, Unmesh and Shah, Mohak},
+ year = 2021,
+ journal = {ACM Transactions on Internet of Things},
+ publisher = {ACM New York, NY, USA},
+ volume = 2,
+ number = 3,
+ pages = {1--49}
+}
-@article{krizhevsky2012imagenet,
- title={Imagenet classification with deep convolutional neural networks},
- author={Krizhevsky, Alex and Sutskever, Ilya and Hinton, Geoffrey E},
- journal={Advances in neural information processing systems},
- volume={25},
- year={2012}
+@misc{dong2022splitnets,
+ title = {SplitNets: Designing Neural Architectures for Efficient Distributed Computing on Head-Mounted Systems},
+ author = {Xin Dong and Barbara De Salvo and Meng Li and Chiao Liu and Zhongnan Qu and H. T. Kung and Ziyun Li},
+ year = 2022,
+ eprint = {2204.04705},
+ archiveprefix = {arXiv},
+ primaryclass = {cs.LG}
}
-@article{paszke2019pytorch,
- title={Pytorch: An imperative style, high-performance deep learning library},
- author={Paszke, Adam and Gross, Sam and Massa, Francisco and Lerer, Adam and Bradbury, James and Chanan, Gregory and Killeen, Trevor and Lin, Zeming and Gimelshein, Natalia and Antiga, Luca and others},
- journal={Advances in neural information processing systems},
- volume={32},
- year={2019}
+@article{duisterhof2019learning,
+ title = {Learning to seek: Autonomous source seeking with deep reinforcement learning onboard a nano drone microcontroller},
+ author = {Duisterhof, Bardienus P and Krishnan, Srivatsan and Cruz, Jonathan J and Banbury, Colby R and Fu, William and Faust, Aleksandra and de Croon, Guido CHE and Reddi, Vijay Janapa},
+ year = 2019,
+ journal = {arXiv preprint arXiv:1909.11236}
}
-@inproceedings{seide2016cntk,
- title={CNTK: Microsoft's open-source deep-learning toolkit},
- author={Seide, Frank and Agarwal, Amit},
- booktitle={Proceedings of the 22nd ACM SIGKDD international conference on knowledge discovery and data mining},
- pages={2135--2135},
- year={2016}
+@inproceedings{duisterhof2021sniffy,
+ title = {Sniffy bug: A fully autonomous swarm of gas-seeking nano quadcopters in cluttered environments},
+ author = {Duisterhof, Bardienus P and Li, Shushuai and Burgu{\'e}s, Javier and Reddi, Vijay Janapa and de Croon, Guido CHE},
+ year = 2021,
+ booktitle = {2021 IEEE/RSJ International Conference on Intelligent Robots and Systems (IROS)},
+ pages = {9099--9106},
+ organization = {IEEE}
+}
+
+@article{dwork2014algorithmic,
+ title = {The algorithmic foundations of differential privacy},
+ author = {Dwork, Cynthia and Roth, Aaron and others},
+ year = 2014,
+ journal = {Foundations and Trends{\textregistered} in Theoretical Computer Science},
+ publisher = {Now Publishers, Inc.},
+ volume = 9,
+ number = {3--4},
+ pages = {211--407}
+}
+
+@article{electronics12102287,
+ title = {Reviewing Federated Learning Aggregation Algorithms; Strategies, Contributions, Limitations and Future Perspectives},
+ author = {Moshawrab, Mohammad and Adda, Mehdi and Bouzouane, Abdenour and Ibrahim, Hussein and Raad, Ali},
+ year = 2023,
+ journal = {Electronics},
+ volume = 12,
+ number = 10,
+ doi = {10.3390/electronics12102287},
+ issn = {2079-9292},
+ url = {https://www.mdpi.com/2079-9292/12/10/2287},
+ article-number = 2287
}
-@inproceedings{kung1979systolic,
- title={Systolic arrays (for VLSI)},
- author={Kung, Hsiang Tsung and Leiserson, Charles E},
- booktitle={Sparse Matrix Proceedings 1978},
- volume={1},
- pages={256--282},
- year={1979},
- organization={Society for industrial and applied mathematics Philadelphia, PA, USA}
+@misc{energyproblem,
+ title = {Computing's energy problem (and what we can do about it)},
+ author = {ISSCC},
+ year = 2014,
+ url = {https://ieeexplore.ieee.org/document/6757323},
+ urldate = {2014-03-06}
}
+@article{esteva2017dermatologist,
+ title = {Dermatologist-level classification of skin cancer with deep neural networks},
+ author = {Esteva, Andre and Kuprel, Brett and Novoa, Roberto A and Ko, Justin and Swetter, Susan M and Blau, Helen M and Thrun, Sebastian},
+ year = 2017,
+ journal = {nature},
+ publisher = {Nature Publishing Group},
+ volume = 542,
+ number = 7639,
+ pages = {115--118}
+}
-@article{li2014communication,
- title={Communication efficient distributed machine learning with the parameter server},
- author={Li, Mu and Andersen, David G and Smola, Alexander J and Yu, Kai},
- journal={Advances in Neural Information Processing Systems},
- volume={27},
- year={2014}
+@misc{fahim2021hls4ml,
+ title = {hls4ml: An Open-Source Codesign Workflow to Empower Scientific Low-Power Machine Learning Devices},
+ author = {Farah Fahim and Benjamin Hawks and Christian Herwig and James Hirschauer and Sergo Jindariani and Nhan Tran and Luca P. Carloni and Giuseppe Di Guglielmo and Philip Harris and Jeffrey Krupa and Dylan Rankin and Manuel Blanco Valentin and Josiah Hester and Yingyi Luo and John Mamish and Seda Orgrenci-Memik and Thea Aarrestad and Hamza Javed and Vladimir Loncar and Maurizio Pierini and Adrian Alan Pol and Sioni Summers and Javier Duarte and Scott Hauck and Shih-Chieh Hsu and Jennifer Ngadiuba and Mia Liu and Duc Hoang and Edward Kreinar and Zhenbin Wu},
+ year = 2021,
+ eprint = {2103.05579},
+ archiveprefix = {arXiv},
+ primaryclass = {cs.LG}
}
-@inproceedings{abadi2016tensorflow,
- title={$\{$TensorFlow$\}$: a system for $\{$Large-Scale$\}$ machine learning},
- author={Abadi, Mart{\'\i}n and Barham, Paul and Chen, Jianmin and Chen, Zhifeng and Davis, Andy and Dean, Jeffrey and Devin, Matthieu and Ghemawat, Sanjay and Irving, Geoffrey and Isard, Michael and others},
- booktitle={12th USENIX symposium on operating systems design and implementation (OSDI 16)},
- pages={265--283},
- year={2016}
+@misc{frankle_lottery_2019,
+ title = {The {Lottery} {Ticket} {Hypothesis}: {Finding} {Sparse}, {Trainable} {Neural} {Networks}},
+ shorttitle = {The {Lottery} {Ticket} {Hypothesis}},
+ author = {Frankle, Jonathan and Carbin, Michael},
+ year = 2019,
+ month = mar,
+ publisher = {arXiv},
+ doi = {10.48550/arXiv.1803.03635},
+ url = {http://arxiv.org/abs/1803.03635},
+ urldate = {2023-10-20},
+ note = {arXiv:1803.03635 [cs]},
+ abstract = {Neural network pruning techniques can reduce the parameter counts of trained networks by over 90\%, decreasing storage requirements and improving computational performance of inference without compromising accuracy. However, contemporary experience is that the sparse architectures produced by pruning are difficult to train from the start, which would similarly improve training performance. We find that a standard pruning technique naturally uncovers subnetworks whose initializations made them capable of training effectively. Based on these results, we articulate the "lottery ticket hypothesis:" dense, randomly-initialized, feed-forward networks contain subnetworks ("winning tickets") that - when trained in isolation - reach test accuracy comparable to the original network in a similar number of iterations. The winning tickets we find have won the initialization lottery: their connections have initial weights that make training particularly effective. We present an algorithm to identify winning tickets and a series of experiments that support the lottery ticket hypothesis and the importance of these fortuitous initializations. We consistently find winning tickets that are less than 10-20\% of the size of several fully-connected and convolutional feed-forward architectures for MNIST and CIFAR10. Above this size, the winning tickets that we find learn faster than the original network and reach higher test accuracy.},
+ keywords = {Computer Science - Artificial Intelligence, Computer Science - Machine Learning, Computer Science - Neural and Evolutionary Computing},
+ file = {arXiv Fulltext PDF:/Users/jeffreyma/Zotero/storage/6STHYGW5/Frankle and Carbin - 2019 - The Lottery Ticket Hypothesis Finding Sparse, Tra.pdf:application/pdf;arXiv.org Snapshot:/Users/jeffreyma/Zotero/storage/QGNSCTQB/1803.html:text/html}
}
-@article{dean2012large,
- title={Large scale distributed deep networks},
- author={Dean, Jeffrey and Corrado, Greg and Monga, Rajat and Chen, Kai and Devin, Matthieu and Mao, Mark and Ranzato, Marc'aurelio and Senior, Andrew and Tucker, Paul and Yang, Ke and others},
- journal={Advances in neural information processing systems},
- volume={25},
- year={2012}
+@article{gaviria2022dollar,
+ title = {The Dollar Street Dataset: Images Representing the Geographic and Socioeconomic Diversity of the World},
+ author = {Gaviria Rojas, William and Diamos, Sudnya and Kini, Keertan and Kanter, David and Janapa Reddi, Vijay and Coleman, Cody},
+ year = 2022,
+ journal = {Advances in Neural Information Processing Systems},
+ volume = 35,
+ pages = {12979--12990}
}
-@inproceedings{tokui2015chainer,
- title={Chainer: a next-generation open source framework for deep learning},
- author={Tokui, Seiya and Oono, Kenta and Hido, Shohei and Clayton, Justin},
- booktitle={Proceedings of workshop on machine learning systems (LearningSys) in the twenty-ninth annual conference on neural information processing systems (NIPS)},
- volume={5},
- pages={1--6},
- year={2015}
+@article{Gebru_Morgenstern_Vecchione_Vaughan_Wallach_III_Crawford_2021,
+ title = {Datasheets for datasets},
+ author = {Gebru, Timnit and Morgenstern, Jamie and Vecchione, Briana and Vaughan, Jennifer Wortman and Wallach, Hanna and III, Hal Daumé and Crawford, Kate},
+ year = 2021,
+ journal = {Communications of the ACM},
+ volume = 64,
+ number = 12,
+ pages = {86–92},
+ doi = {10.1145/3458723}
}
-@article{chollet2018keras,
- title={Keras: The python deep learning library},
- author={Chollet, Fran{\c{c}}ois and others},
- journal={Astrophysics source code library},
- pages={ascl--1806},
- year={2018}
+@article{goodfellow2020generative,
+ title = {Generative adversarial networks},
+ author = {Goodfellow, Ian and Pouget-Abadie, Jean and Mirza, Mehdi and Xu, Bing and Warde-Farley, David and Ozair, Sherjil and Courville, Aaron and Bengio, Yoshua},
+ year = 2020,
+ journal = {Communications of the ACM},
+ publisher = {ACM New York, NY, USA},
+ volume = 63,
+ number = 11,
+ pages = {139--144}
}
-@article{lai2018cmsis,
- title={Cmsis-nn: Efficient neural network kernels for arm cortex-m cpus},
- author={Lai, Liangzhen and Suda, Naveen and Chandra, Vikas},
- journal={arXiv preprint arXiv:1801.06601},
- year={2018}
+@misc{Google,
+ title = {Information quality & content moderation},
+ author = {Google},
+ url = {https://blog.google/documents/83/}
}
-@article{lin2020mcunet,
- title={Mcunet: Tiny deep learning on iot devices},
- author={Lin, Ji and Chen, Wei-Ming and Lin, Yujun and Gan, Chuang and Han, Song and others},
- journal={Advances in Neural Information Processing Systems},
- volume={33},
- pages={11711--11722},
- year={2020}
+@misc{gordon_morphnet_2018,
+ title = {{MorphNet}: {Fast} \& {Simple} {Resource}-{Constrained} {Structure} {Learning} of {Deep} {Networks}},
+ shorttitle = {{MorphNet}},
+ author = {Gordon, Ariel and Eban, Elad and Nachum, Ofir and Chen, Bo and Wu, Hao and Yang, Tien-Ju and Choi, Edward},
+ year = 2018,
+ month = apr,
+ publisher = {arXiv},
+ doi = {10.48550/arXiv.1711.06798},
+ url = {http://arxiv.org/abs/1711.06798},
+ urldate = {2023-10-20},
+ note = {arXiv:1711.06798 [cs, stat]},
+ abstract = {We present MorphNet, an approach to automate the design of neural network structures. MorphNet iteratively shrinks and expands a network, shrinking via a resource-weighted sparsifying regularizer on activations and expanding via a uniform multiplicative factor on all layers. In contrast to previous approaches, our method is scalable to large networks, adaptable to specific resource constraints (e.g. the number of floating-point operations per inference), and capable of increasing the network's performance. When applied to standard network architectures on a wide variety of datasets, our approach discovers novel structures in each domain, obtaining higher performance while respecting the resource constraint.},
+ keywords = {Computer Science - Machine Learning, Statistics - Machine Learning},
+ file = {arXiv Fulltext PDF:/Users/jeffreyma/Zotero/storage/GV7N4CZC/Gordon et al. - 2018 - MorphNet Fast & Simple Resource-Constrained Struc.pdf:application/pdf;arXiv.org Snapshot:/Users/jeffreyma/Zotero/storage/K6FUV82F/1711.html:text/html}
}
-@article{ramcharan2017deep,
- title={Deep learning for image-based cassava disease detection},
- author={Ramcharan, Amanda and Baranowski, Kelsee and McCloskey, Peter and Ahmed, Babuali and Legg, James and Hughes, David P},
- journal={Frontiers in plant science},
- volume={8},
- pages={1852},
- year={2017},
- publisher={Frontiers Media SA}
+@inproceedings{gordon2018morphnet,
+ title = {Morphnet: Fast \& simple resource-constrained structure learning of deep networks},
+ author = {Gordon, Ariel and Eban, Elad and Nachum, Ofir and Chen, Bo and Wu, Hao and Yang, Tien-Ju and Choi, Edward},
+ year = 2018,
+ booktitle = {Proceedings of the IEEE conference on computer vision and pattern recognition},
+ pages = {1586--1595}
}
-@article{seyedzadeh2018machine,
- title={Machine learning for estimation of building energy consumption and performance: a review},
- author={Seyedzadeh, Saleh and Rahimian, Farzad Pour and Glesk, Ivan and Roper, Marc},
- journal={Visualization in Engineering},
- volume={6},
- pages={1--20},
- year={2018},
- publisher={Springer}
+@article{gruslys2016memory,
+ title = {Memory-efficient backpropagation through time},
+ author = {Gruslys, Audrunas and Munos, R{\'e}mi and Danihelka, Ivo and Lanctot, Marc and Graves, Alex},
+ year = 2016,
+ journal = {Advances in neural information processing systems},
+ volume = 29
}
+@article{han2015deep,
+ title = {Deep compression: Compressing deep neural networks with pruning, trained quantization and huffman coding},
+ author = {Han, Song and Mao, Huizi and Dally, William J},
+ year = 2015,
+ journal = {arXiv preprint arXiv:1510.00149}
+}
-@article{duisterhof2019learning,
- title={Learning to seek: Autonomous source seeking with deep reinforcement learning onboard a nano drone microcontroller},
- author={Duisterhof, Bardienus P and Krishnan, Srivatsan and Cruz, Jonathan J and Banbury, Colby R and Fu, William and Faust, Aleksandra and de Croon, Guido CHE and Reddi, Vijay Janapa},
- journal={arXiv preprint arXiv:1909.11236},
- year={2019}
+@misc{han2016deep,
+ title = {Deep Compression: Compressing Deep Neural Networks with Pruning, Trained Quantization and Huffman Coding},
+ author = {Song Han and Huizi Mao and William J. Dally},
+ year = 2016,
+ eprint = {1510.00149},
+ archiveprefix = {arXiv},
+ primaryclass = {cs.CV}
}
-@inproceedings{duisterhof2021sniffy,
- title={Sniffy bug: A fully autonomous swarm of gas-seeking nano quadcopters in cluttered environments},
- author={Duisterhof, Bardienus P and Li, Shushuai and Burgu{\'e}s, Javier and Reddi, Vijay Janapa and de Croon, Guido CHE},
- booktitle={2021 IEEE/RSJ International Conference on Intelligent Robots and Systems (IROS)},
- pages={9099--9106},
- year={2021},
- organization={IEEE}
+@misc{he_structured_2023,
+ title = {Structured {Pruning} for {Deep} {Convolutional} {Neural} {Networks}: {A} survey},
+ shorttitle = {Structured {Pruning} for {Deep} {Convolutional} {Neural} {Networks}},
+ author = {He, Yang and Xiao, Lingao},
+ year = 2023,
+ month = mar,
+ publisher = {arXiv},
+ doi = {10.48550/arXiv.2303.00566},
+ url = {http://arxiv.org/abs/2303.00566},
+ urldate = {2023-10-20},
+ note = {arXiv:2303.00566 [cs]},
+ abstract = {The remarkable performance of deep Convolutional neural networks (CNNs) is generally attributed to their deeper and wider architectures, which can come with significant computational costs. Pruning neural networks has thus gained interest since it effectively lowers storage and computational costs. In contrast to weight pruning, which results in unstructured models, structured pruning provides the benefit of realistic acceleration by producing models that are friendly to hardware implementation. The special requirements of structured pruning have led to the discovery of numerous new challenges and the development of innovative solutions. This article surveys the recent progress towards structured pruning of deep CNNs. We summarize and compare the state-of-the-art structured pruning techniques with respect to filter ranking methods, regularization methods, dynamic execution, neural architecture search, the lottery ticket hypothesis, and the applications of pruning. While discussing structured pruning algorithms, we briefly introduce the unstructured pruning counterpart to emphasize their differences. Furthermore, we provide insights into potential research opportunities in the field of structured pruning. A curated list of neural network pruning papers can be found at https://github.com/he-y/Awesome-Pruning},
+ keywords = {Computer Science - Computer Vision and Pattern Recognition},
+ file = {arXiv Fulltext PDF:/Users/jeffreyma/Zotero/storage/K5RGQQA9/He and Xiao - 2023 - Structured Pruning for Deep Convolutional Neural N.pdf:application/pdf;arXiv.org Snapshot:/Users/jeffreyma/Zotero/storage/U7PVPU4C/2303.html:text/html}
}
-@misc{Vectorbo78:online,
-author = {},
-title = {Vector-borne diseases},
-howpublished = {\url{https://www.who.int/news-room/fact-sheets/detail/vector-borne-diseases}},
-month = {},
-year = {},
-note = {(Accessed on 10/17/2023)}
+@inproceedings{he2016deep,
+ title = {Deep residual learning for image recognition},
+ author = {He, Kaiming and Zhang, Xiangyu and Ren, Shaoqing and Sun, Jian},
+ year = 2016,
+ booktitle = {Proceedings of the IEEE conference on computer vision and pattern recognition},
+ pages = {770--778}
}
-@article{tirtalistyani2022indonesia,
- title={Indonesia rice irrigation system: Time for innovation},
- author={Tirtalistyani, Rose and Murtiningrum, Murtiningrum and Kanwar, Rameshwar S},
- journal={Sustainability},
- volume={14},
- number={19},
- pages={12477},
- year={2022},
- publisher={MDPI}
+@inproceedings{hendrycks2021natural,
+ title = {Natural adversarial examples},
+ author = {Hendrycks, Dan and Zhao, Kevin and Basart, Steven and Steinhardt, Jacob and Song, Dawn},
+ year = 2021,
+ booktitle = {Proceedings of the IEEE/CVF Conference on Computer Vision and Pattern Recognition},
+ pages = {15262--15271}
}
-@article{han2015deep,
- title={Deep compression: Compressing deep neural networks with pruning, trained quantization and huffman coding},
- author={Han, Song and Mao, Huizi and Dally, William J},
- journal={arXiv preprint arXiv:1510.00149},
- year={2015}
+@misc{hinton_distilling_2015,
+ title = {Distilling the {Knowledge} in a {Neural} {Network}},
+ author = {Hinton, Geoffrey and Vinyals, Oriol and Dean, Jeff},
+ year = 2015,
+ month = mar,
+ publisher = {arXiv},
+ doi = {10.48550/arXiv.1503.02531},
+ url = {http://arxiv.org/abs/1503.02531},
+ urldate = {2023-10-20},
+ note = {arXiv:1503.02531 [cs, stat]},
+ abstract = {A very simple way to improve the performance of almost any machine learning algorithm is to train many different models on the same data and then to average their predictions. Unfortunately, making predictions using a whole ensemble of models is cumbersome and may be too computationally expensive to allow deployment to a large number of users, especially if the individual models are large neural nets. Caruana and his collaborators have shown that it is possible to compress the knowledge in an ensemble into a single model which is much easier to deploy and we develop this approach further using a different compression technique. We achieve some surprising results on MNIST and we show that we can significantly improve the acoustic model of a heavily used commercial system by distilling the knowledge in an ensemble of models into a single model. We also introduce a new type of ensemble composed of one or more full models and many specialist models which learn to distinguish fine-grained classes that the full models confuse. Unlike a mixture of experts, these specialist models can be trained rapidly and in parallel.},
+ keywords = {Computer Science - Machine Learning, Computer Science - Neural and Evolutionary Computing, Statistics - Machine Learning},
+ file = {arXiv Fulltext PDF:/Users/jeffreyma/Zotero/storage/VREDW45A/Hinton et al. - 2015 - Distilling the Knowledge in a Neural Network.pdf:application/pdf;arXiv.org Snapshot:/Users/jeffreyma/Zotero/storage/8MNJG4RP/1503.html:text/html}
}
-@misc{han2016deep,
- title={Deep Compression: Compressing Deep Neural Networks with Pruning, Trained Quantization and Huffman Coding},
- author={Song Han and Huizi Mao and William J. Dally},
- year={2016},
- eprint={1510.00149},
- archivePrefix={arXiv},
- primaryClass={cs.CV}
+@misc{hinton2015distilling,
+ title = {Distilling the Knowledge in a Neural Network},
+ author = {Geoffrey Hinton and Oriol Vinyals and Jeff Dean},
+ year = 2015,
+ eprint = {1503.02531},
+ archiveprefix = {arXiv},
+ primaryclass = {stat.ML}
}
-@article{lecun1989optimal,
- title={Optimal brain damage},
- author={LeCun, Yann and Denker, John and Solla, Sara},
- journal={Advances in neural information processing systems},
- volume={2},
- year={1989}
+@article{Holland_Hosny_Newman_Joseph_Chmielinski_2020,
+ title = {The Dataset Nutrition label},
+ author = {Holland, Sarah and Hosny, Ahmed and Newman, Sarah and Joseph, Joshua and Chmielinski, Kasia},
+ year = 2020,
+ journal = {Data Protection and Privacy},
+ doi = {10.5040/9781509932771.ch-001}
}
-@book{barroso2019datacenter,
- title={The datacenter as a computer: Designing warehouse-scale machines},
- author={Barroso, Luiz Andr{\'e} and H{\"o}lzle, Urs and Ranganathan, Parthasarathy},
- year={2019},
- publisher={Springer Nature}
+@inproceedings{hong2023publishing,
+ title = {Publishing Efficient On-device Models Increases Adversarial Vulnerability},
+ author = {Hong, Sanghyun and Carlini, Nicholas and Kurakin, Alexey},
+ year = 2023,
+ booktitle = {2023 IEEE Conference on Secure and Trustworthy Machine Learning (SaTML)},
+ pages = {271--290},
+ organization = {IEEE}
}
-@article{howard2017mobilenets,
- title={Mobilenets: Efficient convolutional neural networks for mobile vision applications},
- author={Howard, Andrew G and Zhu, Menglong and Chen, Bo and Kalenichenko, Dmitry and Wang, Weijun and Weyand, Tobias and Andreetto, Marco and Adam, Hartwig},
- journal={arXiv preprint arXiv:1704.04861},
- year={2017}
+@misc{howard_mobilenets_2017,
+ title = {{MobileNets}: {Efficient} {Convolutional} {Neural} {Networks} for {Mobile} {Vision} {Applications}},
+ shorttitle = {{MobileNets}},
+ author = {Howard, Andrew G. and Zhu, Menglong and Chen, Bo and Kalenichenko, Dmitry and Wang, Weijun and Weyand, Tobias and Andreetto, Marco and Adam, Hartwig},
+ year = 2017,
+ month = apr,
+ publisher = {arXiv},
+ doi = {10.48550/arXiv.1704.04861},
+ url = {http://arxiv.org/abs/1704.04861},
+ urldate = {2023-10-20},
+ note = {arXiv:1704.04861 [cs]},
+ abstract = {We present a class of efficient models called MobileNets for mobile and embedded vision applications. MobileNets are based on a streamlined architecture that uses depth-wise separable convolutions to build light weight deep neural networks. We introduce two simple global hyper-parameters that efficiently trade off between latency and accuracy. These hyper-parameters allow the model builder to choose the right sized model for their application based on the constraints of the problem. We present extensive experiments on resource and accuracy tradeoffs and show strong performance compared to other popular models on ImageNet classification. We then demonstrate the effectiveness of MobileNets across a wide range of applications and use cases including object detection, finegrain classification, face attributes and large scale geo-localization.},
+ keywords = {Computer Science - Computer Vision and Pattern Recognition},
+ file = {arXiv Fulltext PDF:/Users/jeffreyma/Zotero/storage/IJ9P9ID9/Howard et al. - 2017 - MobileNets Efficient Convolutional Neural Network.pdf:application/pdf;arXiv.org Snapshot:/Users/jeffreyma/Zotero/storage/D9TS95GJ/1704.html:text/html}
}
-@inproceedings{he2016deep,
- title={Deep residual learning for image recognition},
- author={He, Kaiming and Zhang, Xiangyu and Ren, Shaoqing and Sun, Jian},
- booktitle={Proceedings of the IEEE conference on computer vision and pattern recognition},
- pages={770--778},
- year={2016}
+@misc{howard2017mobilenets,
+ title = {MobileNets: Efficient Convolutional Neural Networks for Mobile Vision Applications},
+ author = {Andrew G. Howard and Menglong Zhu and Bo Chen and Dmitry Kalenichenko and Weijun Wang and Tobias Weyand and Marco Andreetto and Hartwig Adam},
+ year = 2017,
+ journal = {arXiv preprint arXiv:1704.04861},
+ eprint = {1704.04861},
+ archiveprefix = {arXiv},
+ primaryclass = {cs.CV}
}
-@inproceedings{jouppi2017datacenter,
- title={In-datacenter performance analysis of a tensor processing unit},
- author={Jouppi, Norman P and Young, Cliff and Patil, Nishant and Patterson, David and Agrawal, Gaurav and Bajwa, Raminder and Bates, Sarah and Bhatia, Suresh and Boden, Nan and Borchers, Al and others},
- booktitle={Proceedings of the 44th annual international symposium on computer architecture},
- pages={1--12},
- year={2017}
+@misc{iandola_squeezenet_2016,
+ title = {{SqueezeNet}: {AlexNet}-level accuracy with 50x fewer parameters and {\textless}0.{5MB} model size},
+ shorttitle = {{SqueezeNet}},
+ author = {Iandola, Forrest N. and Han, Song and Moskewicz, Matthew W. and Ashraf, Khalid and Dally, William J. and Keutzer, Kurt},
+ year = 2016,
+ month = nov,
+ publisher = {arXiv},
+ doi = {10.48550/arXiv.1602.07360},
+ url = {http://arxiv.org/abs/1602.07360},
+ urldate = {2023-10-20},
+ note = {arXiv:1602.07360 [cs]},
+ abstract = {Recent research on deep neural networks has focused primarily on improving accuracy. For a given accuracy level, it is typically possible to identify multiple DNN architectures that achieve that accuracy level. With equivalent accuracy, smaller DNN architectures offer at least three advantages: (1) Smaller DNNs require less communication across servers during distributed training. (2) Smaller DNNs require less bandwidth to export a new model from the cloud to an autonomous car. (3) Smaller DNNs are more feasible to deploy on FPGAs and other hardware with limited memory. To provide all of these advantages, we propose a small DNN architecture called SqueezeNet. SqueezeNet achieves AlexNet-level accuracy on ImageNet with 50x fewer parameters. Additionally, with model compression techniques we are able to compress SqueezeNet to less than 0.5MB (510x smaller than AlexNet). The SqueezeNet architecture is available for download here: https://github.com/DeepScale/SqueezeNet},
+ keywords = {Computer Science - Artificial Intelligence, Computer Science - Computer Vision and Pattern Recognition},
+ file = {arXiv Fulltext PDF:/Users/jeffreyma/Zotero/storage/X3ZX9UTZ/Iandola et al. - 2016 - SqueezeNet AlexNet-level accuracy with 50x fewer .pdf:application/pdf;arXiv.org Snapshot:/Users/jeffreyma/Zotero/storage/DHI96QVT/1602.html:text/html}
}
@article{iandola2016squeezenet,
- title={SqueezeNet: AlexNet-level accuracy with 50x fewer parameters and< 0.5 MB model size},
- author={Iandola, Forrest N and Han, Song and Moskewicz, Matthew W and Ashraf, Khalid and Dally, William J and Keutzer, Kurt},
- journal={arXiv preprint arXiv:1602.07360},
- year={2016}
+ title = {SqueezeNet: AlexNet-level accuracy with 50x fewer parameters and< 0.5 MB model size},
+ author = {Iandola, Forrest N and Han, Song and Moskewicz, Matthew W and Ashraf, Khalid and Dally, William J and Keutzer, Kurt},
+ year = 2016,
+ journal = {arXiv preprint arXiv:1602.07360}
}
-@article{li2019edge,
- title={Edge AI: On-demand accelerating deep neural network inference via edge computing},
- author={Li, En and Zeng, Liekang and Zhou, Zhi and Chen, Xu},
- journal={IEEE Transactions on Wireless Communications},
- volume={19},
- number={1},
- pages={447--457},
- year={2019},
- publisher={IEEE}
+@inproceedings{ignatov2018ai,
+ title = {Ai benchmark: Running deep neural networks on android smartphones},
+ author = {Ignatov, Andrey and Timofte, Radu and Chou, William and Wang, Ke and Wu, Max and Hartley, Tim and Van Gool, Luc},
+ year = 2018,
+ booktitle = {Proceedings of the European Conference on Computer Vision (ECCV) Workshops},
+ pages = {0--0}
}
-
-@book{rosenblatt1957perceptron,
- title={The perceptron, a perceiving and recognizing automaton Project Para},
- author={Rosenblatt, Frank},
- year={1957},
- publisher={Cornell Aeronautical Laboratory}
+@inproceedings{ijcai2021p592,
+ title = {Hardware-Aware Neural Architecture Search: Survey and Taxonomy},
+ author = {Benmeziane, Hadjer and El Maghraoui, Kaoutar and Ouarnoughi, Hamza and Niar, Smail and Wistuba, Martin and Wang, Naigang},
+ year = 2021,
+ month = 8,
+ booktitle = {Proceedings of the Thirtieth International Joint Conference on Artificial Intelligence, {IJCAI-21}},
+ publisher = {International Joint Conferences on Artificial Intelligence Organization},
+ pages = {4322--4329},
+ doi = {10.24963/ijcai.2021/592},
+ url = {https://doi.org/10.24963/ijcai.2021/592},
+ note = {Survey Track},
+ editor = {Zhi-Hua Zhou}
}
-@article{rumelhart1986learning,
- title={Learning representations by back-propagating errors},
- author={Rumelhart, David E and Hinton, Geoffrey E and Williams, Ronald J},
- journal={nature},
- volume={323},
- number={6088},
- pages={533--536},
- year={1986},
- publisher={Nature Publishing Group UK London}
+@misc{intquantfordeepinf,
+ title = {Integer Quantization for Deep Learning Inference: Principles and Empirical Evaluation)},
+ author = {Wu and Judd, Zhang and Isaev, Micikevicius},
+ year = 2020,
+ doi = {10.48550/arXiv.2004.09602},
+ url = {https://arxiv.org/abs/2004.09602},
+ urldate = {2020-04-20},
+ abstract = {Quantization techniques can reduce the size of Deep Neural Networks and improve inference latency and throughput by taking advantage of high throughput integer instructions. In this paper we review the mathematical aspects of quantization parameters and evaluate their choices on a wide range of neural network models for different application domains, including vision, speech, and language. We focus on quantization techniques that are amenable to acceleration by processors with high-throughput integer math pipelines. We also present a workflow for 8-bit quantization that is able to maintain accuracy within 1% of the floating-point baseline on all networks studied, including models that are more difficult to quantize, such as MobileNets and BERT-large.}
}
-@book{warden2019tinyml,
- title={Tinyml: Machine learning with tensorflow lite on arduino and ultra-low-power microcontrollers},
- author={Warden, Pete and Situnayake, Daniel},
- year={2019},
- publisher={O'Reilly Media}
+@inproceedings{jia2014caffe,
+ title = {Caffe: Convolutional architecture for fast feature embedding},
+ author = {Jia, Yangqing and Shelhamer, Evan and Donahue, Jeff and Karayev, Sergey and Long, Jonathan and Girshick, Ross and Guadarrama, Sergio and Darrell, Trevor},
+ year = 2014,
+ booktitle = {Proceedings of the 22nd ACM international conference on Multimedia},
+ pages = {675--678}
}
-@inproceedings{jouppi2017datacenter,
- title={In-datacenter performance analysis of a tensor processing unit},
- author={Jouppi, Norman P and Young, Cliff and Patil, Nishant and Patterson, David and Agrawal, Gaurav and Bajwa, Raminder and Bates, Sarah and Bhatia, Suresh and Boden, Nan and Borchers, Al and others},
- booktitle={Proceedings of the 44th annual international symposium on computer architecture},
- pages={1--12},
- year={2017}
+@article{jia2023life,
+ title = {Life-threatening ventricular arrhythmia detection challenge in implantable cardioverter--defibrillators},
+ author = {Jia, Zhenge and Li, Dawei and Xu, Xiaowei and Li, Na and Hong, Feng and Ping, Lichuan and Shi, Yiyu},
+ year = 2023,
+ journal = {Nature Machine Intelligence},
+ publisher = {Nature Publishing Group UK London},
+ volume = 5,
+ number = 5,
+ pages = {554--555}
}
-@misc{mcmahan2023communicationefficient,
- title={Communication-Efficient Learning of Deep Networks from Decentralized Data},
- author={H. Brendan McMahan and Eider Moore and Daniel Ramage and Seth Hampson and Blaise Agüera y Arcas},
- year={2023},
- eprint={1602.05629},
- archivePrefix={arXiv},
- primaryClass={cs.LG}
+@misc{jiang2019accuracy,
+ title = {Accuracy vs. Efficiency: Achieving Both through FPGA-Implementation Aware Neural Architecture Search},
+ author = {Weiwen Jiang and Xinyi Zhang and Edwin H. -M. Sha and Lei Yang and Qingfeng Zhuge and Yiyu Shi and Jingtong Hu},
+ year = 2019,
+ eprint = {1901.11211},
+ archiveprefix = {arXiv},
+ primaryclass = {cs.DC}
+}
+
+@article{Johnson-Roberson_Barto_Mehta_Sridhar_Rosaen_Vasudevan_2017,
+ title = {Driving in the matrix: Can virtual worlds replace human-generated annotations for real world tasks?},
+ author = {Johnson-Roberson, Matthew and Barto, Charles and Mehta, Rounak and Sridhar, Sharath Nittur and Rosaen, Karl and Vasudevan, Ram},
+ year = 2017,
+ journal = {2017 IEEE International Conference on Robotics and Automation (ICRA)},
+ doi = {10.1109/icra.2017.7989092}
+}
+
+@article{jordan_machine_2015,
+ title = {Machine learning: {Trends}, perspectives, and prospects},
+ shorttitle = {Machine learning},
+ author = {Jordan, M. I. and Mitchell, T. M.},
+ year = 2015,
+ month = jul,
+ journal = {Science},
+ volume = 349,
+ number = 6245,
+ pages = {255--260},
+ doi = {10.1126/science.aaa8415},
+ issn = {0036-8075, 1095-9203},
+ url = {https://www.science.org/doi/10.1126/science.aaa8415},
+ urldate = {2023-10-25},
+ language = {en},
+ file = {Jordan and Mitchell - 2015 - Machine learning Trends, perspectives, and prospe.pdf:/Users/alex/Zotero/storage/RGU3CQ4Q/Jordan and Mitchell - 2015 - Machine learning Trends, perspectives, and prospe.pdf:application/pdf}
}
-@article{li2017learning,
- title={Learning without forgetting},
- author={Li, Zhizhong and Hoiem, Derek},
- journal={IEEE transactions on pattern analysis and machine intelligence},
- volume={40},
- number={12},
- pages={2935--2947},
- year={2017},
- publisher={IEEE}
+@inproceedings{jouppi2017datacenter,
+ title = {In-datacenter performance analysis of a tensor processing unit},
+ author = {Jouppi, Norman P and Young, Cliff and Patil, Nishant and Patterson, David and Agrawal, Gaurav and Bajwa, Raminder and Bates, Sarah and Bhatia, Suresh and Boden, Nan and Borchers, Al and others},
+ year = 2017,
+ booktitle = {Proceedings of the 44th annual international symposium on computer architecture},
+ pages = {1--12}
}
-@article{krizhevsky2012imagenet,
- title={Imagenet classification with deep convolutional neural networks},
- author={Krizhevsky, Alex and Sutskever, Ilya and Hinton, Geoffrey E},
- journal={Advances in neural information processing systems},
- volume={25},
- year={2012}
+@article{kairouz2015secure,
+ title = {Secure multi-party differential privacy},
+ author = {Kairouz, Peter and Oh, Sewoong and Viswanath, Pramod},
+ year = 2015,
+ journal = {Advances in neural information processing systems},
+ volume = 28
}
-@inproceedings{chen2018tvm,
- title={$\{$TVM$\}$: An automated $\{$End-to-End$\}$ optimizing compiler for deep learning},
- author={Chen, Tianqi and Moreau, Thierry and Jiang, Ziheng and Zheng, Lianmin and Yan, Eddie and Shen, Haichen and Cowan, Meghan and Wang, Leyuan and Hu, Yuwei and Ceze, Luis and others},
- booktitle={13th USENIX Symposium on Operating Systems Design and Implementation (OSDI 18)},
- pages={578--594},
- year={2018}
+@article{karargyris2023federated,
+ title = {Federated benchmarking of medical artificial intelligence with MedPerf},
+ author = {Karargyris, Alexandros and Umeton, Renato and Sheller, Micah J and Aristizabal, Alejandro and George, Johnu and Wuest, Anna and Pati, Sarthak and Kassem, Hasan and Zenk, Maximilian and Baid, Ujjwal and others},
+ year = 2023,
+ journal = {Nature Machine Intelligence},
+ publisher = {Nature Publishing Group UK London},
+ volume = 5,
+ number = 7,
+ pages = {799--810}
}
-@article{paszke2019pytorch,
- title={Pytorch: An imperative style, high-performance deep learning library},
- author={Paszke, Adam and Gross, Sam and Massa, Francisco and Lerer, Adam and Bradbury, James and Chanan, Gregory and Killeen, Trevor and Lin, Zeming and Gimelshein, Natalia and Antiga, Luca and others},
- journal={Advances in neural information processing systems},
- volume={32},
- year={2019}
+@article{kiela2021dynabench,
+ title = {Dynabench: Rethinking benchmarking in NLP},
+ author = {Kiela, Douwe and Bartolo, Max and Nie, Yixin and Kaushik, Divyansh and Geiger, Atticus and Wu, Zhengxuan and Vidgen, Bertie and Prasad, Grusha and Singh, Amanpreet and Ringshia, Pratik and others},
+ year = 2021,
+ journal = {arXiv preprint arXiv:2104.14337}
}
-@inproceedings{abadi2016tensorflow,
- title={$\{$TensorFlow$\}$: a system for $\{$Large-Scale$\}$ machine learning},
- author={Abadi, Mart{\'\i}n and Barham, Paul and Chen, Jianmin and Chen, Zhifeng and Davis, Andy and Dean, Jeffrey and Devin, Matthieu and Ghemawat, Sanjay and Irving, Geoffrey and Isard, Michael and others},
- booktitle={12th USENIX symposium on operating systems design and implementation (OSDI 16)},
- pages={265--283},
- year={2016}
+@inproceedings{koh2021wilds,
+ title = {Wilds: A benchmark of in-the-wild distribution shifts},
+ author = {Koh, Pang Wei and Sagawa, Shiori and Marklund, Henrik and Xie, Sang Michael and Zhang, Marvin and Balsubramani, Akshay and Hu, Weihua and Yasunaga, Michihiro and Phillips, Richard Lanas and Gao, Irena and others},
+ year = 2021,
+ booktitle = {International Conference on Machine Learning},
+ pages = {5637--5664},
+ organization = {PMLR}
}
-@misc{chollet2015,
-author = {François Chollet },
-title = {keras},
-year = {2015},
-publisher = {GitHub},
-journal = {GitHub repository},
-howpublished = {\url{https://github.com/fchollet/keras}},
-commit = {5bcac37}
+@article{kolda_tensor_2009,
+ title = {Tensor {Decompositions} and {Applications}},
+ author = {Kolda, Tamara G. and Bader, Brett W.},
+ year = 2009,
+ month = aug,
+ journal = {SIAM Review},
+ volume = 51,
+ number = 3,
+ pages = {455--500},
+ doi = {10.1137/07070111X},
+ issn = {0036-1445, 1095-7200},
+ url = {http://epubs.siam.org/doi/10.1137/07070111X},
+ urldate = {2023-10-20},
+ abstract = {This survey provides an overview of higher-order tensor decompositions, their applications, and available software. A tensor is a multidimensional or N -way array. Decompositions of higher-order tensors (i.e., N -way arrays with N ≥ 3) have applications in psychometrics, chemometrics, signal processing, numerical linear algebra, computer vision, numerical analysis, data mining, neuroscience, graph analysis, and elsewhere. Two particular tensor decompositions can be considered to be higher-order extensions of the matrix singular value decomposition: CANDECOMP/PARAFAC (CP) decomposes a tensor as a sum of rank-one tensors, and the Tucker decomposition is a higher-order form of principal component analysis. There are many other tensor decompositions, including INDSCAL, PARAFAC2, CANDELINC, DEDICOM, and PARATUCK2 as well as nonnegative variants of all of the above. The N-way Toolbox, Tensor Toolbox, and Multilinear Engine are examples of software packages for working with tensors.},
+ language = {en},
+ file = {Kolda and Bader - 2009 - Tensor Decompositions and Applications.pdf:/Users/jeffreyma/Zotero/storage/Q7ZG2267/Kolda and Bader - 2009 - Tensor Decompositions and Applications.pdf:application/pdf}
+}
+
+@article{koshti2011cumulative,
+ title = {Cumulative sum control chart},
+ author = {Koshti, VV},
+ year = 2011,
+ journal = {International journal of physics and mathematical sciences},
+ volume = 1,
+ number = 1,
+ pages = {28--32}
}
-@article{vaswani2017attention,
- title={Attention is all you need},
- author={Vaswani, Ashish and Shazeer, Noam and Parmar, Niki and Uszkoreit, Jakob and Jones, Llion and Gomez, Aidan N and Kaiser, {\L}ukasz and Polosukhin, Illia},
- journal={Advances in neural information processing systems},
- volume={30},
- year={2017}
+@misc{krishna2023raman,
+ title = {RAMAN: A Re-configurable and Sparse tinyML Accelerator for Inference on Edge},
+ author = {Adithya Krishna and Srikanth Rohit Nudurupati and Chandana D G and Pritesh Dwivedi and André van Schaik and Mahesh Mehendale and Chetan Singh Thakur},
+ year = 2023,
+ eprint = {2306.06493},
+ archiveprefix = {arXiv},
+ primaryclass = {cs.NE}
}
-@article{goodfellow2020generative,
- title={Generative adversarial networks},
- author={Goodfellow, Ian and Pouget-Abadie, Jean and Mirza, Mehdi and Xu, Bing and Warde-Farley, David and Ozair, Sherjil and Courville, Aaron and Bengio, Yoshua},
- journal={Communications of the ACM},
- volume={63},
- number={11},
- pages={139--144},
- year={2020},
- publisher={ACM New York, NY, USA}
+@article{krishnamoorthi2018quantizing,
+ title = {Quantizing deep convolutional networks for efficient inference: A whitepaper},
+ author = {Krishnamoorthi, Raghuraman},
+ year = 2018,
+ journal = {arXiv preprint arXiv:1806.08342}
}
-@article{bank2023autoencoders,
- title={Autoencoders},
- author={Bank, Dor and Koenigstein, Noam and Giryes, Raja},
- journal={Machine Learning for Data Science Handbook: Data Mining and Knowledge Discovery Handbook},
- pages={353--374},
- year={2023},
- publisher={Springer}
+@article{Krishnan_Rajpurkar_Topol_2022,
+ title = {Self-supervised learning in medicine and Healthcare},
+ author = {Krishnan, Rayan and Rajpurkar, Pranav and Topol, Eric J.},
+ year = 2022,
+ journal = {Nature Biomedical Engineering},
+ volume = 6,
+ number = 12,
+ pages = {1346–1352},
+ doi = {10.1038/s41551-022-00914-1}
}
+@article{krizhevsky2012imagenet,
+ title = {Imagenet classification with deep convolutional neural networks},
+ author = {Krizhevsky, Alex and Sutskever, Ilya and Hinton, Geoffrey E},
+ year = 2012,
+ journal = {Advances in neural information processing systems},
+ volume = 25
+}
+@inproceedings{kung1979systolic,
+ title = {Systolic arrays (for VLSI)},
+ author = {Kung, Hsiang Tsung and Leiserson, Charles E},
+ year = 1979,
+ booktitle = {Sparse Matrix Proceedings 1978},
+ volume = 1,
+ pages = {256--282},
+ organization = {Society for industrial and applied mathematics Philadelphia, PA, USA}
+}
-@article{Aledhari_Razzak_Parizi_Saeed_2020, title={Federated learning: A survey on enabling technologies, Protocols, and applications}, volume={8}, DOI={10.1109/access.2020.3013541}, journal={IEEE Access}, author={Aledhari, Mohammed and Razzak, Rehma and Parizi, Reza M. and Saeed, Fahad}, year={2020}, pages={140699–140725}}
-
-@article{Bender_Friedman_2018, title={Data statements for natural language processing: Toward mitigating system bias and enabling better science}, volume={6}, DOI={10.1162/tacl_a_00041}, journal={Transactions of the Association for Computational Linguistics}, author={Bender, Emily M. and Friedman, Batya}, year={2018}, pages={587–604}}
-
-@article{Chapelle_Scholkopf_Zien, title={Semi-supervised learning (Chapelle, O. et al., eds.; 2006) [book reviews]}, volume={20}, DOI={10.1109/tnn.2009.2015974}, number={3}, journal={IEEE Transactions on Neural Networks}, author={Chapelle, O. and Scholkopf, B. and Zien, Eds., A.}, year={2009}, pages={542–542}}
-
-@article{Gebru_Morgenstern_Vecchione_Vaughan_Wallach_III_Crawford_2021, title={Datasheets for datasets}, volume={64}, DOI={10.1145/3458723}, number={12}, journal={Communications of the ACM}, author={Gebru, Timnit and Morgenstern, Jamie and Vecchione, Briana and Vaughan, Jennifer Wortman and Wallach, Hanna and III, Hal Daumé and Crawford, Kate}, year={2021}, pages={86–92}}
+@misc{kung2018packing,
+ title = {Packing Sparse Convolutional Neural Networks for Efficient Systolic Array Implementations: Column Combining Under Joint Optimization},
+ author = {H. T. Kung and Bradley McDanel and Sai Qian Zhang},
+ year = 2018,
+ eprint = {1811.04770},
+ archiveprefix = {arXiv},
+ primaryclass = {cs.LG}
+}
+
+@incollection{kurkova_survey_2018,
+ title = {A {Survey} on {Deep} {Transfer} {Learning}},
+ author = {Tan, Chuanqi and Sun, Fuchun and Kong, Tao and Zhang, Wenchang and Yang, Chao and Liu, Chunfang},
+ year = 2018,
+ booktitle = {Artificial {Neural} {Networks} and {Machine} {Learning} – {ICANN} 2018},
+ publisher = {Springer International Publishing},
+ address = {Cham},
+ volume = 11141,
+ pages = {270--279},
+ doi = {10.1007/978-3-030-01424-7_27},
+ isbn = {978-3-030-01423-0 978-3-030-01424-7},
+ url = {http://link.springer.com/10.1007/978-3-030-01424-7_27},
+ urldate = {2023-10-26},
+ note = {Series Title: Lecture Notes in Computer Science},
+ language = {en},
+ editor = {Kůrková, Věra and Manolopoulos, Yannis and Hammer, Barbara and Iliadis, Lazaros and Maglogiannis, Ilias},
+ file = {Tan et al. - 2018 - A Survey on Deep Transfer Learning.pdf:/Users/alex/Zotero/storage/5NZ36SGB/Tan et al. - 2018 - A Survey on Deep Transfer Learning.pdf:application/pdf}
+}
-@article{Holland_Hosny_Newman_Joseph_Chmielinski_2020, title={The Dataset Nutrition label}, DOI={10.5040/9781509932771.ch-001}, journal={Data Protection and Privacy}, author={Holland, Sarah and Hosny, Ahmed and Newman, Sarah and Joseph, Joshua and Chmielinski, Kasia}, year={2020}}
+@misc{kuzmin2022fp8,
+ title = {FP8 Quantization: The Power of the Exponent},
+ author = {Andrey Kuzmin and Mart Van Baalen and Yuwei Ren and Markus Nagel and Jorn Peters and Tijmen Blankevoort},
+ year = 2022,
+ eprint = {2208.09225},
+ archiveprefix = {arXiv},
+ primaryclass = {cs.LG}
+}
-@article{Johnson-Roberson_Barto_Mehta_Sridhar_Rosaen_Vasudevan_2017, title={Driving in the matrix: Can virtual worlds replace human-generated annotations for real world tasks?}, DOI={10.1109/icra.2017.7989092}, journal={2017 IEEE International Conference on Robotics and Automation (ICRA)}, author={Johnson-Roberson, Matthew and Barto, Charles and Mehta, Rounak and Sridhar, Sharath Nittur and Rosaen, Karl and Vasudevan, Ram}, year={2017}}
+@misc{kwon_tinytrain_2023,
+ title = {{TinyTrain}: {Deep} {Neural} {Network} {Training} at the {Extreme} {Edge}},
+ shorttitle = {{TinyTrain}},
+ author = {Kwon, Young D. and Li, Rui and Venieris, Stylianos I. and Chauhan, Jagmohan and Lane, Nicholas D. and Mascolo, Cecilia},
+ year = 2023,
+ month = jul,
+ publisher = {arXiv},
+ url = {http://arxiv.org/abs/2307.09988},
+ urldate = {2023-10-26},
+ note = {arXiv:2307.09988 [cs]},
+ language = {en},
+ keywords = {Computer Science - Computer Vision and Pattern Recognition, Computer Science - Machine Learning},
+ file = {Kwon et al. - 2023 - TinyTrain Deep Neural Network Training at the Ext.pdf:/Users/alex/Zotero/storage/L2ST472U/Kwon et al. - 2023 - TinyTrain Deep Neural Network Training at the Ext.pdf:application/pdf}
+}
-@article{Krishnan_Rajpurkar_Topol_2022, title={Self-supervised learning in medicine and Healthcare}, volume={6}, DOI={10.1038/s41551-022-00914-1}, number={12}, journal={Nature Biomedical Engineering}, author={Krishnan, Rayan and Rajpurkar, Pranav and Topol, Eric J.}, year={2022}, pages={1346–1352}}
+@article{kwon2023tinytrain,
+ title = {TinyTrain: Deep Neural Network Training at the Extreme Edge},
+ author = {Kwon, Young D and Li, Rui and Venieris, Stylianos I and Chauhan, Jagmohan and Lane, Nicholas D and Mascolo, Cecilia},
+ year = 2023,
+ journal = {arXiv preprint arXiv:2307.09988}
+}
-@article{Northcutt_Athalye_Mueller_2021, title={Pervasive Label Errors in Test Sets Destabilize Machine Learning Benchmarks}, DOI={ https://doi.org/10.48550/arXiv.2103.14749 arXiv-issued DOI via DataCite}, journal={arXiv}, author={Northcutt, Curtis G and Athalye, Anish and Mueller, Jonas}, year={2021}, month={Mar}}
+@misc{Labelbox,
+ journal = {Labelbox},
+ url = {https://labelbox.com/}
+}
-@article{Pushkarna_Zaldivar_Kjartansson_2022, title={Data cards: Purposeful and transparent dataset documentation for responsible ai}, DOI={10.1145/3531146.3533231}, journal={2022 ACM Conference on Fairness, Accountability, and Transparency}, author={Pushkarna, Mahima and Zaldivar, Andrew and Kjartansson, Oddur}, year={2022}}
+@article{lai2018cmsis,
+ title = {Cmsis-nn: Efficient neural network kernels for arm cortex-m cpus},
+ author = {Lai, Liangzhen and Suda, Naveen and Chandra, Vikas},
+ year = 2018,
+ journal = {arXiv preprint arXiv:1801.06601}
+}
-@article{Ratner_Hancock_Dunnmon_Goldman_Ré_2018, title={Snorkel metal: Weak supervision for multi-task learning.}, DOI={10.1145/3209889.3209898}, journal={Proceedings of the Second Workshop on Data Management for End-To-End Machine Learning}, author={Ratner, Alex and Hancock, Braden and Dunnmon, Jared and Goldman, Roger and Ré, Christopher}, year={2018}}
+@misc{lai2018cmsisnn,
+ title = {CMSIS-NN: Efficient Neural Network Kernels for Arm Cortex-M CPUs},
+ author = {Liangzhen Lai and Naveen Suda and Vikas Chandra},
+ year = 2018,
+ eprint = {1801.06601},
+ archiveprefix = {arXiv},
+ primaryclass = {cs.NE}
+}
-@article{Sheng_Zhang_2019, title={Machine learning with crowdsourcing: A brief summary of the past research and Future Directions}, volume={33}, DOI={10.1609/aaai.v33i01.33019837}, number={01}, journal={Proceedings of the AAAI Conference on Artificial Intelligence}, author={Sheng, Victor S. and Zhang, Jing}, year={2019}, pages={9837–9843}}
+@inproceedings{lecun_optimal_1989,
+ title = {Optimal {Brain} {Damage}},
+ author = {LeCun, Yann and Denker, John and Solla, Sara},
+ year = 1989,
+ booktitle = {Advances in {Neural} {Information} {Processing} {Systems}},
+ publisher = {Morgan-Kaufmann},
+ volume = 2,
+ url = {https://proceedings.neurips.cc/paper/1989/hash/6c9882bbac1c7093bd25041881277658-Abstract.html},
+ urldate = {2023-10-20},
+ abstract = {We have used information-theoretic ideas to derive a class of prac(cid:173) tical and nearly optimal schemes for adapting the size of a neural network. By removing unimportant weights from a network, sev(cid:173) eral improvements can be expected: better generalization, fewer training examples required, and improved speed of learning and/or classification. The basic idea is to use second-derivative informa(cid:173) tion to make a tradeoff between network complexity and training set error. Experiments confirm the usefulness of the methods on a real-world application.},
+ file = {Full Text PDF:/Users/jeffreyma/Zotero/storage/BYHQQSST/LeCun et al. - 1989 - Optimal Brain Damage.pdf:application/pdf}
+}
-@misc{Google, url={https://blog.google/documents/83/}, title={Information quality & content moderation}, author={Google}}
+@article{lecun1989optimal,
+ title = {Optimal brain damage},
+ author = {LeCun, Yann and Denker, John and Solla, Sara},
+ year = 1989,
+ journal = {Advances in neural information processing systems},
+ volume = 2
+}
-@misc{Labelbox, url={https://labelbox.com/}, journal={Labelbox}}
+@article{li2014communication,
+ title = {Communication efficient distributed machine learning with the parameter server},
+ author = {Li, Mu and Andersen, David G and Smola, Alexander J and Yu, Kai},
+ year = 2014,
+ journal = {Advances in Neural Information Processing Systems},
+ volume = 27
+}
-@misc{Perrigo_2023, title={OpenAI used Kenyan workers on less than $2 per hour: Exclusive}, url={https://time.com/6247678/openai-chatgpt-kenya-workers/}, journal={Time}, publisher={Time}, author={Perrigo, Billy}, year={2023}, month={Jan}}
+@article{li2016lightrnn,
+ title = {LightRNN: Memory and computation-efficient recurrent neural networks},
+ author = {Li, Xiang and Qin, Tao and Yang, Jian and Liu, Tie-Yan},
+ year = 2016,
+ journal = {Advances in Neural Information Processing Systems},
+ volume = 29
+}
-@misc{ScaleAI, url={https://scale.com/data-engine}, journal={ScaleAI}}
+@article{li2017deep,
+ title = {Deep reinforcement learning: An overview},
+ author = {Li, Yuxi},
+ year = 2017,
+ journal = {arXiv preprint arXiv:1701.07274}
+}
-@misc{Team_2023, title={Data-centric AI for the Enterprise}, url={https://snorkel.ai/}, journal={Snorkel AI}, author={Team, Snorkel}, year={2023}, month={Aug}}
+@article{li2017learning,
+ title = {Learning without forgetting},
+ author = {Li, Zhizhong and Hoiem, Derek},
+ year = 2017,
+ journal = {IEEE transactions on pattern analysis and machine intelligence},
+ publisher = {IEEE},
+ volume = 40,
+ number = 12,
+ pages = {2935--2947}
+}
-@misc{VinBrain, url={https://vinbrain.net/aiscaler}, journal={VinBrain}}
+@article{li2019edge,
+ title = {Edge AI: On-demand accelerating deep neural network inference via edge computing},
+ author = {Li, En and Zeng, Liekang and Zhou, Zhi and Chen, Xu},
+ year = 2019,
+ journal = {IEEE Transactions on Wireless Communications},
+ publisher = {IEEE},
+ volume = 19,
+ number = 1,
+ pages = {447--457}
+}
-@article{Ardila_Branson_Davis_Henretty_Kohler_Meyer_Morais_Saunders_Tyers_Weber_2020, title={ Common Voice: A Massively-Multilingual Speech Corpus }, journal={Proceedings of the 12th Conference on Language Resources and Evaluation}, author={Ardila, Rosana and Branson, Megan and Davis, Kelly and Henretty, Michael and Kohler, Michael and Meyer, Josh and Morais, Reuben and Saunders, Lindsay and Tyers, Francis M. and Weber, Gregor}, year={2020}, month={May}, pages={4218–4222}}
+@misc{liao_can_2023,
+ title = {Can {Unstructured} {Pruning} {Reduce} the {Depth} in {Deep} {Neural} {Networks}?},
+ author = {Liao, Zhu and Quétu, Victor and Nguyen, Van-Tam and Tartaglione, Enzo},
+ year = 2023,
+ month = aug,
+ publisher = {arXiv},
+ doi = {10.48550/arXiv.2308.06619},
+ url = {http://arxiv.org/abs/2308.06619},
+ urldate = {2023-10-20},
+ note = {arXiv:2308.06619 [cs]},
+ abstract = {Pruning is a widely used technique for reducing the size of deep neural networks while maintaining their performance. However, such a technique, despite being able to massively compress deep models, is hardly able to remove entire layers from a model (even when structured): is this an addressable task? In this study, we introduce EGP, an innovative Entropy Guided Pruning algorithm aimed at reducing the size of deep neural networks while preserving their performance. The key focus of EGP is to prioritize pruning connections in layers with low entropy, ultimately leading to their complete removal. Through extensive experiments conducted on popular models like ResNet-18 and Swin-T, our findings demonstrate that EGP effectively compresses deep neural networks while maintaining competitive performance levels. Our results not only shed light on the underlying mechanism behind the advantages of unstructured pruning, but also pave the way for further investigations into the intricate relationship between entropy, pruning techniques, and deep learning performance. The EGP algorithm and its insights hold great promise for advancing the field of network compression and optimization. The source code for EGP is released open-source.},
+ keywords = {Computer Science - Artificial Intelligence, Computer Science - Machine Learning},
+ file = {arXiv Fulltext PDF:/Users/jeffreyma/Zotero/storage/V6P3XB5H/Liao et al. - 2023 - Can Unstructured Pruning Reduce the Depth in Deep .pdf:application/pdf;arXiv.org Snapshot:/Users/jeffreyma/Zotero/storage/WSQ4ZUH4/2308.html:text/html}
+}
+
+@misc{lin_-device_2022,
+ title = {On-{Device} {Training} {Under} {256KB} {Memory}},
+ author = {Lin, Ji and Zhu, Ligeng and Chen, Wei-Ming and Wang, Wei-Chen and Gan, Chuang and Han, Song},
+ year = 2022,
+ month = nov,
+ publisher = {arXiv},
+ url = {http://arxiv.org/abs/2206.15472},
+ urldate = {2023-10-26},
+ note = {arXiv:2206.15472 [cs]},
+ language = {en},
+ keywords = {Computer Science - Computer Vision and Pattern Recognition},
+ annote = {Comment: NeurIPS 2022},
+ file = {Lin et al. - 2022 - On-Device Training Under 256KB Memory.pdf:/Users/alex/Zotero/storage/GMF6SWGT/Lin et al. - 2022 - On-Device Training Under 256KB Memory.pdf:application/pdf}
+}
+
+@misc{lin_-device_2022-1,
+ title = {On-{Device} {Training} {Under} {256KB} {Memory}},
+ author = {Lin, Ji and Zhu, Ligeng and Chen, Wei-Ming and Wang, Wei-Chen and Gan, Chuang and Han, Song},
+ year = 2022,
+ month = nov,
+ publisher = {arXiv},
+ url = {http://arxiv.org/abs/2206.15472},
+ urldate = {2023-10-25},
+ note = {arXiv:2206.15472 [cs]},
+ language = {en},
+ keywords = {Computer Science - Computer Vision and Pattern Recognition},
+ annote = {Comment: NeurIPS 2022},
+ file = {Lin et al. - 2022 - On-Device Training Under 256KB Memory.pdf:/Users/alex/Zotero/storage/DNIY32R2/Lin et al. - 2022 - On-Device Training Under 256KB Memory.pdf:application/pdf}
+}
-@article{vinuesa2020role,
- title={The role of artificial intelligence in achieving the Sustainable Development Goals},
- author={Vinuesa, Ricardo and Azizpour, Hossein and Leite, Iolanda and Balaam, Madeline and Dignum, Virginia and Domisch, Sami and Fell{\"a}nder, Anna and Langhans, Simone Daniela and Tegmark, Max and Fuso Nerini, Francesco},
- journal={Nature communications},
- volume={11},
- number={1},
- pages={1--10},
- year={2020},
- publisher={Nature Publishing Group}
+@misc{lin_mcunet_2020,
+ title = {{MCUNet}: {Tiny} {Deep} {Learning} on {IoT} {Devices}},
+ shorttitle = {{MCUNet}},
+ author = {Lin, Ji and Chen, Wei-Ming and Lin, Yujun and Cohn, John and Gan, Chuang and Han, Song},
+ year = 2020,
+ month = nov,
+ publisher = {arXiv},
+ doi = {10.48550/arXiv.2007.10319},
+ url = {http://arxiv.org/abs/2007.10319},
+ urldate = {2023-10-20},
+ note = {arXiv:2007.10319 [cs]},
+ abstract = {Machine learning on tiny IoT devices based on microcontroller units (MCU) is appealing but challenging: the memory of microcontrollers is 2-3 orders of magnitude smaller even than mobile phones. We propose MCUNet, a framework that jointly designs the efficient neural architecture (TinyNAS) and the lightweight inference engine (TinyEngine), enabling ImageNet-scale inference on microcontrollers. TinyNAS adopts a two-stage neural architecture search approach that first optimizes the search space to fit the resource constraints, then specializes the network architecture in the optimized search space. TinyNAS can automatically handle diverse constraints (i.e.device, latency, energy, memory) under low search costs.TinyNAS is co-designed with TinyEngine, a memory-efficient inference library to expand the search space and fit a larger model. TinyEngine adapts the memory scheduling according to the overall network topology rather than layer-wise optimization, reducing the memory usage by 4.8x, and accelerating the inference by 1.7-3.3x compared to TF-Lite Micro and CMSIS-NN. MCUNet is the first to achieves {\textgreater}70\% ImageNet top1 accuracy on an off-the-shelf commercial microcontroller, using 3.5x less SRAM and 5.7x less Flash compared to quantized MobileNetV2 and ResNet-18. On visual\&audio wake words tasks, MCUNet achieves state-of-the-art accuracy and runs 2.4-3.4x faster than MobileNetV2 and ProxylessNAS-based solutions with 3.7-4.1x smaller peak SRAM. Our study suggests that the era of always-on tiny machine learning on IoT devices has arrived. Code and models can be found here: https://tinyml.mit.edu.},
+ keywords = {Computer Science - Computer Vision and Pattern Recognition},
+ file = {arXiv Fulltext PDF:/Users/jeffreyma/Zotero/storage/IX2JN4P9/Lin et al. - 2020 - MCUNet Tiny Deep Learning on IoT Devices.pdf:application/pdf;arXiv.org Snapshot:/Users/jeffreyma/Zotero/storage/BAKHZ46Y/2007.html:text/html},
+ language = {en},
+ annote = {Comment: NeurIPS 2020 (spotlight)}
}
-@inproceedings{altayeb2022classifying,
- title={Classifying mosquito wingbeat sound using TinyML},
- author={Altayeb, Moez and Zennaro, Marco and Rovai, Marcelo},
- booktitle={Proceedings of the 2022 ACM Conference on Information Technology for Social Good},
- pages={132--137},
- year={2022}
+@inproceedings{lin2014microsoft,
+ title = {Microsoft coco: Common objects in context},
+ author = {Lin, Tsung-Yi and Maire, Michael and Belongie, Serge and Hays, James and Perona, Pietro and Ramanan, Deva and Doll{\'a}r, Piotr and Zitnick, C Lawrence},
+ year = 2014,
+ booktitle = {Computer Vision--ECCV 2014: 13th European Conference, Zurich, Switzerland, September 6-12, 2014, Proceedings, Part V 13},
+ pages = {740--755},
+ organization = {Springer}
}
-@article{yamashita2023coffee,
- title={Coffee disease classification at the edge using deep learning},
- author={Yamashita, Jo{\~a}o Vitor Yukio Bordin and Leite, Jo{\~a}o Paulo RR},
- journal={Smart Agricultural Technology},
- volume={4},
- pages={100183},
- year={2023},
- publisher={Elsevier}
+@article{lin2020mcunet,
+ title = {Mcunet: Tiny deep learning on iot devices},
+ author = {Lin, Ji and Chen, Wei-Ming and Lin, Yujun and Gan, Chuang and Han, Song and others},
+ year = 2020,
+ journal = {Advances in Neural Information Processing Systems},
+ volume = 33,
+ pages = {11711--11722},
+ eprint = {2007.10319},
+ archiveprefix = {arXiv},
+ primaryclass = {cs.CV}
+}
+
+@article{lin2022device,
+ title = {On-device training under 256kb memory},
+ author = {Lin, Ji and Zhu, Ligeng and Chen, Wei-Ming and Wang, Wei-Chen and Gan, Chuang and Han, Song},
+ year = 2022,
+ journal = {Advances in Neural Information Processing Systems},
+ volume = 35,
+ pages = {22941--22954}
}
-@inproceedings{bamoumen2022tinyml,
- title={How TinyML Can be Leveraged to Solve Environmental Problems: A Survey},
- author={Bamoumen, Hatim and Temouden, Anas and Benamar, Nabil and Chtouki, Yousra},
- booktitle={2022 International Conference on Innovation and Intelligence for Informatics, Computing, and Technologies (3ICT)},
- pages={338--343},
- year={2022},
- organization={IEEE}
+@misc{lu_notes_2016,
+ title = {Notes on {Low}-rank {Matrix} {Factorization}},
+ author = {Lu, Yuan and Yang, Jie},
+ year = 2016,
+ month = may,
+ publisher = {arXiv},
+ doi = {10.48550/arXiv.1507.00333},
+ url = {http://arxiv.org/abs/1507.00333},
+ urldate = {2023-10-20},
+ note = {arXiv:1507.00333 [cs]},
+ abstract = {Low-rank matrix factorization (MF) is an important technique in data science. The key idea of MF is that there exists latent structures in the data, by uncovering which we could obtain a compressed representation of the data. By factorizing an original matrix to low-rank matrices, MF provides a unified method for dimension reduction, clustering, and matrix completion. In this article we review several important variants of MF, including: Basic MF, Non-negative MF, Orthogonal non-negative MF. As can be told from their names, non-negative MF and orthogonal non-negative MF are variants of basic MF with non-negativity and/or orthogonality constraints. Such constraints are useful in specific senarios. In the first part of this article, we introduce, for each of these models, the application scenarios, the distinctive properties, and the optimizing method. By properly adapting MF, we can go beyond the problem of clustering and matrix completion. In the second part of this article, we will extend MF to sparse matrix compeletion, enhance matrix compeletion using various regularization methods, and make use of MF for (semi-)supervised learning by introducing latent space reinforcement and transformation. We will see that MF is not only a useful model but also as a flexible framework that is applicable for various prediction problems.},
+ keywords = {Computer Science - Information Retrieval, Computer Science - Machine Learning, Mathematics - Numerical Analysis},
+ file = {arXiv Fulltext PDF:/Users/jeffreyma/Zotero/storage/4QED5ZU9/Lu and Yang - 2016 - Notes on Low-rank Matrix Factorization.pdf:application/pdf;arXiv.org Snapshot:/Users/jeffreyma/Zotero/storage/XIBZBDJQ/1507.html:text/html}
}
-@inproceedings{ooko2021tinyml,
- title={TinyML in Africa: Opportunities and challenges},
- author={Ooko, Samson Otieno and Ogore, Marvin Muyonga and Nsenga, Jimmy and Zennaro, Marco},
- booktitle={2021 IEEE Globecom Workshops (GC Wkshps)},
- pages={1--6},
- year={2021},
- organization={IEEE}
+@article{lundberg2017unified,
+ title = {A unified approach to interpreting model predictions},
+ author = {Lundberg, Scott M and Lee, Su-In},
+ year = 2017,
+ journal = {Advances in neural information processing systems},
+ volume = 30
}
+@article{mattson2020mlperf,
+ title = {Mlperf training benchmark},
+ author = {Mattson, Peter and Cheng, Christine and Diamos, Gregory and Coleman, Cody and Micikevicius, Paulius and Patterson, David and Tang, Hanlin and Wei, Gu-Yeon and Bailis, Peter and Bittorf, Victor and others},
+ year = 2020,
+ journal = {Proceedings of Machine Learning and Systems},
+ volume = 2,
+ pages = {336--349}
+}
+
+@inproceedings{mcmahan2017communication,
+ title = {Communication-efficient learning of deep networks from decentralized data},
+ author = {McMahan, Brendan and Moore, Eider and Ramage, Daniel and Hampson, Seth and y Arcas, Blaise Aguera},
+ year = 2017,
+ booktitle = {Artificial intelligence and statistics},
+ pages = {1273--1282},
+ organization = {PMLR}
+}
+
+@inproceedings{mcmahan2023communicationefficient,
+ title = {Communication-efficient learning of deep networks from decentralized data},
+ author = {McMahan, Brendan and Moore, Eider and Ramage, Daniel and Hampson, Seth and y Arcas, Blaise Aguera},
+ year = 2017,
+ booktitle = {Artificial intelligence and statistics},
+ pages = {1273--1282},
+ organization = {PMLR}
+}
+
+@article{moshawrab2023reviewing,
+ title = {Reviewing Federated Learning Aggregation Algorithms; Strategies, Contributions, Limitations and Future Perspectives},
+ author = {Moshawrab, Mohammad and Adda, Mehdi and Bouzouane, Abdenour and Ibrahim, Hussein and Raad, Ali},
+ year = 2023,
+ journal = {Electronics},
+ publisher = {MDPI},
+ volume = 12,
+ number = 10,
+ pages = 2287
+}
+
+@inproceedings{nguyen2023re,
+ title = {Re-thinking Model Inversion Attacks Against Deep Neural Networks},
+ author = {Nguyen, Ngoc-Bao and Chandrasegaran, Keshigeyan and Abdollahzadeh, Milad and Cheung, Ngai-Man},
+ year = 2023,
+ booktitle = {Proceedings of the IEEE/CVF Conference on Computer Vision and Pattern Recognition},
+ pages = {16384--16393}
+}
-@inproceedings{zennaro2022tinyml,
- title={TinyML: applied AI for development},
- author={Zennaro, Marco and Plancher, Brian and Reddi, V Janapa},
- booktitle={The UN 7th Multi-stakeholder Forum on Science, Technology and Innovation for the Sustainable Development Goals},
- pages={2022--05},
- year={2022}
+@misc{noauthor_deep_nodate,
+ title = {Deep {Learning} {Model} {Compression} (ii) {\textbar} by {Ivy} {Gu} {\textbar} {Medium}},
+ author = {Ivy Gu},
+ year = 2023,
+ url = {https://ivygdy.medium.com/deep-learning-model-compression-ii-546352ea9453},
+ urldate = {2023-10-20}
}
-@article{zennarobridging,
- title={Bridging the Digital Divide: the Promising Impact of TinyML for Developing Countries},
- author={Zennaro, Marco and Plancher, Brian and Reddi, Vijay Janapa}
+@misc{noauthor_introduction_nodate,
+ title = {An {Introduction} to {Separable} {Convolutions} - {Analytics} {Vidhya}},
+ author = {Hegde, Sumant},
+ year = 2023,
+ url = {https://www.analyticsvidhya.com/blog/2021/11/an-introduction-to-separable-convolutions/},
+ urldate = {2023-10-20}
}
+@misc{noauthor_knowledge_nodate,
+ title = {Knowledge {Distillation} - {Neural} {Network} {Distiller}},
+ author = {IntelLabs},
+ year = 2023,
+ url = {https://intellabs.github.io/distiller/knowledge_distillation.html},
+ urldate = {2023-10-20}
+}
-@misc{Sheth_2022, title={Eletect - TinyML and IOT based Smart Wildlife Tracker}, url={https://www.hackster.io/dhruvsheth_/eletect-tinyml-and-iot-based-smart-wildlife-tracker-c03e5a}, journal={Hackster.io}, author={Sheth, Dhruv}, year={2022}, month={Mar}}
+@article{Northcutt_Athalye_Mueller_2021,
+ title = {Pervasive Label Errors in Test Sets Destabilize Machine Learning Benchmarks},
+ author = {Northcutt, Curtis G and Athalye, Anish and Mueller, Jonas},
+ year = 2021,
+ month = {Mar},
+ journal = {arXiv},
+ doi = { https://doi.org/10.48550/arXiv.2103.14749 arXiv-issued DOI via DataCite}
+}
-@misc{Verma_2022, title={Elephant AI}, url={https://www.hackster.io/dual_boot/elephant-ai-ba71e9}, journal={Hackster.io}, author={Verma, Team Dual_Boot: Swapnil}, year={2022}, month={Mar}}
+@inproceedings{ooko2021tinyml,
+ title = {TinyML in Africa: Opportunities and challenges},
+ author = {Ooko, Samson Otieno and Ogore, Marvin Muyonga and Nsenga, Jimmy and Zennaro, Marco},
+ year = 2021,
+ booktitle = {2021 IEEE Globecom Workshops (GC Wkshps)},
+ pages = {1--6},
+ organization = {IEEE}
+}
-@misc{Rao_2021, url={https://www.wevolver.com/article/tinyml-unlocks-new-possibilities-for-sustainable-development-technologies}, journal={www.wevolver.com}, author={Rao, Ravi}, year={2021}, month={Dec}}
+@misc{ou_low_2023,
+ title = {Low {Rank} {Optimization} for {Efficient} {Deep} {Learning}: {Making} {A} {Balance} between {Compact} {Architecture} and {Fast} {Training}},
+ shorttitle = {Low {Rank} {Optimization} for {Efficient} {Deep} {Learning}},
+ author = {Ou, Xinwei and Chen, Zhangxin and Zhu, Ce and Liu, Yipeng},
+ year = 2023,
+ month = mar,
+ publisher = {arXiv},
+ url = {http://arxiv.org/abs/2303.13635},
+ urldate = {2023-10-20},
+ note = {arXiv:2303.13635 [cs]},
+ abstract = {Deep neural networks have achieved great success in many data processing applications. However, the high computational complexity and storage cost makes deep learning hard to be used on resource-constrained devices, and it is not environmental-friendly with much power cost. In this paper, we focus on low-rank optimization for efficient deep learning techniques. In the space domain, deep neural networks are compressed by low rank approximation of the network parameters, which directly reduces the storage requirement with a smaller number of network parameters. In the time domain, the network parameters can be trained in a few subspaces, which enables efficient training for fast convergence. The model compression in the spatial domain is summarized into three categories as pre-train, pre-set, and compression-aware methods, respectively. With a series of integrable techniques discussed, such as sparse pruning, quantization, and entropy coding, we can ensemble them in an integration framework with lower computational complexity and storage. Besides of summary of recent technical advances, we have two findings for motivating future works: one is that the effective rank outperforms other sparse measures for network compression. The other is a spatial and temporal balance for tensorized neural networks.},
+ keywords = {Computer Science - Machine Learning},
+ file = {arXiv.org Snapshot:/Users/jeffreyma/Zotero/storage/SPSZ2HR9/2303.html:text/html;Full Text PDF:/Users/jeffreyma/Zotero/storage/6TUEBTEX/Ou et al. - 2023 - Low Rank Optimization for Efficient Deep Learning.pdf:application/pdf}
+}
+
+@article{pan_survey_2010,
+ title = {A {Survey} on {Transfer} {Learning}},
+ author = {Pan, Sinno Jialin and Yang, Qiang},
+ year = 2010,
+ month = oct,
+ journal = {IEEE Transactions on Knowledge and Data Engineering},
+ volume = 22,
+ number = 10,
+ pages = {1345--1359},
+ doi = {10.1109/TKDE.2009.191},
+ issn = {1041-4347},
+ url = {http://ieeexplore.ieee.org/document/5288526/},
+ urldate = {2023-10-25},
+ language = {en},
+ file = {Pan and Yang - 2010 - A Survey on Transfer Learning.pdf:/Users/alex/Zotero/storage/T3H8E5K8/Pan and Yang - 2010 - A Survey on Transfer Learning.pdf:application/pdf}
+}
+
+@article{pan2009survey,
+ title = {A survey on transfer learning},
+ author = {Pan, Sinno Jialin and Yang, Qiang},
+ year = 2009,
+ journal = {IEEE Transactions on knowledge and data engineering},
+ publisher = {IEEE},
+ volume = 22,
+ number = 10,
+ pages = {1345--1359}
+}
+
+@article{parisi_continual_2019,
+ title = {Continual lifelong learning with neural networks: {A} review},
+ shorttitle = {Continual lifelong learning with neural networks},
+ author = {Parisi, German I. and Kemker, Ronald and Part, Jose L. and Kanan, Christopher and Wermter, Stefan},
+ year = 2019,
+ month = may,
+ journal = {Neural Networks},
+ volume = 113,
+ pages = {54--71},
+ doi = {10.1016/j.neunet.2019.01.012},
+ issn = {08936080},
+ url = {https://linkinghub.elsevier.com/retrieve/pii/S0893608019300231},
+ urldate = {2023-10-26},
+ language = {en},
+ file = {Parisi et al. - 2019 - Continual lifelong learning with neural networks .pdf:/Users/alex/Zotero/storage/TCGHD5TW/Parisi et al. - 2019 - Continual lifelong learning with neural networks .pdf:application/pdf}
+}
+@article{paszke2019pytorch,
+ title = {Pytorch: An imperative style, high-performance deep learning library},
+ author = {Paszke, Adam and Gross, Sam and Massa, Francisco and Lerer, Adam and Bradbury, James and Chanan, Gregory and Killeen, Trevor and Lin, Zeming and Gimelshein, Natalia and Antiga, Luca and others},
+ year = 2019,
+ journal = {Advances in neural information processing systems},
+ volume = 32
+}
-@misc{hinton_distilling_2015,
- title = {Distilling the {Knowledge} in a {Neural} {Network}},
- url = {http://arxiv.org/abs/1503.02531},
- doi = {10.48550/arXiv.1503.02531},
- abstract = {A very simple way to improve the performance of almost any machine learning algorithm is to train many different models on the same data and then to average their predictions. Unfortunately, making predictions using a whole ensemble of models is cumbersome and may be too computationally expensive to allow deployment to a large number of users, especially if the individual models are large neural nets. Caruana and his collaborators have shown that it is possible to compress the knowledge in an ensemble into a single model which is much easier to deploy and we develop this approach further using a different compression technique. We achieve some surprising results on MNIST and we show that we can significantly improve the acoustic model of a heavily used commercial system by distilling the knowledge in an ensemble of models into a single model. We also introduce a new type of ensemble composed of one or more full models and many specialist models which learn to distinguish fine-grained classes that the full models confuse. Unlike a mixture of experts, these specialist models can be trained rapidly and in parallel.},
- urldate = {2023-10-20},
- publisher = {arXiv},
- author = {Hinton, Geoffrey and Vinyals, Oriol and Dean, Jeff},
- month = mar,
- year = {2015},
- note = {arXiv:1503.02531 [cs, stat]},
- keywords = {Computer Science - Machine Learning, Computer Science - Neural and Evolutionary Computing, Statistics - Machine Learning},
- file = {arXiv Fulltext PDF:/Users/jeffreyma/Zotero/storage/VREDW45A/Hinton et al. - 2015 - Distilling the Knowledge in a Neural Network.pdf:application/pdf;arXiv.org Snapshot:/Users/jeffreyma/Zotero/storage/8MNJG4RP/1503.html:text/html},
+@misc{Perrigo_2023,
+ title = {OpenAI used Kenyan workers on less than $2 per hour: Exclusive},
+ author = {Perrigo, Billy},
+ year = 2023,
+ month = {Jan},
+ journal = {Time},
+ publisher = {Time},
+ url = {https://time.com/6247678/openai-chatgpt-kenya-workers/}
}
-@misc{frankle_lottery_2019,
- title = {The {Lottery} {Ticket} {Hypothesis}: {Finding} {Sparse}, {Trainable} {Neural} {Networks}},
- shorttitle = {The {Lottery} {Ticket} {Hypothesis}},
- url = {http://arxiv.org/abs/1803.03635},
- doi = {10.48550/arXiv.1803.03635},
- abstract = {Neural network pruning techniques can reduce the parameter counts of trained networks by over 90\%, decreasing storage requirements and improving computational performance of inference without compromising accuracy. However, contemporary experience is that the sparse architectures produced by pruning are difficult to train from the start, which would similarly improve training performance. We find that a standard pruning technique naturally uncovers subnetworks whose initializations made them capable of training effectively. Based on these results, we articulate the "lottery ticket hypothesis:" dense, randomly-initialized, feed-forward networks contain subnetworks ("winning tickets") that - when trained in isolation - reach test accuracy comparable to the original network in a similar number of iterations. The winning tickets we find have won the initialization lottery: their connections have initial weights that make training particularly effective. We present an algorithm to identify winning tickets and a series of experiments that support the lottery ticket hypothesis and the importance of these fortuitous initializations. We consistently find winning tickets that are less than 10-20\% of the size of several fully-connected and convolutional feed-forward architectures for MNIST and CIFAR10. Above this size, the winning tickets that we find learn faster than the original network and reach higher test accuracy.},
- urldate = {2023-10-20},
- publisher = {arXiv},
- author = {Frankle, Jonathan and Carbin, Michael},
- month = mar,
- year = {2019},
- note = {arXiv:1803.03635 [cs]},
- keywords = {Computer Science - Artificial Intelligence, Computer Science - Machine Learning, Computer Science - Neural and Evolutionary Computing},
- file = {arXiv Fulltext PDF:/Users/jeffreyma/Zotero/storage/6STHYGW5/Frankle and Carbin - 2019 - The Lottery Ticket Hypothesis Finding Sparse, Tra.pdf:application/pdf;arXiv.org Snapshot:/Users/jeffreyma/Zotero/storage/QGNSCTQB/1803.html:text/html},
+@inproceedings{Prakash_2023,
+ title = {{CFU} Playground: Full-Stack Open-Source Framework for Tiny Machine Learning ({TinyML}) Acceleration on {FPGAs}},
+ author = {Shvetank Prakash and Tim Callahan and Joseph Bushagour and Colby Banbury and Alan V. Green and Pete Warden and Tim Ansell and Vijay Janapa Reddi},
+ year = 2023,
+ month = {apr},
+ booktitle = {2023 {IEEE} International Symposium on Performance Analysis of Systems and Software ({ISPASS})},
+ publisher = {{IEEE}},
+ doi = {10.1109/ispass57527.2023.00024},
+ url = {https://doi.org/10.1109%2Fispass57527.2023.00024}
+}
+
+@inproceedings{prakash_cfu_2023,
+ title = {{CFU} {Playground}: {Full}-{Stack} {Open}-{Source} {Framework} for {Tiny} {Machine} {Learning} ({tinyML}) {Acceleration} on {FPGAs}},
+ shorttitle = {{CFU} {Playground}},
+ author = {Prakash, Shvetank and Callahan, Tim and Bushagour, Joseph and Banbury, Colby and Green, Alan V. and Warden, Pete and Ansell, Tim and Reddi, Vijay Janapa},
+ year = 2023,
+ month = apr,
+ booktitle = {2023 {IEEE} {International} {Symposium} on {Performance} {Analysis} of {Systems} and {Software} ({ISPASS})},
+ pages = {157--167},
+ doi = {10.1109/ISPASS57527.2023.00024},
+ url = {http://arxiv.org/abs/2201.01863},
+ urldate = {2023-10-25},
+ note = {arXiv:2201.01863 [cs]},
+ language = {en},
+ keywords = {Computer Science - Machine Learning, Computer Science - Distributed, Parallel, and Cluster Computing, Computer Science - Hardware Architecture},
+ file = {Prakash et al. - 2023 - CFU Playground Full-Stack Open-Source Framework f.pdf:/Users/alex/Zotero/storage/BZNRIDTL/Prakash et al. - 2023 - CFU Playground Full-Stack Open-Source Framework f.pdf:application/pdf}
+}
+
+@article{preparednesspublic,
+ title = {Public Health Law},
+ author = {Preparedness, Emergency}
+}
+
+@article{Pushkarna_Zaldivar_Kjartansson_2022,
+ title = {Data cards: Purposeful and transparent dataset documentation for responsible ai},
+ author = {Pushkarna, Mahima and Zaldivar, Andrew and Kjartansson, Oddur},
+ year = 2022,
+ journal = {2022 ACM Conference on Fairness, Accountability, and Transparency},
+ doi = {10.1145/3531146.3533231}
}
@article{qi_efficient_2021,
- title = {An efficient pruning scheme of deep neural networks for {Internet} of {Things} applications},
- volume = {2021},
- doi = {10.1186/s13634-021-00744-4},
- abstract = {Nowadays, deep neural networks (DNNs) have been rapidly deployed to realize a number of functionalities like sensing, imaging, classification, recognition, etc. However, the computational-intensive requirement of DNNs makes it difficult to be applicable for resource-limited Internet of Things (IoT) devices. In this paper, we propose a novel pruning-based paradigm that aims to reduce the computational cost of DNNs, by uncovering a more compact structure and learning the effective weights therein, on the basis of not compromising the expressive capability of DNNs. In particular, our algorithm can achieve efficient end-to-end training that transfers a redundant neural network to a compact one with a specifically targeted compression rate directly. We comprehensively evaluate our approach on various representative benchmark datasets and compared with typical advanced convolutional neural network (CNN) architectures. The experimental results verify the superior performance and robust effectiveness of our scheme. For example, when pruning VGG on CIFAR-10, our proposed scheme is able to significantly reduce its FLOPs (floating-point operations) and number of parameters with a proportion of 76.2\% and 94.1\%, respectively, while still maintaining a satisfactory accuracy. To sum up, our scheme could facilitate the integration of DNNs into the common machine-learning-based IoT framework and establish distributed training of neural networks in both cloud and edge.},
- journal = {EURASIP Journal on Advances in Signal Processing},
- author = {Qi, Chen and Shen, Shibo and Li, Rongpeng and Zhifeng, Zhao and Liu, Qing and Liang, Jing and Zhang, Honggang},
- month = jun,
- year = {2021},
- file = {Full Text PDF:/Users/jeffreyma/Zotero/storage/AGWCC5VS/Qi et al. - 2021 - An efficient pruning scheme of deep neural network.pdf:application/pdf},
+ title = {An efficient pruning scheme of deep neural networks for {Internet} of {Things} applications},
+ author = {Qi, Chen and Shen, Shibo and Li, Rongpeng and Zhifeng, Zhao and Liu, Qing and Liang, Jing and Zhang, Honggang},
+ year = 2021,
+ month = jun,
+ journal = {EURASIP Journal on Advances in Signal Processing},
+ volume = 2021,
+ doi = {10.1186/s13634-021-00744-4},
+ abstract = {Nowadays, deep neural networks (DNNs) have been rapidly deployed to realize a number of functionalities like sensing, imaging, classification, recognition, etc. However, the computational-intensive requirement of DNNs makes it difficult to be applicable for resource-limited Internet of Things (IoT) devices. In this paper, we propose a novel pruning-based paradigm that aims to reduce the computational cost of DNNs, by uncovering a more compact structure and learning the effective weights therein, on the basis of not compromising the expressive capability of DNNs. In particular, our algorithm can achieve efficient end-to-end training that transfers a redundant neural network to a compact one with a specifically targeted compression rate directly. We comprehensively evaluate our approach on various representative benchmark datasets and compared with typical advanced convolutional neural network (CNN) architectures. The experimental results verify the superior performance and robust effectiveness of our scheme. For example, when pruning VGG on CIFAR-10, our proposed scheme is able to significantly reduce its FLOPs (floating-point operations) and number of parameters with a proportion of 76.2\% and 94.1\%, respectively, while still maintaining a satisfactory accuracy. To sum up, our scheme could facilitate the integration of DNNs into the common machine-learning-based IoT framework and establish distributed training of neural networks in both cloud and edge.},
+ file = {Full Text PDF:/Users/jeffreyma/Zotero/storage/AGWCC5VS/Qi et al. - 2021 - An efficient pruning scheme of deep neural network.pdf:application/pdf}
}
-@misc{noauthor_knowledge_nodate,
- title = {Knowledge {Distillation} - {Neural} {Network} {Distiller}},
- url = {https://intellabs.github.io/distiller/knowledge_distillation.html},
- author = {IntelLabs},
- urldate = {2023-10-20},
- year = {2023}
+@misc{quantdeep,
+ title = {Quantizing deep convolutional networks for efficient inference: A whitepaper},
+ author = {Krishnamoorthi},
+ year = 2018,
+ month = jun,
+ publisher = {arXiv},
+ doi = {10.48550/arXiv.1806.08342},
+ url = {https://arxiv.org/abs/1806.08342},
+ urldate = {2018-06-21},
+ abstract = {We present an overview of techniques for quantizing convolutional neural networks for inference with integer weights and activations. Per-channel quantization of weights and per-layer quantization of activations to 8-bits of precision post-training produces classification accuracies within 2% of floating point networks for a wide variety of CNN architectures. Model sizes can be reduced by a factor of 4 by quantizing weights to 8-bits, even when 8-bit arithmetic is not supported. This can be achieved with simple, post training quantization of weights.We benchmark latencies of quantized networks on CPUs and DSPs and observe a speedup of 2x-3x for quantized implementations compared to floating point on CPUs. Speedups of up to 10x are observed on specialized processors with fixed point SIMD capabilities, like the Qualcomm QDSPs with HVX. Quantization-aware training can provide further improvements, reducing the gap to floating point to 1% at 8-bit precision. Quantization-aware training also allows for reducing the precision of weights to four bits with accuracy losses ranging from 2% to 10%, with higher accuracy drop for smaller networks.We introduce tools in TensorFlow and TensorFlowLite for quantizing convolutional networks and review best practices for quantization-aware training to obtain high accuracy with quantized weights and activations. We recommend that per-channel quantization of weights and per-layer quantization of activations be the preferred quantization scheme for hardware acceleration and kernel optimization. We also propose that future processors and hardware accelerators for optimized inference support precisions of 4, 8 and 16 bits.}
}
-@misc{noauthor_deep_nodate,
- title = {Deep {Learning} {Model} {Compression} (ii) {\textbar} by {Ivy} {Gu} {\textbar} {Medium}},
- url = {https://ivygdy.medium.com/deep-learning-model-compression-ii-546352ea9453},
- urldate = {2023-10-20},
- author = {Ivy Gu},
- year = {2023}
+@article{ramcharan2017deep,
+ title = {Deep learning for image-based cassava disease detection},
+ author = {Ramcharan, Amanda and Baranowski, Kelsee and McCloskey, Peter and Ahmed, Babuali and Legg, James and Hughes, David P},
+ year = 2017,
+ journal = {Frontiers in plant science},
+ publisher = {Frontiers Media SA},
+ volume = 8,
+ pages = 1852
}
-@misc{lu_notes_2016,
- title = {Notes on {Low}-rank {Matrix} {Factorization}},
- url = {http://arxiv.org/abs/1507.00333},
- doi = {10.48550/arXiv.1507.00333},
- abstract = {Low-rank matrix factorization (MF) is an important technique in data science. The key idea of MF is that there exists latent structures in the data, by uncovering which we could obtain a compressed representation of the data. By factorizing an original matrix to low-rank matrices, MF provides a unified method for dimension reduction, clustering, and matrix completion. In this article we review several important variants of MF, including: Basic MF, Non-negative MF, Orthogonal non-negative MF. As can be told from their names, non-negative MF and orthogonal non-negative MF are variants of basic MF with non-negativity and/or orthogonality constraints. Such constraints are useful in specific senarios. In the first part of this article, we introduce, for each of these models, the application scenarios, the distinctive properties, and the optimizing method. By properly adapting MF, we can go beyond the problem of clustering and matrix completion. In the second part of this article, we will extend MF to sparse matrix compeletion, enhance matrix compeletion using various regularization methods, and make use of MF for (semi-)supervised learning by introducing latent space reinforcement and transformation. We will see that MF is not only a useful model but also as a flexible framework that is applicable for various prediction problems.},
- urldate = {2023-10-20},
- publisher = {arXiv},
- author = {Lu, Yuan and Yang, Jie},
- month = may,
- year = {2016},
- note = {arXiv:1507.00333 [cs]},
- keywords = {Computer Science - Information Retrieval, Computer Science - Machine Learning, Mathematics - Numerical Analysis},
- file = {arXiv Fulltext PDF:/Users/jeffreyma/Zotero/storage/4QED5ZU9/Lu and Yang - 2016 - Notes on Low-rank Matrix Factorization.pdf:application/pdf;arXiv.org Snapshot:/Users/jeffreyma/Zotero/storage/XIBZBDJQ/1507.html:text/html},
+@misc{Rao_2021,
+ author = {Rao, Ravi},
+ year = 2021,
+ month = {Dec},
+ journal = {www.wevolver.com},
+ url = {https://www.wevolver.com/article/tinyml-unlocks-new-possibilities-for-sustainable-development-technologies}
}
-@misc{ou_low_2023,
- title = {Low {Rank} {Optimization} for {Efficient} {Deep} {Learning}: {Making} {A} {Balance} between {Compact} {Architecture} and {Fast} {Training}},
- shorttitle = {Low {Rank} {Optimization} for {Efficient} {Deep} {Learning}},
- url = {http://arxiv.org/abs/2303.13635},
- abstract = {Deep neural networks have achieved great success in many data processing applications. However, the high computational complexity and storage cost makes deep learning hard to be used on resource-constrained devices, and it is not environmental-friendly with much power cost. In this paper, we focus on low-rank optimization for efficient deep learning techniques. In the space domain, deep neural networks are compressed by low rank approximation of the network parameters, which directly reduces the storage requirement with a smaller number of network parameters. In the time domain, the network parameters can be trained in a few subspaces, which enables efficient training for fast convergence. The model compression in the spatial domain is summarized into three categories as pre-train, pre-set, and compression-aware methods, respectively. With a series of integrable techniques discussed, such as sparse pruning, quantization, and entropy coding, we can ensemble them in an integration framework with lower computational complexity and storage. Besides of summary of recent technical advances, we have two findings for motivating future works: one is that the effective rank outperforms other sparse measures for network compression. The other is a spatial and temporal balance for tensorized neural networks.},
- urldate = {2023-10-20},
- publisher = {arXiv},
- author = {Ou, Xinwei and Chen, Zhangxin and Zhu, Ce and Liu, Yipeng},
- month = mar,
- year = {2023},
- note = {arXiv:2303.13635 [cs]},
- keywords = {Computer Science - Machine Learning},
- file = {arXiv.org Snapshot:/Users/jeffreyma/Zotero/storage/SPSZ2HR9/2303.html:text/html;Full Text PDF:/Users/jeffreyma/Zotero/storage/6TUEBTEX/Ou et al. - 2023 - Low Rank Optimization for Efficient Deep Learning.pdf:application/pdf},
+@article{Ratner_Hancock_Dunnmon_Goldman_Ré_2018,
+ title = {Snorkel metal: Weak supervision for multi-task learning.},
+ author = {Ratner, Alex and Hancock, Braden and Dunnmon, Jared and Goldman, Roger and Ré, Christopher},
+ year = 2018,
+ journal = {Proceedings of the Second Workshop on Data Management for End-To-End Machine Learning},
+ doi = {10.1145/3209889.3209898}
}
-@misc{he_structured_2023,
- title = {Structured {Pruning} for {Deep} {Convolutional} {Neural} {Networks}: {A} survey},
- shorttitle = {Structured {Pruning} for {Deep} {Convolutional} {Neural} {Networks}},
- url = {http://arxiv.org/abs/2303.00566},
- doi = {10.48550/arXiv.2303.00566},
- abstract = {The remarkable performance of deep Convolutional neural networks (CNNs) is generally attributed to their deeper and wider architectures, which can come with significant computational costs. Pruning neural networks has thus gained interest since it effectively lowers storage and computational costs. In contrast to weight pruning, which results in unstructured models, structured pruning provides the benefit of realistic acceleration by producing models that are friendly to hardware implementation. The special requirements of structured pruning have led to the discovery of numerous new challenges and the development of innovative solutions. This article surveys the recent progress towards structured pruning of deep CNNs. We summarize and compare the state-of-the-art structured pruning techniques with respect to filter ranking methods, regularization methods, dynamic execution, neural architecture search, the lottery ticket hypothesis, and the applications of pruning. While discussing structured pruning algorithms, we briefly introduce the unstructured pruning counterpart to emphasize their differences. Furthermore, we provide insights into potential research opportunities in the field of structured pruning. A curated list of neural network pruning papers can be found at https://github.com/he-y/Awesome-Pruning},
- urldate = {2023-10-20},
- publisher = {arXiv},
- author = {He, Yang and Xiao, Lingao},
- month = mar,
- year = {2023},
- note = {arXiv:2303.00566 [cs]},
- keywords = {Computer Science - Computer Vision and Pattern Recognition},
- file = {arXiv Fulltext PDF:/Users/jeffreyma/Zotero/storage/K5RGQQA9/He and Xiao - 2023 - Structured Pruning for Deep Convolutional Neural N.pdf:application/pdf;arXiv.org Snapshot:/Users/jeffreyma/Zotero/storage/U7PVPU4C/2303.html:text/html},
+@inproceedings{reddi2020mlperf,
+ title = {Mlperf inference benchmark},
+ author = {Reddi, Vijay Janapa and Cheng, Christine and Kanter, David and Mattson, Peter and Schmuelling, Guenther and Wu, Carole-Jean and Anderson, Brian and Breughe, Maximilien and Charlebois, Mark and Chou, William and others},
+ year = 2020,
+ booktitle = {2020 ACM/IEEE 47th Annual International Symposium on Computer Architecture (ISCA)},
+ pages = {446--459},
+ organization = {IEEE}
}
-@misc{blalock_what_2020,
- title = {What is the {State} of {Neural} {Network} {Pruning}?},
- url = {http://arxiv.org/abs/2003.03033},
- doi = {10.48550/arXiv.2003.03033},
- abstract = {Neural network pruning---the task of reducing the size of a network by removing parameters---has been the subject of a great deal of work in recent years. We provide a meta-analysis of the literature, including an overview of approaches to pruning and consistent findings in the literature. After aggregating results across 81 papers and pruning hundreds of models in controlled conditions, our clearest finding is that the community suffers from a lack of standardized benchmarks and metrics. This deficiency is substantial enough that it is hard to compare pruning techniques to one another or determine how much progress the field has made over the past three decades. To address this situation, we identify issues with current practices, suggest concrete remedies, and introduce ShrinkBench, an open-source framework to facilitate standardized evaluations of pruning methods. We use ShrinkBench to compare various pruning techniques and show that its comprehensive evaluation can prevent common pitfalls when comparing pruning methods.},
- urldate = {2023-10-20},
- publisher = {arXiv},
- author = {Blalock, Davis and Ortiz, Jose Javier Gonzalez and Frankle, Jonathan and Guttag, John},
- month = mar,
- year = {2020},
- note = {arXiv:2003.03033 [cs, stat]},
- keywords = {Computer Science - Machine Learning, Statistics - Machine Learning},
- file = {arXiv Fulltext PDF:/Users/jeffreyma/Zotero/storage/MA4QGZ6E/Blalock et al. - 2020 - What is the State of Neural Network Pruning.pdf:application/pdf;arXiv.org Snapshot:/Users/jeffreyma/Zotero/storage/8DFKG4GL/2003.html:text/html},
+@inproceedings{ribeiro2016should,
+ title = {" Why should i trust you?" Explaining the predictions of any classifier},
+ author = {Ribeiro, Marco Tulio and Singh, Sameer and Guestrin, Carlos},
+ year = 2016,
+ booktitle = {Proceedings of the 22nd ACM SIGKDD international conference on knowledge discovery and data mining},
+ pages = {1135--1144}
}
-@misc{chen__inpainting_2022,
- title = {Inpainting {Fluid} {Dynamics} with {Tensor} {Decomposition} ({NumPy})},
- url = {https://medium.com/@xinyu.chen/inpainting-fluid-dynamics-with-tensor-decomposition-numpy-d84065fead4d},
- abstract = {Some simple examples for showing how to use tensor decomposition to reconstruct fluid dynamics},
- language = {en},
- urldate = {2023-10-20},
- journal = {Medium},
- author = {Chen (陈新宇), Xinyu},
- month = mar,
- year = {2022},
+@book{rosenblatt1957perceptron,
+ title = {The perceptron, a perceiving and recognizing automaton Project Para},
+ author = {Rosenblatt, Frank},
+ year = 1957,
+ publisher = {Cornell Aeronautical Laboratory}
}
-@misc{noauthor_introduction_nodate,
- title = {An {Introduction} to {Separable} {Convolutions} - {Analytics} {Vidhya}},
- url = {https://www.analyticsvidhya.com/blog/2021/11/an-introduction-to-separable-convolutions/},
- urldate = {2023-10-20},
- author = {Hegde, Sumant},
- year = {2023}
+@inproceedings{rouhani2017tinydl,
+ title = {TinyDL: Just-in-time deep learning solution for constrained embedded systems},
+ author = {Rouhani, Bita and Mirhoseini, Azalia and Koushanfar, Farinaz},
+ year = 2017,
+ month = {05},
+ pages = {1--4},
+ doi = {10.1109/ISCAS.2017.8050343}
}
-@misc{iandola_squeezenet_2016,
- title = {{SqueezeNet}: {AlexNet}-level accuracy with 50x fewer parameters and {\textless}0.{5MB} model size},
- shorttitle = {{SqueezeNet}},
- url = {http://arxiv.org/abs/1602.07360},
- doi = {10.48550/arXiv.1602.07360},
- abstract = {Recent research on deep neural networks has focused primarily on improving accuracy. For a given accuracy level, it is typically possible to identify multiple DNN architectures that achieve that accuracy level. With equivalent accuracy, smaller DNN architectures offer at least three advantages: (1) Smaller DNNs require less communication across servers during distributed training. (2) Smaller DNNs require less bandwidth to export a new model from the cloud to an autonomous car. (3) Smaller DNNs are more feasible to deploy on FPGAs and other hardware with limited memory. To provide all of these advantages, we propose a small DNN architecture called SqueezeNet. SqueezeNet achieves AlexNet-level accuracy on ImageNet with 50x fewer parameters. Additionally, with model compression techniques we are able to compress SqueezeNet to less than 0.5MB (510x smaller than AlexNet). The SqueezeNet architecture is available for download here: https://github.com/DeepScale/SqueezeNet},
- urldate = {2023-10-20},
- publisher = {arXiv},
- author = {Iandola, Forrest N. and Han, Song and Moskewicz, Matthew W. and Ashraf, Khalid and Dally, William J. and Keutzer, Kurt},
- month = nov,
- year = {2016},
- note = {arXiv:1602.07360 [cs]},
- keywords = {Computer Science - Artificial Intelligence, Computer Science - Computer Vision and Pattern Recognition},
- file = {arXiv Fulltext PDF:/Users/jeffreyma/Zotero/storage/X3ZX9UTZ/Iandola et al. - 2016 - SqueezeNet AlexNet-level accuracy with 50x fewer .pdf:application/pdf;arXiv.org Snapshot:/Users/jeffreyma/Zotero/storage/DHI96QVT/1602.html:text/html},
+@article{rumelhart1986learning,
+ title = {Learning representations by back-propagating errors},
+ author = {Rumelhart, David E and Hinton, Geoffrey E and Williams, Ronald J},
+ year = 1986,
+ journal = {nature},
+ publisher = {Nature Publishing Group UK London},
+ volume = 323,
+ number = 6088,
+ pages = {533--536}
}
-@misc{howard_mobilenets_2017,
- title = {{MobileNets}: {Efficient} {Convolutional} {Neural} {Networks} for {Mobile} {Vision} {Applications}},
- shorttitle = {{MobileNets}},
- url = {http://arxiv.org/abs/1704.04861},
- doi = {10.48550/arXiv.1704.04861},
- abstract = {We present a class of efficient models called MobileNets for mobile and embedded vision applications. MobileNets are based on a streamlined architecture that uses depth-wise separable convolutions to build light weight deep neural networks. We introduce two simple global hyper-parameters that efficiently trade off between latency and accuracy. These hyper-parameters allow the model builder to choose the right sized model for their application based on the constraints of the problem. We present extensive experiments on resource and accuracy tradeoffs and show strong performance compared to other popular models on ImageNet classification. We then demonstrate the effectiveness of MobileNets across a wide range of applications and use cases including object detection, finegrain classification, face attributes and large scale geo-localization.},
- urldate = {2023-10-20},
- publisher = {arXiv},
- author = {Howard, Andrew G. and Zhu, Menglong and Chen, Bo and Kalenichenko, Dmitry and Wang, Weijun and Weyand, Tobias and Andreetto, Marco and Adam, Hartwig},
- month = apr,
- year = {2017},
- note = {arXiv:1704.04861 [cs]},
- keywords = {Computer Science - Computer Vision and Pattern Recognition},
- file = {arXiv Fulltext PDF:/Users/jeffreyma/Zotero/storage/IJ9P9ID9/Howard et al. - 2017 - MobileNets Efficient Convolutional Neural Network.pdf:application/pdf;arXiv.org Snapshot:/Users/jeffreyma/Zotero/storage/D9TS95GJ/1704.html:text/html},
+@article{ruvolo_ella_nodate,
+ title = {{ELLA}: {An} {Efficient} {Lifelong} {Learning} {Algorithm}},
+ author = {Ruvolo, Paul and Eaton, Eric},
+ language = {en},
+ file = {Ruvolo and Eaton - ELLA An Efficient Lifelong Learning Algorithm.pdf:/Users/alex/Zotero/storage/QA5G29GL/Ruvolo and Eaton - ELLA An Efficient Lifelong Learning Algorithm.pdf:application/pdf}
}
-@misc{tan_efficientnet_2020,
- title = {{EfficientNet}: {Rethinking} {Model} {Scaling} for {Convolutional} {Neural} {Networks}},
- shorttitle = {{EfficientNet}},
- url = {http://arxiv.org/abs/1905.11946},
- doi = {10.48550/arXiv.1905.11946},
- abstract = {Convolutional Neural Networks (ConvNets) are commonly developed at a fixed resource budget, and then scaled up for better accuracy if more resources are available. In this paper, we systematically study model scaling and identify that carefully balancing network depth, width, and resolution can lead to better performance. Based on this observation, we propose a new scaling method that uniformly scales all dimensions of depth/width/resolution using a simple yet highly effective compound coefficient. We demonstrate the effectiveness of this method on scaling up MobileNets and ResNet. To go even further, we use neural architecture search to design a new baseline network and scale it up to obtain a family of models, called EfficientNets, which achieve much better accuracy and efficiency than previous ConvNets. In particular, our EfficientNet-B7 achieves state-of-the-art 84.3\% top-1 accuracy on ImageNet, while being 8.4x smaller and 6.1x faster on inference than the best existing ConvNet. Our EfficientNets also transfer well and achieve state-of-the-art accuracy on CIFAR-100 (91.7\%), Flowers (98.8\%), and 3 other transfer learning datasets, with an order of magnitude fewer parameters. Source code is at https://github.com/tensorflow/tpu/tree/master/models/official/efficientnet.},
- urldate = {2023-10-20},
- publisher = {arXiv},
- author = {Tan, Mingxing and Le, Quoc V.},
- month = sep,
- year = {2020},
- note = {arXiv:1905.11946 [cs, stat]},
- keywords = {Computer Science - Computer Vision and Pattern Recognition, Computer Science - Machine Learning, Statistics - Machine Learning},
- file = {arXiv Fulltext PDF:/Users/jeffreyma/Zotero/storage/KISBF35I/Tan and Le - 2020 - EfficientNet Rethinking Model Scaling for Convolu.pdf:application/pdf;arXiv.org Snapshot:/Users/jeffreyma/Zotero/storage/TUD4PH4M/1905.html:text/html},
+@misc{ScaleAI,
+ journal = {ScaleAI},
+ url = {https://scale.com/data-engine}
}
-@misc{lin_mcunet_2020,
- title = {{MCUNet}: {Tiny} {Deep} {Learning} on {IoT} {Devices}},
- shorttitle = {{MCUNet}},
- url = {http://arxiv.org/abs/2007.10319},
- doi = {10.48550/arXiv.2007.10319},
- abstract = {Machine learning on tiny IoT devices based on microcontroller units (MCU) is appealing but challenging: the memory of microcontrollers is 2-3 orders of magnitude smaller even than mobile phones. We propose MCUNet, a framework that jointly designs the efficient neural architecture (TinyNAS) and the lightweight inference engine (TinyEngine), enabling ImageNet-scale inference on microcontrollers. TinyNAS adopts a two-stage neural architecture search approach that first optimizes the search space to fit the resource constraints, then specializes the network architecture in the optimized search space. TinyNAS can automatically handle diverse constraints (i.e.device, latency, energy, memory) under low search costs.TinyNAS is co-designed with TinyEngine, a memory-efficient inference library to expand the search space and fit a larger model. TinyEngine adapts the memory scheduling according to the overall network topology rather than layer-wise optimization, reducing the memory usage by 4.8x, and accelerating the inference by 1.7-3.3x compared to TF-Lite Micro and CMSIS-NN. MCUNet is the first to achieves {\textgreater}70\% ImageNet top1 accuracy on an off-the-shelf commercial microcontroller, using 3.5x less SRAM and 5.7x less Flash compared to quantized MobileNetV2 and ResNet-18. On visual\&audio wake words tasks, MCUNet achieves state-of-the-art accuracy and runs 2.4-3.4x faster than MobileNetV2 and ProxylessNAS-based solutions with 3.7-4.1x smaller peak SRAM. Our study suggests that the era of always-on tiny machine learning on IoT devices has arrived. Code and models can be found here: https://tinyml.mit.edu.},
- urldate = {2023-10-20},
- publisher = {arXiv},
- author = {Lin, Ji and Chen, Wei-Ming and Lin, Yujun and Cohn, John and Gan, Chuang and Han, Song},
- month = nov,
- year = {2020},
- note = {arXiv:2007.10319 [cs]},
- keywords = {Computer Science - Computer Vision and Pattern Recognition},
- file = {arXiv Fulltext PDF:/Users/jeffreyma/Zotero/storage/IX2JN4P9/Lin et al. - 2020 - MCUNet Tiny Deep Learning on IoT Devices.pdf:application/pdf;arXiv.org Snapshot:/Users/jeffreyma/Zotero/storage/BAKHZ46Y/2007.html:text/html},
+@inproceedings{schwarzschild2021just,
+ title = {Just how toxic is data poisoning? a unified benchmark for backdoor and data poisoning attacks},
+ author = {Schwarzschild, Avi and Goldblum, Micah and Gupta, Arjun and Dickerson, John P and Goldstein, Tom},
+ year = 2021,
+ booktitle = {International Conference on Machine Learning},
+ pages = {9389--9398},
+ organization = {PMLR}
}
-@misc{gordon_morphnet_2018,
- title = {{MorphNet}: {Fast} \& {Simple} {Resource}-{Constrained} {Structure} {Learning} of {Deep} {Networks}},
- shorttitle = {{MorphNet}},
- url = {http://arxiv.org/abs/1711.06798},
- doi = {10.48550/arXiv.1711.06798},
- abstract = {We present MorphNet, an approach to automate the design of neural network structures. MorphNet iteratively shrinks and expands a network, shrinking via a resource-weighted sparsifying regularizer on activations and expanding via a uniform multiplicative factor on all layers. In contrast to previous approaches, our method is scalable to large networks, adaptable to specific resource constraints (e.g. the number of floating-point operations per inference), and capable of increasing the network's performance. When applied to standard network architectures on a wide variety of datasets, our approach discovers novel structures in each domain, obtaining higher performance while respecting the resource constraint.},
- urldate = {2023-10-20},
- publisher = {arXiv},
- author = {Gordon, Ariel and Eban, Elad and Nachum, Ofir and Chen, Bo and Wu, Hao and Yang, Tien-Ju and Choi, Edward},
- month = apr,
- year = {2018},
- note = {arXiv:1711.06798 [cs, stat]},
- keywords = {Computer Science - Machine Learning, Statistics - Machine Learning},
- file = {arXiv Fulltext PDF:/Users/jeffreyma/Zotero/storage/GV7N4CZC/Gordon et al. - 2018 - MorphNet Fast & Simple Resource-Constrained Struc.pdf:application/pdf;arXiv.org Snapshot:/Users/jeffreyma/Zotero/storage/K6FUV82F/1711.html:text/html},
+@misc{see_compression_2016,
+ title = {Compression of {Neural} {Machine} {Translation} {Models} via {Pruning}},
+ author = {See, Abigail and Luong, Minh-Thang and Manning, Christopher D.},
+ year = 2016,
+ month = jun,
+ publisher = {arXiv},
+ doi = {10.48550/arXiv.1606.09274},
+ url = {http://arxiv.org/abs/1606.09274},
+ urldate = {2023-10-20},
+ note = {arXiv:1606.09274 [cs]},
+ abstract = {Neural Machine Translation (NMT), like many other deep learning domains, typically suffers from over-parameterization, resulting in large storage sizes. This paper examines three simple magnitude-based pruning schemes to compress NMT models, namely class-blind, class-uniform, and class-distribution, which differ in terms of how pruning thresholds are computed for the different classes of weights in the NMT architecture. We demonstrate the efficacy of weight pruning as a compression technique for a state-of-the-art NMT system. We show that an NMT model with over 200 million parameters can be pruned by 40\% with very little performance loss as measured on the WMT'14 English-German translation task. This sheds light on the distribution of redundancy in the NMT architecture. Our main result is that with retraining, we can recover and even surpass the original performance with an 80\%-pruned model.},
+ keywords = {Computer Science - Artificial Intelligence, Computer Science - Computation and Language, Computer Science - Neural and Evolutionary Computing},
+ file = {arXiv Fulltext PDF:/Users/jeffreyma/Zotero/storage/2CJ4TSNR/See et al. - 2016 - Compression of Neural Machine Translation Models v.pdf:application/pdf}
}
-@inproceedings{lecun_optimal_1989,
- title = {Optimal {Brain} {Damage}},
- volume = {2},
- url = {https://proceedings.neurips.cc/paper/1989/hash/6c9882bbac1c7093bd25041881277658-Abstract.html},
- abstract = {We have used information-theoretic ideas to derive a class of prac(cid:173) tical and nearly optimal schemes for adapting the size of a neural network. By removing unimportant weights from a network, sev(cid:173) eral improvements can be expected: better generalization, fewer training examples required, and improved speed of learning and/or classification. The basic idea is to use second-derivative informa(cid:173) tion to make a tradeoff between network complexity and training set error. Experiments confirm the usefulness of the methods on a real-world application.},
- urldate = {2023-10-20},
- booktitle = {Advances in {Neural} {Information} {Processing} {Systems}},
- publisher = {Morgan-Kaufmann},
- author = {LeCun, Yann and Denker, John and Solla, Sara},
- year = {1989},
- file = {Full Text PDF:/Users/jeffreyma/Zotero/storage/BYHQQSST/LeCun et al. - 1989 - Optimal Brain Damage.pdf:application/pdf},
+@inproceedings{seide2016cntk,
+ title = {CNTK: Microsoft's open-source deep-learning toolkit},
+ author = {Seide, Frank and Agarwal, Amit},
+ year = 2016,
+ booktitle = {Proceedings of the 22nd ACM SIGKDD international conference on knowledge discovery and data mining},
+ pages = {2135--2135}
+}
+
+@misc{sevilla_compute_2022,
+ title = {Compute {Trends} {Across} {Three} {Eras} of {Machine} {Learning}},
+ author = {Sevilla, Jaime and Heim, Lennart and Ho, Anson and Besiroglu, Tamay and Hobbhahn, Marius and Villalobos, Pablo},
+ year = 2022,
+ month = mar,
+ publisher = {arXiv},
+ url = {http://arxiv.org/abs/2202.05924},
+ urldate = {2023-10-25},
+ note = {arXiv:2202.05924 [cs]},
+ language = {en},
+ keywords = {Computer Science - Machine Learning, Computer Science - Artificial Intelligence, Computer Science - Computers and Society},
+ file = {Sevilla et al. - 2022 - Compute Trends Across Three Eras of Machine Learni.pdf:/Users/alex/Zotero/storage/24N9RZ72/Sevilla et al. - 2022 - Compute Trends Across Three Eras of Machine Learni.pdf:application/pdf}
}
-@article{kolda_tensor_2009,
- title = {Tensor {Decompositions} and {Applications}},
- volume = {51},
- issn = {0036-1445, 1095-7200},
- url = {http://epubs.siam.org/doi/10.1137/07070111X},
- doi = {10.1137/07070111X},
- abstract = {This survey provides an overview of higher-order tensor decompositions, their applications, and available software. A tensor is a multidimensional or N -way array. Decompositions of higher-order tensors (i.e., N -way arrays with N ≥ 3) have applications in psychometrics, chemometrics, signal processing, numerical linear algebra, computer vision, numerical analysis, data mining, neuroscience, graph analysis, and elsewhere. Two particular tensor decompositions can be considered to be higher-order extensions of the matrix singular value decomposition: CANDECOMP/PARAFAC (CP) decomposes a tensor as a sum of rank-one tensors, and the Tucker decomposition is a higher-order form of principal component analysis. There are many other tensor decompositions, including INDSCAL, PARAFAC2, CANDELINC, DEDICOM, and PARATUCK2 as well as nonnegative variants of all of the above. The N-way Toolbox, Tensor Toolbox, and Multilinear Engine are examples of software packages for working with tensors.},
- language = {en},
- number = {3},
- urldate = {2023-10-20},
- journal = {SIAM Review},
- author = {Kolda, Tamara G. and Bader, Brett W.},
- month = aug,
- year = {2009},
- pages = {455--500},
- file = {Kolda and Bader - 2009 - Tensor Decompositions and Applications.pdf:/Users/jeffreyma/Zotero/storage/Q7ZG2267/Kolda and Bader - 2009 - Tensor Decompositions and Applications.pdf:application/pdf},
+@article{seyedzadeh2018machine,
+ title = {Machine learning for estimation of building energy consumption and performance: a review},
+ author = {Seyedzadeh, Saleh and Rahimian, Farzad Pour and Glesk, Ivan and Roper, Marc},
+ year = 2018,
+ journal = {Visualization in Engineering},
+ publisher = {Springer},
+ volume = 6,
+ pages = {1--20}
+}
+
+@article{shamir1979share,
+ title = {How to share a secret},
+ author = {Shamir, Adi},
+ year = 1979,
+ journal = {Communications of the ACM},
+ publisher = {ACm New York, NY, USA},
+ volume = 22,
+ number = 11,
+ pages = {612--613}
+}
+
+@article{Sheng_Zhang_2019,
+ title = {Machine learning with crowdsourcing: A brief summary of the past research and Future Directions},
+ author = {Sheng, Victor S. and Zhang, Jing},
+ year = 2019,
+ journal = {Proceedings of the AAAI Conference on Artificial Intelligence},
+ volume = 33,
+ number = {01},
+ pages = {9837–9843},
+ doi = {10.1609/aaai.v33i01.33019837}
+}
+
+@misc{Sheth_2022,
+ title = {Eletect - TinyML and IOT based Smart Wildlife Tracker},
+ author = {Sheth, Dhruv},
+ year = 2022,
+ month = {Mar},
+ journal = {Hackster.io},
+ url = {https://www.hackster.io/dhruvsheth_/eletect-tinyml-and-iot-based-smart-wildlife-tracker-c03e5a}
+}
+
+@inproceedings{shi2022data,
+ title = {Data selection for efficient model update in federated learning},
+ author = {Shi, Hongrui and Radu, Valentin},
+ year = 2022,
+ booktitle = {Proceedings of the 2nd European Workshop on Machine Learning and Systems},
+ pages = {72--78}
+}
+
+@article{smestad2023systematic,
+ title = {A Systematic Literature Review on Client Selection in Federated Learning},
+ author = {Smestad, Carl and Li, Jingyue},
+ year = 2023,
+ journal = {arXiv preprint arXiv:2306.04862}
}
-@misc{see_compression_2016,
- title = {Compression of {Neural} {Machine} {Translation} {Models} via {Pruning}},
- url = {http://arxiv.org/abs/1606.09274},
- doi = {10.48550/arXiv.1606.09274},
- abstract = {Neural Machine Translation (NMT), like many other deep learning domains, typically suffers from over-parameterization, resulting in large storage sizes. This paper examines three simple magnitude-based pruning schemes to compress NMT models, namely class-blind, class-uniform, and class-distribution, which differ in terms of how pruning thresholds are computed for the different classes of weights in the NMT architecture. We demonstrate the efficacy of weight pruning as a compression technique for a state-of-the-art NMT system. We show that an NMT model with over 200 million parameters can be pruned by 40\% with very little performance loss as measured on the WMT'14 English-German translation task. This sheds light on the distribution of redundancy in the NMT architecture. Our main result is that with retraining, we can recover and even surpass the original performance with an 80\%-pruned model.},
- urldate = {2023-10-20},
- publisher = {arXiv},
- author = {See, Abigail and Luong, Minh-Thang and Manning, Christopher D.},
- month = jun,
- year = {2016},
- note = {arXiv:1606.09274 [cs]},
- keywords = {Computer Science - Artificial Intelligence, Computer Science - Computation and Language, Computer Science - Neural and Evolutionary Computing},
- file = {arXiv Fulltext PDF:/Users/jeffreyma/Zotero/storage/2CJ4TSNR/See et al. - 2016 - Compression of Neural Machine Translation Models v.pdf:application/pdf},
+@misc{smoothquant,
+ title = {SmoothQuant: Accurate and Efficient Post-Training Quantization for Large Language Models},
+ author = {Xiao and Lin, Seznec and Wu, Demouth and Han},
+ year = 2023,
+ doi = {10.48550/arXiv.2211.10438},
+ url = {https://arxiv.org/abs/2211.10438},
+ urldate = {2023-06-05},
+ abstract = {Large language models (LLMs) show excellent performance but are compute- and memory-intensive. Quantization can reduce memory and accelerate inference. However, existing methods cannot maintain accuracy and hardware efficiency at the same time. We propose SmoothQuant, a training-free, accuracy-preserving, and general-purpose post-training quantization (PTQ) solution to enable 8-bit weight, 8-bit activation (W8A8) quantization for LLMs. Based on the fact that weights are easy to quantize while activations are not, SmoothQuant smooths the activation outliers by offline migrating the quantization difficulty from activations to weights with a mathematically equivalent transformation. SmoothQuant enables an INT8 quantization of both weights and activations for all the matrix multiplications in LLMs, including OPT, BLOOM, GLM, MT-NLG, and LLaMA family. We demonstrate up to 1.56x speedup and 2x memory reduction for LLMs with negligible loss in accuracy. SmoothQuant enables serving 530B LLM within a single node. Our work offers a turn-key solution that reduces hardware costs and democratizes LLMs.}
}
-@misc{liao_can_2023,
- title = {Can {Unstructured} {Pruning} {Reduce} the {Depth} in {Deep} {Neural} {Networks}?},
- url = {http://arxiv.org/abs/2308.06619},
- doi = {10.48550/arXiv.2308.06619},
- abstract = {Pruning is a widely used technique for reducing the size of deep neural networks while maintaining their performance. However, such a technique, despite being able to massively compress deep models, is hardly able to remove entire layers from a model (even when structured): is this an addressable task? In this study, we introduce EGP, an innovative Entropy Guided Pruning algorithm aimed at reducing the size of deep neural networks while preserving their performance. The key focus of EGP is to prioritize pruning connections in layers with low entropy, ultimately leading to their complete removal. Through extensive experiments conducted on popular models like ResNet-18 and Swin-T, our findings demonstrate that EGP effectively compresses deep neural networks while maintaining competitive performance levels. Our results not only shed light on the underlying mechanism behind the advantages of unstructured pruning, but also pave the way for further investigations into the intricate relationship between entropy, pruning techniques, and deep learning performance. The EGP algorithm and its insights hold great promise for advancing the field of network compression and optimization. The source code for EGP is released open-source.},
- urldate = {2023-10-20},
- publisher = {arXiv},
- author = {Liao, Zhu and Quétu, Victor and Nguyen, Van-Tam and Tartaglione, Enzo},
- month = aug,
- year = {2023},
- note = {arXiv:2308.06619 [cs]},
- keywords = {Computer Science - Artificial Intelligence, Computer Science - Machine Learning},
- file = {arXiv Fulltext PDF:/Users/jeffreyma/Zotero/storage/V6P3XB5H/Liao et al. - 2023 - Can Unstructured Pruning Reduce the Depth in Deep .pdf:application/pdf;arXiv.org Snapshot:/Users/jeffreyma/Zotero/storage/WSQ4ZUH4/2308.html:text/html},
+@misc{surveyofquant,
+ title = {A Survey of Quantization Methods for Efficient Neural Network Inference)},
+ author = {Gholami and Kim, Dong and Yao, Mahoney and Keutzer},
+ year = 2021,
+ doi = {10.48550/arXiv.2103.13630},
+ url = {https://arxiv.org/abs/2103.13630},
+ urldate = {2021-06-21},
+ abstract = {As soon as abstract mathematical computations were adapted to computation on digital computers, the problem of efficient representation, manipulation, and communication of the numerical values in those computations arose. Strongly related to the problem of numerical representation is the problem of quantization: in what manner should a set of continuous real-valued numbers be distributed over a fixed discrete set of numbers to minimize the number of bits required and also to maximize the accuracy of the attendant computations? This perennial problem of quantization is particularly relevant whenever memory and/or computational resources are severely restricted, and it has come to the forefront in recent years due to the remarkable performance of Neural Network models in computer vision, natural language processing, and related areas. Moving from floating-point representations to low-precision fixed integer values represented in four bits or less holds the potential to reduce the memory footprint and latency by a factor of 16x; and, in fact, reductions of 4x to 8x are often realized in practice in these applications. Thus, it is not surprising that quantization has emerged recently as an important and very active sub-area of research in the efficient implementation of computations associated with Neural Networks. In this article, we survey approaches to the problem of quantizing the numerical values in deep Neural Network computations, covering the advantages/disadvantages of current methods. With this survey and its organization, we hope to have presented a useful snapshot of the current research in quantization for Neural Networks and to have given an intelligent organization to ease the evaluation of future research in this area.}
}
-@article{kiela2021dynabench,
- title={Dynabench: Rethinking benchmarking in NLP},
- author={Kiela, Douwe and Bartolo, Max and Nie, Yixin and Kaushik, Divyansh and Geiger, Atticus and Wu, Zhengxuan and Vidgen, Bertie and Prasad, Grusha and Singh, Amanpreet and Ringshia, Pratik and others},
- journal={arXiv preprint arXiv:2104.14337},
- year={2021}
+@misc{tan_efficientnet_2020,
+ title = {{EfficientNet}: {Rethinking} {Model} {Scaling} for {Convolutional} {Neural} {Networks}},
+ shorttitle = {{EfficientNet}},
+ author = {Tan, Mingxing and Le, Quoc V.},
+ year = 2020,
+ month = sep,
+ publisher = {arXiv},
+ doi = {10.48550/arXiv.1905.11946},
+ url = {http://arxiv.org/abs/1905.11946},
+ urldate = {2023-10-20},
+ note = {arXiv:1905.11946 [cs, stat]},
+ abstract = {Convolutional Neural Networks (ConvNets) are commonly developed at a fixed resource budget, and then scaled up for better accuracy if more resources are available. In this paper, we systematically study model scaling and identify that carefully balancing network depth, width, and resolution can lead to better performance. Based on this observation, we propose a new scaling method that uniformly scales all dimensions of depth/width/resolution using a simple yet highly effective compound coefficient. We demonstrate the effectiveness of this method on scaling up MobileNets and ResNet. To go even further, we use neural architecture search to design a new baseline network and scale it up to obtain a family of models, called EfficientNets, which achieve much better accuracy and efficiency than previous ConvNets. In particular, our EfficientNet-B7 achieves state-of-the-art 84.3\% top-1 accuracy on ImageNet, while being 8.4x smaller and 6.1x faster on inference than the best existing ConvNet. Our EfficientNets also transfer well and achieve state-of-the-art accuracy on CIFAR-100 (91.7\%), Flowers (98.8\%), and 3 other transfer learning datasets, with an order of magnitude fewer parameters. Source code is at https://github.com/tensorflow/tpu/tree/master/models/official/efficientnet.},
+ keywords = {Computer Science - Computer Vision and Pattern Recognition, Computer Science - Machine Learning, Statistics - Machine Learning},
+ file = {arXiv Fulltext PDF:/Users/jeffreyma/Zotero/storage/KISBF35I/Tan and Le - 2020 - EfficientNet Rethinking Model Scaling for Convolu.pdf:application/pdf;arXiv.org Snapshot:/Users/jeffreyma/Zotero/storage/TUD4PH4M/1905.html:text/html}
}
-@article{beyer2020we,
- title={Are we done with imagenet?},
- author={Beyer, Lucas and H{\'e}naff, Olivier J and Kolesnikov, Alexander and Zhai, Xiaohua and Oord, A{\"a}ron van den},
- journal={arXiv preprint arXiv:2006.07159},
- year={2020}
+@inproceedings{tan2019mnasnet,
+ title = {Mnasnet: Platform-aware neural architecture search for mobile},
+ author = {Tan, Mingxing and Chen, Bo and Pang, Ruoming and Vasudevan, Vijay and Sandler, Mark and Howard, Andrew and Le, Quoc V},
+ year = 2019,
+ booktitle = {Proceedings of the IEEE/CVF conference on computer vision and pattern recognition},
+ pages = {2820--2828}
}
-@article{gaviria2022dollar,
- title={The Dollar Street Dataset: Images Representing the Geographic and Socioeconomic Diversity of the World},
- author={Gaviria Rojas, William and Diamos, Sudnya and Kini, Keertan and Kanter, David and Janapa Reddi, Vijay and Coleman, Cody},
- journal={Advances in Neural Information Processing Systems},
- volume={35},
- pages={12979--12990},
- year={2022}
+
+@misc{tan2020efficientnet,
+ title = {EfficientNet: Rethinking Model Scaling for Convolutional Neural Networks},
+ author = {Mingxing Tan and Quoc V. Le},
+ year = 2020,
+ eprint = {1905.11946},
+ archiveprefix = {arXiv},
+ primaryclass = {cs.LG}
}
-@article{xu2023demystifying,
- title={Demystifying CLIP Data},
- author={Xu, Hu and Xie, Saining and Tan, Xiaoqing Ellen and Huang, Po-Yao and Howes, Russell and Sharma, Vasu and Li, Shang-Wen and Ghosh, Gargi and Zettlemoyer, Luke and Feichtenhofer, Christoph},
- journal={arXiv preprint arXiv:2309.16671},
- year={2023}
+
+@misc{Team_2023,
+ title = {Data-centric AI for the Enterprise},
+ author = {Team, Snorkel},
+ year = 2023,
+ month = {Aug},
+ journal = {Snorkel AI},
+ url = {https://snorkel.ai/}
}
-@inproceedings{coleman2022similarity,
- title={Similarity search for efficient active learning and search of rare concepts},
- author={Coleman, Cody and Chou, Edward and Katz-Samuels, Julian and Culatana, Sean and Bailis, Peter and Berg, Alexander C and Nowak, Robert and Sumbaly, Roshan and Zaharia, Matei and Yalniz, I Zeki},
- booktitle={Proceedings of the AAAI Conference on Artificial Intelligence},
- volume={36},
- number={6},
- pages={6402--6410},
- year={2022}
+
+@misc{Thefutur92:online,
+ title = {The future is being built on Arm: Market diversification continues to drive strong royalty and licensing growth as ecosystem reaches quarter of a trillion chips milestone – Arm®},
+ author = {ARM.com},
+ note = {(Accessed on 09/16/2023)},
+ howpublished = {\url{https://www.arm.com/company/news/2023/02/arm-announces-q3-fy22-results}}
}
-@inproceedings{ribeiro2016should,
- title={" Why should i trust you?" Explaining the predictions of any classifier},
- author={Ribeiro, Marco Tulio and Singh, Sameer and Guestrin, Carlos},
- booktitle={Proceedings of the 22nd ACM SIGKDD international conference on knowledge discovery and data mining},
- pages={1135--1144},
- year={2016}
+
+@misc{threefloat,
+ title = {Three Floating Point Formats},
+ author = {Google},
+ year = 2023,
+ url = {https://storage.googleapis.com/gweb-cloudblog-publish/images/Three_floating-point_formats.max-624x261.png},
+ urldate = {2023-10-20}
}
-@article{lundberg2017unified,
- title={A unified approach to interpreting model predictions},
- author={Lundberg, Scott M and Lee, Su-In},
- journal={Advances in neural information processing systems},
- volume={30},
- year={2017}
+
+@article{tirtalistyani2022indonesia,
+ title = {Indonesia rice irrigation system: Time for innovation},
+ author = {Tirtalistyani, Rose and Murtiningrum, Murtiningrum and Kanwar, Rameshwar S},
+ year = 2022,
+ journal = {Sustainability},
+ publisher = {MDPI},
+ volume = 14,
+ number = 19,
+ pages = 12477
}
-@inproceedings{coleman2022similarity,
- title={Similarity search for efficient active learning and search of rare concepts},
- author={Coleman, Cody and Chou, Edward and Katz-Samuels, Julian and Culatana, Sean and Bailis, Peter and Berg, Alexander C and Nowak, Robert and Sumbaly, Roshan and Zaharia, Matei and Yalniz, I Zeki},
- booktitle={Proceedings of the AAAI Conference on Artificial Intelligence},
- volume={36},
- number={6},
- pages={6402--6410},
- year={2022}
+
+@inproceedings{tokui2015chainer,
+ title = {Chainer: a next-generation open source framework for deep learning},
+ author = {Tokui, Seiya and Oono, Kenta and Hido, Shohei and Clayton, Justin},
+ year = 2015,
+ booktitle = {Proceedings of workshop on machine learning systems (LearningSys) in the twenty-ninth annual conference on neural information processing systems (NIPS)},
+ volume = 5,
+ pages = {1--6}
+}
+
+@article{van_de_ven_three_2022,
+ title = {Three types of incremental learning},
+ author = {Van De Ven, Gido M. and Tuytelaars, Tinne and Tolias, Andreas S.},
+ year = 2022,
+ month = dec,
+ journal = {Nature Machine Intelligence},
+ volume = 4,
+ number = 12,
+ pages = {1185--1197},
+ doi = {10.1038/s42256-022-00568-3},
+ issn = {2522-5839},
+ url = {https://www.nature.com/articles/s42256-022-00568-3},
+ urldate = {2023-10-26},
+ language = {en},
+ file = {Van De Ven et al. - 2022 - Three types of incremental learning.pdf:/Users/alex/Zotero/storage/5ZAHXMQN/Van De Ven et al. - 2022 - Three types of incremental learning.pdf:application/pdf}
}
-@misc{threefloat,
- title = {Three Floating Point Formats},
- url = {https://storage.googleapis.com/gweb-cloudblog-publish/images/Three_floating-point_formats.max-624x261.png},
- urldate = {2023-10-20},
- author = {Google},
- year = {2023}
+
+@article{vaswani2017attention,
+ title = {Attention is all you need},
+ author = {Vaswani, Ashish and Shazeer, Noam and Parmar, Niki and Uszkoreit, Jakob and Jones, Llion and Gomez, Aidan N and Kaiser, {\L}ukasz and Polosukhin, Illia},
+ year = 2017,
+ journal = {Advances in neural information processing systems},
+ volume = 30
}
-@misc{energyproblem,
- title = {Computing's energy problem (and what we can do about it)},
- url = {https://ieeexplore.ieee.org/document/6757323},
- urldate = {2014-03-06},
- author = {ISSCC},
- year = {2014}
+
+@misc{Vectorbo78:online,
+ title = {Vector-borne diseases},
+ note = {(Accessed on 10/17/2023)},
+ howpublished = {\url{https://www.who.int/news-room/fact-sheets/detail/vector-borne-diseases}}
}
-@misc{surveyofquant,
- title = {A Survey of Quantization Methods for Efficient Neural Network Inference)},
- url = {https://arxiv.org/abs/2103.13630},
- urldate = {2021-06-21},
- author = {Gholami and Kim, Dong and Yao, Mahoney and Keutzer},
- year = {2021},
- doi = {10.48550/arXiv.2103.13630},
- abstract = {As soon as abstract mathematical computations were adapted to computation on digital computers, the problem of efficient representation, manipulation, and communication of the numerical values in those computations arose. Strongly related to the problem of numerical representation is the problem of quantization: in what manner should a set of continuous real-valued numbers be distributed over a fixed discrete set of numbers to minimize the number of bits required and also to maximize the accuracy of the attendant computations? This perennial problem of quantization is particularly relevant whenever memory and/or computational resources are severely restricted, and it has come to the forefront in recent years due to the remarkable performance of Neural Network models in computer vision, natural language processing, and related areas. Moving from floating-point representations to low-precision fixed integer values represented in four bits or less holds the potential to reduce the memory footprint and latency by a factor of 16x; and, in fact, reductions of 4x to 8x are often realized in practice in these applications. Thus, it is not surprising that quantization has emerged recently as an important and very active sub-area of research in the efficient implementation of computations associated with Neural Networks. In this article, we survey approaches to the problem of quantizing the numerical values in deep Neural Network computations, covering the advantages/disadvantages of current methods. With this survey and its organization, we hope to have presented a useful snapshot of the current research in quantization for Neural Networks and to have given an intelligent organization to ease the evaluation of future research in this area.},
+
+@misc{Verma_2022,
+ title = {Elephant AI},
+ author = {Verma, Team Dual_Boot: Swapnil},
+ year = 2022,
+ month = {Mar},
+ journal = {Hackster.io},
+ url = {https://www.hackster.io/dual_boot/elephant-ai-ba71e9}
}
-@misc{intquantfordeepinf,
- title = {Integer Quantization for Deep Learning Inference: Principles and Empirical Evaluation)},
- url = {https://arxiv.org/abs/2004.09602},
- urldate = {2020-04-20},
- author = {Wu and Judd, Zhang and Isaev, Micikevicius},
- year = {2020},
- doi = {10.48550/arXiv.2004.09602},
- abstract = {Quantization techniques can reduce the size of Deep Neural Networks and improve inference latency and throughput by taking advantage of high throughput integer instructions. In this paper we review the mathematical aspects of quantization parameters and evaluate their choices on a wide range of neural network models for different application domains, including vision, speech, and language. We focus on quantization techniques that are amenable to acceleration by processors with high-throughput integer math pipelines. We also present a workflow for 8-bit quantization that is able to maintain accuracy within 1% of the floating-point baseline on all networks studied, including models that are more difficult to quantize, such as MobileNets and BERT-large.},
+
+@misc{villalobos_machine_2022,
+ title = {Machine {Learning} {Model} {Sizes} and the {Parameter} {Gap}},
+ author = {Villalobos, Pablo and Sevilla, Jaime and Besiroglu, Tamay and Heim, Lennart and Ho, Anson and Hobbhahn, Marius},
+ year = 2022,
+ month = jul,
+ publisher = {arXiv},
+ url = {http://arxiv.org/abs/2207.02852},
+ urldate = {2023-10-25},
+ note = {arXiv:2207.02852 [cs]},
+ language = {en},
+ keywords = {Computer Science - Machine Learning, Computer Science - Artificial Intelligence, Computer Science - Computers and Society, Computer Science - Computation and Language},
+ file = {Villalobos et al. - 2022 - Machine Learning Model Sizes and the Parameter Gap.pdf:/Users/alex/Zotero/storage/WW69A82B/Villalobos et al. - 2022 - Machine Learning Model Sizes and the Parameter Gap.pdf:application/pdf}
}
-@misc{deci,
- title = {The Ultimate Guide to Deep Learning Model Quantization and Quantization-Aware Training},
- url = {https://deci.ai/quantization-and-quantization-aware-training/},
+
+@misc{villalobos_trends_2022,
+ title = {Trends in {Training} {Dataset} {Sizes}},
+ author = {Villalobos, Pablo and Ho, Anson},
+ year = 2022,
+ month = sep,
+ journal = {Epoch AI},
+ url = {https://epochai.org/blog/trends-in-training-dataset-sizes}
}
-@misc{awq,
- title = {AWQ: Activation-aware Weight Quantization for LLM Compression and Acceleration},
- url = {https://arxiv.org/abs/2306.00978},
- urldate = {2023-10-03},
- author = {Lin and Tang, Tang and Yang, Dang and Gan, Han},
- year = {2023},
- doi = {10.48550/arXiv.2306.00978},
- abstract = {Large language models (LLMs) have shown excellent performance on various tasks, but the astronomical model size raises the hardware barrier for serving (memory size) and slows down token generation (memory bandwidth). In this paper, we propose Activation-aware Weight Quantization (AWQ), a hardware-friendly approach for LLM low-bit weight-only quantization. Our method is based on the observation that weights are not equally important: protecting only 1% of salient weights can greatly reduce quantization error. We then propose to search for the optimal perchannel scaling that protects the salient weights by observing the activation, not weights. AWQ does not rely on any backpropagation or reconstruction, so it can well preserve LLMs’ generalization ability on different domains and modalities, without overfitting to the calibration set. AWQ outperforms existing work on various language modeling and domain-specific benchmarks. Thanks to better generalization, it achieves excellent quantization performance for instruction-tuned LMs and, for the first time, multi-modal LMs. Alongside AWQ, we implement an efficient and flexible inference framework tailored for LLMs on the edge, offering more than 3× speedup over the Huggingface FP16 implementation on both desktop and mobile GPUs. It also democratizes the deployment of the 70B Llama-2 model on mobile GPU (NVIDIA Jetson Orin 64GB).},
+
+@misc{VinBrain,
+ journal = {VinBrain},
+ url = {https://vinbrain.net/aiscaler}
}
-@misc{smoothquant,
- title = {SmoothQuant: Accurate and Efficient Post-Training Quantization for Large Language Models},
- url = {https://arxiv.org/abs/2211.10438},
- urldate = {2023-06-05},
- author = {Xiao and Lin, Seznec and Wu, Demouth and Han},
- year = {2023},
- doi = {10.48550/arXiv.2211.10438},
- abstract = {Large language models (LLMs) show excellent performance but are compute- and memory-intensive. Quantization can reduce memory and accelerate inference. However, existing methods cannot maintain accuracy and hardware efficiency at the same time. We propose SmoothQuant, a training-free, accuracy-preserving, and general-purpose post-training quantization (PTQ) solution to enable 8-bit weight, 8-bit activation (W8A8) quantization for LLMs. Based on the fact that weights are easy to quantize while activations are not, SmoothQuant smooths the activation outliers by offline migrating the quantization difficulty from activations to weights with a mathematically equivalent transformation. SmoothQuant enables an INT8 quantization of both weights and activations for all the matrix multiplications in LLMs, including OPT, BLOOM, GLM, MT-NLG, and LLaMA family. We demonstrate up to 1.56x speedup and 2x memory reduction for LLMs with negligible loss in accuracy. SmoothQuant enables serving 530B LLM within a single node. Our work offers a turn-key solution that reduces hardware costs and democratizes LLMs.},
+
+@article{vinuesa2020role,
+ title = {The role of artificial intelligence in achieving the Sustainable Development Goals},
+ author = {Vinuesa, Ricardo and Azizpour, Hossein and Leite, Iolanda and Balaam, Madeline and Dignum, Virginia and Domisch, Sami and Fell{\"a}nder, Anna and Langhans, Simone Daniela and Tegmark, Max and Fuso Nerini, Francesco},
+ year = 2020,
+ journal = {Nature communications},
+ publisher = {Nature Publishing Group},
+ volume = 11,
+ number = 1,
+ pages = {1--10}
}
-@misc{deepcompress,
- title = {Deep Compression: Compressing Deep Neural Networks with Pruning, Trained Quantization and Huffman Coding},
- url = {https://arxiv.org/abs/1510.00149},
- urldate = {2016-02-15},
- author = {Han and Mao and Dally},
- year = {2016},
- doi = {10.48550/arXiv.1510.00149},
- abstract = {Neural networks are both computationally intensive and memory intensive, making them difficult to deploy on embedded systems with limited hardware resources. To address this limitation, we introduce "deep compression", a three stage pipeline: pruning, trained quantization and Huffman coding, that work together to reduce the storage requirement of neural networks by 35x to 49x without affecting their accuracy. Our method first prunes the network by learning only the important connections. Next, we quantize the weights to enforce weight sharing, finally, we apply Huffman coding. After the first two steps we retrain the network to fine tune the remaining connections and the quantized centroids. Pruning, reduces the number of connections by 9x to 13x; Quantization then reduces the number of bits that represent each connection from 32 to 5. On the ImageNet dataset, our method reduced the storage required by AlexNet by 35x, from 240MB to 6.9MB, without loss of accuracy. Our method reduced the size of VGG-16 by 49x from 552MB to 11.3MB, again with no loss of accuracy. This allows fitting the model into on-chip SRAM cache rather than off-chip DRAM memory. Our compression method also facilitates the use of complex neural networks in mobile applications where application size and download bandwidth are constrained. Benchmarked on CPU, GPU and mobile GPU, compressed network has 3x to 4x layerwise speedup and 3x to 7x better energy efficiency.},
+
+@article{warden2018speech,
+ title = {Speech commands: A dataset for limited-vocabulary speech recognition},
+ author = {Warden, Pete},
+ year = 2018,
+ journal = {arXiv preprint arXiv:1804.03209}
}
-@misc{quantdeep,
- title = {Quantizing deep convolutional networks for efficient inference: A whitepaper},
- url = {https://arxiv.org/abs/1806.08342},
- doi = {10.48550/arXiv.1806.08342},
- abstract = {We present an overview of techniques for quantizing convolutional neural networks for inference with integer weights and activations. Per-channel quantization of weights and per-layer quantization of activations to 8-bits of precision post-training produces classification accuracies within 2% of floating point networks for a wide variety of CNN architectures. Model sizes can be reduced by a factor of 4 by quantizing weights to 8-bits, even when 8-bit arithmetic is not supported. This can be achieved with simple, post training quantization of weights.We benchmark latencies of quantized networks on CPUs and DSPs and observe a speedup of 2x-3x for quantized implementations compared to floating point on CPUs. Speedups of up to 10x are observed on specialized processors with fixed point SIMD capabilities, like the Qualcomm QDSPs with HVX. Quantization-aware training can provide further improvements, reducing the gap to floating point to 1% at 8-bit precision. Quantization-aware training also allows for reducing the precision of weights to four bits with accuracy losses ranging from 2% to 10%, with higher accuracy drop for smaller networks.We introduce tools in TensorFlow and TensorFlowLite for quantizing convolutional networks and review best practices for quantization-aware training to obtain high accuracy with quantized weights and activations. We recommend that per-channel quantization of weights and per-layer quantization of activations be the preferred quantization scheme for hardware acceleration and kernel optimization. We also propose that future processors and hardware accelerators for optimized inference support precisions of 4, 8 and 16 bits.},
- urldate = {2018-06-21},
- publisher = {arXiv},
- author = {Krishnamoorthi},
- month = jun,
- year = {2018},
+
+@book{warden2019tinyml,
+ title = {Tinyml: Machine learning with tensorflow lite on arduino and ultra-low-power microcontrollers},
+ author = {Warden, Pete and Situnayake, Daniel},
+ year = 2019,
+ publisher = {O'Reilly Media}
+}
+
+@article{weiss_survey_2016,
+ title = {A survey of transfer learning},
+ author = {Weiss, Karl and Khoshgoftaar, Taghi M. and Wang, DingDing},
+ year = 2016,
+ month = dec,
+ journal = {Journal of Big Data},
+ volume = 3,
+ number = 1,
+ pages = 9,
+ doi = {10.1186/s40537-016-0043-6},
+ issn = {2196-1115},
+ url = {http://journalofbigdata.springeropen.com/articles/10.1186/s40537-016-0043-6},
+ urldate = {2023-10-25},
+ language = {en},
+ file = {Weiss et al. - 2016 - A survey of transfer learning.pdf:/Users/alex/Zotero/storage/3FN2Y6EA/Weiss et al. - 2016 - A survey of transfer learning.pdf:application/pdf}
}
-@inproceedings{ijcai2021p592,
- title = {Hardware-Aware Neural Architecture Search: Survey and Taxonomy},
- author = {Benmeziane, Hadjer and El Maghraoui, Kaoutar and Ouarnoughi, Hamza and Niar, Smail and Wistuba, Martin and Wang, Naigang},
- booktitle = {Proceedings of the Thirtieth International Joint Conference on
- Artificial Intelligence, {IJCAI-21}},
- publisher = {International Joint Conferences on Artificial Intelligence Organization},
- editor = {Zhi-Hua Zhou},
- pages = {4322--4329},
- year = {2021},
- month = {8},
- note = {Survey Track},
- doi = {10.24963/ijcai.2021/592},
- url = {https://doi.org/10.24963/ijcai.2021/592},
-}
-
-@InProceedings{Zhang_2020_CVPR_Workshops,
-author = {Zhang, Li Lyna and Yang, Yuqing and Jiang, Yuhang and Zhu, Wenwu and Liu, Yunxin},
-title = {Fast Hardware-Aware Neural Architecture Search},
-booktitle = {Proceedings of the IEEE/CVF Conference on Computer Vision and Pattern Recognition (CVPR) Workshops},
-month = {June},
-year = {2020}
+
+@inproceedings{wu2019fbnet,
+ title = {Fbnet: Hardware-aware efficient convnet design via differentiable neural architecture search},
+ author = {Wu, Bichen and Dai, Xiaoliang and Zhang, Peizhao and Wang, Yanghan and Sun, Fei and Wu, Yiming and Tian, Yuandong and Vajda, Peter and Jia, Yangqing and Keutzer, Kurt},
+ year = 2019,
+ booktitle = {Proceedings of the IEEE/CVF conference on computer vision and pattern recognition},
+ pages = {10734--10742}
}
-@misc{jiang2019accuracy,
- title={Accuracy vs. Efficiency: Achieving Both through FPGA-Implementation Aware Neural Architecture Search},
- author={Weiwen Jiang and Xinyi Zhang and Edwin H. -M. Sha and Lei Yang and Qingfeng Zhuge and Yiyu Shi and Jingtong Hu},
- year={2019},
- eprint={1901.11211},
- archivePrefix={arXiv},
- primaryClass={cs.DC}
+@article{wu2022sustainable,
+ title = {Sustainable ai: Environmental implications, challenges and opportunities},
+ author = {Wu, Carole-Jean and Raghavendra, Ramya and Gupta, Udit and Acun, Bilge and Ardalani, Newsha and Maeng, Kiwan and Chang, Gloria and Aga, Fiona and Huang, Jinshi and Bai, Charles and others},
+ year = 2022,
+ journal = {Proceedings of Machine Learning and Systems},
+ volume = 4,
+ pages = {795--813}
}
-@misc{yang2020coexploration,
- title={Co-Exploration of Neural Architectures and Heterogeneous ASIC Accelerator Designs Targeting Multiple Tasks},
- author={Lei Yang and Zheyu Yan and Meng Li and Hyoukjun Kwon and Liangzhen Lai and Tushar Krishna and Vikas Chandra and Weiwen Jiang and Yiyu Shi},
- year={2020},
- eprint={2002.04116},
- archivePrefix={arXiv},
- primaryClass={cs.LG}
+@inproceedings{xie2020adversarial,
+ title = {Adversarial examples improve image recognition},
+ author = {Xie, Cihang and Tan, Mingxing and Gong, Boqing and Wang, Jiang and Yuille, Alan L and Le, Quoc V},
+ year = 2020,
+ booktitle = {Proceedings of the IEEE/CVF conference on computer vision and pattern recognition},
+ pages = {819--828}
}
-@misc{chu2021discovering,
- title={Discovering Multi-Hardware Mobile Models via Architecture Search},
- author={Grace Chu and Okan Arikan and Gabriel Bender and Weijun Wang and Achille Brighton and Pieter-Jan Kindermans and Hanxiao Liu and Berkin Akin and Suyog Gupta and Andrew Howard},
- year={2021},
- eprint={2008.08178},
- archivePrefix={arXiv},
- primaryClass={cs.CV}
+@article{xu2018alternating,
+ title = {Alternating multi-bit quantization for recurrent neural networks},
+ author = {Xu, Chen and Yao, Jianqiang and Lin, Zhouchen and Ou, Wenwu and Cao, Yuanbin and Wang, Zhirong and Zha, Hongbin},
+ year = 2018,
+ journal = {arXiv preprint arXiv:1802.00150}
}
-@misc{lin2020mcunet,
- title={MCUNet: Tiny Deep Learning on IoT Devices},
- author={Ji Lin and Wei-Ming Chen and Yujun Lin and John Cohn and Chuang Gan and Song Han},
- year={2020},
- eprint={2007.10319},
- archivePrefix={arXiv},
- primaryClass={cs.CV}
+@article{xu2023demystifying,
+ title = {Demystifying CLIP Data},
+ author = {Xu, Hu and Xie, Saining and Tan, Xiaoqing Ellen and Huang, Po-Yao and Howes, Russell and Sharma, Vasu and Li, Shang-Wen and Ghosh, Gargi and Zettlemoyer, Luke and Feichtenhofer, Christoph},
+ year = 2023,
+ journal = {arXiv preprint arXiv:2309.16671}
}
-@misc{zhang2019autoshrink,
- title={AutoShrink: A Topology-aware NAS for Discovering Efficient Neural Architecture},
- author={Tunhou Zhang and Hsin-Pai Cheng and Zhenwen Li and Feng Yan and Chengyu Huang and Hai Li and Yiran Chen},
- year={2019},
- eprint={1911.09251},
- archivePrefix={arXiv},
- primaryClass={cs.LG}
+@article{xu2023federated,
+ title = {Federated Learning of Gboard Language Models with Differential Privacy},
+ author = {Xu, Zheng and Zhang, Yanxiang and Andrew, Galen and Choquette-Choo, Christopher A and Kairouz, Peter and McMahan, H Brendan and Rosenstock, Jesse and Zhang, Yuanbo},
+ year = 2023,
+ journal = {arXiv preprint arXiv:2305.18465}
}
-@misc{lai2018cmsisnn,
- title={CMSIS-NN: Efficient Neural Network Kernels for Arm Cortex-M CPUs},
- author={Liangzhen Lai and Naveen Suda and Vikas Chandra},
- year={2018},
- eprint={1801.06601},
- archivePrefix={arXiv},
- primaryClass={cs.NE}
+@article{yamashita2023coffee,
+ title = {Coffee disease classification at the edge using deep learning},
+ author = {Yamashita, Jo{\~a}o Vitor Yukio Bordin and Leite, Jo{\~a}o Paulo RR},
+ year = 2023,
+ journal = {Smart Agricultural Technology},
+ publisher = {Elsevier},
+ volume = 4,
+ pages = 100183
}
-@misc{zhou2021analognets,
- title={AnalogNets: ML-HW Co-Design of Noise-robust TinyML Models and Always-On Analog Compute-in-Memory Accelerator},
- author={Chuteng Zhou and Fernando Garcia Redondo and Julian Büchel and Irem Boybat and Xavier Timoneda Comas and S. R. Nandakumar and Shidhartha Das and Abu Sebastian and Manuel Le Gallo and Paul N. Whatmough},
- year={2021},
- eprint={2111.06503},
- archivePrefix={arXiv},
- primaryClass={cs.AR}
+@misc{yang2020coexploration,
+ title = {Co-Exploration of Neural Architectures and Heterogeneous ASIC Accelerator Designs Targeting Multiple Tasks},
+ author = {Lei Yang and Zheyu Yan and Meng Li and Hyoukjun Kwon and Liangzhen Lai and Tushar Krishna and Vikas Chandra and Weiwen Jiang and Yiyu Shi},
+ year = 2020,
+ eprint = {2002.04116},
+ archiveprefix = {arXiv},
+ primaryclass = {cs.LG}
}
-@misc{krishna2023raman,
- title={RAMAN: A Re-configurable and Sparse tinyML Accelerator for Inference on Edge},
- author={Adithya Krishna and Srikanth Rohit Nudurupati and Chandana D G and Pritesh Dwivedi and André van Schaik and Mahesh Mehendale and Chetan Singh Thakur},
- year={2023},
- eprint={2306.06493},
- archivePrefix={arXiv},
- primaryClass={cs.NE}
+@inproceedings{yang2023online,
+ title = {Online Model Compression for Federated Learning with Large Models},
+ author = {Yang, Tien-Ju and Xiao, Yonghui and Motta, Giovanni and Beaufays, Fran{\c{c}}oise and Mathews, Rajiv and Chen, Mingqing},
+ year = 2023,
+ booktitle = {ICASSP 2023-2023 IEEE International Conference on Acoustics, Speech and Signal Processing (ICASSP)},
+ pages = {1--5},
+ organization = {IEEE}
}
-@misc{kung2018packing,
- title={Packing Sparse Convolutional Neural Networks for Efficient Systolic Array Implementations: Column Combining Under Joint Optimization},
- author={H. T. Kung and Bradley McDanel and Sai Qian Zhang},
- year={2018},
- eprint={1811.04770},
- archivePrefix={arXiv},
- primaryClass={cs.LG}
+@inproceedings{zennaro2022tinyml,
+ title = {TinyML: applied AI for development},
+ author = {Zennaro, Marco and Plancher, Brian and Reddi, V Janapa},
+ year = 2022,
+ booktitle = {The UN 7th Multi-stakeholder Forum on Science, Technology and Innovation for the Sustainable Development Goals},
+ pages = {2022--05}
}
-@misc{fahim2021hls4ml,
- title={hls4ml: An Open-Source Codesign Workflow to Empower Scientific Low-Power Machine Learning Devices},
- author={Farah Fahim and Benjamin Hawks and Christian Herwig and James Hirschauer and Sergo Jindariani and Nhan Tran and Luca P. Carloni and Giuseppe Di Guglielmo and Philip Harris and Jeffrey Krupa and Dylan Rankin and Manuel Blanco Valentin and Josiah Hester and Yingyi Luo and John Mamish and Seda Orgrenci-Memik and Thea Aarrestad and Hamza Javed and Vladimir Loncar and Maurizio Pierini and Adrian Alan Pol and Sioni Summers and Javier Duarte and Scott Hauck and Shih-Chieh Hsu and Jennifer Ngadiuba and Mia Liu and Duc Hoang and Edward Kreinar and Zhenbin Wu},
- year={2021},
- eprint={2103.05579},
- archivePrefix={arXiv},
- primaryClass={cs.LG}
+@article{zennarobridging,
+ title = {Bridging the Digital Divide: the Promising Impact of TinyML for Developing Countries},
+ author = {Zennaro, Marco and Plancher, Brian and Reddi, Vijay Janapa}
}
-@inproceedings{Prakash_2023,
- doi = {10.1109/ispass57527.2023.00024},
-
- url = {https://doi.org/10.1109%2Fispass57527.2023.00024},
-
- year = 2023,
- month = {apr},
-
- publisher = {{IEEE}
-},
-
- author = {Shvetank Prakash and Tim Callahan and Joseph Bushagour and Colby Banbury and Alan V. Green and Pete Warden and Tim Ansell and Vijay Janapa Reddi},
-
- title = {{CFU} Playground: Full-Stack Open-Source Framework for Tiny Machine Learning ({TinyML}) Acceleration on {FPGAs}},
-
- booktitle = {2023 {IEEE} International Symposium on Performance Analysis of Systems and Software ({ISPASS})}
-}
-
-
-@Article{app112211073,
-AUTHOR = {Kwon, Jisu and Park, Daejin},
-TITLE = {Hardware/Software Co-Design for TinyML Voice-Recognition Application on Resource Frugal Edge Devices},
-JOURNAL = {Applied Sciences},
-VOLUME = {11},
-YEAR = {2021},
-NUMBER = {22},
-ARTICLE-NUMBER = {11073},
-URL = {https://www.mdpi.com/2076-3417/11/22/11073},
-ISSN = {2076-3417},
-ABSTRACT = {On-device artificial intelligence has attracted attention globally, and attempts to combine the internet of things and TinyML (machine learning) applications are increasing. Although most edge devices have limited resources, time and energy costs are important when running TinyML applications. In this paper, we propose a structure in which the part that preprocesses externally input data in the TinyML application is distributed to the hardware. These processes are performed using software in the microcontroller unit of an edge device. Furthermore, resistor–transistor logic, which perform not only windowing using the Hann function, but also acquire audio raw data, is added to the inter-integrated circuit sound module that collects audio data in the voice-recognition application. As a result of the experiment, the windowing function was excluded from the TinyML application of the embedded board. When the length of the hardware-implemented Hann window is 80 and the quantization degree is 2−5, the exclusion causes a decrease in the execution time of the front-end function and energy consumption by 8.06% and 3.27%, respectively.},
-DOI = {10.3390/app112211073}
+@inproceedings{Zhang_2020_CVPR_Workshops,
+ title = {Fast Hardware-Aware Neural Architecture Search},
+ author = {Zhang, Li Lyna and Yang, Yuqing and Jiang, Yuhang and Zhu, Wenwu and Liu, Yunxin},
+ year = 2020,
+ month = {June},
+ booktitle = {Proceedings of the IEEE/CVF Conference on Computer Vision and Pattern Recognition (CVPR) Workshops}
}
-@misc{dong2022splitnets,
- title={SplitNets: Designing Neural Architectures for Efficient Distributed Computing on Head-Mounted Systems},
- author={Xin Dong and Barbara De Salvo and Meng Li and Chiao Liu and Zhongnan Qu and H. T. Kung and Ziyun Li},
- year={2022},
- eprint={2204.04705},
- archivePrefix={arXiv},
- primaryClass={cs.LG}
+@misc{zhang2019autoshrink,
+ title = {AutoShrink: A Topology-aware NAS for Discovering Efficient Neural Architecture},
+ author = {Tunhou Zhang and Hsin-Pai Cheng and Zhenwen Li and Feng Yan and Chengyu Huang and Hai Li and Yiran Chen},
+ year = 2019,
+ eprint = {1911.09251},
+ archiveprefix = {arXiv},
+ primaryclass = {cs.LG}
+}
+
+@article{zhao2018federated,
+ title = {Federated learning with non-iid data},
+ author = {Zhao, Yue and Li, Meng and Lai, Liangzhen and Suda, Naveen and Civin, Damon and Chandra, Vikas},
+ year = 2018,
+ journal = {arXiv preprint arXiv:1806.00582}
+}
+
+@misc{zhou_deep_2023,
+ title = {Deep {Class}-{Incremental} {Learning}: {A} {Survey}},
+ shorttitle = {Deep {Class}-{Incremental} {Learning}},
+ author = {Zhou, Da-Wei and Wang, Qi-Wei and Qi, Zhi-Hong and Ye, Han-Jia and Zhan, De-Chuan and Liu, Ziwei},
+ year = 2023,
+ month = feb,
+ publisher = {arXiv},
+ url = {http://arxiv.org/abs/2302.03648},
+ urldate = {2023-10-26},
+ note = {arXiv:2302.03648 [cs]},
+ language = {en},
+ keywords = {Computer Science - Computer Vision and Pattern Recognition, Computer Science - Machine Learning},
+ annote = {Comment: Code is available at https://github.com/zhoudw-zdw/CIL\_Survey/},
+ file = {Zhou et al. - 2023 - Deep Class-Incremental Learning A Survey.pdf:/Users/alex/Zotero/storage/859VZG7W/Zhou et al. - 2023 - Deep Class-Incremental Learning A Survey.pdf:application/pdf}
}
@misc{kuzmin2022fp8,
@@ -2260,7 +2918,7 @@ @ARTICLE{Sze2017-ak
}
@inproceedings{ignatov2018ai,
- title={Ai benchmark: Running deep neural networks on android smartphones},
+title={Ai benchmark: Running deep neural networks on android smartphones},
author={Ignatov, Andrey and Timofte, Radu and Chou, William and Wang, Ke and Wu, Max and Hartley, Tim and Van Gool, Luc},
booktitle={Proceedings of the European Conference on Computer Vision (ECCV) Workshops},
pages={0--0},
@@ -2345,4 +3003,41 @@ @inproceedings{Jouppi2023TPUv4
keywords = {warehouse scale computer, embeddings, supercomputer, domain specific architecture, reconfigurable, TPU, large language model, power usage effectiveness, CO2 equivalent emissions, energy, optical interconnect, IPU, machine learning, GPU, carbon emissions},
location = {Orlando, FL, USA},
series = {ISCA '23}
-}
\ No newline at end of file
+}
+
+@misc{zhou2021analognets,
+ title = {AnalogNets: ML-HW Co-Design of Noise-robust TinyML Models and Always-On Analog Compute-in-Memory Accelerator},
+ author = {Chuteng Zhou and Fernando Garcia Redondo and Julian Büchel and Irem Boybat and Xavier Timoneda Comas and S. R. Nandakumar and Shidhartha Das and Abu Sebastian and Manuel Le Gallo and Paul N. Whatmough},
+ year = 2021,
+ eprint = {2111.06503},
+ archiveprefix = {arXiv},
+ primaryclass = {cs.AR}
+}
+
+@article{zhuang_comprehensive_2021,
+ title = {A {Comprehensive} {Survey} on {Transfer} {Learning}},
+ author = {Zhuang, Fuzhen and Qi, Zhiyuan and Duan, Keyu and Xi, Dongbo and Zhu, Yongchun and Zhu, Hengshu and Xiong, Hui and He, Qing},
+ year = 2021,
+ month = jan,
+ journal = {Proceedings of the IEEE},
+ volume = 109,
+ number = 1,
+ pages = {43--76},
+ doi = {10.1109/JPROC.2020.3004555},
+ issn = {0018-9219, 1558-2256},
+ url = {https://ieeexplore.ieee.org/document/9134370/},
+ urldate = {2023-10-25},
+ language = {en},
+ file = {Zhuang et al. - 2021 - A Comprehensive Survey on Transfer Learning.pdf:/Users/alex/Zotero/storage/CHJB2WE4/Zhuang et al. - 2021 - A Comprehensive Survey on Transfer Learning.pdf:application/pdf}
+}
+
+@article{zhuang2020comprehensive,
+ title = {A comprehensive survey on transfer learning},
+ author = {Zhuang, Fuzhen and Qi, Zhiyuan and Duan, Keyu and Xi, Dongbo and Zhu, Yongchun and Zhu, Hengshu and Xiong, Hui and He, Qing},
+ year = 2020,
+ journal = {Proceedings of the IEEE},
+ publisher = {IEEE},
+ volume = 109,
+ number = 1,
+ pages = {43--76}
+}
diff --git a/workflow.qmd b/workflow.qmd
index 1031b77a..36a18c77 100644
--- a/workflow.qmd
+++ b/workflow.qmd
@@ -1,6 +1,6 @@
# AI Workflow
-
+
In this chapter, we'll explore the machine learning (ML) workflow, setting the stage for subsequent chapters that delve into the specifics. To ensure we don't lose sight of the bigger picture, this chapter offers a high-level overview of the steps involved in the ML workflow.