Add all 2024 conference sessions to your calendar. You can add this address to your online calendaring system if you want to receive updates dynamically.
2024-10-18T12:30:00Z – 2024-10-18T13:45:00Z
VIS Full Papers
Look, Learn, Language Models
2024-10-18T12:30:00Z – 2024-10-18T13:45:00Z
Chair: Nicole Sultanum
6 presentations in this session. See more »
VIS Full Papers
Where the Networks Are
2024-10-18T12:30:00Z – 2024-10-18T13:45:00Z
Chair: Oliver Deussen
6 presentations in this session. See more »
VIS Full Papers
Human and Machine Visualization Literacy
2024-10-18T12:30:00Z – 2024-10-18T13:45:00Z
Chair: Bum Chul Kwon
6 presentations in this session. See more »
VIS Full Papers
Flow, Topology, and Uncertainty
2024-10-18T12:30:00Z – 2024-10-18T13:45:00Z
Chair: Bei Wang
6 presentations in this session. See more »
2024-10-18T14:15:00Z – 2024-10-18T15:00:00Z
Conference Events
Test of Time Awards
2024-10-18T14:15:00Z – 2024-10-18T15:00:00Z
Chair: Ross Maciejewski
1 presentations in this session. See more »
2024-10-18T15:00:00Z – 2024-10-18T16:30:00Z
Conference Events
IEEE VIS Capstone and Closing
2024-10-18T15:00:00Z – 2024-10-18T16:30:00Z
Chair: Paul Rosen, Kristi Potter, Remco Chang
3 presentations in this session. See more »
2024-10-15T12:30:00Z – 2024-10-15T13:45:00Z
Conference Events
Opening Session
2024-10-15T12:30:00Z – 2024-10-15T13:45:00Z
Chair: Paul Rosen, Kristi Potter, Remco Chang
2 presentations in this session. See more »
2024-10-15T14:15:00Z – 2024-10-15T15:45:00Z
VIS Short Papers
VGTC Awards & Best Short Papers
2024-10-15T14:15:00Z – 2024-10-15T15:45:00Z
Chair: Charles Perin, Johanna Beyer, Takayuki Itoh, Hongfen Yu, Chaoli Wang
4 presentations in this session. See more »
2024-10-15T15:35:00Z – 2024-10-15T16:05:00Z
Conference Events
VIS Governance
2024-10-15T15:35:00Z – 2024-10-15T16:05:00Z
Chair: Petra Isenberg, Jean-Daniel Fekete
2 presentations in this session. See more »
2024-10-15T16:00:00Z – 2024-10-15T17:30:00Z
VIS Full Papers
Best Full Papers
2024-10-15T16:00:00Z – 2024-10-15T17:30:00Z
Chair: Claudio Silva
6 presentations in this session. See more »
2024-10-15T18:00:00Z – 2024-10-15T19:00:00Z
VIS Arts Program
VISAP Keynote: The Golden Age of Visualization Dissensus
2024-10-15T18:00:00Z – 2024-10-15T19:00:00Z
Chair: Pedro Cruz, Rewa Wright, Rebecca Ruige Xu, Lori Jacques, Santiago Echeverry, Kate Terrado, Todd Linkner, Alberto Cairo
0 presentations in this session. See more »
2024-10-15T19:00:00Z – 2024-10-15T21:00:00Z
Conference Events
Posters
2024-10-15T19:00:00Z – 2024-10-15T21:00:00Z
0 presentations in this session. See more »
VIS Arts Program
VISAP Artist Talks
2024-10-15T19:00:00Z – 2024-10-15T21:00:00Z
Chair: Pedro Cruz, Rewa Wright, Rebecca Ruige Xu, Lori Jacques, Santiago Echeverry, Kate Terrado, Todd Linkner
16 presentations in this session. See more »
2024-10-17T12:30:00Z – 2024-10-17T13:45:00Z
VIS Full Papers
Visualization Recommendation
2024-10-17T12:30:00Z – 2024-10-17T13:45:00Z
Chair: Johannes Knittel
6 presentations in this session. See more »
VIS Full Papers
Model-checking and Validation
2024-10-17T12:30:00Z – 2024-10-17T13:45:00Z
Chair: Michael Correll
6 presentations in this session. See more »
VIS Full Papers
Embeddings and Document Spatialization
2024-10-17T12:30:00Z – 2024-10-17T13:45:00Z
Chair: Alex Endert
6 presentations in this session. See more »
VIS Short Papers
Short Papers: Perception and Representation
2024-10-17T12:30:00Z – 2024-10-17T13:45:00Z
Chair: Anjana Arunkumar
8 presentations in this session. See more »
VIS Panels
Panel: Human-Centered Computing Research in South America: Status Quo, Opportunities, and Challenges
2024-10-17T12:30:00Z – 2024-10-17T13:45:00Z
Chair: Chaoli Wang
0 presentations in this session. See more »
2024-10-17T14:15:00Z – 2024-10-17T15:30:00Z
VIS Full Papers
Applications: Sports. Games, and Finance
2024-10-17T14:15:00Z – 2024-10-17T15:30:00Z
Chair: Marc Streit
6 presentations in this session. See more »
VIS Full Papers
Visual Design: Sketching and Labeling
2024-10-17T14:15:00Z – 2024-10-17T15:30:00Z
Chair: Jonathan C. Roberts
6 presentations in this session. See more »
VIS Full Papers
Topological Data Analysis
2024-10-17T14:15:00Z – 2024-10-17T15:30:00Z
Chair: Ingrid Hotz
6 presentations in this session. See more »
VIS Short Papers
Short Papers: Text and Multimedia
2024-10-17T14:15:00Z – 2024-10-17T15:30:00Z
Chair: Min Lu
8 presentations in this session. See more »
VIS Panels
Panel: (Yet Another) Evaluation Needed? A Panel Discussion on Evaluation Trends in Visualization
2024-10-17T14:15:00Z – 2024-10-17T15:30:00Z
Chair: Ghulam Jilani Quadri, Danielle Albers Szafir, Arran Zeyu Wang, Hyeon Jeon
0 presentations in this session. See more »
VIS Arts Program
VISAP Pictorials
2024-10-17T14:15:00Z – 2024-10-17T15:30:00Z
Chair: Pedro Cruz, Rewa Wright, Rebecca Ruige Xu, Lori Jacques, Santiago Echeverry, Kate Terrado, Todd Linkner
8 presentations in this session. See more »
2024-10-17T15:30:00Z – 2024-10-17T16:00:00Z
Conference Events
IEEE VIS 2025 Kickoff
2024-10-17T15:30:00Z – 2024-10-17T16:00:00Z
Chair: Johanna Schmidt, Kresimir Matković, Barbora Kozlíková, Eduard Gröller
1 presentations in this session. See more »
2024-10-17T16:00:00Z – 2024-10-17T17:15:00Z
VIS Full Papers
Once Upon a Visualization
2024-10-17T16:00:00Z – 2024-10-17T17:15:00Z
Chair: Marti Hearst
6 presentations in this session. See more »
VIS Full Papers
Visualization Design Methods
2024-10-17T16:00:00Z – 2024-10-17T17:15:00Z
Chair: Miriah Meyer
6 presentations in this session. See more »
VIS Full Papers
The Toolboxes of Visualization
2024-10-17T16:00:00Z – 2024-10-17T17:15:00Z
Chair: Dominik Moritz
6 presentations in this session. See more »
VIS Short Papers
Short Papers: Analytics and Applications
2024-10-17T16:00:00Z – 2024-10-17T17:15:00Z
Chair: Anna Vilanova
8 presentations in this session. See more »
CG&A Invited Partnership Presentations
CG&A: Systems, Theory, and Evaluations
2024-10-17T16:00:00Z – 2024-10-17T17:15:00Z
Chair: Francesca Samsel
6 presentations in this session. See more »
VIS Panels
Panel: Vogue or Visionary? Current Challenges and Future Opportunities in Situated Visualizations
2024-10-17T16:00:00Z – 2024-10-17T17:15:00Z
Chair: Michelle A. Borkin, Melanie Tory
0 presentations in this session. See more »
2024-10-17T17:45:00Z – 2024-10-17T19:00:00Z
VIS Full Papers
Journalism and Public Policy
2024-10-17T17:45:00Z – 2024-10-17T19:00:00Z
Chair: Sungahn Ko
6 presentations in this session. See more »
VIS Full Papers
Applications: Industry, Computing, and Medicine
2024-10-17T17:45:00Z – 2024-10-17T19:00:00Z
Chair: Joern Kohlhammer
6 presentations in this session. See more »
VIS Full Papers
Accessibility and Touch
2024-10-17T17:45:00Z – 2024-10-17T19:00:00Z
Chair: Narges Mahyar
6 presentations in this session. See more »
VIS Full Papers
Motion and Animated Notions
2024-10-17T17:45:00Z – 2024-10-17T19:00:00Z
Chair: Catherine d'Ignazio
6 presentations in this session. See more »
VIS Short Papers
Short Papers: AI and LLM
2024-10-17T17:45:00Z – 2024-10-17T19:00:00Z
Chair: Cindy Xiong Bearfield
8 presentations in this session. See more »
VIS Panels
Panel: Dear Younger Me: A Dialog About Professional Development Beyond The Initial Career Phases
2024-10-17T17:45:00Z – 2024-10-17T19:00:00Z
Chair: Robert M Kirby, Michael Gleicher
0 presentations in this session. See more »
2024-10-16T12:30:00Z – 2024-10-16T13:30:00Z
VIS Full Papers
Virtual: VIS from around the world
2024-10-16T12:30:00Z – 2024-10-16T13:30:00Z
Chair: Mahmood Jasim
6 presentations in this session. See more »
2024-10-16T12:30:00Z – 2024-10-16T13:45:00Z
VIS Full Papers
Text, Annotation, and Metaphor
2024-10-16T12:30:00Z – 2024-10-16T13:45:00Z
Chair: Melanie Tory
6 presentations in this session. See more »
VIS Full Papers
Immersive Visualization and Visual Analytics
2024-10-16T12:30:00Z – 2024-10-16T13:45:00Z
Chair: Lingyun Yu
6 presentations in this session. See more »
VIS Full Papers
Machine Learning for Visualization
2024-10-16T12:30:00Z – 2024-10-16T13:45:00Z
Chair: Joshua Levine
6 presentations in this session. See more »
VIS Short Papers
Short Papers: Graph, Hierarchy and Multidimensional
2024-10-16T12:30:00Z – 2024-10-16T13:45:00Z
Chair: Alfie Abdul-Rahman
8 presentations in this session. See more »
VIS Panels
Panel: What Do Visualization Art Projects Bring to the VIS Community?
2024-10-16T12:30:00Z – 2024-10-16T13:45:00Z
Chair: Xinhuan Shu, Yifang Wang, Junxiu Tang
0 presentations in this session. See more »
2024-10-16T14:15:00Z – 2024-10-16T15:30:00Z
VIS Full Papers
Biological Data Visualization
2024-10-16T14:15:00Z – 2024-10-16T15:30:00Z
Chair: Nils Gehlenborg
6 presentations in this session. See more »
VIS Full Papers
Judgment and Decision-making
2024-10-16T14:15:00Z – 2024-10-16T15:30:00Z
Chair: Wenwen Dou
6 presentations in this session. See more »
VIS Full Papers
Time and Sequences
2024-10-16T14:15:00Z – 2024-10-16T15:30:00Z
Chair: Silvia Miksch
6 presentations in this session. See more »
VIS Full Papers
Dimensionality Reduction
2024-10-16T14:15:00Z – 2024-10-16T15:30:00Z
Chair: Jian Zhao
6 presentations in this session. See more »
VIS Full Papers
Urban Planning, Construction, and Disaster Management
2024-10-16T14:15:00Z – 2024-10-16T15:30:00Z
Chair: Siming Chen
6 presentations in this session. See more »
VIS Arts Program
VISAP Papers
2024-10-16T14:15:00Z – 2024-10-16T15:30:00Z
Chair: Pedro Cruz, Rewa Wright, Rebecca Ruige Xu, Lori Jacques, Santiago Echeverry, Kate Terrado, Todd Linkner
6 presentations in this session. See more »
2024-10-16T16:00:00Z – 2024-10-16T17:15:00Z
VIS Full Papers
Natural Language and Multimodal Interaction
2024-10-16T16:00:00Z – 2024-10-16T17:15:00Z
Chair: Ana Crisan
6 presentations in this session. See more »
VIS Full Papers
Collaboration and Communication
2024-10-16T16:00:00Z – 2024-10-16T17:15:00Z
Chair: Vidya Setlur
6 presentations in this session. See more »
VIS Full Papers
Perception and Cognition
2024-10-16T16:00:00Z – 2024-10-16T17:15:00Z
Chair: Tamara Munzner
6 presentations in this session. See more »
VIS Short Papers
Short Papers: Scientific and Immersive Visualization
2024-10-16T16:00:00Z – 2024-10-16T17:15:00Z
Chair: Bei Wang
8 presentations in this session. See more »
CG&A Invited Partnership Presentations
CG&A: Analytics and Applications
2024-10-16T16:00:00Z – 2024-10-16T17:15:00Z
Chair: Bruce Campbell
6 presentations in this session. See more »
VIS Panels
Panel: 20 Years of Visual Analytics
2024-10-16T16:00:00Z – 2024-10-16T17:15:00Z
Chair: David Ebert, Wolfgang Jentner, Ross Maciejewski, Jieqiong Zhao
0 presentations in this session. See more »
2024-10-16T17:45:00Z – 2024-10-16T19:00:00Z
VIS Full Papers
Designing Palettes and Encodings
2024-10-16T17:45:00Z – 2024-10-16T19:00:00Z
Chair: Khairi Rheda
6 presentations in this session. See more »
VIS Full Papers
Of Nodes and Networks
2024-10-16T17:45:00Z – 2024-10-16T19:00:00Z
Chair: Carolina Nobre
6 presentations in this session. See more »
VIS Full Papers
Scripts, Notebooks, and Provenance
2024-10-16T17:45:00Z – 2024-10-16T19:00:00Z
Chair: Alex Lex
6 presentations in this session. See more »
VIS Short Papers
Short Papers: System design
2024-10-16T17:45:00Z – 2024-10-16T19:00:00Z
Chair: Chris Bryan
8 presentations in this session. See more »
VIS Panels
Panel: Past, Present, and Future of Data Storytelling
2024-10-16T17:45:00Z – 2024-10-16T19:00:00Z
Chair: Haotian Li, Yun Wang, Benjamin Bach, Sheelagh Carpendale, Fanny Chevalier, Nathalie Riche
0 presentations in this session. See more »
Application Spotlights
Application Spotlight: Visualization within the Department of Energy
2024-10-16T17:45:00Z – 2024-10-16T19:00:00Z
Chair: Ana Crisan, Menna El-Assady
0 presentations in this session. See more »
2024-10-16T19:00:00Z – 2024-10-16T19:30:00Z
Conference Events
IEEE VIS Town Hall
2024-10-16T19:00:00Z – 2024-10-16T19:30:00Z
Chair: Ross Maciejewski
0 presentations in this session. See more »
2024-10-16T19:30:00Z – 2024-10-16T20:30:00Z
VIS Panels
Panel: VIS Conference Futures: Community Opinions on Recent Experiences, Challenges, and Opportunities for Hybrid Event Formats
2024-10-16T19:30:00Z – 2024-10-16T20:30:00Z
Chair: Matthew Brehmer, Narges Mahyar
0 presentations in this session. See more »
2024-10-13T12:30:00Z – 2024-10-13T15:30:00Z
VAST Challenge
2024-10-13T12:30:00Z – 2024-10-13T15:30:00Z
Chair: R. Jordan Crouser, Steve Gomez, Jereme Haack
10 presentations in this session. See more »
VISxAI: 7th Workshop on Visualization for AI Explainability
2024-10-13T12:30:00Z – 2024-10-13T15:30:00Z
Chair: Alex Bäuerle, Angie Boggust, Fred Hohman
14 presentations in this session. See more »
1st Workshop on Accessible Data Visualization
2024-10-13T12:30:00Z – 2024-10-13T15:30:00Z
Chair: Brianna Wimer, Laura South
7 presentations in this session. See more »
First-Person Visualizations for Outdoor Physical Activities: Challenges and Opportunities
2024-10-13T12:30:00Z – 2024-10-13T15:30:00Z
Chair: Charles Perin, Tica Lin, Lijie Yao, Yalong Yang, Maxime Cordeil, Wesley Willett
0 presentations in this session. See more »
EduVis: Workshop on Visualization Education, Literacy, and Activities
EduVis: 2nd IEEE VIS Workshop on Visualization Education, Literacy, and Activities (Session 1)
2024-10-13T12:30:00Z – 2024-10-13T15:30:00Z
Chair: Fateme Rajabiyazdi, Mandy Keck, Lonni Besancon, Alon Friedman, Benjamin Bach, Jonathan Roberts, Christina Stoiber, Magdalena Boucher, Lily Ge
11 presentations in this session. See more »
Visualization Analysis and Design
2024-10-13T12:30:00Z – 2024-10-13T15:30:00Z
Chair: Tamara Munzner
0 presentations in this session. See more »
Developing Immersive and Collaborative Visualizations with Web-Technologies
Developing Immersive and Collaborative Visualizations with Web Technologies
2024-10-13T12:30:00Z – 2024-10-13T15:30:00Z
Chair: David Saffo
0 presentations in this session. See more »
2024-10-13T16:00:00Z – 2024-10-13T19:00:00Z
VDS: Visualization in Data Science Symposium
2024-10-13T16:00:00Z – 2024-10-13T19:00:00Z
Chair: Ana Crisan, Dylan Cashman, Saugat Pandey, Alvitta Ottley, John E Wenskovitch
7 presentations in this session. See more »
LDAV: 13th IEEE Symposium on Large Data Analysis and Visualization
LDAV: 14th IEEE Symposium on Large Data Analysis and Visualization
2024-10-13T16:00:00Z – 2024-10-13T19:00:00Z
Chair: Silvio Rizzi, Gunther Weber, Guido Reina, Ken Moreland
6 presentations in this session. See more »
Bio+MedVis Challenges
Bio+Med+Vis Workshop
2024-10-13T16:00:00Z – 2024-10-13T19:00:00Z
Chair: Barbora Kozlikova, Nils Gehlenborg, Laura Garrison, Eric Mörth, Morgan Turner, Simon Warchol
6 presentations in this session. See more »
Workshop on Data Storytelling in an Era of Generative AI
2024-10-13T16:00:00Z – 2024-10-13T19:00:00Z
Chair: Xingyu Lan, Leni Yang, Zezhong Wang, Yun Wang, Danqing Shi, Sheelagh Carpendale
4 presentations in this session. See more »
EduVis: Workshop on Visualization Education, Literacy, and Activities
EduVis: 2nd IEEE VIS Workshop on Visualization Education, Literacy, and Activities (Session 2)
2024-10-13T16:00:00Z – 2024-10-13T19:00:00Z
Chair: Jillian Aurisano, Fateme Rajabiyazdi, Mandy Keck, Lonni Besancon, Alon Friedman, Benjamin Bach, Jonathan Roberts, Christina Stoiber, Magdalena Boucher, Lily Ge
5 presentations in this session. See more »
Generating Color Schemes for your Data Visualizations
2024-10-13T16:00:00Z – 2024-10-13T19:00:00Z
Chair: Theresa-Marie Rhyne
0 presentations in this session. See more »
Running Online User Studies with the reVISit Framework
2024-10-13T16:00:00Z – 2024-10-13T19:00:00Z
Chair: Jack Wilburn
0 presentations in this session. See more »
2024-10-14T12:30:00Z – 2024-10-14T15:30:00Z
VisInPractice
2024-10-14T12:30:00Z – 2024-10-14T15:30:00Z
Chair: Arjun Srinivasan, Ayan Biswas
0 presentations in this session. See more »
SciVis Contest
2024-10-14T12:30:00Z – 2024-10-14T15:30:00Z
Chair: Karen Bemis, Tim Gerrits
3 presentations in this session. See more »
BELIV: evaluation and BEyond - methodoLogIcal approaches for Visualization
BELIV: evaluation and BEyond - methodoLogIcal approaches for Visualization (Session 1)
2024-10-14T12:30:00Z – 2024-10-14T15:30:00Z
Chair: Anastasia Bezerianos, Michael Correll, Kyle Hall, Jürgen Bernard, Dan Keefe, Mai Elshehaly, Mahsan Nourani
6 presentations in this session. See more »
Progressive Data Analysis and Visualization (PDAV) Workshop.
Progressive Data Analysis and Visualization (PDAV) Workshop
2024-10-14T12:30:00Z – 2024-10-14T15:30:00Z
Chair: Alex Ulmer, Jaemin Jo, Michael Sedlmair, Jean-Daniel Fekete
3 presentations in this session. See more »
Uncertainty Visualization: Applications, Techniques, Software, and Decision Frameworks
2024-10-14T12:30:00Z – 2024-10-14T15:30:00Z
Chair: Tushar M. Athawale, Chris R. Johnson, Kristi Potter, Paul Rosen, David Pugmire
13 presentations in this session. See more »
Visualization for Climate Action and Sustainability
2024-10-14T12:30:00Z – 2024-10-14T15:30:00Z
Chair: Benjamin Bach, Fanny Chevalier, Helen-Nicole Kostis, Mark SubbaRao, Yvonne Jansen, Robert Soden
13 presentations in this session. See more »
LLM4Vis: Large Language Models for Information Visualization
2024-10-14T12:30:00Z – 2024-10-14T15:30:00Z
Chair: Enamul Hoque
0 presentations in this session. See more »
2024-10-14T16:00:00Z – 2024-10-14T19:00:00Z
TopoInVis: Workshop on Topological Data Analysis and Visualization
2024-10-14T16:00:00Z – 2024-10-14T19:00:00Z
Chair: Federico Iuricich, Yue Zhang
6 presentations in this session. See more »
BELIV: evaluation and BEyond - methodoLogIcal approaches for Visualization
BELIV: evaluation and BEyond - methodoLogIcal approaches for Visualization (Sesssion 2)
2024-10-14T16:00:00Z – 2024-10-14T19:00:00Z
Chair: Anastasia Bezerianos, Michael Correll, Kyle Hall, Jürgen Bernard, Dan Keefe, Mai Elshehaly, Mahsan Nourani
11 presentations in this session. See more »
NLVIZ Workshop: Exploring Research Opportunities for Natural Language, Text, and Data Visualization
2024-10-14T16:00:00Z – 2024-10-14T19:00:00Z
Chair: Vidya Setlur, Arjun Srinivasan
11 presentations in this session. See more »
EnergyVis 2024: 4th Workshop on Energy Data Visualization
2024-10-14T16:00:00Z – 2024-10-14T19:00:00Z
Chair: Kenny Gruchalla, Anjana Arunkumar, Sarah Goodwin, Arnaud Prouzeau, Lyn Bartram
11 presentations in this session. See more »
VISions of the Future: Workshop on Sustainable Practices within Visualization and Physicalisation
2024-10-14T16:00:00Z – 2024-10-14T19:00:00Z
Chair: Georgia Panagiotidou, Luiz Morais, Sarah Hayes, Derya Akbaba, Tatiana Losev, Andrew McNutt
5 presentations in this session. See more »
Enabling Scientific Discovery: A Tutorial for Harnessing the Power of the National Science Data Fabric for Large-Scale Data Analysis
2024-10-14T16:00:00Z – 2024-10-14T19:00:00Z
Chair: Amy Gooch
0 presentations in this session. See more »
Preparing, Conducting, and Analyzing Participatory Design Sessions for Information Visualizations
2024-10-14T16:00:00Z – 2024-10-14T19:00:00Z
Chair: Adriana Arcia
0 presentations in this session. See more »
Add all 2024 conference sessions to your calendar. You can add this address to your online calendaring system if you want to receive updates dynamically.
2024-10-18T12:30:00Z – 2024-10-18T13:45:00Z
VIS Full Papers
Look, Learn, Language Models
2024-10-18T12:30:00Z – 2024-10-18T13:45:00Z
Chair: Nicole Sultanum
6 presentations in this session. See more »
VIS Full Papers
Where the Networks Are
2024-10-18T12:30:00Z – 2024-10-18T13:45:00Z
Chair: Oliver Deussen
6 presentations in this session. See more »
VIS Full Papers
Human and Machine Visualization Literacy
2024-10-18T12:30:00Z – 2024-10-18T13:45:00Z
Chair: Bum Chul Kwon
6 presentations in this session. See more »
VIS Full Papers
Flow, Topology, and Uncertainty
2024-10-18T12:30:00Z – 2024-10-18T13:45:00Z
Chair: Bei Wang
6 presentations in this session. See more »
2024-10-18T14:15:00Z – 2024-10-18T15:00:00Z
Conference Events
Test of Time Awards
2024-10-18T14:15:00Z – 2024-10-18T15:00:00Z
Chair: Ross Maciejewski
1 presentations in this session. See more »
2024-10-18T15:00:00Z – 2024-10-18T16:30:00Z
Conference Events
IEEE VIS Capstone and Closing
2024-10-18T15:00:00Z – 2024-10-18T16:30:00Z
Chair: Paul Rosen, Kristi Potter, Remco Chang
3 presentations in this session. See more »
2024-10-15T12:30:00Z – 2024-10-15T13:45:00Z
Conference Events
Opening Session
2024-10-15T12:30:00Z – 2024-10-15T13:45:00Z
Chair: Paul Rosen, Kristi Potter, Remco Chang
2 presentations in this session. See more »
2024-10-15T14:15:00Z – 2024-10-15T15:45:00Z
VIS Short Papers
VGTC Awards & Best Short Papers
2024-10-15T14:15:00Z – 2024-10-15T15:45:00Z
Chair: Charles Perin, Johanna Beyer, Takayuki Itoh, Hongfen Yu, Chaoli Wang
4 presentations in this session. See more »
2024-10-15T15:35:00Z – 2024-10-15T16:05:00Z
Conference Events
VIS Governance
2024-10-15T15:35:00Z – 2024-10-15T16:05:00Z
Chair: Petra Isenberg, Jean-Daniel Fekete
2 presentations in this session. See more »
2024-10-15T16:00:00Z – 2024-10-15T17:30:00Z
VIS Full Papers
Best Full Papers
2024-10-15T16:00:00Z – 2024-10-15T17:30:00Z
Chair: Claudio Silva
6 presentations in this session. See more »
2024-10-15T18:00:00Z – 2024-10-15T19:00:00Z
VIS Arts Program
VISAP Keynote: The Golden Age of Visualization Dissensus
2024-10-15T18:00:00Z – 2024-10-15T19:00:00Z
Chair: Pedro Cruz, Rewa Wright, Rebecca Ruige Xu, Lori Jacques, Santiago Echeverry, Kate Terrado, Todd Linkner, Alberto Cairo
0 presentations in this session. See more »
2024-10-15T19:00:00Z – 2024-10-15T21:00:00Z
Conference Events
Posters
2024-10-15T19:00:00Z – 2024-10-15T21:00:00Z
0 presentations in this session. See more »
VIS Arts Program
VISAP Artist Talks
2024-10-15T19:00:00Z – 2024-10-15T21:00:00Z
Chair: Pedro Cruz, Rewa Wright, Rebecca Ruige Xu, Lori Jacques, Santiago Echeverry, Kate Terrado, Todd Linkner
16 presentations in this session. See more »
2024-10-17T12:30:00Z – 2024-10-17T13:45:00Z
VIS Full Papers
Visualization Recommendation
2024-10-17T12:30:00Z – 2024-10-17T13:45:00Z
Chair: Johannes Knittel
6 presentations in this session. See more »
VIS Full Papers
Model-checking and Validation
2024-10-17T12:30:00Z – 2024-10-17T13:45:00Z
Chair: Michael Correll
6 presentations in this session. See more »
VIS Full Papers
Embeddings and Document Spatialization
2024-10-17T12:30:00Z – 2024-10-17T13:45:00Z
Chair: Alex Endert
6 presentations in this session. See more »
VIS Short Papers
Short Papers: Perception and Representation
2024-10-17T12:30:00Z – 2024-10-17T13:45:00Z
Chair: Anjana Arunkumar
8 presentations in this session. See more »
VIS Panels
Panel: Human-Centered Computing Research in South America: Status Quo, Opportunities, and Challenges
2024-10-17T12:30:00Z – 2024-10-17T13:45:00Z
Chair: Chaoli Wang
0 presentations in this session. See more »
2024-10-17T14:15:00Z – 2024-10-17T15:30:00Z
VIS Full Papers
Applications: Sports. Games, and Finance
2024-10-17T14:15:00Z – 2024-10-17T15:30:00Z
Chair: Marc Streit
6 presentations in this session. See more »
VIS Full Papers
Visual Design: Sketching and Labeling
2024-10-17T14:15:00Z – 2024-10-17T15:30:00Z
Chair: Jonathan C. Roberts
6 presentations in this session. See more »
VIS Full Papers
Topological Data Analysis
2024-10-17T14:15:00Z – 2024-10-17T15:30:00Z
Chair: Ingrid Hotz
6 presentations in this session. See more »
VIS Short Papers
Short Papers: Text and Multimedia
2024-10-17T14:15:00Z – 2024-10-17T15:30:00Z
Chair: Min Lu
8 presentations in this session. See more »
VIS Panels
Panel: (Yet Another) Evaluation Needed? A Panel Discussion on Evaluation Trends in Visualization
2024-10-17T14:15:00Z – 2024-10-17T15:30:00Z
Chair: Ghulam Jilani Quadri, Danielle Albers Szafir, Arran Zeyu Wang, Hyeon Jeon
0 presentations in this session. See more »
VIS Arts Program
VISAP Pictorials
2024-10-17T14:15:00Z – 2024-10-17T15:30:00Z
Chair: Pedro Cruz, Rewa Wright, Rebecca Ruige Xu, Lori Jacques, Santiago Echeverry, Kate Terrado, Todd Linkner
8 presentations in this session. See more »
2024-10-17T15:30:00Z – 2024-10-17T16:00:00Z
Conference Events
IEEE VIS 2025 Kickoff
2024-10-17T15:30:00Z – 2024-10-17T16:00:00Z
Chair: Johanna Schmidt, Kresimir Matković, Barbora Kozlíková, Eduard Gröller
1 presentations in this session. See more »
2024-10-17T16:00:00Z – 2024-10-17T17:15:00Z
VIS Full Papers
Once Upon a Visualization
2024-10-17T16:00:00Z – 2024-10-17T17:15:00Z
Chair: Marti Hearst
6 presentations in this session. See more »
VIS Full Papers
Visualization Design Methods
2024-10-17T16:00:00Z – 2024-10-17T17:15:00Z
Chair: Miriah Meyer
6 presentations in this session. See more »
VIS Full Papers
The Toolboxes of Visualization
2024-10-17T16:00:00Z – 2024-10-17T17:15:00Z
Chair: Dominik Moritz
6 presentations in this session. See more »
VIS Short Papers
Short Papers: Analytics and Applications
2024-10-17T16:00:00Z – 2024-10-17T17:15:00Z
Chair: Anna Vilanova
8 presentations in this session. See more »
CG&A Invited Partnership Presentations
CG&A: Systems, Theory, and Evaluations
2024-10-17T16:00:00Z – 2024-10-17T17:15:00Z
Chair: Bruce Campbell
6 presentations in this session. See more »
VIS Panels
Panel: Vogue or Visionary? Current Challenges and Future Opportunities in Situated Visualizations
2024-10-17T16:00:00Z – 2024-10-17T17:15:00Z
Chair: Michelle A. Borkin, Melanie Tory
0 presentations in this session. See more »
2024-10-17T17:45:00Z – 2024-10-17T19:00:00Z
VIS Full Papers
Journalism and Public Policy
2024-10-17T17:45:00Z – 2024-10-17T19:00:00Z
Chair: Sungahn Ko
6 presentations in this session. See more »
VIS Full Papers
Applications: Industry, Computing, and Medicine
2024-10-17T17:45:00Z – 2024-10-17T19:00:00Z
Chair: Joern Kohlhammer
6 presentations in this session. See more »
VIS Full Papers
Accessibility and Touch
2024-10-17T17:45:00Z – 2024-10-17T19:00:00Z
Chair: Narges Mahyar
6 presentations in this session. See more »
VIS Full Papers
Motion and Animated Notions
2024-10-17T17:45:00Z – 2024-10-17T19:00:00Z
Chair: Catherine d'Ignazio
6 presentations in this session. See more »
VIS Short Papers
Short Papers: AI and LLM
2024-10-17T17:45:00Z – 2024-10-17T19:00:00Z
Chair: Cindy Xiong Bearfield
8 presentations in this session. See more »
VIS Panels
Panel: Dear Younger Me: A Dialog About Professional Development Beyond The Initial Career Phases
2024-10-17T17:45:00Z – 2024-10-17T19:00:00Z
Chair: Robert M Kirby, Michael Gleicher
0 presentations in this session. See more »
2024-10-16T12:30:00Z – 2024-10-16T13:30:00Z
VIS Full Papers
Virtual: VIS from around the world
2024-10-16T12:30:00Z – 2024-10-16T13:30:00Z
Chair: Mahmood Jasim
6 presentations in this session. See more »
2024-10-16T12:30:00Z – 2024-10-16T13:45:00Z
VIS Full Papers
Text, Annotation, and Metaphor
2024-10-16T12:30:00Z – 2024-10-16T13:45:00Z
Chair: Melanie Tory
6 presentations in this session. See more »
VIS Full Papers
Immersive Visualization and Visual Analytics
2024-10-16T12:30:00Z – 2024-10-16T13:45:00Z
Chair: Lingyun Yu
6 presentations in this session. See more »
VIS Full Papers
Machine Learning for Visualization
2024-10-16T12:30:00Z – 2024-10-16T13:45:00Z
Chair: Joshua Levine
6 presentations in this session. See more »
VIS Short Papers
Short Papers: Graph, Hierarchy and Multidimensional
2024-10-16T12:30:00Z – 2024-10-16T13:45:00Z
Chair: Alfie Abdul-Rahman
8 presentations in this session. See more »
VIS Panels
Panel: What Do Visualization Art Projects Bring to the VIS Community?
2024-10-16T12:30:00Z – 2024-10-16T13:45:00Z
Chair: Xinhuan Shu, Yifang Wang, Junxiu Tang
0 presentations in this session. See more »
2024-10-16T14:15:00Z – 2024-10-16T15:30:00Z
VIS Full Papers
Biological Data Visualization
2024-10-16T14:15:00Z – 2024-10-16T15:30:00Z
Chair: Nils Gehlenborg
6 presentations in this session. See more »
VIS Full Papers
Judgment and Decision-making
2024-10-16T14:15:00Z – 2024-10-16T15:30:00Z
Chair: Wenwen Dou
6 presentations in this session. See more »
VIS Full Papers
Time and Sequences
2024-10-16T14:15:00Z – 2024-10-16T15:30:00Z
Chair: Silvia Miksch
6 presentations in this session. See more »
VIS Full Papers
Dimensionality Reduction
2024-10-16T14:15:00Z – 2024-10-16T15:30:00Z
Chair: Jian Zhao
6 presentations in this session. See more »
VIS Full Papers
Urban Planning, Construction, and Disaster Management
2024-10-16T14:15:00Z – 2024-10-16T15:30:00Z
Chair: Siming Chen
6 presentations in this session. See more »
VIS Arts Program
VISAP Papers
2024-10-16T14:15:00Z – 2024-10-16T15:30:00Z
Chair: Pedro Cruz, Rewa Wright, Rebecca Ruige Xu, Lori Jacques, Santiago Echeverry, Kate Terrado, Todd Linkner
6 presentations in this session. See more »
2024-10-16T16:00:00Z – 2024-10-16T17:15:00Z
VIS Full Papers
Natural Language and Multimodal Interaction
2024-10-16T16:00:00Z – 2024-10-16T17:15:00Z
Chair: Ana Crisan
6 presentations in this session. See more »
VIS Full Papers
Collaboration and Communication
2024-10-16T16:00:00Z – 2024-10-16T17:15:00Z
Chair: Vidya Setlur
6 presentations in this session. See more »
VIS Full Papers
Perception and Cognition
2024-10-16T16:00:00Z – 2024-10-16T17:15:00Z
Chair: Tamara Munzner
6 presentations in this session. See more »
VIS Short Papers
Short Papers: Scientific and Immersive Visualization
2024-10-16T16:00:00Z – 2024-10-16T17:15:00Z
Chair: Bei Wang
8 presentations in this session. See more »
CG&A Invited Partnership Presentations
CG&A: Analytics and Applications
2024-10-16T16:00:00Z – 2024-10-16T17:15:00Z
Chair: Bruce Campbell
6 presentations in this session. See more »
VIS Panels
Panel: 20 Years of Visual Analytics
2024-10-16T16:00:00Z – 2024-10-16T17:15:00Z
Chair: David Ebert, Wolfgang Jentner, Ross Maciejewski, Jieqiong Zhao
0 presentations in this session. See more »
2024-10-16T17:45:00Z – 2024-10-16T19:00:00Z
VIS Full Papers
Designing Palettes and Encodings
2024-10-16T17:45:00Z – 2024-10-16T19:00:00Z
Chair: Khairi Rheda
6 presentations in this session. See more »
VIS Full Papers
Of Nodes and Networks
2024-10-16T17:45:00Z – 2024-10-16T19:00:00Z
Chair: Carolina Nobre
6 presentations in this session. See more »
VIS Full Papers
Scripts, Notebooks, and Provenance
2024-10-16T17:45:00Z – 2024-10-16T19:00:00Z
Chair: Alex Lex
6 presentations in this session. See more »
VIS Short Papers
Short Papers: System design
2024-10-16T17:45:00Z – 2024-10-16T19:00:00Z
Chair: Chris Bryan
8 presentations in this session. See more »
VIS Panels
Panel: Past, Present, and Future of Data Storytelling
2024-10-16T17:45:00Z – 2024-10-16T19:00:00Z
Chair: Haotian Li, Yun Wang, Benjamin Bach, Sheelagh Carpendale, Fanny Chevalier, Nathalie Riche
0 presentations in this session. See more »
Application Spotlights
Application Spotlight: Visualization within the Department of Energy
2024-10-16T17:45:00Z – 2024-10-16T19:00:00Z
Chair: Ana Crisan, Menna El-Assady
0 presentations in this session. See more »
2024-10-16T19:00:00Z – 2024-10-16T19:30:00Z
Conference Events
IEEE VIS Town Hall
2024-10-16T19:00:00Z – 2024-10-16T19:30:00Z
Chair: Ross Maciejewski
0 presentations in this session. See more »
2024-10-16T19:30:00Z – 2024-10-16T20:30:00Z
VIS Panels
Panel: VIS Conference Futures: Community Opinions on Recent Experiences, Challenges, and Opportunities for Hybrid Event Formats
2024-10-16T19:30:00Z – 2024-10-16T20:30:00Z
Chair: Matthew Brehmer, Narges Mahyar
0 presentations in this session. See more »
2024-10-13T12:30:00Z – 2024-10-13T15:30:00Z
VAST Challenge
2024-10-13T12:30:00Z – 2024-10-13T15:30:00Z
Chair: R. Jordan Crouser, Steve Gomez, Jereme Haack
10 presentations in this session. See more »
VISxAI: 7th Workshop on Visualization for AI Explainability
2024-10-13T12:30:00Z – 2024-10-13T15:30:00Z
Chair: Alex Bäuerle, Angie Boggust, Fred Hohman
14 presentations in this session. See more »
1st Workshop on Accessible Data Visualization
2024-10-13T12:30:00Z – 2024-10-13T15:30:00Z
Chair: Brianna Wimer, Laura South
7 presentations in this session. See more »
First-Person Visualizations for Outdoor Physical Activities: Challenges and Opportunities
2024-10-13T12:30:00Z – 2024-10-13T15:30:00Z
Chair: Charles Perin, Tica Lin, Lijie Yao, Yalong Yang, Maxime Cordeil, Wesley Willett
0 presentations in this session. See more »
EduVis: Workshop on Visualization Education, Literacy, and Activities
EduVis: 2nd IEEE VIS Workshop on Visualization Education, Literacy, and Activities (Session 1)
2024-10-13T12:30:00Z – 2024-10-13T15:30:00Z
Chair: Fateme Rajabiyazdi, Mandy Keck, Lonni Besancon, Alon Friedman, Benjamin Bach, Jonathan Roberts, Christina Stoiber, Magdalena Boucher, Lily Ge
11 presentations in this session. See more »
Visualization Analysis and Design
2024-10-13T12:30:00Z – 2024-10-13T15:30:00Z
Chair: Tamara Munzner
0 presentations in this session. See more »
Developing Immersive and Collaborative Visualizations with Web-Technologies
Developing Immersive and Collaborative Visualizations with Web Technologies
2024-10-13T12:30:00Z – 2024-10-13T15:30:00Z
Chair: David Saffo
0 presentations in this session. See more »
2024-10-13T16:00:00Z – 2024-10-13T19:00:00Z
VDS: Visualization in Data Science Symposium
2024-10-13T16:00:00Z – 2024-10-13T19:00:00Z
Chair: Ana Crisan, Dylan Cashman, Saugat Pandey, Alvitta Ottley, John E Wenskovitch
7 presentations in this session. See more »
LDAV: 13th IEEE Symposium on Large Data Analysis and Visualization
LDAV: 14th IEEE Symposium on Large Data Analysis and Visualization
2024-10-13T16:00:00Z – 2024-10-13T19:00:00Z
Chair: Silvio Rizzi, Gunther Weber, Guido Reina, Ken Moreland
6 presentations in this session. See more »
Bio+MedVis Challenges
Bio+Med+Vis Workshop
2024-10-13T16:00:00Z – 2024-10-13T19:00:00Z
Chair: Barbora Kozlikova, Nils Gehlenborg, Laura Garrison, Eric Mörth, Morgan Turner, Simon Warchol
6 presentations in this session. See more »
Workshop on Data Storytelling in an Era of Generative AI
2024-10-13T16:00:00Z – 2024-10-13T19:00:00Z
Chair: Xingyu Lan, Leni Yang, Zezhong Wang, Yun Wang, Danqing Shi, Sheelagh Carpendale
4 presentations in this session. See more »
EduVis: Workshop on Visualization Education, Literacy, and Activities
EduVis: 2nd IEEE VIS Workshop on Visualization Education, Literacy, and Activities (Session 2)
2024-10-13T16:00:00Z – 2024-10-13T19:00:00Z
Chair: Jillian Aurisano, Fateme Rajabiyazdi, Mandy Keck, Lonni Besancon, Alon Friedman, Benjamin Bach, Jonathan Roberts, Christina Stoiber, Magdalena Boucher, Lily Ge
5 presentations in this session. See more »
Generating Color Schemes for your Data Visualizations
2024-10-13T16:00:00Z – 2024-10-13T19:00:00Z
Chair: Theresa-Marie Rhyne
0 presentations in this session. See more »
Running Online User Studies with the reVISit Framework
2024-10-13T16:00:00Z – 2024-10-13T19:00:00Z
Chair: Jack Wilburn
0 presentations in this session. See more »
2024-10-14T12:30:00Z – 2024-10-14T15:30:00Z
VisInPractice
2024-10-14T12:30:00Z – 2024-10-14T15:30:00Z
Chair: Arjun Srinivasan, Ayan Biswas
0 presentations in this session. See more »
SciVis Contest
2024-10-14T12:30:00Z – 2024-10-14T15:30:00Z
Chair: Karen Bemis, Tim Gerrits
3 presentations in this session. See more »
BELIV: evaluation and BEyond - methodoLogIcal approaches for Visualization
BELIV: evaluation and BEyond - methodoLogIcal approaches for Visualization (Session 1)
2024-10-14T12:30:00Z – 2024-10-14T15:30:00Z
Chair: Anastasia Bezerianos, Michael Correll, Kyle Hall, Jürgen Bernard, Dan Keefe, Mai Elshehaly, Mahsan Nourani
6 presentations in this session. See more »
Progressive Data Analysis and Visualization (PDAV) Workshop.
Progressive Data Analysis and Visualization (PDAV) Workshop
2024-10-14T12:30:00Z – 2024-10-14T15:30:00Z
Chair: Alex Ulmer, Jaemin Jo, Michael Sedlmair, Jean-Daniel Fekete
3 presentations in this session. See more »
Uncertainty Visualization: Applications, Techniques, Software, and Decision Frameworks
2024-10-14T12:30:00Z – 2024-10-14T15:30:00Z
Chair: Tushar M. Athawale, Chris R. Johnson, Kristi Potter, Paul Rosen, David Pugmire
13 presentations in this session. See more »
Visualization for Climate Action and Sustainability
2024-10-14T12:30:00Z – 2024-10-14T15:30:00Z
Chair: Benjamin Bach, Fanny Chevalier, Helen-Nicole Kostis, Mark SubbaRao, Yvonne Jansen, Robert Soden
13 presentations in this session. See more »
LLM4Vis: Large Language Models for Information Visualization
2024-10-14T12:30:00Z – 2024-10-14T15:30:00Z
Chair: Enamul Hoque
0 presentations in this session. See more »
2024-10-14T16:00:00Z – 2024-10-14T19:00:00Z
TopoInVis: Workshop on Topological Data Analysis and Visualization
2024-10-14T16:00:00Z – 2024-10-14T19:00:00Z
Chair: Federico Iuricich, Yue Zhang
6 presentations in this session. See more »
BELIV: evaluation and BEyond - methodoLogIcal approaches for Visualization
BELIV: evaluation and BEyond - methodoLogIcal approaches for Visualization (Sesssion 2)
2024-10-14T16:00:00Z – 2024-10-14T19:00:00Z
Chair: Anastasia Bezerianos, Michael Correll, Kyle Hall, Jürgen Bernard, Dan Keefe, Mai Elshehaly, Mahsan Nourani
11 presentations in this session. See more »
NLVIZ Workshop: Exploring Research Opportunities for Natural Language, Text, and Data Visualization
2024-10-14T16:00:00Z – 2024-10-14T19:00:00Z
Chair: Vidya Setlur, Arjun Srinivasan
11 presentations in this session. See more »
EnergyVis 2024: 4th Workshop on Energy Data Visualization
2024-10-14T16:00:00Z – 2024-10-14T19:00:00Z
Chair: Kenny Gruchalla, Anjana Arunkumar, Sarah Goodwin, Arnaud Prouzeau, Lyn Bartram
11 presentations in this session. See more »
VISions of the Future: Workshop on Sustainable Practices within Visualization and Physicalisation
2024-10-14T16:00:00Z – 2024-10-14T19:00:00Z
Chair: Georgia Panagiotidou, Luiz Morais, Sarah Hayes, Derya Akbaba, Tatiana Losev, Andrew McNutt
5 presentations in this session. See more »
Enabling Scientific Discovery: A Tutorial for Harnessing the Power of the National Science Data Fabric for Large-Scale Data Analysis
2024-10-14T16:00:00Z – 2024-10-14T19:00:00Z
Chair: Amy Gooch
0 presentations in this session. See more »
Preparing, Conducting, and Analyzing Participatory Design Sessions for Information Visualizations
2024-10-14T16:00:00Z – 2024-10-14T19:00:00Z
Chair: Adriana Arcia
0 presentations in this session. See more »
- associated
VAST Challenge
Bayshore II
Chair: R. Jordan Crouser, Steve Gomez, Jereme Haack
10 presentations in this session. See more »
workshopVISxAI: 7th Workshop on Visualization for AI Explainability
Bayshore I
Chair: Alex Bäuerle, Angie Boggust, Fred Hohman
14 presentations in this session. See more »
workshop1st Workshop on Accessible Data Visualization
Bayshore V
Chair: Brianna Wimer, Laura South
7 presentations in this session. See more »
workshopFirst-Person Visualizations for Outdoor Physical Activities: Challenges and Opportunities
Bayshore VII
Chair: Charles Perin, Tica Lin, Lijie Yao, Yalong Yang, Maxime Cordeil, Wesley Willett
0 presentations in this session. See more »
workshopEduVis: 2nd IEEE VIS Workshop on Visualization Education, Literacy, and Activities (Session 1)
Esplanade Suites I + II + III
Chair: Fateme Rajabiyazdi, Mandy Keck, Lonni Besancon, Alon Friedman, Benjamin Bach, Jonathan Roberts, Christina Stoiber, Magdalena Boucher, Lily Ge
11 presentations in this session. See more »
tutorialVisualization Analysis and Design
Bayshore VI
Chair: Tamara Munzner
0 presentations in this session. See more »
tutorialDeveloping Immersive and Collaborative Visualizations with Web Technologies
Bayshore III
Chair: David Saffo
0 presentations in this session. See more »
- associated
VDS: Visualization in Data Science Symposium
Bayshore I
Chair: Ana Crisan, Dylan Cashman, Saugat Pandey, Alvitta Ottley, John E Wenskovitch
7 presentations in this session. See more »
associatedLDAV: 14th IEEE Symposium on Large Data Analysis and Visualization
Bayshore II
Chair: Silvio Rizzi, Gunther Weber, Guido Reina, Ken Moreland
6 presentations in this session. See more »
associatedBio+Med+Vis Workshop
Bayshore V
Chair: Barbora Kozlikova, Nils Gehlenborg, Laura Garrison, Eric Mörth, Morgan Turner, Simon Warchol
6 presentations in this session. See more »
workshopWorkshop on Data Storytelling in an Era of Generative AI
Bayshore VII
Chair: Xingyu Lan, Leni Yang, Zezhong Wang, Yun Wang, Danqing Shi, Sheelagh Carpendale
4 presentations in this session. See more »
workshopEduVis: 2nd IEEE VIS Workshop on Visualization Education, Literacy, and Activities (Session 2)
Esplanade Suites I + II + III
Chair: Jillian Aurisano, Fateme Rajabiyazdi, Mandy Keck, Lonni Besancon, Alon Friedman, Benjamin Bach, Jonathan Roberts, Christina Stoiber, Magdalena Boucher, Lily Ge
5 presentations in this session. See more »
tutorialGenerating Color Schemes for your Data Visualizations
Bayshore VI
Chair: Theresa-Marie Rhyne
0 presentations in this session. See more »
tutorialRunning Online User Studies with the reVISit Framework
Bayshore III
Chair: Jack Wilburn
0 presentations in this session. See more »
- associated
VisInPractice
Bayshore III
Chair: Arjun Srinivasan, Ayan Biswas
0 presentations in this session. See more »
associatedSciVis Contest
Bayshore V
Chair: Karen Bemis, Tim Gerrits
3 presentations in this session. See more »
workshopBELIV: evaluation and BEyond - methodoLogIcal approaches for Visualization (Session 1)
Bayshore I
Chair: Anastasia Bezerianos, Michael Correll, Kyle Hall, Jürgen Bernard, Dan Keefe, Mai Elshehaly, Mahsan Nourani
6 presentations in this session. See more »
workshopProgressive Data Analysis and Visualization (PDAV) Workshop
Bayshore VII
Chair: Alex Ulmer, Jaemin Jo, Michael Sedlmair, Jean-Daniel Fekete
3 presentations in this session. See more »
workshopUncertainty Visualization: Applications, Techniques, Software, and Decision Frameworks
Bayshore VI
Chair: Tushar M. Athawale, Chris R. Johnson, Kristi Potter, Paul Rosen, David Pugmire
13 presentations in this session. See more »
workshopVisualization for Climate Action and Sustainability
Esplanade Suites I + II + III
Chair: Benjamin Bach, Fanny Chevalier, Helen-Nicole Kostis, Mark SubbaRao, Yvonne Jansen, Robert Soden
13 presentations in this session. See more »
tutorialLLM4Vis: Large Language Models for Information Visualization
Bayshore II
Chair: Enamul Hoque
0 presentations in this session. See more »
- workshop
TopoInVis: Workshop on Topological Data Analysis and Visualization
Bayshore III
Chair: Federico Iuricich, Yue Zhang
6 presentations in this session. See more »
workshopBELIV: evaluation and BEyond - methodoLogIcal approaches for Visualization (Sesssion 2)
Bayshore I
Chair: Anastasia Bezerianos, Michael Correll, Kyle Hall, Jürgen Bernard, Dan Keefe, Mai Elshehaly, Mahsan Nourani
11 presentations in this session. See more »
workshopNLVIZ Workshop: Exploring Research Opportunities for Natural Language, Text, and Data Visualization
Bayshore II
Chair: Vidya Setlur, Arjun Srinivasan
11 presentations in this session. See more »
workshopEnergyVis 2024: 4th Workshop on Energy Data Visualization
Bayshore VI
Chair: Kenny Gruchalla, Anjana Arunkumar, Sarah Goodwin, Arnaud Prouzeau, Lyn Bartram
11 presentations in this session. See more »
workshopVISions of the Future: Workshop on Sustainable Practices within Visualization and Physicalisation
Esplanade Suites I + II + III
Chair: Georgia Panagiotidou, Luiz Morais, Sarah Hayes, Derya Akbaba, Tatiana Losev, Andrew McNutt
5 presentations in this session. See more »
tutorialEnabling Scientific Discovery: A Tutorial for Harnessing the Power of the National Science Data Fabric for Large-Scale Data Analysis
Bayshore V
Chair: Amy Gooch
0 presentations in this session. See more »
tutorialPreparing, Conducting, and Analyzing Participatory Design Sessions for Information Visualizations
Bayshore VII
Chair: Adriana Arcia
0 presentations in this session. See more »
- vis
Opening Session
Bayshore I + II + III
Chair: Paul Rosen, Kristi Potter, Remco Chang
2 presentations in this session. See more »
- short
VGTC Awards & Best Short Papers
Bayshore I + II + III
Chair: Charles Perin, Johanna Beyer, Takayuki Itoh, Hongfen Yu, Chaoli Wang
4 presentations in this session. See more »
- vis
VIS Governance
Bayshore I
Chair: Petra Isenberg, Jean-Daniel Fekete
2 presentations in this session. See more »
- full
Best Full Papers
Bayshore I + II + III
Chair: Claudio Silva
6 presentations in this session. See more »
- visap
VISAP Keynote: The Golden Age of Visualization Dissensus
Bayshore I + II + III
Chair: Pedro Cruz, Rewa Wright, Rebecca Ruige Xu, Lori Jacques, Santiago Echeverry, Kate Terrado, Todd Linkner, Alberto Cairo
0 presentations in this session. See more »
- visap
VISAP Artist Talks
Bayshore III
Chair: Pedro Cruz, Rewa Wright, Rebecca Ruige Xu, Lori Jacques, Santiago Echeverry, Kate Terrado, Todd Linkner
16 presentations in this session. See more »
- full
Virtual: VIS from around the world
Palma Ceia I
Chair: Mahmood Jasim
6 presentations in this session. See more »
- full
Text, Annotation, and Metaphor
Bayshore V
Chair: Melanie Tory
6 presentations in this session. See more »
fullImmersive Visualization and Visual Analytics
Bayshore II
Chair: Lingyun Yu
6 presentations in this session. See more »
fullMachine Learning for Visualization
Bayshore I
Chair: Joshua Levine
6 presentations in this session. See more »
shortShort Papers: Graph, Hierarchy and Multidimensional
Bayshore VI
Chair: Alfie Abdul-Rahman
8 presentations in this session. See more »
panelPanel: What Do Visualization Art Projects Bring to the VIS Community?
Bayshore VII
Chair: Xinhuan Shu, Yifang Wang, Junxiu Tang
0 presentations in this session. See more »
- full
Biological Data Visualization
Bayshore I
Chair: Nils Gehlenborg
6 presentations in this session. See more »
fullJudgment and Decision-making
Bayshore II
Chair: Wenwen Dou
6 presentations in this session. See more »
fullTime and Sequences
Bayshore VI
Chair: Silvia Miksch
6 presentations in this session. See more »
fullDimensionality Reduction
Bayshore V
Chair: Jian Zhao
6 presentations in this session. See more »
fullUrban Planning, Construction, and Disaster Management
Bayshore VII
Chair: Siming Chen
6 presentations in this session. See more »
visapVISAP Papers
Bayshore III
Chair: Pedro Cruz, Rewa Wright, Rebecca Ruige Xu, Lori Jacques, Santiago Echeverry, Kate Terrado, Todd Linkner
6 presentations in this session. See more »
- full
Natural Language and Multimodal Interaction
Bayshore I
Chair: Ana Crisan
6 presentations in this session. See more »
fullCollaboration and Communication
Bayshore V
Chair: Vidya Setlur
6 presentations in this session. See more »
fullPerception and Cognition
Bayshore II
Chair: Tamara Munzner
6 presentations in this session. See more »
shortShort Papers: Scientific and Immersive Visualization
Bayshore VI
Chair: Bei Wang
8 presentations in this session. See more »
invitedCG&A: Analytics and Applications
Bayshore III
Chair: Bruce Campbell
6 presentations in this session. See more »
panelPanel: 20 Years of Visual Analytics
Bayshore VII
Chair: David Ebert, Wolfgang Jentner, Ross Maciejewski, Jieqiong Zhao
0 presentations in this session. See more »
- full
Designing Palettes and Encodings
Bayshore II
Chair: Khairi Rheda
6 presentations in this session. See more »
fullOf Nodes and Networks
Bayshore I
Chair: Carolina Nobre
6 presentations in this session. See more »
fullScripts, Notebooks, and Provenance
Bayshore V
Chair: Alex Lex
6 presentations in this session. See more »
shortShort Papers: System design
Bayshore VI
Chair: Chris Bryan
8 presentations in this session. See more »
panelPanel: Past, Present, and Future of Data Storytelling
Bayshore VII
Chair: Haotian Li, Yun Wang, Benjamin Bach, Sheelagh Carpendale, Fanny Chevalier, Nathalie Riche
0 presentations in this session. See more »
applicationApplication Spotlight: Visualization within the Department of Energy
Bayshore III
Chair: Ana Crisan, Menna El-Assady
0 presentations in this session. See more »
- vis
IEEE VIS Town Hall
Bayshore I + II + III
Chair: Ross Maciejewski
0 presentations in this session. See more »
- panel
Panel: VIS Conference Futures: Community Opinions on Recent Experiences, Challenges, and Opportunities for Hybrid Event Formats
Bayshore VII
Chair: Matthew Brehmer, Narges Mahyar
0 presentations in this session. See more »
- full
Visualization Recommendation
Bayshore II
Chair: Johannes Knittel
6 presentations in this session. See more »
fullModel-checking and Validation
Bayshore V
Chair: Michael Correll
6 presentations in this session. See more »
fullEmbeddings and Document Spatialization
Bayshore I
Chair: Alex Endert
6 presentations in this session. See more »
shortShort Papers: Perception and Representation
Bayshore VI
Chair: Anjana Arunkumar
8 presentations in this session. See more »
panelPanel: Human-Centered Computing Research in South America: Status Quo, Opportunities, and Challenges
Bayshore VII
Chair: Chaoli Wang
0 presentations in this session. See more »
- full
Applications: Sports. Games, and Finance
Bayshore V
Chair: Marc Streit
6 presentations in this session. See more »
fullVisual Design: Sketching and Labeling
Bayshore II
Chair: Jonathan C. Roberts
6 presentations in this session. See more »
fullTopological Data Analysis
Bayshore I
Chair: Ingrid Hotz
6 presentations in this session. See more »
shortShort Papers: Text and Multimedia
Bayshore VI
Chair: Min Lu
8 presentations in this session. See more »
panelPanel: (Yet Another) Evaluation Needed? A Panel Discussion on Evaluation Trends in Visualization
Bayshore VII
Chair: Ghulam Jilani Quadri, Danielle Albers Szafir, Arran Zeyu Wang, Hyeon Jeon
0 presentations in this session. See more »
visapVISAP Pictorials
Bayshore III
Chair: Pedro Cruz, Rewa Wright, Rebecca Ruige Xu, Lori Jacques, Santiago Echeverry, Kate Terrado, Todd Linkner
8 presentations in this session. See more »
- vis
IEEE VIS 2025 Kickoff
Bayshore I + II + III
Chair: Johanna Schmidt, Kresimir Matković, Barbora Kozlíková, Eduard Gröller
1 presentations in this session. See more »
- full
Once Upon a Visualization
Bayshore V
Chair: Marti Hearst
6 presentations in this session. See more »
fullVisualization Design Methods
Bayshore II
Chair: Miriah Meyer
6 presentations in this session. See more »
fullThe Toolboxes of Visualization
Bayshore I
Chair: Dominik Moritz
6 presentations in this session. See more »
shortShort Papers: Analytics and Applications
Bayshore VI
Chair: Anna Vilanova
8 presentations in this session. See more »
invitedCG&A: Systems, Theory, and Evaluations
Bayshore III
Chair: Francesca Samsel
6 presentations in this session. See more »
panelPanel: Vogue or Visionary? Current Challenges and Future Opportunities in Situated Visualizations
Bayshore VII
Chair: Michelle A. Borkin, Melanie Tory
0 presentations in this session. See more »
- full
Journalism and Public Policy
Bayshore II
Chair: Sungahn Ko
6 presentations in this session. See more »
fullApplications: Industry, Computing, and Medicine
Bayshore V
Chair: Joern Kohlhammer
6 presentations in this session. See more »
fullAccessibility and Touch
Bayshore I
Chair: Narges Mahyar
6 presentations in this session. See more »
fullMotion and Animated Notions
Bayshore III
Chair: Catherine d'Ignazio
6 presentations in this session. See more »
shortShort Papers: AI and LLM
Bayshore VI
Chair: Cindy Xiong Bearfield
8 presentations in this session. See more »
panelPanel: Dear Younger Me: A Dialog About Professional Development Beyond The Initial Career Phases
Bayshore VII
Chair: Robert M Kirby, Michael Gleicher
0 presentations in this session. See more »
- full
Look, Learn, Language Models
Bayshore V
Chair: Nicole Sultanum
6 presentations in this session. See more »
fullWhere the Networks Are
Bayshore VII
Chair: Oliver Deussen
6 presentations in this session. See more »
fullHuman and Machine Visualization Literacy
Bayshore I + II + III
Chair: Bum Chul Kwon
6 presentations in this session. See more »
fullFlow, Topology, and Uncertainty
Bayshore VI
Chair: Bei Wang
6 presentations in this session. See more »
- vis
Test of Time Awards
Bayshore I
Chair: Ross Maciejewski
1 presentations in this session. See more »
- vis
IEEE VIS Capstone and Closing
Bayshore I + II + III
Chair: Paul Rosen, Kristi Potter, Remco Chang
3 presentations in this session. See more »
2024-10-13T12:30:00Z – 2024-10-13T15:30:00Z
2024-10-13T16:00:00Z – 2024-10-13T19:00:00Z
2024-10-14T12:30:00Z – 2024-10-14T15:30:00Z
2024-10-14T16:00:00Z – 2024-10-14T19:00:00Z
2024-10-15T12:30:00Z – 2024-10-15T13:45:00Z
2024-10-15T14:15:00Z – 2024-10-15T15:45:00Z
2024-10-15T15:35:00Z – 2024-10-15T16:05:00Z
2024-10-15T16:00:00Z – 2024-10-15T17:30:00Z
2024-10-15T18:00:00Z – 2024-10-15T19:00:00Z
2024-10-15T19:00:00Z – 2024-10-15T21:00:00Z
2024-10-16T12:30:00Z – 2024-10-16T13:30:00Z
2024-10-16T12:30:00Z – 2024-10-16T13:45:00Z
2024-10-16T14:15:00Z – 2024-10-16T15:30:00Z
2024-10-16T16:00:00Z – 2024-10-16T17:15:00Z
2024-10-16T17:45:00Z – 2024-10-16T19:00:00Z
2024-10-16T19:00:00Z – 2024-10-16T19:30:00Z
2024-10-16T19:30:00Z – 2024-10-16T20:30:00Z
2024-10-17T12:30:00Z – 2024-10-17T13:45:00Z
2024-10-17T14:15:00Z – 2024-10-17T15:30:00Z
2024-10-17T15:30:00Z – 2024-10-17T16:00:00Z
2024-10-17T16:00:00Z – 2024-10-17T17:15:00Z
2024-10-17T17:45:00Z – 2024-10-17T19:00:00Z
2024-10-18T12:30:00Z – 2024-10-18T13:45:00Z
2024-10-18T14:15:00Z – 2024-10-18T15:00:00Z
2024-10-18T15:00:00Z – 2024-10-18T16:30:00Z
- associated
VAST Challenge
Bayshore II
Chair: R. Jordan Crouser, Steve Gomez, Jereme Haack
10 presentations in this session. See more »
workshopVISxAI: 7th Workshop on Visualization for AI Explainability
Bayshore I
Chair: Alex Bäuerle, Angie Boggust, Fred Hohman
14 presentations in this session. See more »
workshop1st Workshop on Accessible Data Visualization
Bayshore V
Chair: Brianna Wimer, Laura South
7 presentations in this session. See more »
workshopFirst-Person Visualizations for Outdoor Physical Activities: Challenges and Opportunities
Bayshore VII
Chair: Charles Perin, Tica Lin, Lijie Yao, Yalong Yang, Maxime Cordeil, Wesley Willett
0 presentations in this session. See more »
workshopEduVis: 2nd IEEE VIS Workshop on Visualization Education, Literacy, and Activities (Session 1)
Esplanade Suites I + II + III
Chair: Fateme Rajabiyazdi, Mandy Keck, Lonni Besancon, Alon Friedman, Benjamin Bach, Jonathan Roberts, Christina Stoiber, Magdalena Boucher, Lily Ge
11 presentations in this session. See more »
tutorialVisualization Analysis and Design
Bayshore VI
Chair: Tamara Munzner
0 presentations in this session. See more »
tutorialDeveloping Immersive and Collaborative Visualizations with Web Technologies
Bayshore III
Chair: David Saffo
0 presentations in this session. See more »
- associated
VDS: Visualization in Data Science Symposium
Bayshore I
Chair: Ana Crisan, Dylan Cashman, Saugat Pandey, Alvitta Ottley, John E Wenskovitch
7 presentations in this session. See more »
associatedLDAV: 14th IEEE Symposium on Large Data Analysis and Visualization
Bayshore II
Chair: Silvio Rizzi, Gunther Weber, Guido Reina, Ken Moreland
6 presentations in this session. See more »
associatedBio+Med+Vis Workshop
Bayshore V
Chair: Barbora Kozlikova, Nils Gehlenborg, Laura Garrison, Eric Mörth, Morgan Turner, Simon Warchol
6 presentations in this session. See more »
workshopWorkshop on Data Storytelling in an Era of Generative AI
Bayshore VII
Chair: Xingyu Lan, Leni Yang, Zezhong Wang, Yun Wang, Danqing Shi, Sheelagh Carpendale
4 presentations in this session. See more »
workshopEduVis: 2nd IEEE VIS Workshop on Visualization Education, Literacy, and Activities (Session 2)
Esplanade Suites I + II + III
Chair: Jillian Aurisano, Fateme Rajabiyazdi, Mandy Keck, Lonni Besancon, Alon Friedman, Benjamin Bach, Jonathan Roberts, Christina Stoiber, Magdalena Boucher, Lily Ge
5 presentations in this session. See more »
tutorialGenerating Color Schemes for your Data Visualizations
Bayshore VI
Chair: Theresa-Marie Rhyne
0 presentations in this session. See more »
tutorialRunning Online User Studies with the reVISit Framework
Bayshore III
Chair: Jack Wilburn
0 presentations in this session. See more »
- associated
VisInPractice
Bayshore III
Chair: Arjun Srinivasan, Ayan Biswas
0 presentations in this session. See more »
associatedSciVis Contest
Bayshore V
Chair: Karen Bemis, Tim Gerrits
3 presentations in this session. See more »
workshopBELIV: evaluation and BEyond - methodoLogIcal approaches for Visualization (Session 1)
Bayshore I
Chair: Anastasia Bezerianos, Michael Correll, Kyle Hall, Jürgen Bernard, Dan Keefe, Mai Elshehaly, Mahsan Nourani
6 presentations in this session. See more »
workshopProgressive Data Analysis and Visualization (PDAV) Workshop
Bayshore VII
Chair: Alex Ulmer, Jaemin Jo, Michael Sedlmair, Jean-Daniel Fekete
3 presentations in this session. See more »
workshopUncertainty Visualization: Applications, Techniques, Software, and Decision Frameworks
Bayshore VI
Chair: Tushar M. Athawale, Chris R. Johnson, Kristi Potter, Paul Rosen, David Pugmire
13 presentations in this session. See more »
workshopVisualization for Climate Action and Sustainability
Esplanade Suites I + II + III
Chair: Benjamin Bach, Fanny Chevalier, Helen-Nicole Kostis, Mark SubbaRao, Yvonne Jansen, Robert Soden
13 presentations in this session. See more »
tutorialLLM4Vis: Large Language Models for Information Visualization
Bayshore II
Chair: Enamul Hoque
0 presentations in this session. See more »
- workshop
TopoInVis: Workshop on Topological Data Analysis and Visualization
Bayshore III
Chair: Federico Iuricich, Yue Zhang
6 presentations in this session. See more »
workshopBELIV: evaluation and BEyond - methodoLogIcal approaches for Visualization (Sesssion 2)
Bayshore I
Chair: Anastasia Bezerianos, Michael Correll, Kyle Hall, Jürgen Bernard, Dan Keefe, Mai Elshehaly, Mahsan Nourani
11 presentations in this session. See more »
workshopNLVIZ Workshop: Exploring Research Opportunities for Natural Language, Text, and Data Visualization
Bayshore II
Chair: Vidya Setlur, Arjun Srinivasan
11 presentations in this session. See more »
workshopEnergyVis 2024: 4th Workshop on Energy Data Visualization
Bayshore VI
Chair: Kenny Gruchalla, Anjana Arunkumar, Sarah Goodwin, Arnaud Prouzeau, Lyn Bartram
11 presentations in this session. See more »
workshopVISions of the Future: Workshop on Sustainable Practices within Visualization and Physicalisation
Esplanade Suites I + II + III
Chair: Georgia Panagiotidou, Luiz Morais, Sarah Hayes, Derya Akbaba, Tatiana Losev, Andrew McNutt
5 presentations in this session. See more »
tutorialEnabling Scientific Discovery: A Tutorial for Harnessing the Power of the National Science Data Fabric for Large-Scale Data Analysis
Bayshore V
Chair: Amy Gooch
0 presentations in this session. See more »
tutorialPreparing, Conducting, and Analyzing Participatory Design Sessions for Information Visualizations
Bayshore VII
Chair: Adriana Arcia
0 presentations in this session. See more »
- vis
Opening Session
Bayshore I + II + III
Chair: Paul Rosen, Kristi Potter, Remco Chang
2 presentations in this session. See more »
- short
VGTC Awards & Best Short Papers
Bayshore I + II + III
Chair: Charles Perin, Johanna Beyer, Takayuki Itoh, Hongfen Yu, Chaoli Wang
4 presentations in this session. See more »
- vis
VIS Governance
Bayshore III
Chair: Petra Isenberg, Jean-Daniel Fekete
2 presentations in this session. See more »
- full
Best Full Papers
Bayshore I + II + III
Chair: Claudio Silva
6 presentations in this session. See more »
- visap
VISAP Keynote: The Golden Age of Visualization Dissensus
Bayshore I + II + III
Chair: Pedro Cruz, Rewa Wright, Rebecca Ruige Xu, Lori Jacques, Santiago Echeverry, Kate Terrado, Todd Linkner, Alberto Cairo
0 presentations in this session. See more »
- visap
VISAP Artist Talks
Bayshore III
Chair: Pedro Cruz, Rewa Wright, Rebecca Ruige Xu, Lori Jacques, Santiago Echeverry, Kate Terrado, Todd Linkner
16 presentations in this session. See more »
- full
Virtual: VIS from around the world
Palma Ceia I
Chair: Mahmood Jasim
6 presentations in this session. See more »
- full
Text, Annotation, and Metaphor
Bayshore V
Chair: Melanie Tory
6 presentations in this session. See more »
fullImmersive Visualization and Visual Analytics
Bayshore II
Chair: Lingyun Yu
6 presentations in this session. See more »
fullMachine Learning for Visualization
Bayshore I
Chair: Joshua Levine
6 presentations in this session. See more »
shortShort Papers: Graph, Hierarchy and Multidimensional
Bayshore VI
Chair: Alfie Abdul-Rahman
8 presentations in this session. See more »
panelPanel: What Do Visualization Art Projects Bring to the VIS Community?
Bayshore VII
Chair: Xinhuan Shu, Yifang Wang, Junxiu Tang
0 presentations in this session. See more »
- full
Biological Data Visualization
Bayshore I
Chair: Nils Gehlenborg
6 presentations in this session. See more »
fullJudgment and Decision-making
Bayshore II
Chair: Wenwen Dou
6 presentations in this session. See more »
fullTime and Sequences
Bayshore VI
Chair: Silvia Miksch
6 presentations in this session. See more »
fullDimensionality Reduction
Bayshore V
Chair: Jian Zhao
6 presentations in this session. See more »
fullUrban Planning, Construction, and Disaster Management
Bayshore VII
Chair: Siming Chen
6 presentations in this session. See more »
visapVISAP Papers
Bayshore III
Chair: Pedro Cruz, Rewa Wright, Rebecca Ruige Xu, Lori Jacques, Santiago Echeverry, Kate Terrado, Todd Linkner
6 presentations in this session. See more »
- full
Natural Language and Multimodal Interaction
Bayshore I
Chair: Ana Crisan
6 presentations in this session. See more »
fullCollaboration and Communication
Bayshore V
Chair: Vidya Setlur
6 presentations in this session. See more »
fullPerception and Cognition
Bayshore II
Chair: Tamara Munzner
6 presentations in this session. See more »
shortShort Papers: Scientific and Immersive Visualization
Bayshore VI
Chair: Bei Wang
8 presentations in this session. See more »
invitedCG&A: Analytics and Applications
Bayshore III
Chair: Bruce Campbell
6 presentations in this session. See more »
panelPanel: 20 Years of Visual Analytics
Bayshore VII
Chair: David Ebert, Wolfgang Jentner, Ross Maciejewski, Jieqiong Zhao
0 presentations in this session. See more »
- full
Designing Palettes and Encodings
Bayshore II
Chair: Khairi Rheda
6 presentations in this session. See more »
fullOf Nodes and Networks
Bayshore I
Chair: Carolina Nobre
6 presentations in this session. See more »
fullScripts, Notebooks, and Provenance
Bayshore V
Chair: Alex Lex
6 presentations in this session. See more »
shortShort Papers: System design
Bayshore VI
Chair: Chris Bryan
8 presentations in this session. See more »
panelPanel: Past, Present, and Future of Data Storytelling
Bayshore VII
Chair: Haotian Li, Yun Wang, Benjamin Bach, Sheelagh Carpendale, Fanny Chevalier, Nathalie Riche
0 presentations in this session. See more »
applicationApplication Spotlight: Visualization within the Department of Energy
Bayshore III
Chair: Ana Crisan, Menna El-Assady
0 presentations in this session. See more »
- vis
IEEE VIS Town Hall
Bayshore I + II + III
Chair: Ross Maciejewski
0 presentations in this session. See more »
- panel
Panel: VIS Conference Futures: Community Opinions on Recent Experiences, Challenges, and Opportunities for Hybrid Event Formats
Bayshore VII
Chair: Matthew Brehmer, Narges Mahyar
0 presentations in this session. See more »
- full
Visualization Recommendation
Bayshore II
Chair: Johannes Knittel
6 presentations in this session. See more »
fullModel-checking and Validation
Bayshore V
Chair: Michael Correll
6 presentations in this session. See more »
fullEmbeddings and Document Spatialization
Bayshore I
Chair: Alex Endert
6 presentations in this session. See more »
shortShort Papers: Perception and Representation
Bayshore VI
Chair: Anjana Arunkumar
8 presentations in this session. See more »
panelPanel: Human-Centered Computing Research in South America: Status Quo, Opportunities, and Challenges
Bayshore VII
Chair: Chaoli Wang
0 presentations in this session. See more »
- full
Applications: Sports. Games, and Finance
Bayshore V
Chair: Marc Streit
6 presentations in this session. See more »
fullVisual Design: Sketching and Labeling
Bayshore II
Chair: Jonathan C. Roberts
6 presentations in this session. See more »
fullTopological Data Analysis
Bayshore I
Chair: Ingrid Hotz
6 presentations in this session. See more »
shortShort Papers: Text and Multimedia
Bayshore VI
Chair: Min Lu
8 presentations in this session. See more »
panelPanel: (Yet Another) Evaluation Needed? A Panel Discussion on Evaluation Trends in Visualization
Bayshore VII
Chair: Ghulam Jilani Quadri, Danielle Albers Szafir, Arran Zeyu Wang, Hyeon Jeon
0 presentations in this session. See more »
visapVISAP Pictorials
Bayshore III
Chair: Pedro Cruz, Rewa Wright, Rebecca Ruige Xu, Lori Jacques, Santiago Echeverry, Kate Terrado, Todd Linkner
8 presentations in this session. See more »
- vis
IEEE VIS 2025 Kickoff
Bayshore I + II + III
Chair: Johanna Schmidt, Kresimir Matković, Barbora Kozlíková, Eduard Gröller
1 presentations in this session. See more »
- full
Once Upon a Visualization
Bayshore V
Chair: Marti Hearst
6 presentations in this session. See more »
fullVisualization Design Methods
Bayshore II
Chair: Miriah Meyer
6 presentations in this session. See more »
fullThe Toolboxes of Visualization
Bayshore I
Chair: Dominik Moritz
6 presentations in this session. See more »
shortShort Papers: Analytics and Applications
Bayshore VI
Chair: Anna Vilanova
8 presentations in this session. See more »
invitedCG&A: Systems, Theory, and Evaluations
Bayshore III
Chair: Bruce Campbell
6 presentations in this session. See more »
panelPanel: Vogue or Visionary? Current Challenges and Future Opportunities in Situated Visualizations
Bayshore VII
Chair: Michelle A. Borkin, Melanie Tory
0 presentations in this session. See more »
- full
Journalism and Public Policy
Bayshore II
Chair: Sungahn Ko
6 presentations in this session. See more »
fullApplications: Industry, Computing, and Medicine
Bayshore V
Chair: Joern Kohlhammer
6 presentations in this session. See more »
fullAccessibility and Touch
Bayshore I
Chair: Narges Mahyar
6 presentations in this session. See more »
fullMotion and Animated Notions
Bayshore III
Chair: Catherine d'Ignazio
6 presentations in this session. See more »
shortShort Papers: AI and LLM
Bayshore VI
Chair: Cindy Xiong Bearfield
8 presentations in this session. See more »
panelPanel: Dear Younger Me: A Dialog About Professional Development Beyond The Initial Career Phases
Bayshore VII
Chair: Robert M Kirby, Michael Gleicher
0 presentations in this session. See more »
- full
Look, Learn, Language Models
Bayshore V
Chair: Nicole Sultanum
6 presentations in this session. See more »
fullWhere the Networks Are
Bayshore VII
Chair: Oliver Deussen
6 presentations in this session. See more »
fullHuman and Machine Visualization Literacy
Bayshore I + II + III
Chair: Bum Chul Kwon
6 presentations in this session. See more »
fullFlow, Topology, and Uncertainty
Bayshore VI
Chair: Bei Wang
6 presentations in this session. See more »
- vis
Test of Time Awards
Bayshore I
Chair: Ross Maciejewski
1 presentations in this session. See more »
- vis
IEEE VIS Capstone and Closing
Bayshore I + II + III
Chair: Paul Rosen, Kristi Potter, Remco Chang
3 presentations in this session. See more »
2024-10-13T12:30:00Z – 2024-10-13T15:30:00Z
2024-10-13T16:00:00Z – 2024-10-13T19:00:00Z
2024-10-14T12:30:00Z – 2024-10-14T15:30:00Z
2024-10-14T16:00:00Z – 2024-10-14T19:00:00Z
2024-10-15T12:30:00Z – 2024-10-15T13:45:00Z
2024-10-15T14:15:00Z – 2024-10-15T15:45:00Z
2024-10-15T15:35:00Z – 2024-10-15T16:05:00Z
2024-10-15T16:00:00Z – 2024-10-15T17:30:00Z
2024-10-15T18:00:00Z – 2024-10-15T19:00:00Z
2024-10-15T19:00:00Z – 2024-10-15T21:00:00Z
2024-10-16T12:30:00Z – 2024-10-16T13:30:00Z
2024-10-16T12:30:00Z – 2024-10-16T13:45:00Z
2024-10-16T14:15:00Z – 2024-10-16T15:30:00Z
2024-10-16T16:00:00Z – 2024-10-16T17:15:00Z
2024-10-16T17:45:00Z – 2024-10-16T19:00:00Z
2024-10-16T19:00:00Z – 2024-10-16T19:30:00Z
2024-10-16T19:30:00Z – 2024-10-16T20:30:00Z
2024-10-17T12:30:00Z – 2024-10-17T13:45:00Z
2024-10-17T14:15:00Z – 2024-10-17T15:30:00Z
2024-10-17T15:30:00Z – 2024-10-17T16:00:00Z
2024-10-17T16:00:00Z – 2024-10-17T17:15:00Z
2024-10-17T17:45:00Z – 2024-10-17T19:00:00Z
2024-10-18T12:30:00Z – 2024-10-18T13:45:00Z
2024-10-18T14:15:00Z – 2024-10-18T15:00:00Z
2024-10-18T15:00:00Z – 2024-10-18T16:30:00Z
CG&A Invited Partnership Presentations
https://ieeevis.org/year/2024/program/event_v-cga.html
2024-10-16T16:00:00Z – 2024-10-17T17:15:00Z
Add all of this event's sessions to your calendar.
CG&A: Analytics and Applications
2024-10-16T16:00:00Z – 2024-10-16T17:15:00Z
Chair: Bruce Campbell
6 presentations in this session. See more »
CG&A: Systems, Theory, and Evaluations
2024-10-17T16:00:00Z – 2024-10-17T17:15:00Z
Chair: Francesca Samsel
6 presentations in this session. See more »
CG&A Invited Partnership Presentations
https://ieeevis.org/year/2024/program/event_v-cga.html
2024-10-16T16:00:00Z – 2024-10-17T17:15:00Z
Add all of this event's sessions to your calendar.
CG&A: Analytics and Applications
2024-10-16T16:00:00Z – 2024-10-16T17:15:00Z
Chair: Bruce Campbell
6 presentations in this session. See more »
CG&A: Systems, Theory, and Evaluations
2024-10-17T16:00:00Z – 2024-10-17T17:15:00Z
Chair: Bruce Campbell
6 presentations in this session. See more »
DiffSeer: Difference-Based Dynamic Weighted Graph Visualization
Xiaolin Wen -
Yong Wang -
Meixuan Wu -
Fengjie Wang -
Xuanwu Yue -
Qiaomu Shen -
Yuxin Ma -
Min Zhu -
Download preprint PDF
Access paper PDF
DOI: 10.1109/MCG.2023.3248289
Room: Bayshore III
2024-10-17T16:00:00Z GMT-0600 Change your timezone on the schedule page
2024-10-17T16:00:00Z
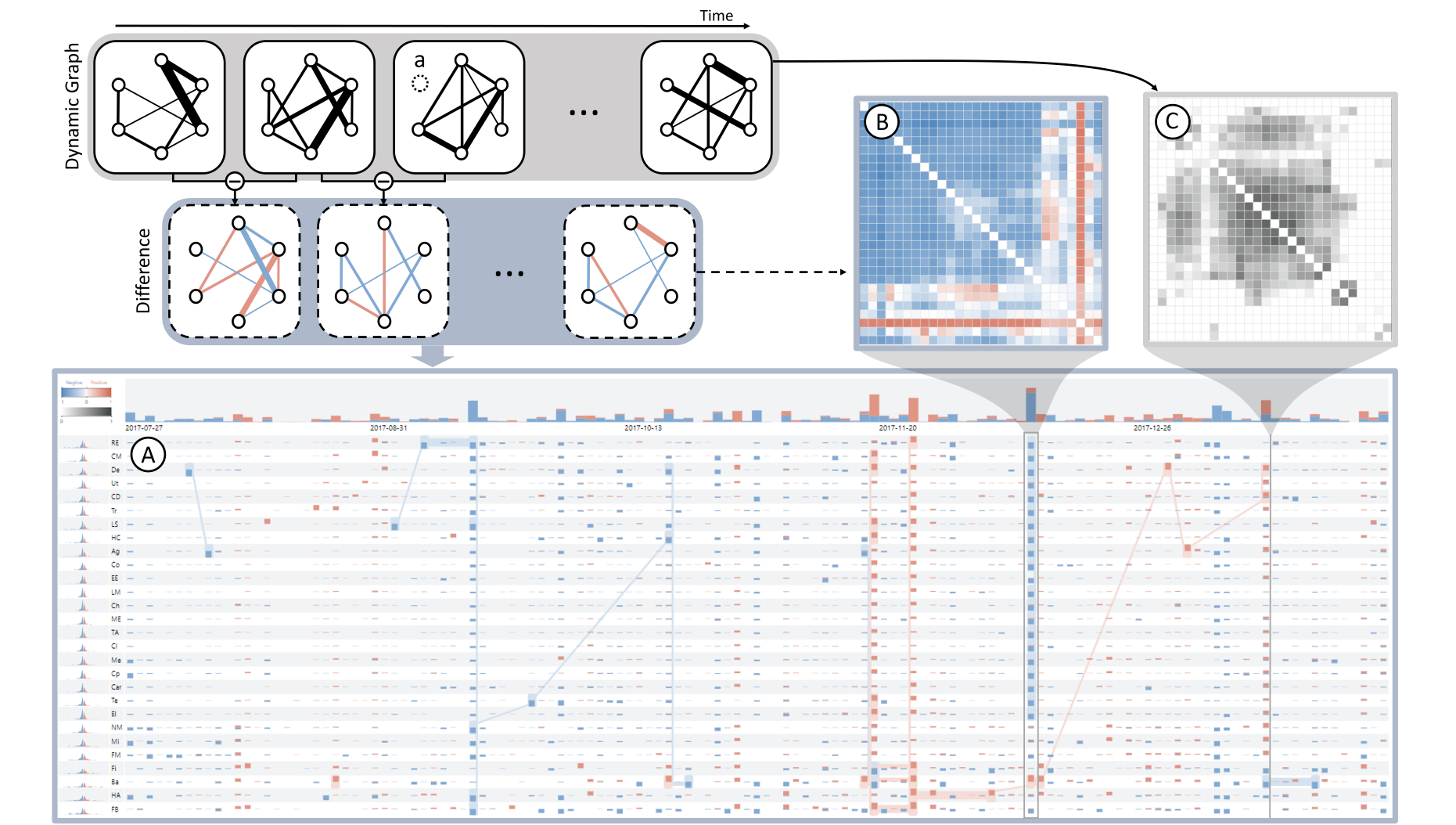
Fast forward
Keywords
Visibility Graph, Spatial Patterns, Weight Change, In-depth Interviews, Temporal Changes, Temporal Evolution, Negative Changes, Interesting Patterns, Edge Weights, Real-world Datasets, Graph Structure, Visual Approach, Dynamic Visualization, Dynamic Graph, Financial Networks, Graph Datasets, Similar Evolutionary Patterns, User Interviews, Similar Changes, Chinese New Year, Sector Indices, Original Graph, Red Rectangle, Nodes In Order, Stock Market Crash, Stacked Bar Charts, Different Types Of Matrices, Chinese New, Blue Rectangle
Abstract
Existing dynamic weighted graph visualization approaches rely on users’ mental comparison to perceive temporal evolution of dynamic weighted graphs, hindering users from effectively analyzing changes across multiple timeslices. We propose DiffSeer, a novel approach for dynamic weighted graph visualization by explicitly visualizing the differences of graph structures (e.g., edge weight differences) between adjacent timeslices. Specifically, we present a novel nested matrix design that overviews the graph structure differences over a time period as well as shows graph structure details in the timeslices of user interest. By collectively considering the overall temporal evolution and structure details in each timeslice, an optimization-based node reordering strategy is developed to group nodes with similar evolution patterns and highlight interesting graph structure details in each timeslice. We conducted two case studies on real-world graph datasets and in-depth interviews with 12 target users to evaluate DiffSeer. The results demonstrate its effectiveness in visualizing dynamic weighted graphs.
DiffSeer: Difference-Based Dynamic Weighted Graph Visualization
Xiaolin Wen -
Yong Wang -
Meixuan Wu -
Fengjie Wang -
Xuanwu Yue -
Qiaomu Shen -
Yuxin Ma -
Min Zhu -
Download preprint PDF
Access paper PDF
DOI: 10.1109/MCG.2023.3248289
Room: Bayshore III
2024-10-17T16:00:00Z GMT-0600 Change your timezone on the schedule page
2024-10-17T16:00:00Z
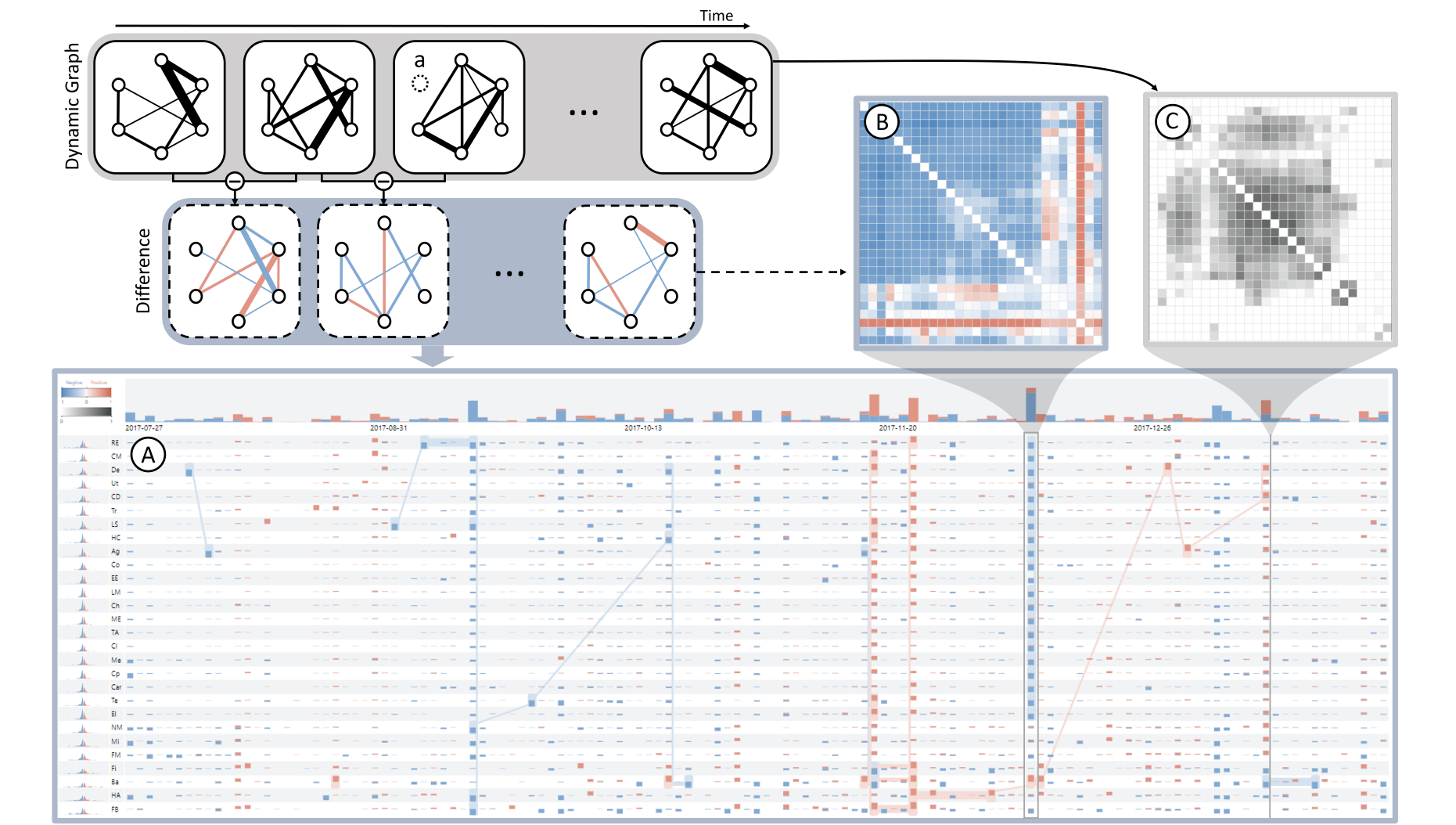
Fast forward
Keywords
Visibility Graph, Spatial Patterns, Weight Change, In-depth Interviews, Temporal Changes, Temporal Evolution, Negative Changes, Interesting Patterns, Edge Weights, Real-world Datasets, Graph Structure, Visual Approach, Dynamic Visualization, Dynamic Graph, Financial Networks, Graph Datasets, Similar Evolutionary Patterns, User Interviews, Similar Changes, Chinese New Year, Sector Indices, Original Graph, Red Rectangle, Nodes In Order, Stock Market Crash, Stacked Bar Charts, Different Types Of Matrices, Chinese New, Blue Rectangle
Abstract
Existing dynamic weighted graph visualization approaches rely on users’ mental comparison to perceive temporal evolution of dynamic weighted graphs, hindering users from effectively analyzing changes across multiple timeslices. We propose DiffSeer, a novel approach for dynamic weighted graph visualization by explicitly visualizing the differences of graph structures (e.g., edge weight differences) between adjacent timeslices. Specifically, we present a novel nested matrix design that overviews the graph structure differences over a time period as well as shows graph structure details in the timeslices of user interest. By collectively considering the overall temporal evolution and structure details in each timeslice, an optimization-based node reordering strategy is developed to group nodes with similar evolution patterns and highlight interesting graph structure details in each timeslice. We conducted two case studies on real-world graph datasets and in-depth interviews with 12 target users to evaluate DiffSeer. The results demonstrate its effectiveness in visualizing dynamic weighted graphs.
Improving Property Graph Layouts by Leveraging Attribute Similarity for Structurally Equivalent Nodes
Patrick Mackey - Pacific Northwest National Lab, Richland, United States
Jacob Miller - University of Arizona, Tucson, United States. Pacific Northwest National Laboratory, Richland, United States
Liz Faultersack - Pacific Northwest National Laboratory, Richland, United States
Download camera-ready PDF
Room: Bayshore VI
2024-10-16T12:48:00Z GMT-0600 Change your timezone on the schedule page
2024-10-16T12:48:00Z
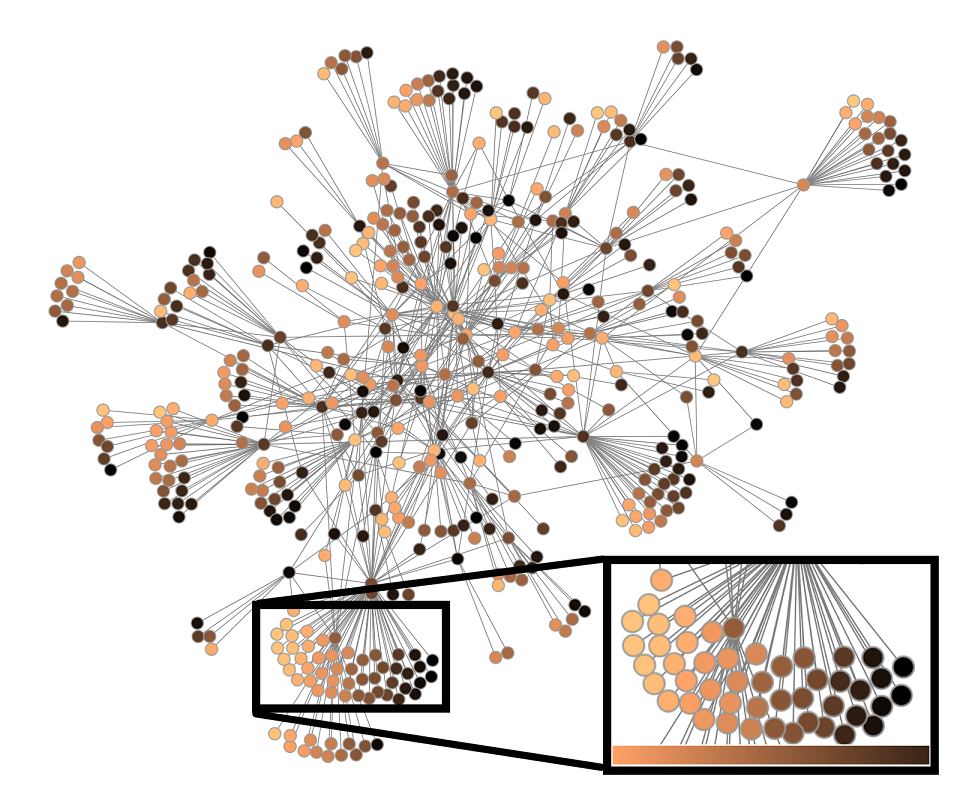
Fast forward
Keywords
graph drawing, network visualization, property graphs, attributed networks
Abstract
Many real-world networks contain structurally-equivalent nodes. These are defined as vertices that share the same set of neighboring nodes, making them interchangeable with a traditional graph layout approach. However, many real-world graphs also have properties associated with nodes, adding additional meaning to them. We present an approach for swapping locations of structurally-equivalent nodes in graph layout so that those with more similar properties have closer proximity to each other. This improves the usefulness of the visualization from an attribute perspective without negatively impacting the visualization from a structural perspective. We include an algorithm for finding these sets of nodes in linear time, as well as methodologies for ordering nodes based on their attribute similarity, which works for scalar, ordinal, multidimensional, and categorical data.
Improving Property Graph Layouts by Leveraging Attribute Similarity for Structurally Equivalent Nodes
Patrick Mackey - Pacific Northwest National Lab, Richland, United States
Jacob Miller - University of Arizona, Tucson, United States. Pacific Northwest National Laboratory, Richland, United States
Liz Faultersack - Pacific Northwest National Laboratory, Richland, United States
Download camera-ready PDF
Room: Bayshore VI
2024-10-16T12:48:00Z GMT-0600 Change your timezone on the schedule page
2024-10-16T12:48:00Z
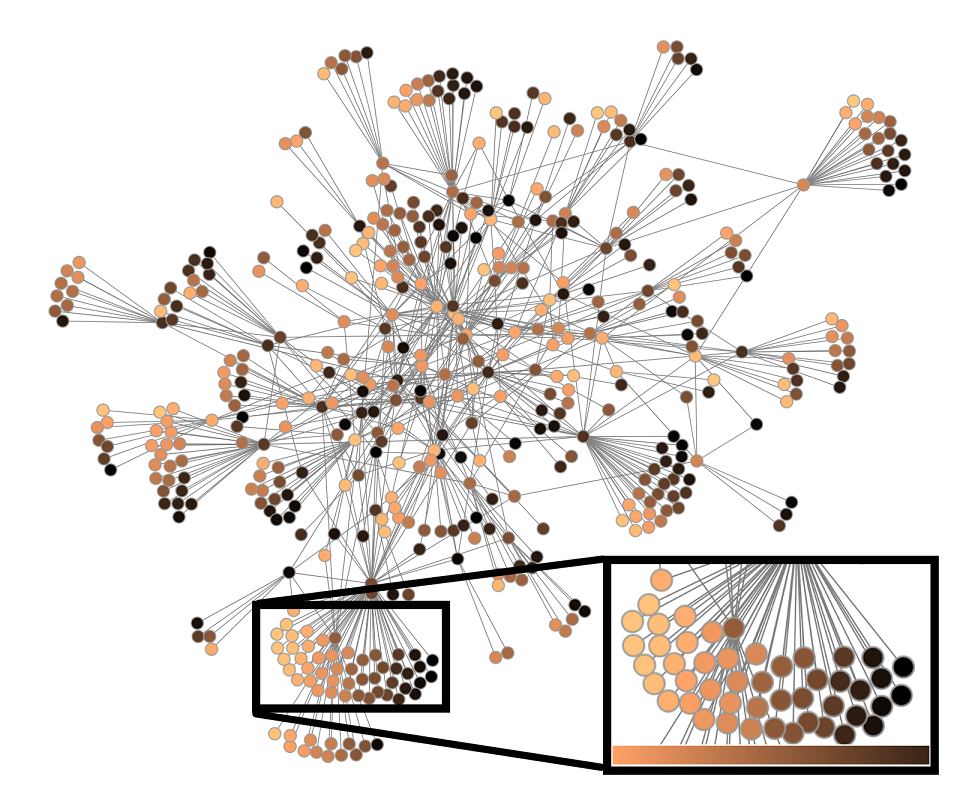
Fast forward
Keywords
graph drawing, network visualization, property graphs, attributed networks
Abstract
Many real-world networks contain structurally-equivalent nodes. These are defined as vertices that share the same set of neighboring nodes, making them interchangeable with a traditional graph layout approach. However, many real-world graphs also have properties associated with nodes, adding additional meaning to them. We present an approach for swapping locations of structurally-equivalent nodes in graph layout so that those with more similar properties have closer proximity to each other. This improves the usefulness of the visualization from an attribute perspective without negatively impacting the visualization from a structural perspective. We include an algorithm for finding these sets of nodes in linear time, as well as methodologies for ordering nodes based on their attribute similarity, which works for scalar, ordinal, multidimensional, and categorical data.
FAVis: Visual Analytics of Factor Analysis for Psychological Research
Yikai Lu - University of Notre Dame, Notre Dame, United States. University of Notre Dame, Notre Dame, United States
Chaoli Wang - University of Notre Dame, Notre Dame, United States
Screen-reader Accessible PDF
Download preprint PDF
Download camera-ready PDF
Room: Bayshore VI
2024-10-17T16:00:00Z GMT-0600 Change your timezone on the schedule page
2024-10-17T16:00:00Z
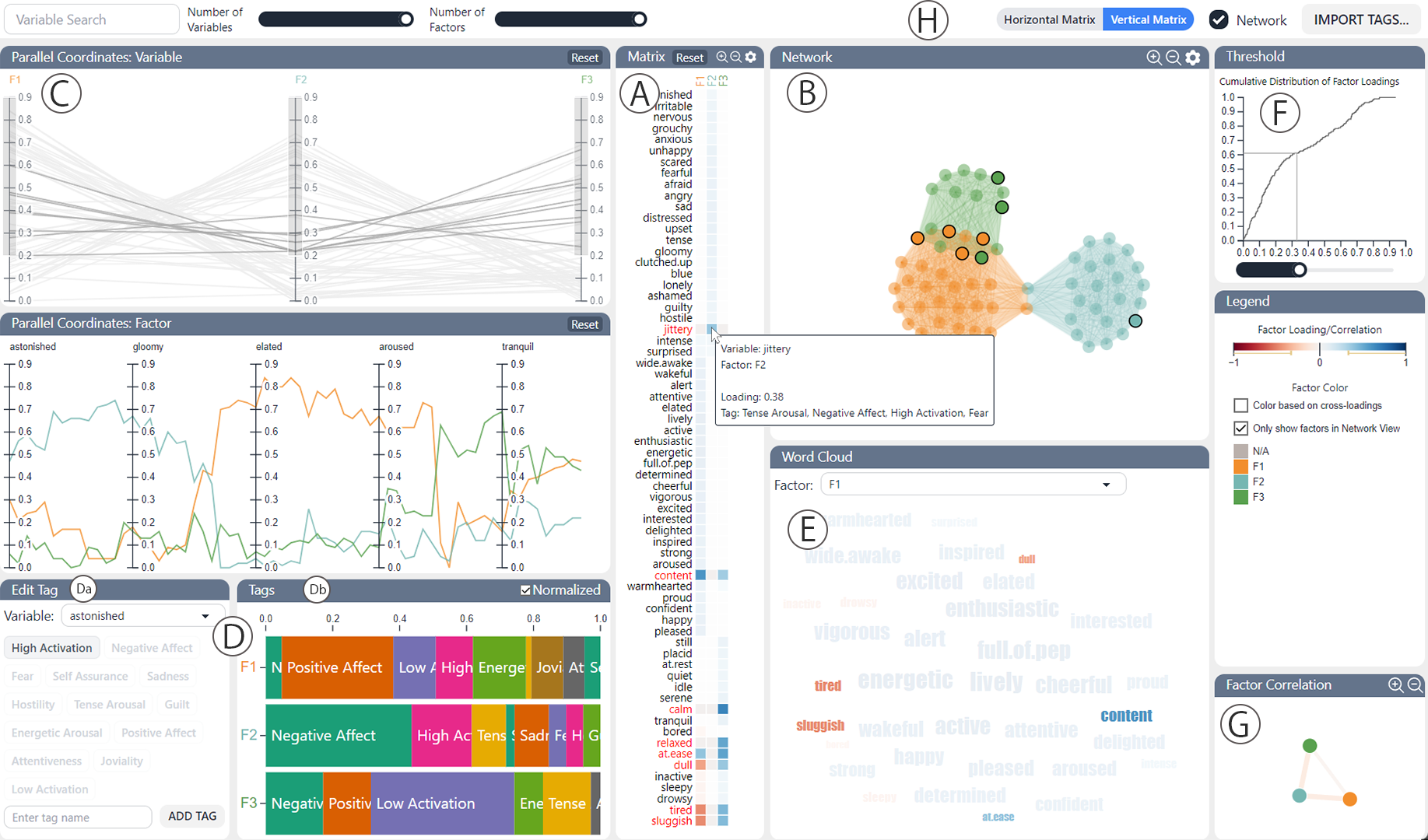
Fast forward
Keywords
Machine Learning, Statistics, Modelling, and Simulation Applications, Coordinated and Multiple Views, High-dimensional Data
Abstract
Psychological research often involves understanding psychological constructs through conducting factor analysis on data collected by a questionnaire, which can comprise hundreds of questions. Without interactive systems for interpreting factor models, researchers are frequently exposed to subjectivity, potentially leading to misinterpretations or overlooked crucial information. This paper introduces FAVis, a novel interactive visualization tool designed to aid researchers in interpreting and evaluating factor analysis results. FAVis enhances the understanding of relationships between variables and factors by supporting multiple views for visualizing factor loadings and correlations, allowing users to analyze information from various perspectives. The primary feature of FAVis is to enable users to set optimal thresholds for factor loadings to balance clarity and information retention. FAVis also allows users to assign tags to variables, enhancing the understanding of factors by linking them to their associated psychological constructs. Our user study demonstrates the utility of FAVis in various tasks.
FAVis: Visual Analytics of Factor Analysis for Psychological Research
Yikai Lu - University of Notre Dame, Notre Dame, United States. University of Notre Dame, Notre Dame, United States
Chaoli Wang - University of Notre Dame, Notre Dame, United States
Screen-reader Accessible PDF
Download preprint PDF
Download camera-ready PDF
Room: Bayshore VI
2024-10-17T16:00:00Z GMT-0600 Change your timezone on the schedule page
2024-10-17T16:00:00Z
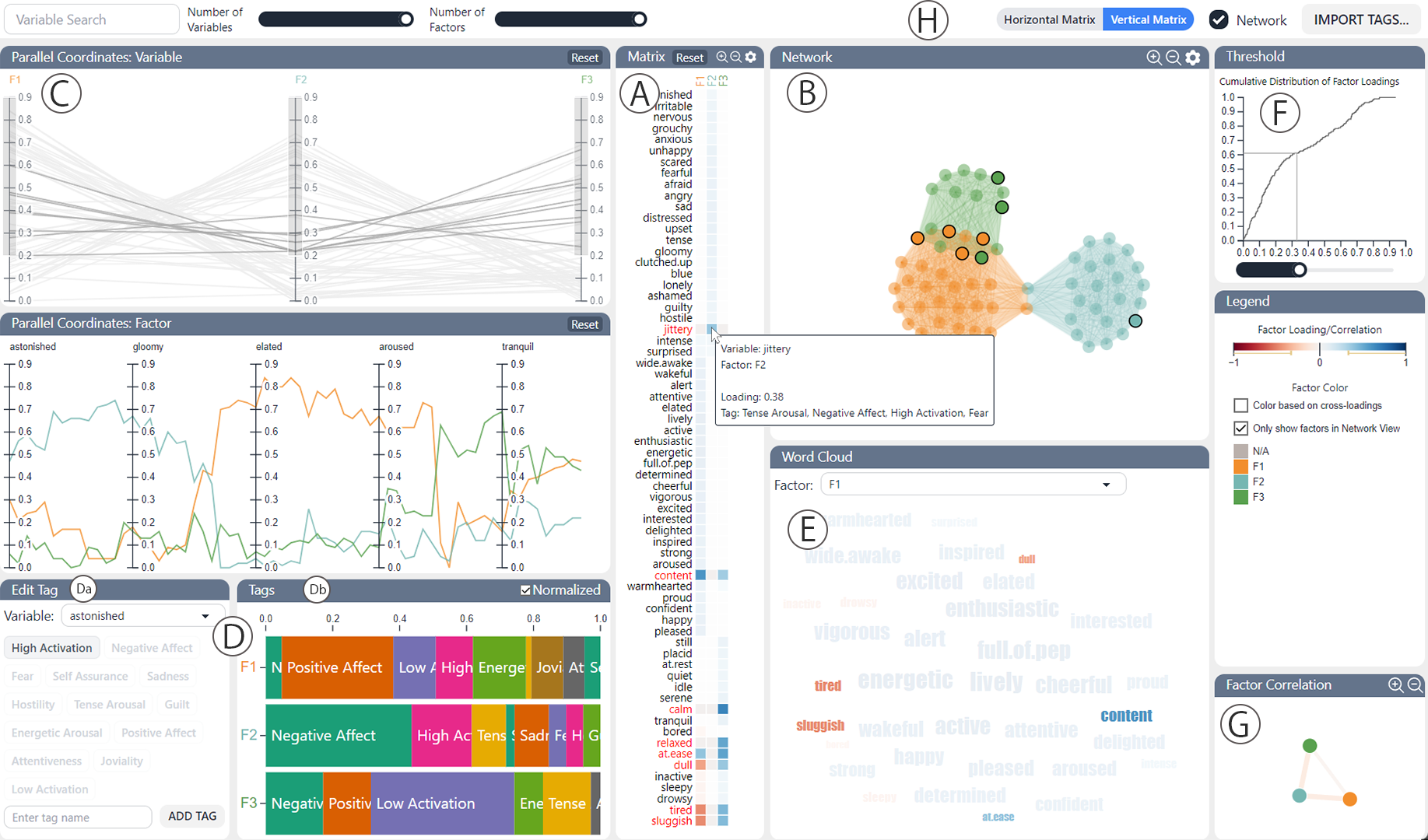
Fast forward
Keywords
Machine Learning, Statistics, Modelling, and Simulation Applications, Coordinated and Multiple Views, High-dimensional Data
Abstract
Psychological research often involves understanding psychological constructs through conducting factor analysis on data collected by a questionnaire, which can comprise hundreds of questions. Without interactive systems for interpreting factor models, researchers are frequently exposed to subjectivity, potentially leading to misinterpretations or overlooked crucial information. This paper introduces FAVis, a novel interactive visualization tool designed to aid researchers in interpreting and evaluating factor analysis results. FAVis enhances the understanding of relationships between variables and factors by supporting multiple views for visualizing factor loadings and correlations, allowing users to analyze information from various perspectives. The primary feature of FAVis is to enable users to set optimal thresholds for factor loadings to balance clarity and information retention. FAVis also allows users to assign tags to variables, enhancing the understanding of factors by linking them to their associated psychological constructs. Our user study demonstrates the utility of FAVis in various tasks.
Investigating the Apple Vision Pro Spatial Computing Platform for GPU-Based Volume Visualization
Camilla Hrycak - University of Duisburg-Essen, Duisburg, Germany
David Lewakis - University of Duisburg-Essen, Duisburg, Germany
Jens Harald Krueger - University of Duisburg-Essen, Duisburg, Germany
Download preprint PDF
Download camera-ready PDF
Room: Bayshore VI
2024-10-16T16:18:00Z GMT-0600 Change your timezone on the schedule page
2024-10-16T16:18:00Z
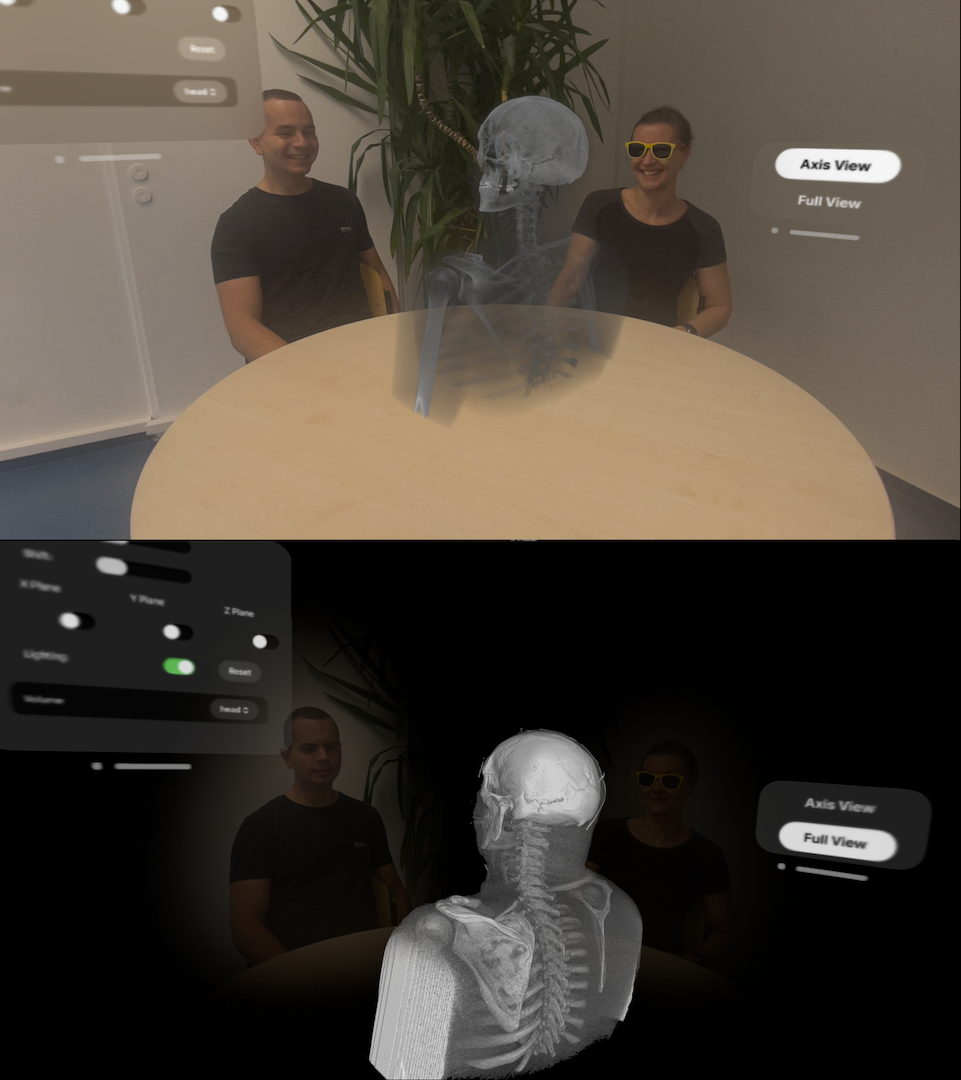
Fast forward
Keywords
Apple Vision Pro, Volume Rendering, Virtual Reality, Augmented Reality
Abstract
In this paper, we analyze the Apple Vision Pro hardware and the visionOS software platform, assessing their capabilities for volume rendering of structured grids---a prevalent technique across various applications. The Apple Vision Pro supports multiple display modes, from classical augmented reality (AR) using video see-through technology to immersive virtual reality (VR) environments that exclusively render virtual objects. These modes utilize different APIs and exhibit distinct capabilities. Our focus is on direct volume rendering, selected for its implementation challenges due to the native graphics APIs being predominantly oriented towards surface shading. Volume rendering is particularly vital in fields where AR and VR visualizations offer substantial benefits, such as in medicine and manufacturing. Despite its initial high cost, we anticipate that the Vision Pro will become more accessible and affordable over time, following Apple's track record of market expansion. As these devices become more prevalent, understanding how to effectively program and utilize them becomes increasingly important, offering significant opportunities for innovation and practical applications in various sectors.
Investigating the Apple Vision Pro Spatial Computing Platform for GPU-Based Volume Visualization
Camilla Hrycak - University of Duisburg-Essen, Duisburg, Germany
David Lewakis - University of Duisburg-Essen, Duisburg, Germany
Jens Harald Krueger - University of Duisburg-Essen, Duisburg, Germany
Download preprint PDF
Download camera-ready PDF
Room: Bayshore VI
2024-10-16T16:18:00Z GMT-0600 Change your timezone on the schedule page
2024-10-16T16:18:00Z
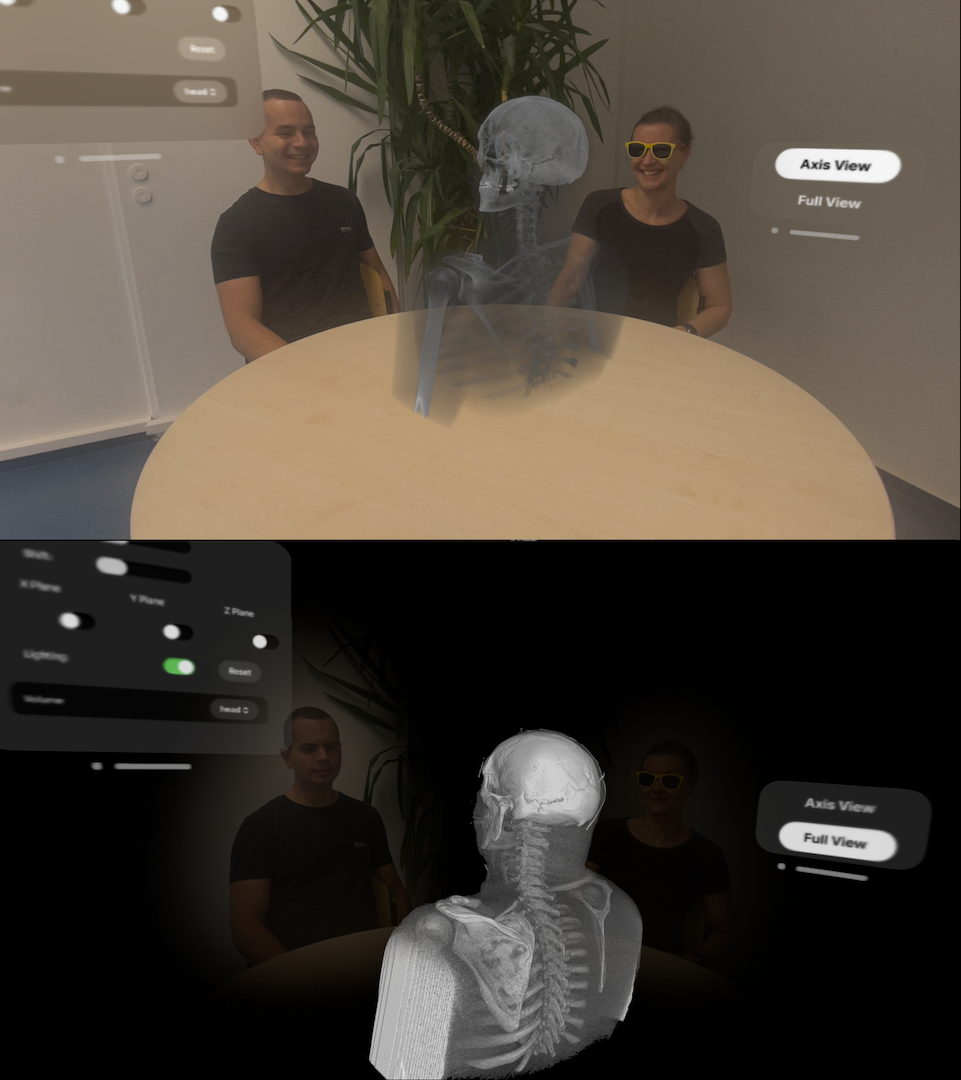
Fast forward
Keywords
Apple Vision Pro, Volume Rendering, Virtual Reality, Augmented Reality
Abstract
In this paper, we analyze the Apple Vision Pro hardware and the visionOS software platform, assessing their capabilities for volume rendering of structured grids---a prevalent technique across various applications. The Apple Vision Pro supports multiple display modes, from classical augmented reality (AR) using video see-through technology to immersive virtual reality (VR) environments that exclusively render virtual objects. These modes utilize different APIs and exhibit distinct capabilities. Our focus is on direct volume rendering, selected for its implementation challenges due to the native graphics APIs being predominantly oriented towards surface shading. Volume rendering is particularly vital in fields where AR and VR visualizations offer substantial benefits, such as in medicine and manufacturing. Despite its initial high cost, we anticipate that the Vision Pro will become more accessible and affordable over time, following Apple's track record of market expansion. As these devices become more prevalent, understanding how to effectively program and utilize them becomes increasingly important, offering significant opportunities for innovation and practical applications in various sectors.
DaVE - A Curated Database of Visualization Examples
Jens Koenen - RWTH Aachen University, Aachen, Germany
Marvin Petersen - RPTU Kaiserslautern-Landau, Kaiserslautern, Germany
Christoph Garth - RPTU Kaiserslautern-Landau, Kaiserslautern, Germany
Tim Gerrits - RWTH Aachen University, Aachen, Germany
Screen-reader Accessible PDF
Download preprint PDF
Download camera-ready PDF
Room: Bayshore VI
2024-10-16T17:45:00Z GMT-0600 Change your timezone on the schedule page
2024-10-16T17:45:00Z
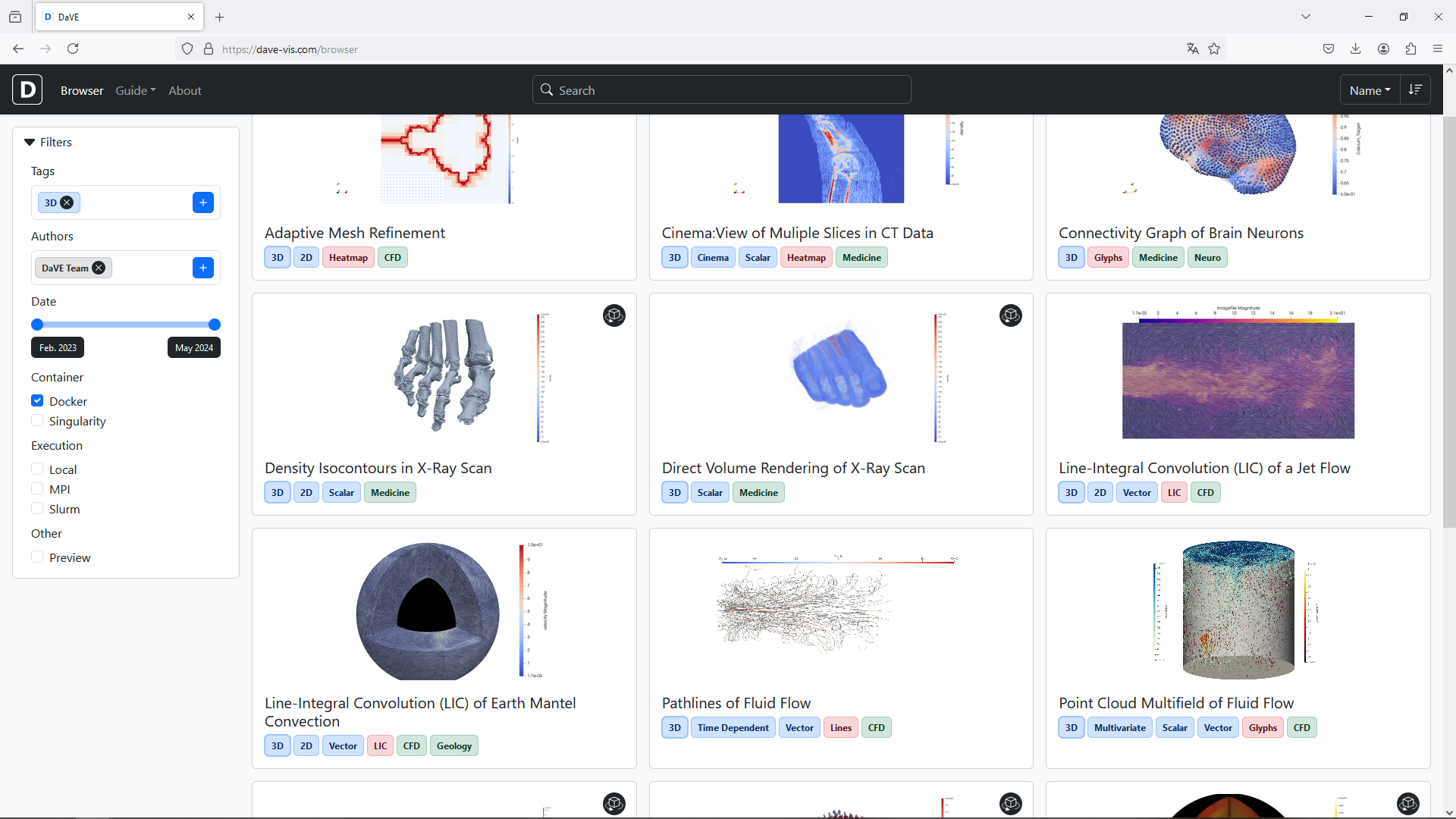
Fast forward
Keywords
Visualization, Curated Database, High-Performance Computing
Abstract
Visualization, from simple line plots to complex high-dimensional visual analysis systems, has established itself throughout numerous domains to explore, analyze, and evaluate data. Applying such visualizations in the context of simulation science where High-Performance Computing (HPC) produces ever-growing amounts of data that is more complex, potentially multidimensional, and multi-modal, takes up resources and a high level of technological experience often not available to domain experts. In this work, we present DaVE - a curated database of visualization examples, which aims to provide state-of-the-art and advanced visualization methods that arise in the context of HPC applications. Based on domain- or data-specific descriptors entered by the user, DaVE provides a list of appropriate visualization techniques, each accompanied by descriptions, examples, references, and resources. Sample code, adaptable container templates, and recipes for easy integration in HPC applications can be downloaded for easy access to high-fidelity visualizations. While the database is currently filled with a limited number of entries based on a broad evaluation of needs and challenges of current HPC users, DaVE is designed to be easily extended by experts from both the visualization and HPC communities.
DaVE - A Curated Database of Visualization Examples
Jens Koenen - RWTH Aachen University, Aachen, Germany
Marvin Petersen - RPTU Kaiserslautern-Landau, Kaiserslautern, Germany
Christoph Garth - RPTU Kaiserslautern-Landau, Kaiserslautern, Germany
Tim Gerrits - RWTH Aachen University, Aachen, Germany
Screen-reader Accessible PDF
Download preprint PDF
Download camera-ready PDF
Room: Bayshore VI
2024-10-16T17:45:00Z GMT-0600 Change your timezone on the schedule page
2024-10-16T17:45:00Z
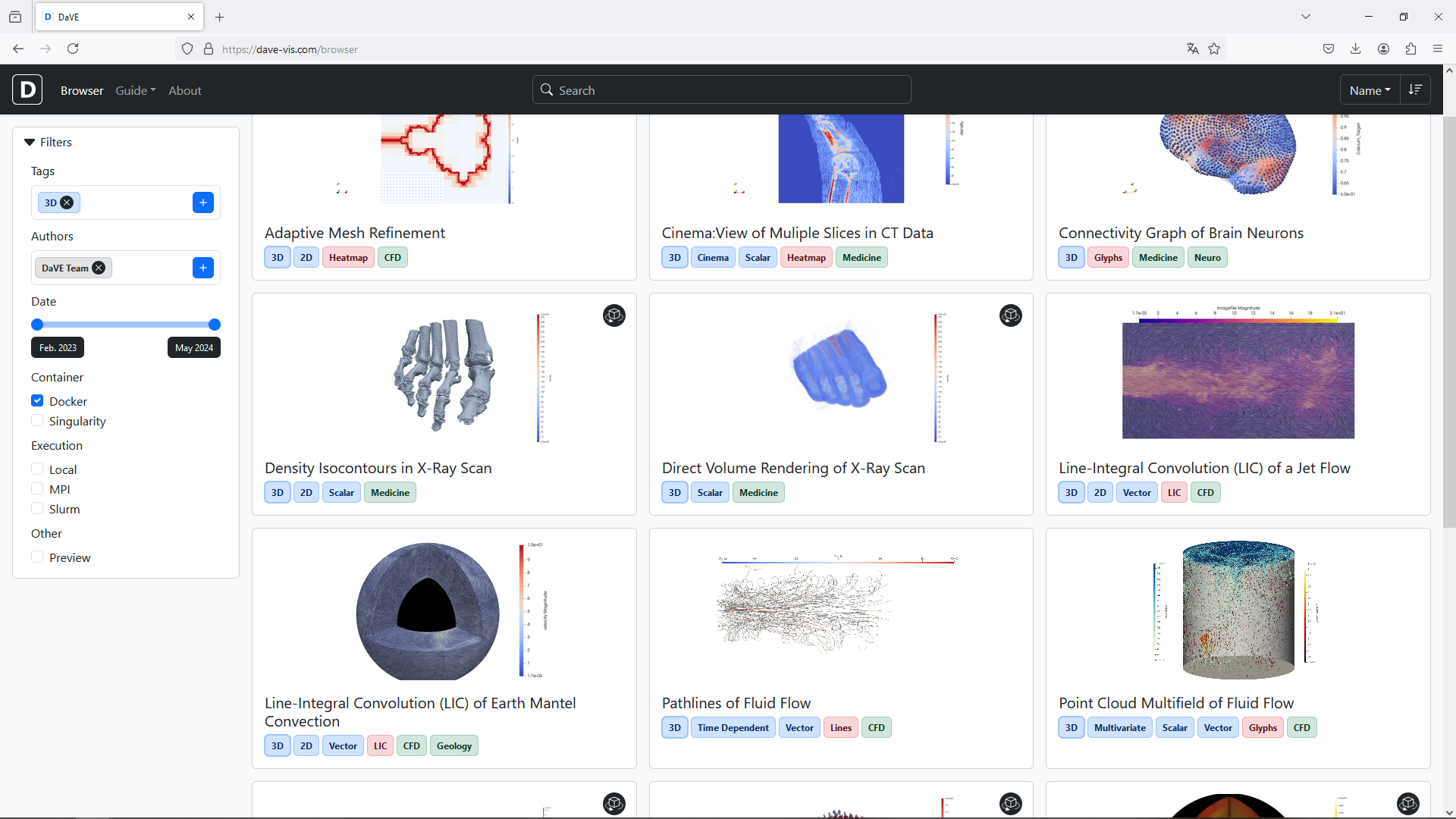
Fast forward
Keywords
Visualization, Curated Database, High-Performance Computing
Abstract
Visualization, from simple line plots to complex high-dimensional visual analysis systems, has established itself throughout numerous domains to explore, analyze, and evaluate data. Applying such visualizations in the context of simulation science where High-Performance Computing (HPC) produces ever-growing amounts of data that is more complex, potentially multidimensional, and multi-modal, takes up resources and a high level of technological experience often not available to domain experts. In this work, we present DaVE - a curated database of visualization examples, which aims to provide state-of-the-art and advanced visualization methods that arise in the context of HPC applications. Based on domain- or data-specific descriptors entered by the user, DaVE provides a list of appropriate visualization techniques, each accompanied by descriptions, examples, references, and resources. Sample code, adaptable container templates, and recipes for easy integration in HPC applications can be downloaded for easy access to high-fidelity visualizations. While the database is currently filled with a limited number of entries based on a broad evaluation of needs and challenges of current HPC users, DaVE is designed to be easily extended by experts from both the visualization and HPC communities.
Feature Clock: High-Dimensional Effects in Two-Dimensional Plots
Olga Ovcharenko - ETH Zürich, Zürich, Switzerland
Rita Sevastjanova - ETH Zürich, Zürich, Switzerland
Valentina Boeva - ETH Zurich, Zürich, Switzerland
Screen-reader Accessible PDF
Download camera-ready PDF
Download Supplemental Material
Room: Bayshore VI
2024-10-16T13:06:00Z GMT-0600 Change your timezone on the schedule page
2024-10-16T13:06:00Z
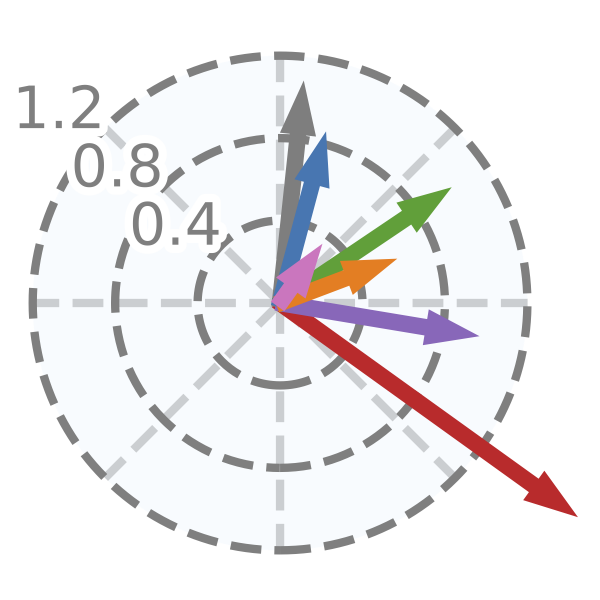
Fast forward
Keywords
High-dimensional data, nonlinear dimensionality reduction, feature importance, visualization
Abstract
Humans struggle to perceive and interpret high-dimensional data. Therefore, high-dimensional data are often projected into two dimensions for visualization. Many applications benefit from complex nonlinear dimensionality reduction techniques, but the effects of individual high-dimensional features are hard to explain in the two-dimensional space. Most visualization solutions use multiple two-dimensional plots, each showing the effect of one high-dimensional feature in two dimensions; this approach creates a need for a visual inspection of k plots for a k-dimensional input space. Our solution, Feature Clock, provides a novel approach that eliminates the need to inspect these k plots to grasp the influence of original features on the data structure depicted in two dimensions. Feature Clock enhances the explainability and compactness of visualizations of embedded data and is available in an open-source Python library.
Feature Clock: High-Dimensional Effects in Two-Dimensional Plots
Olga Ovcharenko - ETH Zürich, Zürich, Switzerland
Rita Sevastjanova - ETH Zürich, Zürich, Switzerland
Valentina Boeva - ETH Zurich, Zürich, Switzerland
Screen-reader Accessible PDF
Download camera-ready PDF
Download Supplemental Material
Room: Bayshore VI
2024-10-16T13:06:00Z GMT-0600 Change your timezone on the schedule page
2024-10-16T13:06:00Z
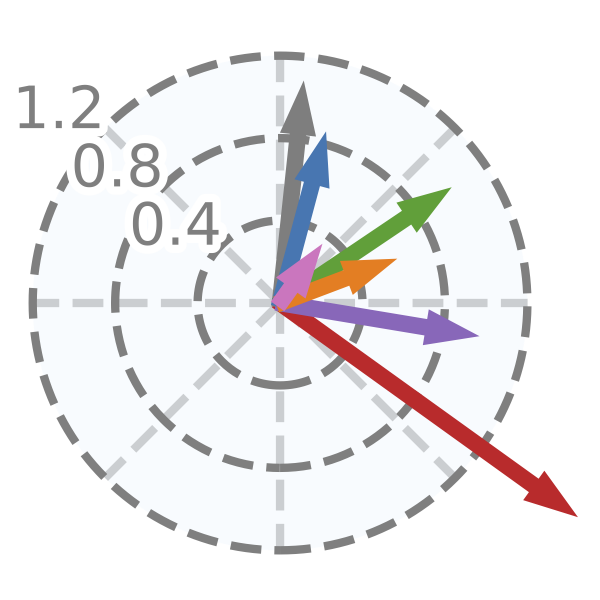
Fast forward
Keywords
High-dimensional data, nonlinear dimensionality reduction, feature importance, visualization
Abstract
Humans struggle to perceive and interpret high-dimensional data. Therefore, high-dimensional data are often projected into two dimensions for visualization. Many applications benefit from complex nonlinear dimensionality reduction techniques, but the effects of individual high-dimensional features are hard to explain in the two-dimensional space. Most visualization solutions use multiple two-dimensional plots, each showing the effect of one high-dimensional feature in two dimensions; this approach creates a need for a visual inspection of k plots for a k-dimensional input space. Our solution, Feature Clock, provides a novel approach that eliminates the need to inspect these k plots to grasp the influence of original features on the data structure depicted in two dimensions. Feature Clock enhances the explainability and compactness of visualizations of embedded data and is available in an open-source Python library.
Opening the Black Box of 3D Reconstruction Error Analysis with VECTOR
Racquel Fygenson - Northeastern University, Boston, United States
Kazi Jawad - Weta FX, Auckland, New Zealand
Zongzhan Li - Art Center, Pasadena, United States
Francois Ayoub - California Institute of Technology, Pasadena, United States
Robert G Deen - California Institute of Technology, Pasadena, United States
Scott Davidoff - California Institute of Technology, Pasadena, United States
Dominik Moritz - Carnegie Mellon University, Pittsburgh, United States
Mauricio Hess-Flores - NASA-JPL, Pasadena, United States
Download preprint PDF
Download camera-ready PDF
Download Supplemental Material
Room: Bayshore VI
2024-10-17T15:18:00Z GMT-0600 Change your timezone on the schedule page
2024-10-17T15:18:00Z
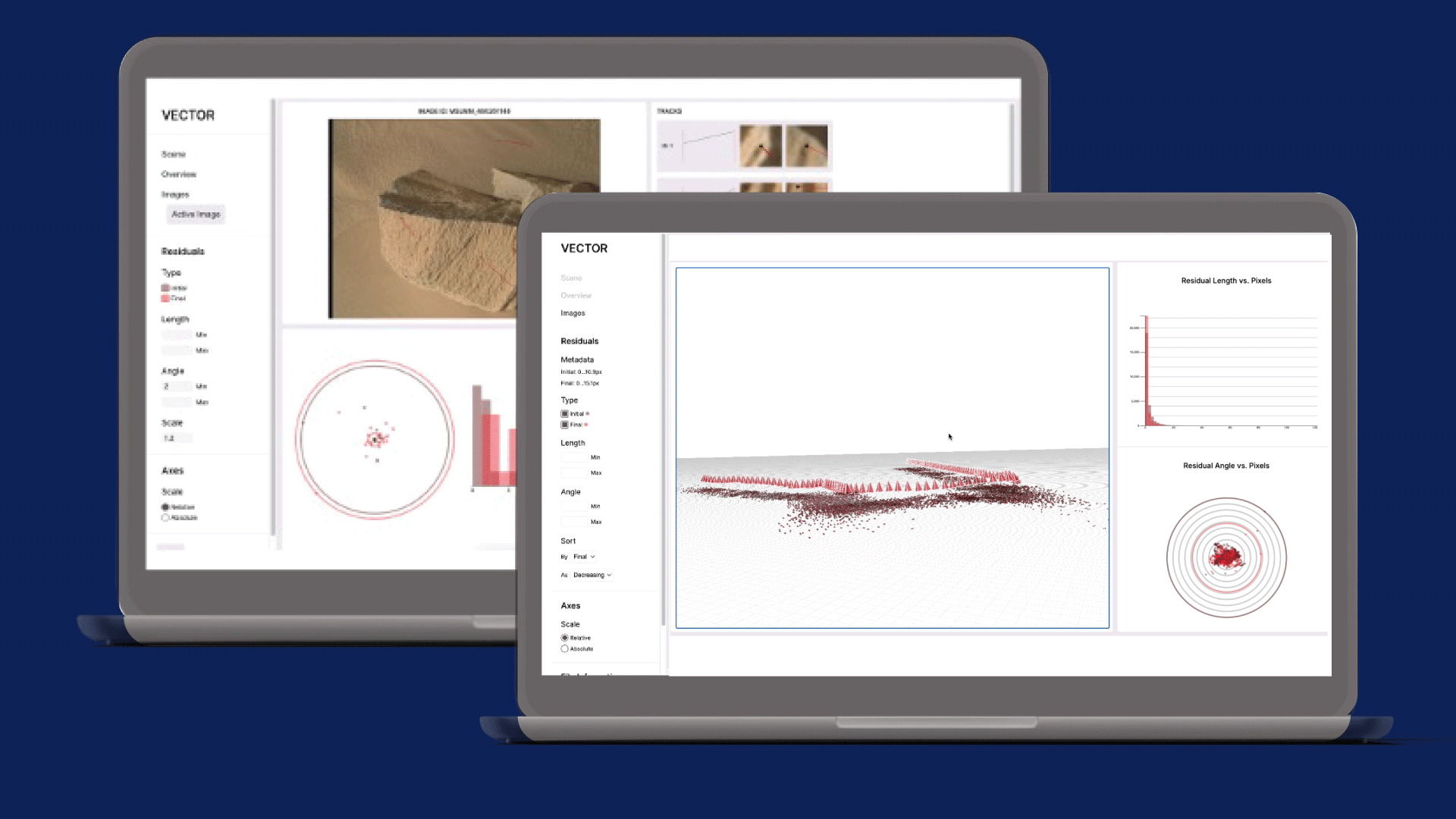
Fast forward
Keywords
Computer vision, stereo image processing, optimization, error analysis, uncertainty, SLAM, SfM, robotics
Abstract
Reconstruction of 3D scenes from 2D images is a technical challenge that impacts domains from Earth and planetary sciences and space exploration to augmented and virtual reality. Typically, reconstruction algorithms first identify common features across images and then minimize reconstruction errors after estimating the shape of the terrain. This bundle adjustment (BA) step optimizes around a single, simplifying scalar value that obfuscates many possible causes of reconstruction errors (e.g., initial estimate of the position and orientation of the camera, lighting conditions, ease of feature detection in the terrain). Reconstruction errors can lead to inaccurate scientific inferences or endanger a spacecraft exploring a remote environment. To address this challenge, we present VECTOR, a visual analysis tool that improves error inspection for stereo reconstruction BA. VECTOR provides analysts with previously unavailable visibility into feature locations, camera pose, and computed 3D points. VECTOR was developed in partnership with the Perseverance Mars Rover and Ingenuity Mars Helicopter terrain reconstruction team at the NASA Jet Propulsion Laboratory. We report on how this tool was used to debug and improve terrain reconstruction for the Mars 2020 mission.
Opening the Black Box of 3D Reconstruction Error Analysis with VECTOR
Racquel Fygenson - Northeastern University, Boston, United States
Kazi Jawad - Weta FX, Auckland, New Zealand
Zongzhan Li - Art Center, Pasadena, United States
Francois Ayoub - California Institute of Technology, Pasadena, United States
Robert G Deen - California Institute of Technology, Pasadena, United States
Scott Davidoff - California Institute of Technology, Pasadena, United States
Dominik Moritz - Carnegie Mellon University, Pittsburgh, United States
Mauricio Hess-Flores - NASA-JPL, Pasadena, United States
Download preprint PDF
Download camera-ready PDF
Download Supplemental Material
Room: Bayshore VI
2024-10-17T15:18:00Z GMT-0600 Change your timezone on the schedule page
2024-10-17T15:18:00Z
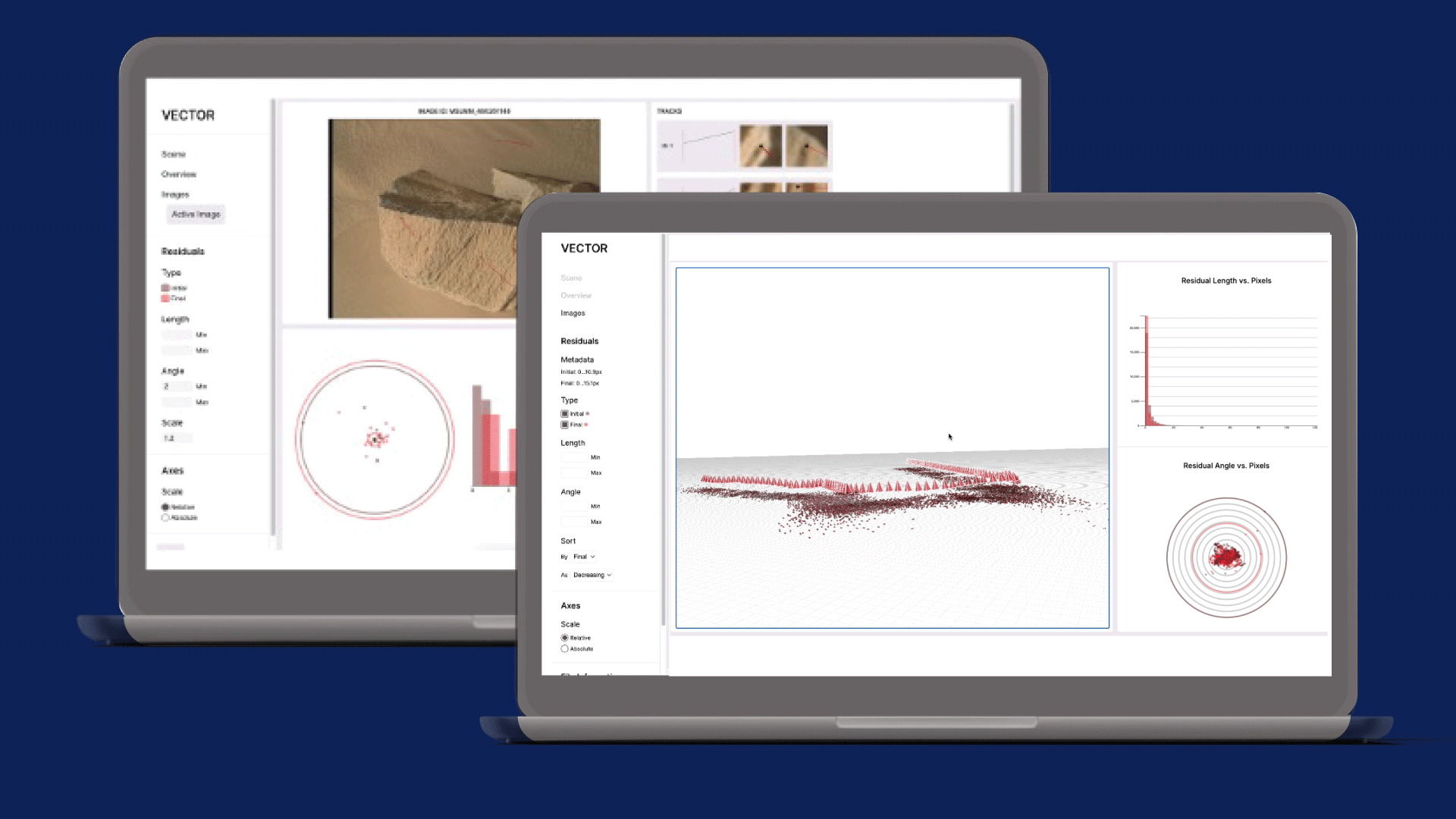
Fast forward
Keywords
Computer vision, stereo image processing, optimization, error analysis, uncertainty, SLAM, SfM, robotics
Abstract
Reconstruction of 3D scenes from 2D images is a technical challenge that impacts domains from Earth and planetary sciences and space exploration to augmented and virtual reality. Typically, reconstruction algorithms first identify common features across images and then minimize reconstruction errors after estimating the shape of the terrain. This bundle adjustment (BA) step optimizes around a single, simplifying scalar value that obfuscates many possible causes of reconstruction errors (e.g., initial estimate of the position and orientation of the camera, lighting conditions, ease of feature detection in the terrain). Reconstruction errors can lead to inaccurate scientific inferences or endanger a spacecraft exploring a remote environment. To address this challenge, we present VECTOR, a visual analysis tool that improves error inspection for stereo reconstruction BA. VECTOR provides analysts with previously unavailable visibility into feature locations, camera pose, and computed 3D points. VECTOR was developed in partnership with the Perseverance Mars Rover and Ingenuity Mars Helicopter terrain reconstruction team at the NASA Jet Propulsion Laboratory. We report on how this tool was used to debug and improve terrain reconstruction for the Mars 2020 mission.
Honorable Mention
Visualizations on Smart Watches while Running: It Actually Helps!
Sarina Kashanj - University of Victoria, Victoria, Canada
Xiyao Wang - University of Victoria, Victoira, Canada. Delft University of Technology, Delft, Netherlands
Charles Perin - University of Victoria, Victoria, Canada
Download preprint PDF
Download camera-ready PDF
Download Supplemental Material
Room: Bayshore VI
2024-10-16T18:39:00Z GMT-0600 Change your timezone on the schedule page
2024-10-16T18:39:00Z

Fast forward
Keywords
Running, Visualization, Smartwatch visualization.
Abstract
Millions of runners rely on smart watches that display running-related metrics such as pace, heart rate and distance for training and racing—mostly with text and numbers. Although research tells us that visualizations are a good alternative to text on smart watches, we know little about how visualizations can help in realistic running scenarios. We conducted a study in which 20 runners completed running-related tasks on an outdoor track using both text and visualizations. Our results show that runners are 1.5 to 8 times faster in completing those tasks with visualizations than with text, prefer visualizations to text, and would use such visualizations while running — if available on their smart watch.
Honorable Mention
Visualizations on Smart Watches while Running: It Actually Helps!
Sarina Kashanj - University of Victoria, Victoria, Canada
Xiyao Wang - University of Victoria, Victoira, Canada. Delft University of Technology, Delft, Netherlands
Charles Perin - University of Victoria, Victoria, Canada
Download preprint PDF
Download camera-ready PDF
Download Supplemental Material
Room: Bayshore VI
2024-10-16T18:39:00Z GMT-0600 Change your timezone on the schedule page
2024-10-16T18:39:00Z

Fast forward
Keywords
Running, Visualization, Smartwatch visualization.
Abstract
Millions of runners rely on smart watches that display running-related metrics such as pace, heart rate and distance for training and racing—mostly with text and numbers. Although research tells us that visualizations are a good alternative to text on smart watches, we know little about how visualizations can help in realistic running scenarios. We conducted a study in which 20 runners completed running-related tasks on an outdoor track using both text and visualizations. Our results show that runners are 1.5 to 8 times faster in completing those tasks with visualizations than with text, prefer visualizations to text, and would use such visualizations while running — if available on their smart watch.
Best Paper Award
PyGWalker: On-the-fly Assistant for Exploratory Visual Data Analysis
Yue Yu - The Hong Kong University of Science and Technology, Hong Kong, China. Kanaries Data Inc., Hangzhou, China
Leixian Shen - The Hong Kong University of Science and Technology, Hong Kong, China
Fei Long - Kanaries Data Inc., Hangzhou, China
Huamin Qu - The Hong Kong University of Science and Technology, Hong Kong, China
Hao Chen - Kanaries Data Inc., Hangzhou, China
Download preprint PDF
Download camera-ready PDF
Room: Bayshore I + II + III
2024-10-15T15:21:00Z GMT-0600 Change your timezone on the schedule page
2024-10-15T15:21:00Z
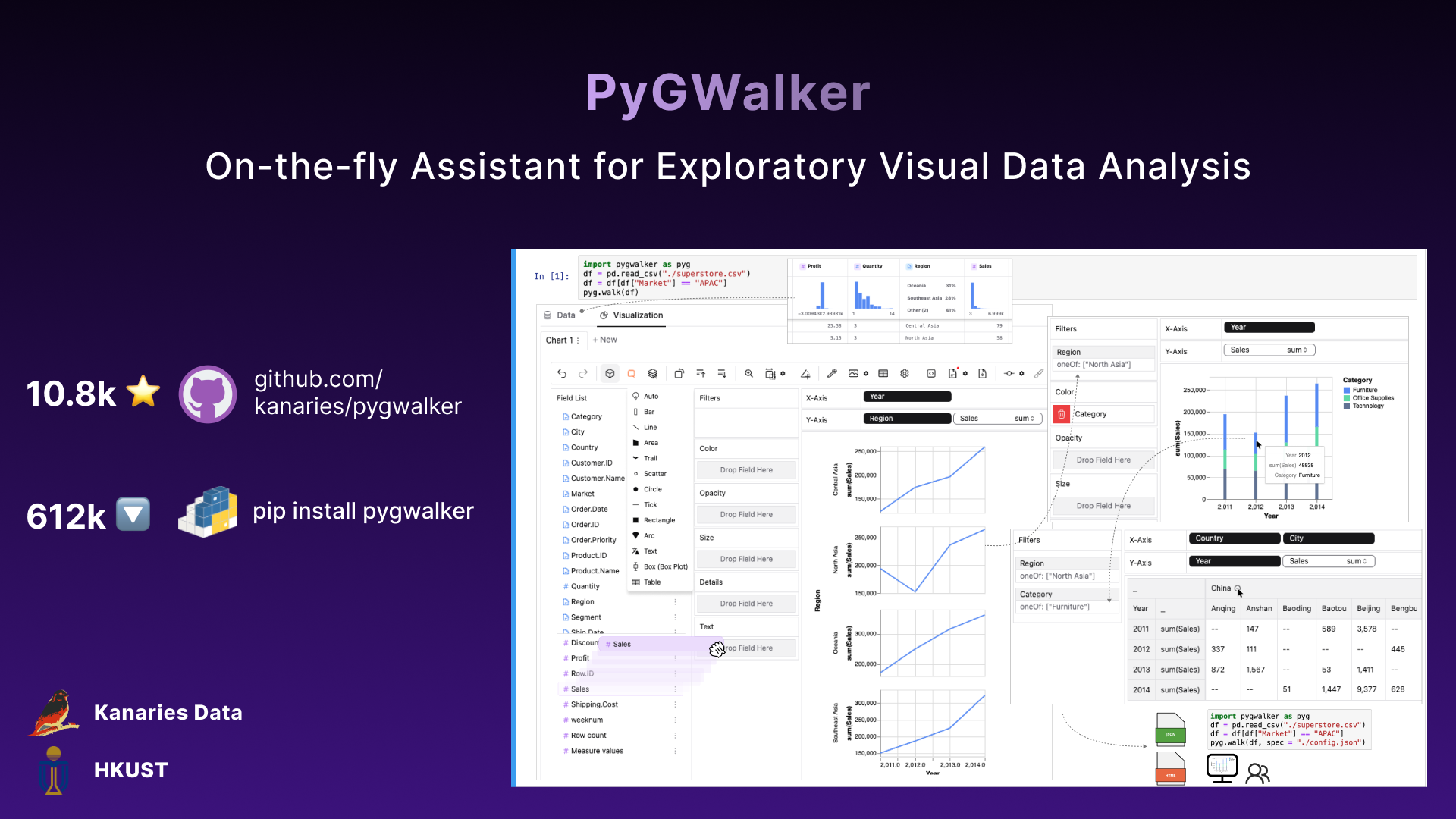
Fast forward
Keywords
Data Visualization; Exploratory Data Analysis; Computational Notebooks
Abstract
Exploratory visual data analysis tools empower data analysts to efficiently and intuitively explore data insights throughout the entire analysis cycle. However, the gap between common programmatic analysis (e.g., within computational notebooks) and exploratory visual analysis leads to a disjointed and inefficient data analysis experience. To bridge this gap, we developed PyGWalker, a Python library that offers on-the-fly assistance for exploratory visual data analysis. It features a lightweight and intuitive GUI with a shelf builder modality. Its loosely coupled architecture supports multiple computational environments to accommodate varying data sizes. Since its release in February 2023, PyGWalker has gained much attention, with 612k downloads on PyPI and over 10.5k stars on GitHub as of June 2024. This demonstrates its value to the data science and visualization community, with researchers and developers integrating it into their own applications and studies.
Best Paper Award
PyGWalker: On-the-fly Assistant for Exploratory Visual Data Analysis
Yue Yu - The Hong Kong University of Science and Technology, Hong Kong, China. Kanaries Data Inc., Hangzhou, China
Leixian Shen - The Hong Kong University of Science and Technology, Hong Kong, China
Fei Long - Kanaries Data Inc., Hangzhou, China
Huamin Qu - The Hong Kong University of Science and Technology, Hong Kong, China
Hao Chen - Kanaries Data Inc., Hangzhou, China
Download preprint PDF
Download camera-ready PDF
Room: Bayshore I + II + III
2024-10-15T15:21:00Z GMT-0600 Change your timezone on the schedule page
2024-10-15T15:21:00Z
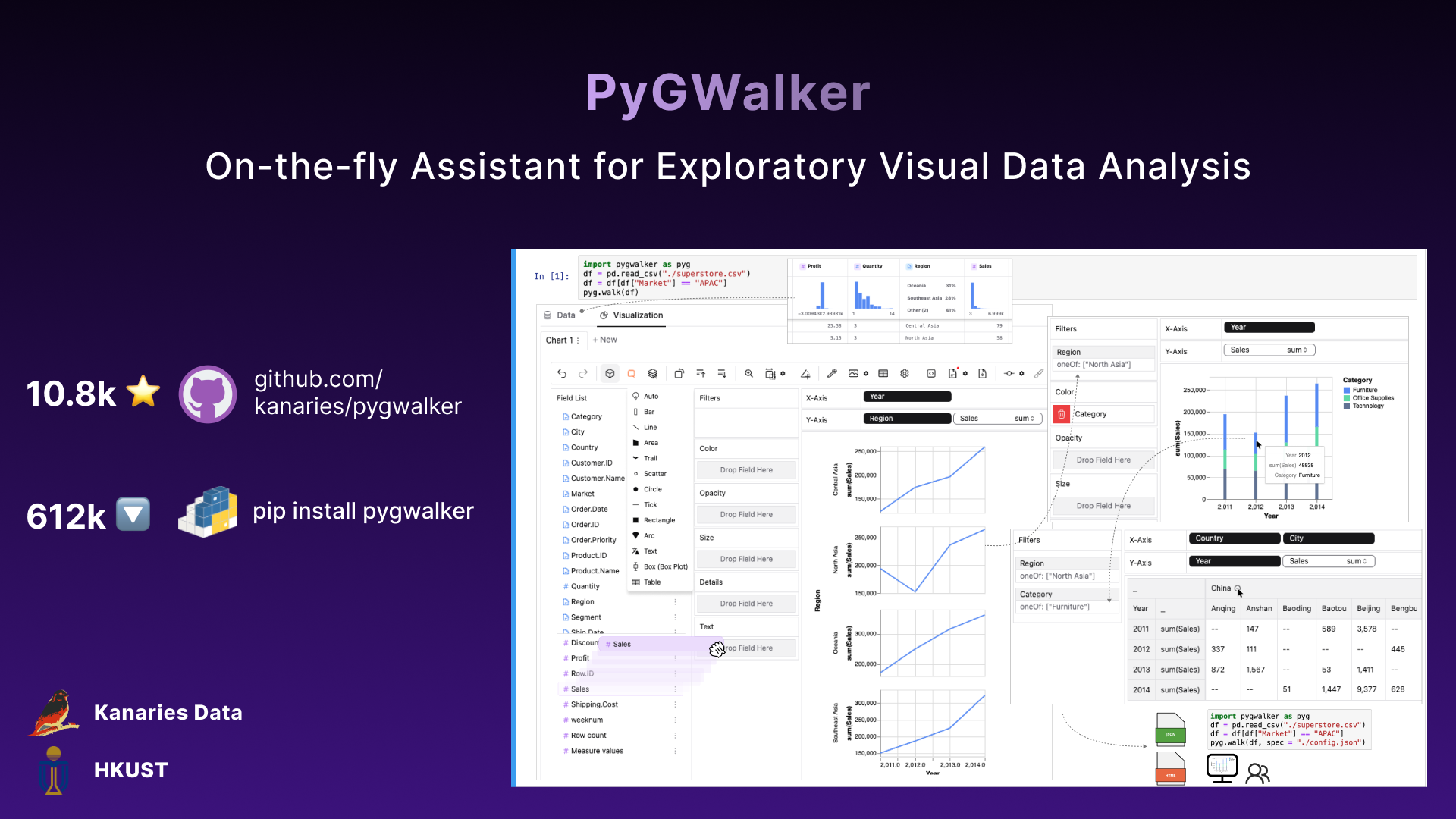
Fast forward
Keywords
Data Visualization; Exploratory Data Analysis; Computational Notebooks
Abstract
Exploratory visual data analysis tools empower data analysts to efficiently and intuitively explore data insights throughout the entire analysis cycle. However, the gap between common programmatic analysis (e.g., within computational notebooks) and exploratory visual analysis leads to a disjointed and inefficient data analysis experience. To bridge this gap, we developed PyGWalker, a Python library that offers on-the-fly assistance for exploratory visual data analysis. It features a lightweight and intuitive GUI with a shelf builder modality. Its loosely coupled architecture supports multiple computational environments to accommodate varying data sizes. Since its release in February 2023, PyGWalker has gained much attention, with 612k downloads on PyPI and over 10.5k stars on GitHub as of June 2024. This demonstrates its value to the data science and visualization community, with researchers and developers integrating it into their own applications and studies.
Active Appearance and Spatial Variation Can Improve Visibility in Area Labels for Augmented Reality
Hojung Kwon - Brown University, Providence, United States
Yuanbo Li - Brown University, Providence, United States
Xiaohan Ye - Brown University, Providence, United States
Praccho Muna-McQuay - Brown University, Providence, United States
Liuren Yin - Duke University, Durham, United States
James Tompkin - Brown University, Providence, United States
Download camera-ready PDF
Room: Bayshore VI
2024-10-16T17:03:00Z GMT-0600 Change your timezone on the schedule page
2024-10-16T17:03:00Z
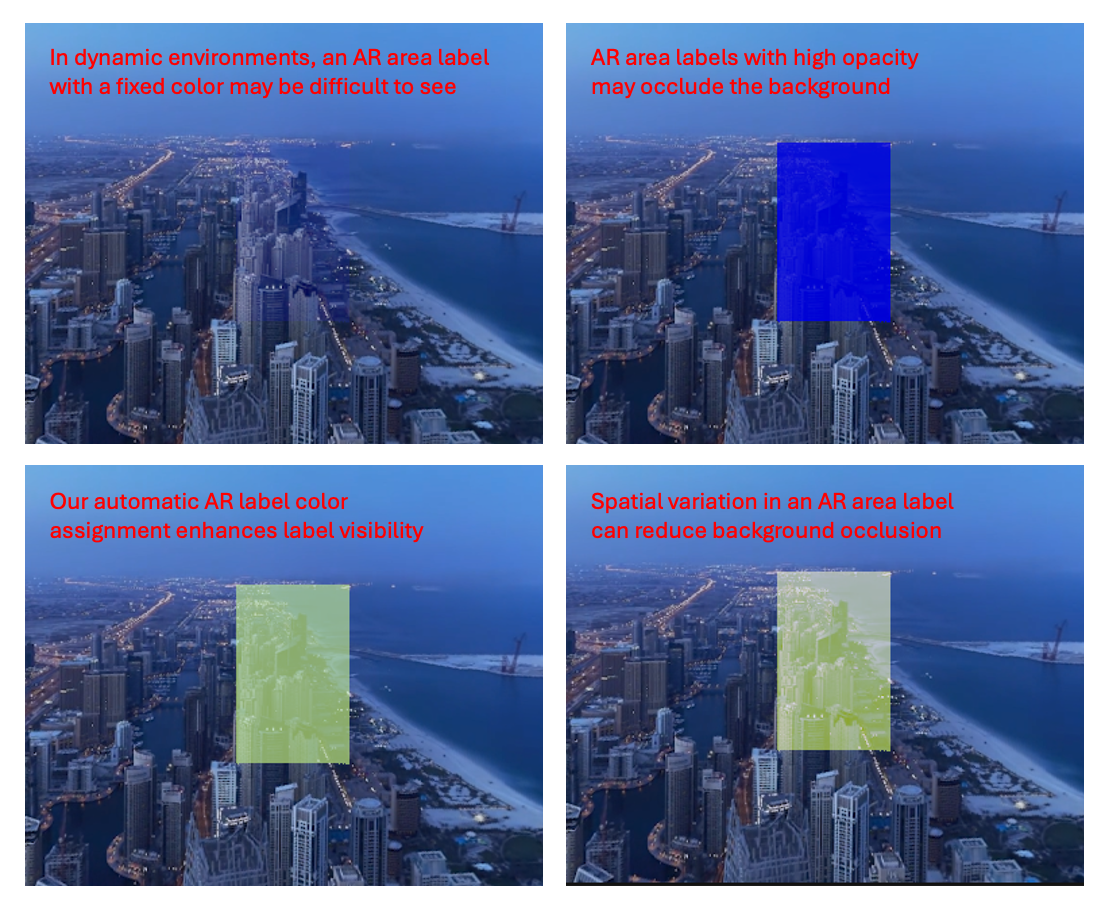
Fast forward
Keywords
Augmented reality, active labels, environment-adaptive
Abstract
Augmented reality (AR) area labels can visualize real world regions with arbitrary boundaries and show invisible objects or features. But environment conditions such as lighting and clutter can decrease fixed or passive label visibility, and labels that have high opacity levels can occlude crucial details in the environment. We design and evaluate active AR area label visualization modes to enhance visibility across real-life environments, while still retaining environment details within the label. For this, we define a distant characteristic color from the environment in perceptual CIELAB space, then introduce spatial variations among label pixel colors based on the underlying environment variation. In a user study with 18 participants, we found that our active label visualization modes can be comparable in visibility to a fixed green baseline by Gabbard et al., and can outperform it with added spatial variation in cluttered environments, across varying levels of lighting (e.g., nighttime), and in environments with colors similar to the fixed baseline color.
Active Appearance and Spatial Variation Can Improve Visibility in Area Labels for Augmented Reality
Hojung Kwon - Brown University, Providence, United States
Yuanbo Li - Brown University, Providence, United States
Xiaohan Ye - Brown University, Providence, United States
Praccho Muna-McQuay - Brown University, Providence, United States
Liuren Yin - Duke University, Durham, United States
James Tompkin - Brown University, Providence, United States
Download camera-ready PDF
Room: Bayshore VI
2024-10-16T17:03:00Z GMT-0600 Change your timezone on the schedule page
2024-10-16T17:03:00Z
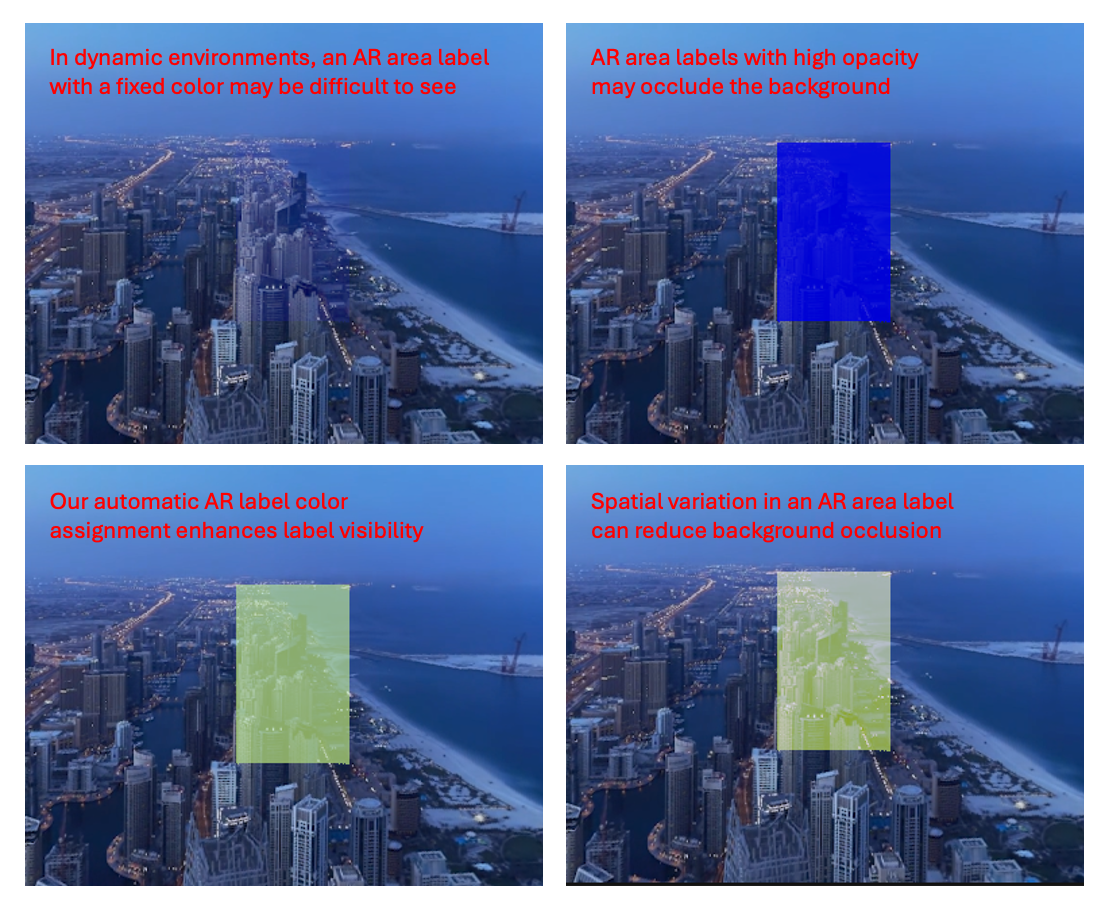
Fast forward
Keywords
Augmented reality, active labels, environment-adaptive
Abstract
Augmented reality (AR) area labels can visualize real world regions with arbitrary boundaries and show invisible objects or features. But environment conditions such as lighting and clutter can decrease fixed or passive label visibility, and labels that have high opacity levels can occlude crucial details in the environment. We design and evaluate active AR area label visualization modes to enhance visibility across real-life environments, while still retaining environment details within the label. For this, we define a distant characteristic color from the environment in perceptual CIELAB space, then introduce spatial variations among label pixel colors based on the underlying environment variation. In a user study with 18 participants, we found that our active label visualization modes can be comparable in visibility to a fixed green baseline by Gabbard et al., and can outperform it with added spatial variation in cluttered environments, across varying levels of lighting (e.g., nighttime), and in environments with colors similar to the fixed baseline color.
An Overview + Detail Layout for Visualizing Compound Graphs
Chang Han - University of Utah, Salt Lake City, United States
Justin Lieffers - University of Arizona, Tucson, United States
Clayton Morrison - University of Arizona, Tucson, United States
Katherine E. Isaacs - The University of Utah, Salt Lake City, United States
Download preprint PDF
Download camera-ready PDF
Room: Bayshore VI
2024-10-16T12:39:00Z GMT-0600 Change your timezone on the schedule page
2024-10-16T12:39:00Z
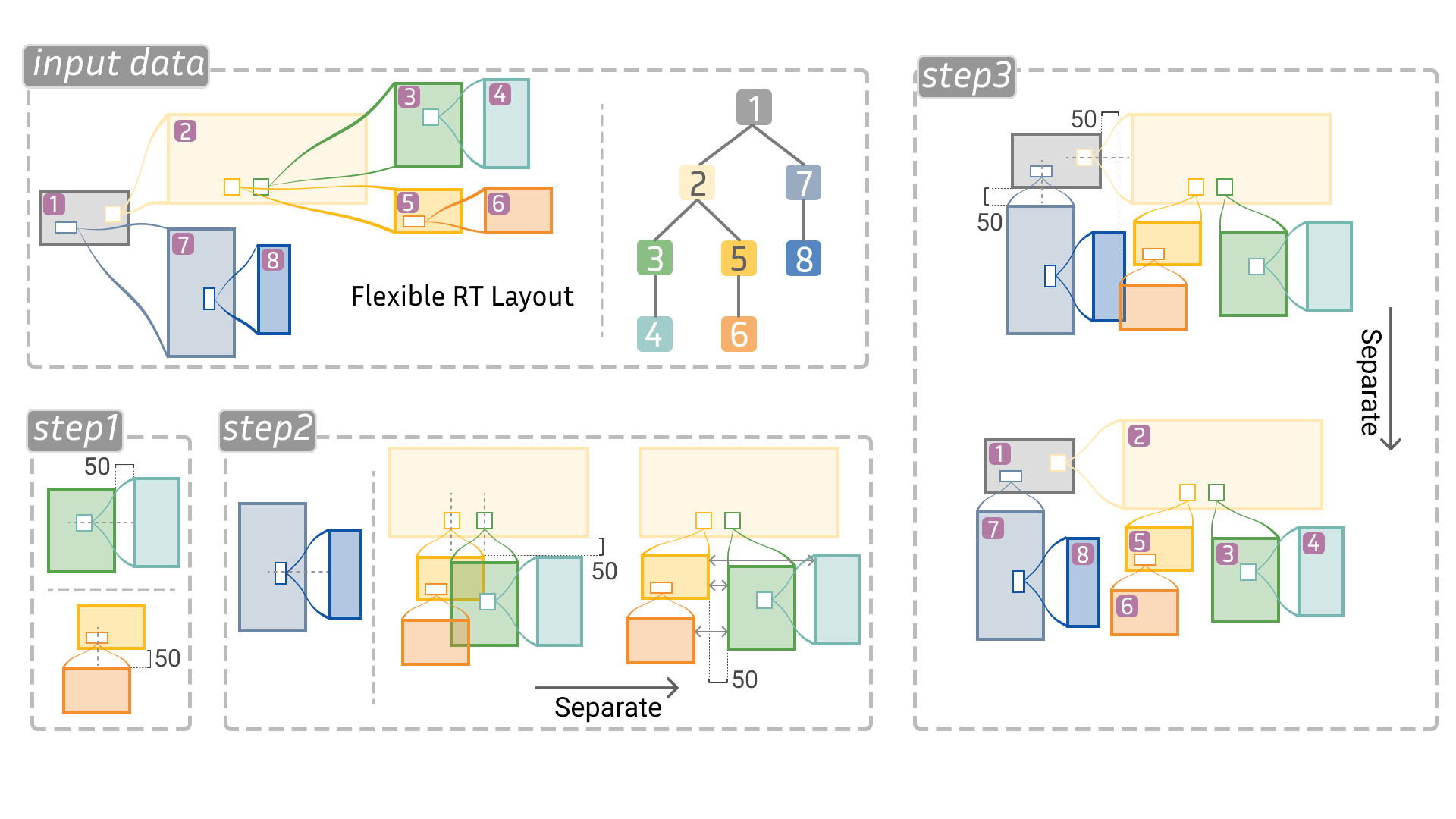
Fast forward
Keywords
compound graphs, network layout, graph drawing, network visualization, graph visualization
Abstract
Compound graphs are networks in which vertices can be grouped into larger subsets, with these subsets capable of further grouping, resulting in a nesting that can be many levels deep. In several applications, including biological workflows, chemical equations, and computational data flow analysis, these graphs often exhibit a tree-like nesting structure, where sibling clusters are disjoint. Common compound graph layouts prioritize the lowest level of the grouping, down to the individual ungrouped vertices, which can make the higher level grouped structures more difficult to discern, especially in deeply nested networks. Leveraging the additional structure of the tree-like nesting, we contribute an overview+detail layout for this class of compound graphs that preserves the saliency of the higher level network structure when groups are expanded to show internal nested structure. Our layout draws inner structures adjacent to their parents, using a modified tree layout to place substructures. We describe our algorithm and then present case studies demonstrating the layout's utility to a domain expert working on data flow analysis. Finally, we discuss network parameters and analysis situations in which our layout is well suited.
An Overview + Detail Layout for Visualizing Compound Graphs
Chang Han - University of Utah, Salt Lake City, United States
Justin Lieffers - University of Arizona, Tucson, United States
Clayton Morrison - University of Arizona, Tucson, United States
Katherine E. Isaacs - The University of Utah, Salt Lake City, United States
Download preprint PDF
Download camera-ready PDF
Room: Bayshore VI
2024-10-16T12:39:00Z GMT-0600 Change your timezone on the schedule page
2024-10-16T12:39:00Z
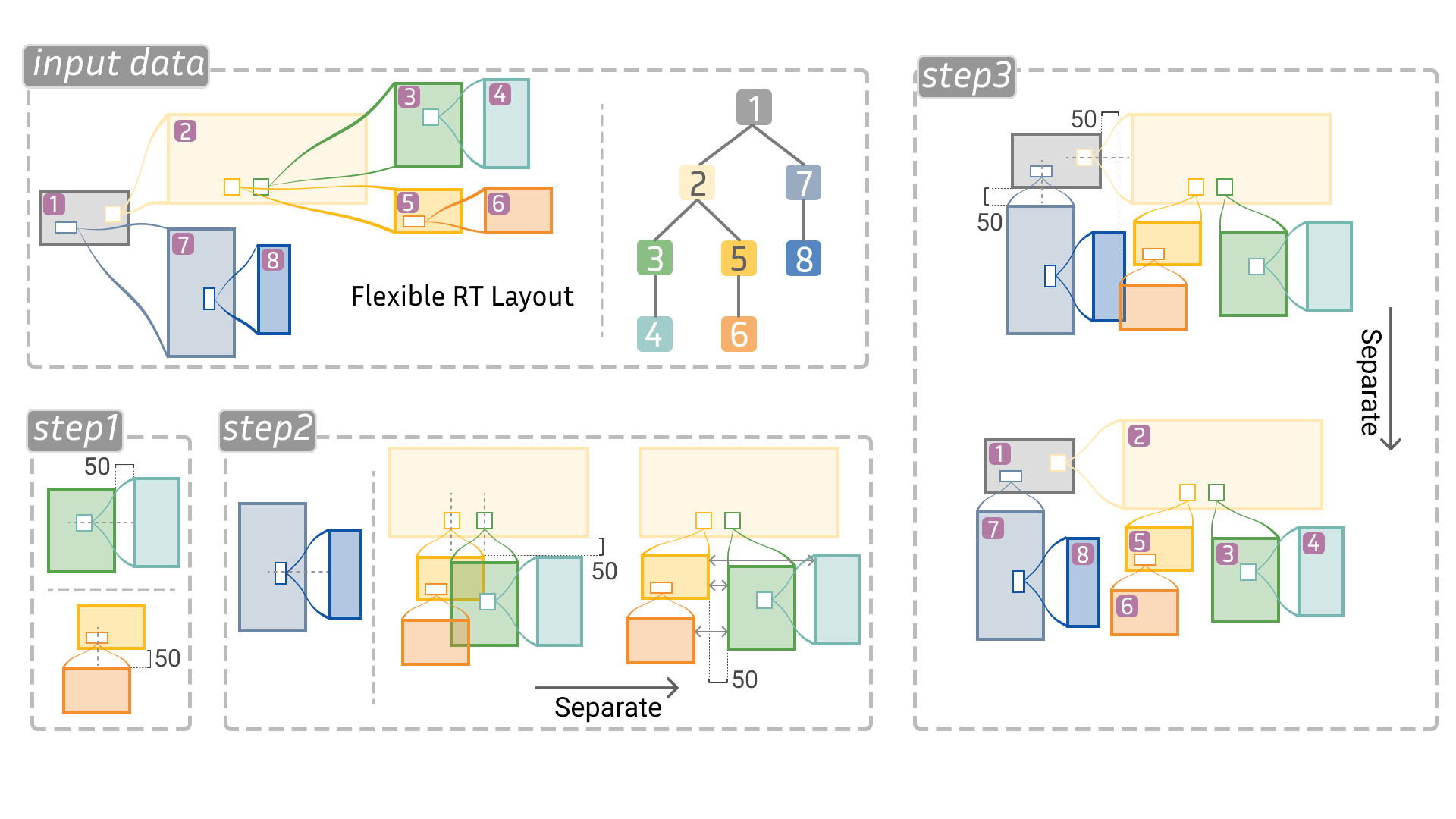
Fast forward
Keywords
compound graphs, network layout, graph drawing, network visualization, graph visualization
Abstract
Compound graphs are networks in which vertices can be grouped into larger subsets, with these subsets capable of further grouping, resulting in a nesting that can be many levels deep. In several applications, including biological workflows, chemical equations, and computational data flow analysis, these graphs often exhibit a tree-like nesting structure, where sibling clusters are disjoint. Common compound graph layouts prioritize the lowest level of the grouping, down to the individual ungrouped vertices, which can make the higher level grouped structures more difficult to discern, especially in deeply nested networks. Leveraging the additional structure of the tree-like nesting, we contribute an overview+detail layout for this class of compound graphs that preserves the saliency of the higher level network structure when groups are expanded to show internal nested structure. Our layout draws inner structures adjacent to their parents, using a modified tree layout to place substructures. We describe our algorithm and then present case studies demonstrating the layout's utility to a domain expert working on data flow analysis. Finally, we discuss network parameters and analysis situations in which our layout is well suited.
Micro Visualizations on a Smartwatch: Assessing Reading Performance While Walking
Fairouz Grioui - University of Stuttgart, Stuttgart, Germany
Tanja Blascheck - University of Stuttgart, Stuttgart, Germany
Lijie Yao - Université Paris-Saclay, CNRS, Orsay, France. Inria, Saclay, France
Petra Isenberg - Université Paris-Saclay, CNRS, Orsay, France. Inria, Saclay, France
Download preprint PDF
Download camera-ready PDF
Download Supplemental Material
Room: Bayshore VI
2024-10-16T18:48:00Z GMT-0600 Change your timezone on the schedule page
2024-10-16T18:48:00Z

Fast forward
Keywords
micro and mobile visualization, smartwatch
Abstract
With two studies, we assess how different walking trajectories (straight line, circular, and infinity) and speeds (2 km/h, 4 km/h, and 6 km/h) influence the accuracy and response time of participants reading micro visualizations on a smartwatch. We showed our participants common watch face micro visualizations including date, time, weather information, and four complications showing progress charts of fitness data. Our findings suggest that while walking trajectories did not significantly affect reading performance, overall walking activity, especially at high speeds, hurt reading accuracy and, to some extent, response time.
Micro Visualizations on a Smartwatch: Assessing Reading Performance While Walking
Fairouz Grioui - University of Stuttgart, Stuttgart, Germany
Tanja Blascheck - University of Stuttgart, Stuttgart, Germany
Lijie Yao - Université Paris-Saclay, CNRS, Orsay, France. Inria, Saclay, France
Petra Isenberg - Université Paris-Saclay, CNRS, Orsay, France. Inria, Saclay, France
Download preprint PDF
Download camera-ready PDF
Download Supplemental Material
Room: Bayshore VI
2024-10-16T18:48:00Z GMT-0600 Change your timezone on the schedule page
2024-10-16T18:48:00Z

Fast forward
Keywords
micro and mobile visualization, smartwatch
Abstract
With two studies, we assess how different walking trajectories (straight line, circular, and infinity) and speeds (2 km/h, 4 km/h, and 6 km/h) influence the accuracy and response time of participants reading micro visualizations on a smartwatch. We showed our participants common watch face micro visualizations including date, time, weather information, and four complications showing progress charts of fitness data. Our findings suggest that while walking trajectories did not significantly affect reading performance, overall walking activity, especially at high speeds, hurt reading accuracy and, to some extent, response time.
Visualizing an Exascale Data Center Digital Twin: Considerations, Challenges and Opportunities
Matthias Maiterth - Oak Ridge National Laboratory, Oak Ridge, United States
Wes Brewer - Oak Ridge National Laboratory, Oak Ridge, United States
Dane De Wet - Oak Ridge National Laboratory, Oak Ridge, United States
Scott Greenwood - Oak Ridge National Laboratory, Oak Ridge, United States
Vineet Kumar - Oak Ridge National Laboratory, Oak Ridge, United States
Jesse Hines - Oak Ridge National Laboratory, Oak Ridge, United States
Sedrick L Bouknight - Oak Ridge National Laboratory, Oak Ridge, United States
Zhe Wang - Oak Ridge National Laboratory, Oak Ridge, United States
Tim Dykes - Hewlett Packard Enterprise, Berkshire, United Kingdom
Feiyi Wang - Oak Ridge National Laboratory, Oak Ridge, United States
Download camera-ready PDF
Download Supplemental Material
Room: Bayshore VI
2024-10-16T18:03:00Z GMT-0600 Change your timezone on the schedule page
2024-10-16T18:03:00Z

Fast forward
Keywords
Digital Twin, Data Center, Information Representation, Massively Parallel Systems, Operational Data Analytics, Simulation, Augmented Reality
Abstract
Digital twins are an excellent tool to model, visualize, and simulate complex systems, to understand and optimize their operation. In this work, we present the technical challenges of real-time visualization of a digital twin of the Frontier supercomputer. We show the initial prototype and current state of the twin and highlight technical design challenges of visualizing such a large High Performance Computing (HPC) system. The goal is to understand the use of augmented reality as a primary way to extract information and collaborate on digital twins of complex systems. This leverages the spatio-temporal aspect of a 3D representation of a digital twin, with the ability to view historical and real-time telemetry, triggering simulations of a system state and viewing the results, which can be augmented via dashboards for details. Finally, we discuss considerations and opportunities for augmented reality of digital twins of large-scale, parallel computers.
Visualizing an Exascale Data Center Digital Twin: Considerations, Challenges and Opportunities
Matthias Maiterth - Oak Ridge National Laboratory, Oak Ridge, United States
Wes Brewer - Oak Ridge National Laboratory, Oak Ridge, United States
Dane De Wet - Oak Ridge National Laboratory, Oak Ridge, United States
Scott Greenwood - Oak Ridge National Laboratory, Oak Ridge, United States
Vineet Kumar - Oak Ridge National Laboratory, Oak Ridge, United States
Jesse Hines - Oak Ridge National Laboratory, Oak Ridge, United States
Sedrick L Bouknight - Oak Ridge National Laboratory, Oak Ridge, United States
Zhe Wang - Oak Ridge National Laboratory, Oak Ridge, United States
Tim Dykes - Hewlett Packard Enterprise, Berkshire, United Kingdom
Feiyi Wang - Oak Ridge National Laboratory, Oak Ridge, United States
Download camera-ready PDF
Download Supplemental Material
Room: Bayshore VI
2024-10-16T18:03:00Z GMT-0600 Change your timezone on the schedule page
2024-10-16T18:03:00Z

Fast forward
Keywords
Digital Twin, Data Center, Information Representation, Massively Parallel Systems, Operational Data Analytics, Simulation, Augmented Reality
Abstract
Digital twins are an excellent tool to model, visualize, and simulate complex systems, to understand and optimize their operation. In this work, we present the technical challenges of real-time visualization of a digital twin of the Frontier supercomputer. We show the initial prototype and current state of the twin and highlight technical design challenges of visualizing such a large High Performance Computing (HPC) system. The goal is to understand the use of augmented reality as a primary way to extract information and collaborate on digital twins of complex systems. This leverages the spatio-temporal aspect of a 3D representation of a digital twin, with the ability to view historical and real-time telemetry, triggering simulations of a system state and viewing the results, which can be augmented via dashboards for details. Finally, we discuss considerations and opportunities for augmented reality of digital twins of large-scale, parallel computers.
Curve Segment Neighborhood-based Vector Field Exploration
Nguyen K Phan - University of Houston, Houston, United States
Guoning Chen - University of Houston, Houston, United States
Download camera-ready PDF
Download Supplemental Material
Room: Bayshore VI
2024-10-18T13:30:00Z GMT-0600 Change your timezone on the schedule page
2024-10-18T13:30:00Z
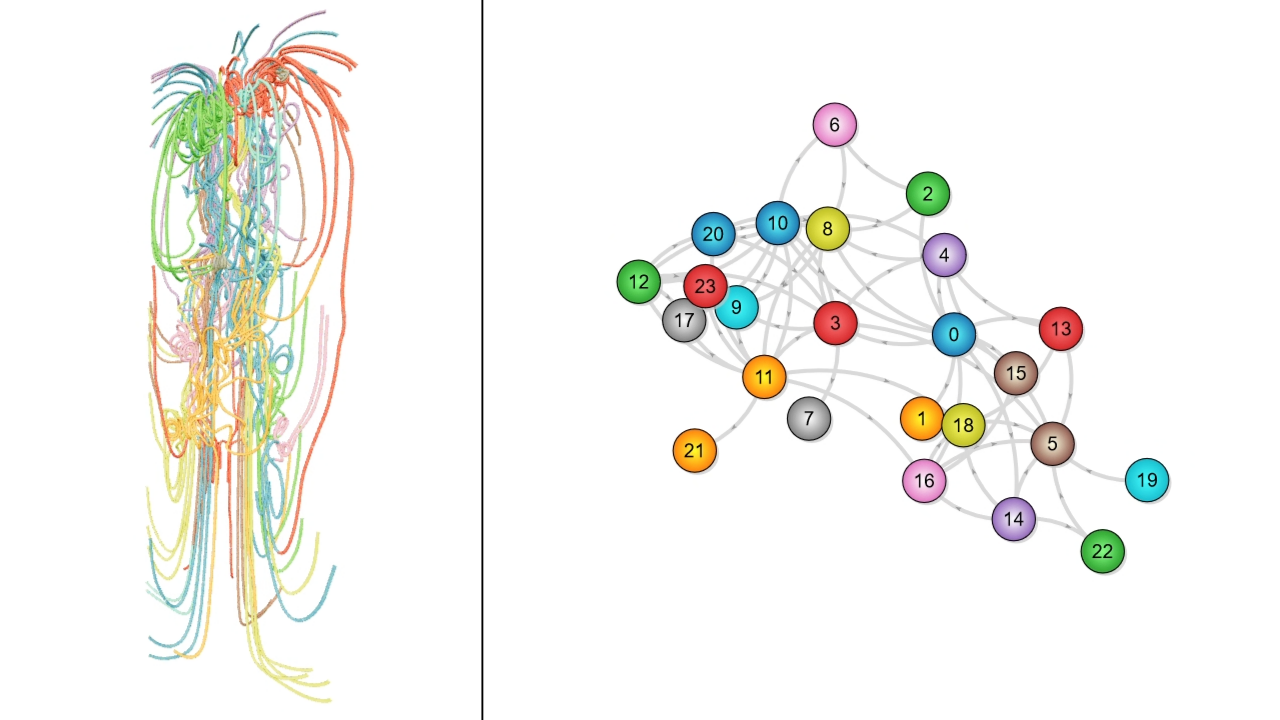
Fast forward
Keywords
Vector field, neighbor search, community detection
Abstract
Integral curves have been widely used to represent and analyze various vector fields. In this paper, we propose a Curve Segment Neighborhood Graph (CSNG) to capture the relationships between neighboring curve segments. This graph representation enables us to adapt the fast community detection algorithm, i.e., the Louvain algorithm, to identify individual graph communities from CSNG. Our results show that these communities often correspond to the features of the flow. To achieve a multi-level interactive exploration of the detected communities, we adapt a force-directed layout that allows users to refine and re-group communities based on their domain knowledge. We incorporate the proposed techniques into an interactive system to enable effective analysis and interpretation of complex patterns in large-scale integral curve datasets.
Curve Segment Neighborhood-based Vector Field Exploration
Nguyen K Phan - University of Houston, Houston, United States
Guoning Chen - University of Houston, Houston, United States
Download camera-ready PDF
Download Supplemental Material
Room: Bayshore VI
2024-10-18T13:30:00Z GMT-0600 Change your timezone on the schedule page
2024-10-18T13:30:00Z
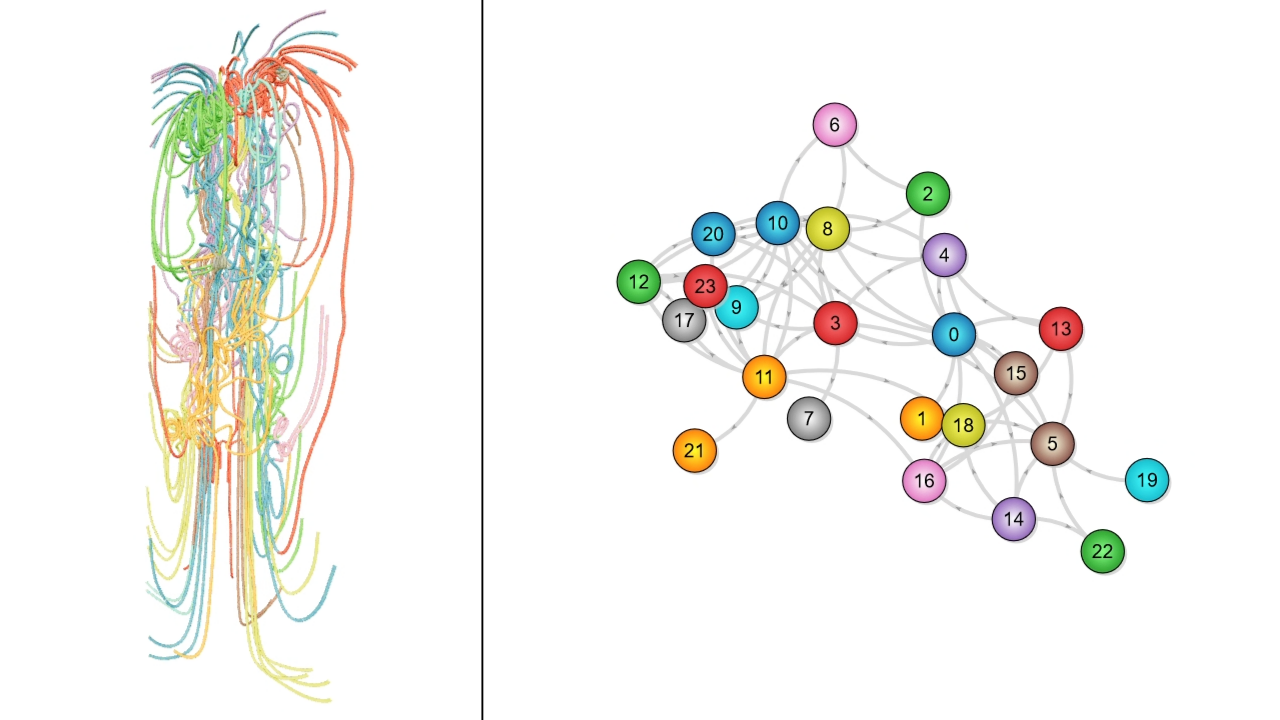
Fast forward
Keywords
Vector field, neighbor search, community detection
Abstract
Integral curves have been widely used to represent and analyze various vector fields. In this paper, we propose a Curve Segment Neighborhood Graph (CSNG) to capture the relationships between neighboring curve segments. This graph representation enables us to adapt the fast community detection algorithm, i.e., the Louvain algorithm, to identify individual graph communities from CSNG. Our results show that these communities often correspond to the features of the flow. To achieve a multi-level interactive exploration of the detected communities, we adapt a force-directed layout that allows users to refine and re-group communities based on their domain knowledge. We incorporate the proposed techniques into an interactive system to enable effective analysis and interpretation of complex patterns in large-scale integral curve datasets.
Counterpoint: Orchestrating Large-Scale Custom Animated Visualizations
Venkatesh Sivaraman - Carnegie Mellon University, Pittsburgh, United States
Frank Elavsky - Carnegie Mellon University, Pittsburgh, United States
Dominik Moritz - Carnegie Mellon University, Pittsburgh, United States
Adam Perer - Carnegie Mellon University, Pittsburgh, United States
Screen-reader Accessible PDF
Download camera-ready PDF
Download Supplemental Material
Room: Bayshore VI
2024-10-16T17:54:00Z GMT-0600 Change your timezone on the schedule page
2024-10-16T17:54:00Z

Fast forward
Keywords
Visualization Toolkits, Animation, Web Interfaces, Software System Structures
Abstract
Custom animated visualizations of large, complex datasets are helpful across many domains, but they are hard to develop. Much of the difficulty arises from maintaining visualization state across many animated graphical elements that may change in number over time. We contribute Counterpoint, a framework for state management designed to help implement such visualizations in JavaScript. Using Counterpoint, developers can manipulate large collections of marks with reactive attributes that are easy to render in scalable APIs such as Canvas and WebGL. Counterpoint also helps orchestrate the entry and exit of graphical elements using the concept of a rendering "stage." Through a performance evaluation, we show that Counterpoint adds minimal overhead over current high-performance rendering techniques while simplifying implementation. We provide two examples of visualizations created using Counterpoint that illustrate its flexibility and compatibility with other visualization toolkits as well as considerations for users with disabilities. Counterpoint is open-source and available at https://github.com/cmudig/counterpoint.
Counterpoint: Orchestrating Large-Scale Custom Animated Visualizations
Venkatesh Sivaraman - Carnegie Mellon University, Pittsburgh, United States
Frank Elavsky - Carnegie Mellon University, Pittsburgh, United States
Dominik Moritz - Carnegie Mellon University, Pittsburgh, United States
Adam Perer - Carnegie Mellon University, Pittsburgh, United States
Screen-reader Accessible PDF
Download camera-ready PDF
Download Supplemental Material
Room: Bayshore VI
2024-10-16T17:54:00Z GMT-0600 Change your timezone on the schedule page
2024-10-16T17:54:00Z

Fast forward
Keywords
Visualization Toolkits, Animation, Web Interfaces, Software System Structures
Abstract
Custom animated visualizations of large, complex datasets are helpful across many domains, but they are hard to develop. Much of the difficulty arises from maintaining visualization state across many animated graphical elements that may change in number over time. We contribute Counterpoint, a framework for state management designed to help implement such visualizations in JavaScript. Using Counterpoint, developers can manipulate large collections of marks with reactive attributes that are easy to render in scalable APIs such as Canvas and WebGL. Counterpoint also helps orchestrate the entry and exit of graphical elements using the concept of a rendering "stage." Through a performance evaluation, we show that Counterpoint adds minimal overhead over current high-performance rendering techniques while simplifying implementation. We provide two examples of visualizations created using Counterpoint that illustrate its flexibility and compatibility with other visualization toolkits as well as considerations for users with disabilities. Counterpoint is open-source and available at https://github.com/cmudig/counterpoint.
Fields, Bridges, and Foundations: How Researchers Browse Citation Network Visualizations
Kiroong Choe - Seoul National University, Seoul, Korea, Republic of
Eunhye Kim - Seoul National University, Seoul, Korea, Republic of
Sangwon Park - Dept. of Electrical and Computer Engineering, SNU, Seoul, Korea, Republic of
Jinwook Seo - Seoul National University, Seoul, Korea, Republic of
Screen-reader Accessible PDF
Download preprint PDF
Download camera-ready PDF
Room: Bayshore VI
2024-10-16T12:57:00Z GMT-0600 Change your timezone on the schedule page
2024-10-16T12:57:00Z
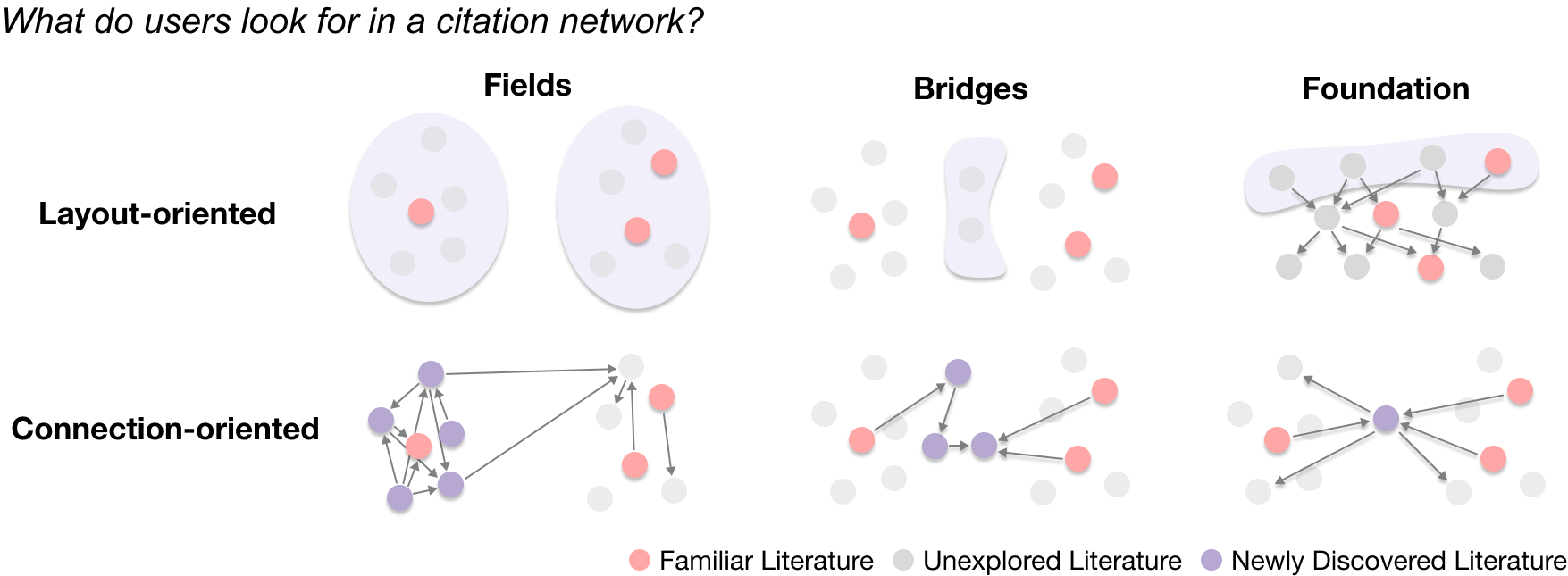
Fast forward
Keywords
Literature search, network visualization
Abstract
Visualizing citation relations with network structures is widely used, but the visual complexity can make it challenging for individual researchers to navigate through them. We collected data from 18 researchers using an interface that we designed using network simplification methods and analyzed how users browsed and identified important papers. Our analysis reveals six major patterns used for identifying papers of interest, which can be categorized into three key components: Fields, Bridges, and Foundations, each viewed from two distinct perspectives: layout-oriented and connection-oriented. The connection-oriented approach was found to be more reliable for selecting relevant papers, but the layout-oriented method was adopted more often, even though it led to unexpected results and user frustration. Our findings emphasize the importance of integrating these components and the necessity to balance visual layouts with meaningful connections to enhance the effectiveness of citation networks in academic browsing systems.
Fields, Bridges, and Foundations: How Researchers Browse Citation Network Visualizations
Kiroong Choe - Seoul National University, Seoul, Korea, Republic of
Eunhye Kim - Seoul National University, Seoul, Korea, Republic of
Sangwon Park - Dept. of Electrical and Computer Engineering, SNU, Seoul, Korea, Republic of
Jinwook Seo - Seoul National University, Seoul, Korea, Republic of
Screen-reader Accessible PDF
Download preprint PDF
Download camera-ready PDF
Room: Bayshore VI
2024-10-16T12:57:00Z GMT-0600 Change your timezone on the schedule page
2024-10-16T12:57:00Z
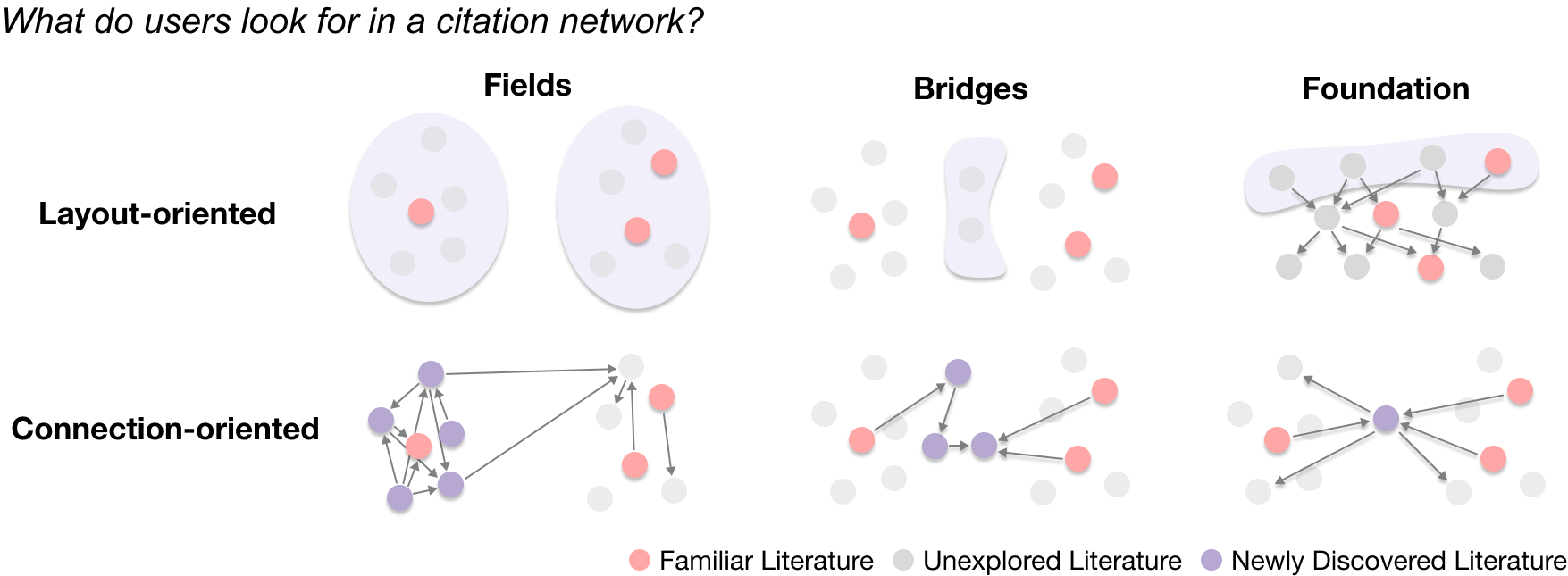
Fast forward
Keywords
Literature search, network visualization
Abstract
Visualizing citation relations with network structures is widely used, but the visual complexity can make it challenging for individual researchers to navigate through them. We collected data from 18 researchers using an interface that we designed using network simplification methods and analyzed how users browsed and identified important papers. Our analysis reveals six major patterns used for identifying papers of interest, which can be categorized into three key components: Fields, Bridges, and Foundations, each viewed from two distinct perspectives: layout-oriented and connection-oriented. The connection-oriented approach was found to be more reliable for selecting relevant papers, but the layout-oriented method was adopted more often, even though it led to unexpected results and user frustration. Our findings emphasize the importance of integrating these components and the necessity to balance visual layouts with meaningful connections to enhance the effectiveness of citation networks in academic browsing systems.
Can GPT-4 Models Detect Misleading Visualizations?
Jason Huang Alexander - University of Massachusetts Amherst, Amherst, United States
Priyal H Nanda - University of Masssachusetts Amherst, Amherst, United States
Kai-Cheng Yang - Northeastern University, Boston, United States
Ali Sarvghad - University of Massachusetts Amherst, Amherst, United States
Screen-reader Accessible PDF
Download camera-ready PDF
Room: Bayshore VI
2024-10-17T18:12:00Z GMT-0600 Change your timezone on the schedule page
2024-10-17T18:12:00Z

Fast forward
Keywords
Misleading visualizations, GPT-4, large vision language model, misinformation
Abstract
The proliferation of misleading visualizations online, particularly during critical events like public health crises and elections, poses a significant risk of misinformation. This work investigates the capability of GPT-4 models (4V, 4o, and 4o mini) to detect misleading visualizations. Utilizing a dataset of tweet-visualization pairs with various visual misleaders, we tested these models under four experimental conditions with different levels of guidance. Our results demonstrate that GPT-4 models can detect misleading visualizations with moderate accuracy without prior training (naive zero-shot) and that performance considerably improves by providing the model with the definitions of misleaders (guided zero-shot). Our results indicate that a single prompt engineering technique does not necessarily yield the best results for all types of misleaders. We found that guided few-shot was more effective for reasoning misleaders, while guided zero-shot performed better for design misleaders. This study underscores the feasibility of using large vision-language models to combat misinformation and emphasizes the importance of optimizing prompt engineering to enhance detection accuracy.
Can GPT-4 Models Detect Misleading Visualizations?
Jason Huang Alexander - University of Massachusetts Amherst, Amherst, United States
Priyal H Nanda - University of Masssachusetts Amherst, Amherst, United States
Kai-Cheng Yang - Northeastern University, Boston, United States
Ali Sarvghad - University of Massachusetts Amherst, Amherst, United States
Screen-reader Accessible PDF
Download camera-ready PDF
Room: Bayshore VI
2024-10-17T18:12:00Z GMT-0600 Change your timezone on the schedule page
2024-10-17T18:12:00Z

Fast forward
Keywords
Misleading visualizations, GPT-4, large vision language model, misinformation
Abstract
The proliferation of misleading visualizations online, particularly during critical events like public health crises and elections, poses a significant risk of misinformation. This work investigates the capability of GPT-4 models (4V, 4o, and 4o mini) to detect misleading visualizations. Utilizing a dataset of tweet-visualization pairs with various visual misleaders, we tested these models under four experimental conditions with different levels of guidance. Our results demonstrate that GPT-4 models can detect misleading visualizations with moderate accuracy without prior training (naive zero-shot) and that performance considerably improves by providing the model with the definitions of misleaders (guided zero-shot). Our results indicate that a single prompt engineering technique does not necessarily yield the best results for all types of misleaders. We found that guided few-shot was more effective for reasoning misleaders, while guided zero-shot performed better for design misleaders. This study underscores the feasibility of using large vision-language models to combat misinformation and emphasizes the importance of optimizing prompt engineering to enhance detection accuracy.
Honorable Mention
A Ridge-based Approach for Extraction and Visualization of 3D Atmospheric Fronts
Anne Gossing - Zuse Institute Berlin, Berlin, Germany
Andreas Beckert - Universität Hamburg, Hamburg, Germany
Christoph Fischer - Universität Hamburg, Hamburg, Germany
Nicolas Klenert - Zuse Institute Berlin, Berlin, Germany
Vijay Natarajan - Indian Institute of Science, Bangalore, India
George Pacey - Freie Universität Berlin, Berlin, Germany
Thorwin Vogt - Universität Hamburg, Hamburg, Germany
Marc Rautenhaus - Universität Hamburg, Hamburg, Germany
Daniel Baum - Zuse Institute Berlin, Berlin, Germany
Download camera-ready PDF
Room: Bayshore VI
2024-10-16T16:09:00Z GMT-0600 Change your timezone on the schedule page
2024-10-16T16:09:00Z

Fast forward
Keywords
Atmospheric front, ridge surface, visual analysis.
Abstract
An atmospheric front is an imaginary surface that separates two distinct air masses and is commonly defined as the warm-air side of a frontal zone with high gradients of atmospheric temperature and humidity (Fig. 1, left). These fronts are a widely used conceptual model in meteorology, which are often encountered in the literature as two-dimensional (2D) front lines on surface analysis charts. This paper presents a method for computing three-dimensional (3D) atmospheric fronts as surfaces that is capable of extracting continuous and well-confined features suitable for 3D visual analysis, spatio- temporal tracking, and statistical analyses (Fig. 1, middle, right). Recently developed contour-based methods for 3D front extraction rely on computing the third derivative of a moist potential temperature field. Additionally, they require the field to be smoothed to obtain continuous large-scale structures. This paper demonstrates the feasibility of an alternative method to front extraction using ridge surface computation. The proposed method requires only the second derivative of the input field and produces accurate structures even from unsmoothed data. An application of the ridge-based method to a data set corresponding to Cyclone Friederike demonstrates its benefits and utility towards visual analysis of the full 3D structure of fronts.
Honorable Mention
A Ridge-based Approach for Extraction and Visualization of 3D Atmospheric Fronts
Anne Gossing - Zuse Institute Berlin, Berlin, Germany
Andreas Beckert - Universität Hamburg, Hamburg, Germany
Christoph Fischer - Universität Hamburg, Hamburg, Germany
Nicolas Klenert - Zuse Institute Berlin, Berlin, Germany
Vijay Natarajan - Indian Institute of Science, Bangalore, India
George Pacey - Freie Universität Berlin, Berlin, Germany
Thorwin Vogt - Universität Hamburg, Hamburg, Germany
Marc Rautenhaus - Universität Hamburg, Hamburg, Germany
Daniel Baum - Zuse Institute Berlin, Berlin, Germany
Download camera-ready PDF
Room: Bayshore VI
2024-10-16T16:09:00Z GMT-0600 Change your timezone on the schedule page
2024-10-16T16:09:00Z

Fast forward
Keywords
Atmospheric front, ridge surface, visual analysis.
Abstract
An atmospheric front is an imaginary surface that separates two distinct air masses and is commonly defined as the warm-air side of a frontal zone with high gradients of atmospheric temperature and humidity (Fig. 1, left). These fronts are a widely used conceptual model in meteorology, which are often encountered in the literature as two-dimensional (2D) front lines on surface analysis charts. This paper presents a method for computing three-dimensional (3D) atmospheric fronts as surfaces that is capable of extracting continuous and well-confined features suitable for 3D visual analysis, spatio- temporal tracking, and statistical analyses (Fig. 1, middle, right). Recently developed contour-based methods for 3D front extraction rely on computing the third derivative of a moist potential temperature field. Additionally, they require the field to be smoothed to obtain continuous large-scale structures. This paper demonstrates the feasibility of an alternative method to front extraction using ridge surface computation. The proposed method requires only the second derivative of the input field and produces accurate structures even from unsmoothed data. An application of the ridge-based method to a data set corresponding to Cyclone Friederike demonstrates its benefits and utility towards visual analysis of the full 3D structure of fronts.
Towards a Quality Approach to Hierarchical Color Maps
Tobias Mertz - Fraunhofer IGD, Darmstadt, Germany
Jörn Kohlhammer - Fraunhofer IGD, Darmstadt, Germany. TU Darmstadt, Darmstadt, Germany
Screen-reader Accessible PDF
Download preprint PDF
Download camera-ready PDF
Download Supplemental Material
Room: Bayshore VI
2024-10-17T12:48:00Z GMT-0600 Change your timezone on the schedule page
2024-10-17T12:48:00Z
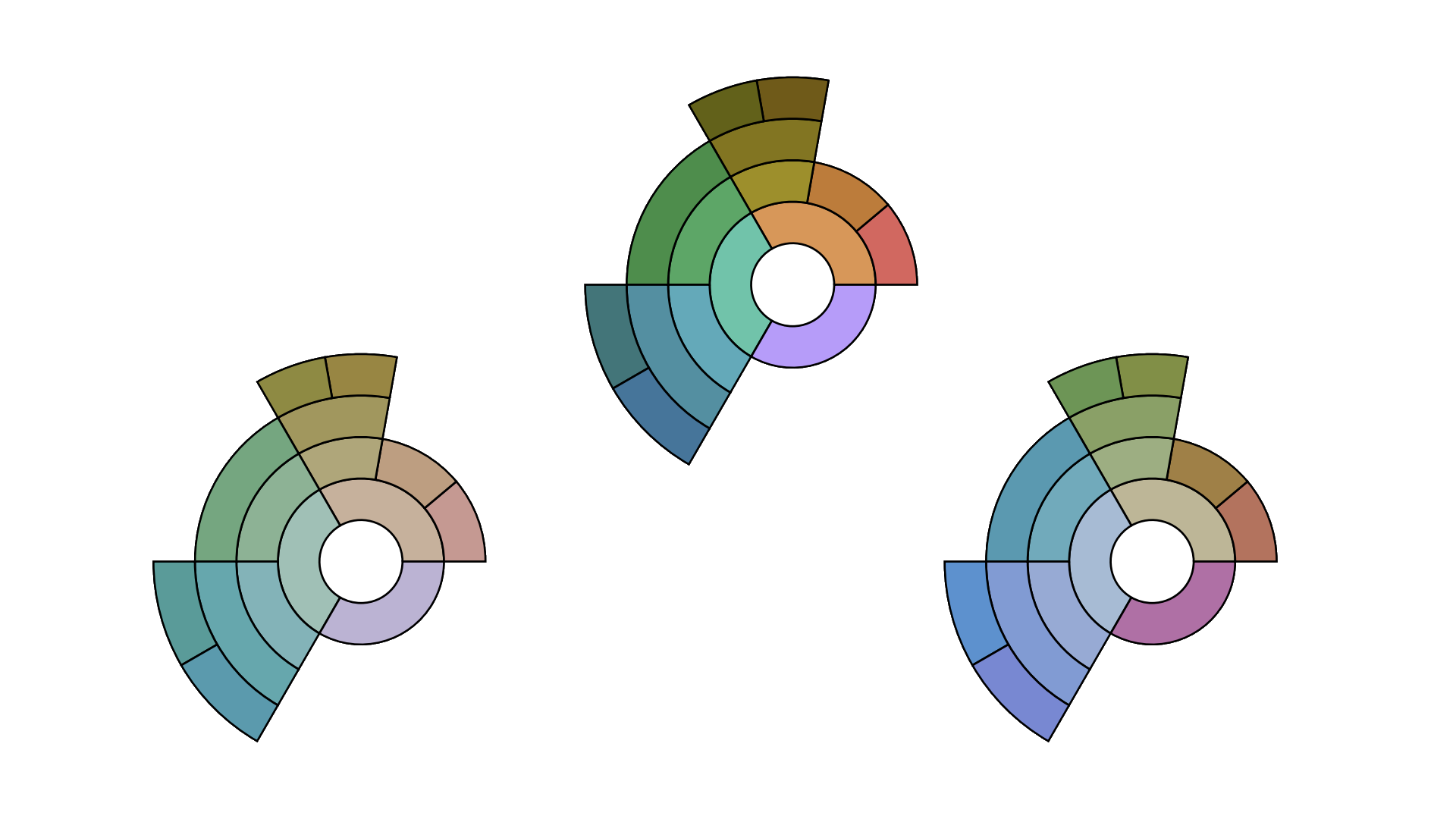
Fast forward
Keywords
Guidelines, Color, Graph/Network and Tree Data.
Abstract
To improve the perception of hierarchical structures in data sets, several color map generation algorithms have been proposed to take this structure into account. But the design of hierarchical color maps elicits different requirements to those of color maps for tabular data. Within this paper, we make an initial effort to put design rules from the color map literature into the context of hierarchical color maps. We investigate the impact of several design decisions and provide recommendations for various analysis scenarios. Thus, we lay the foundation for objective quality criteria to evaluate hierarchical color maps.
Towards a Quality Approach to Hierarchical Color Maps
Tobias Mertz - Fraunhofer IGD, Darmstadt, Germany
Jörn Kohlhammer - Fraunhofer IGD, Darmstadt, Germany. TU Darmstadt, Darmstadt, Germany
Screen-reader Accessible PDF
Download preprint PDF
Download camera-ready PDF
Download Supplemental Material
Room: Bayshore VI
2024-10-17T12:48:00Z GMT-0600 Change your timezone on the schedule page
2024-10-17T12:48:00Z
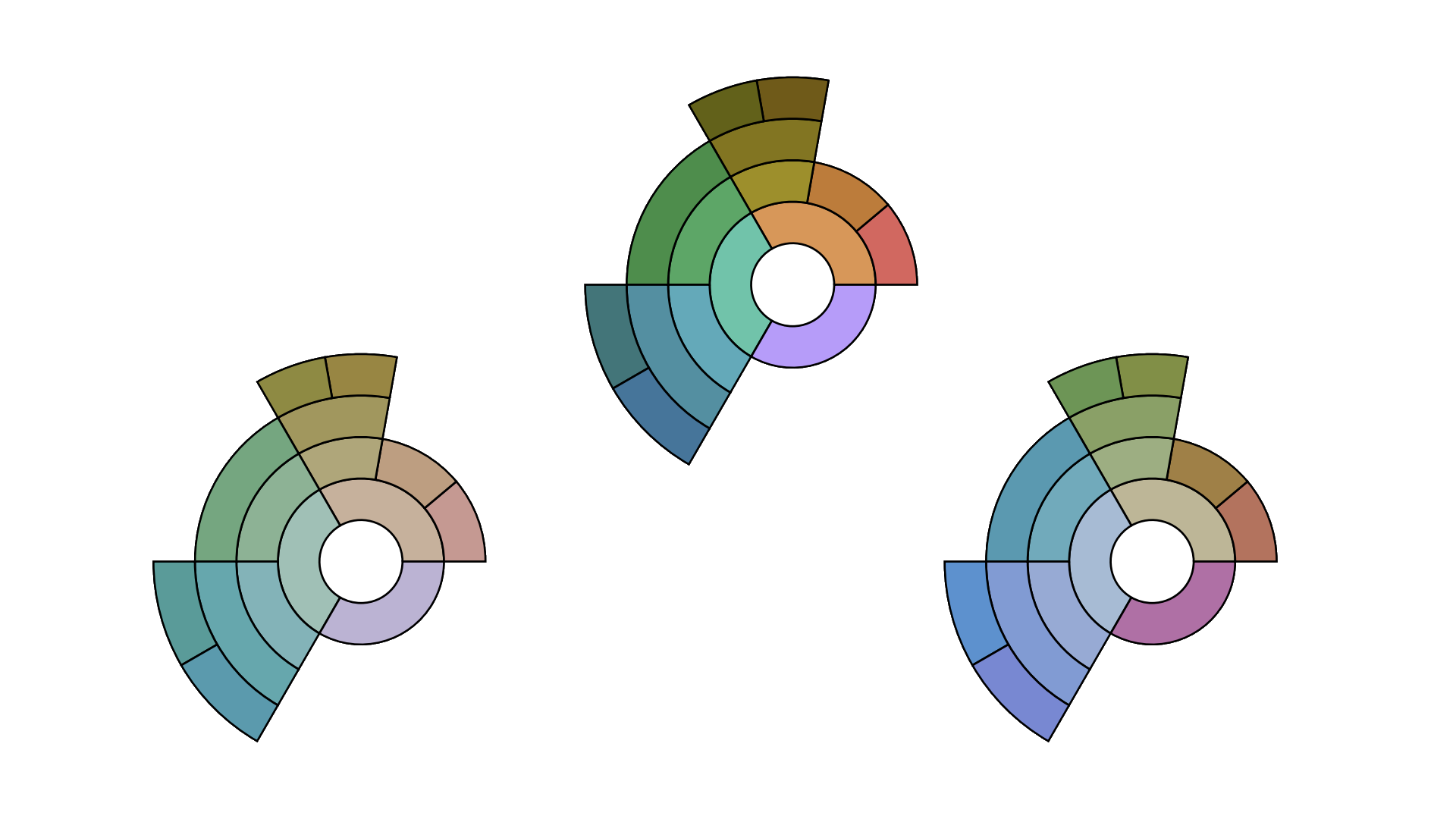
Fast forward
Keywords
Guidelines, Color, Graph/Network and Tree Data.
Abstract
To improve the perception of hierarchical structures in data sets, several color map generation algorithms have been proposed to take this structure into account. But the design of hierarchical color maps elicits different requirements to those of color maps for tabular data. Within this paper, we make an initial effort to put design rules from the color map literature into the context of hierarchical color maps. We investigate the impact of several design decisions and provide recommendations for various analysis scenarios. Thus, we lay the foundation for objective quality criteria to evaluate hierarchical color maps.
Two-point Equidistant Projection and Degree-of-interest Filtering for Smooth Exploration of Geo-referenced Networks
Max Franke - University of Stuttgart, Stuttgart, Germany
Samuel Beck - University of Stuttgart, Stuttgart, Germany
Steffen Koch - University of Stuttgart, Stuttgart, Germany
Download camera-ready PDF
Room: Bayshore VI
2024-10-17T16:45:00Z GMT-0600 Change your timezone on the schedule page
2024-10-17T16:45:00Z

Fast forward
Keywords
Geographical projection, geo-referenced graph, degree-of-interest function, ego-perspective exploration.
Abstract
The visualization and interactive exploration of geo-referenced networks poses challenges if the network's nodes are not evenly distributed. Our approach proposes new ways of realizing animated transitions for exploring such networks from an ego-perspective. We aim to reduce the required screen estate while maintaining the viewers' mental map of distances and directions. A preliminary study provides first insights of the comprehensiveness of animated geographic transitions regarding directional relationships between start and end point in different projections. Two use cases showcase how ego-perspective graph exploration can be supported using less screen space than previous approaches.
Two-point Equidistant Projection and Degree-of-interest Filtering for Smooth Exploration of Geo-referenced Networks
Max Franke - University of Stuttgart, Stuttgart, Germany
Samuel Beck - University of Stuttgart, Stuttgart, Germany
Steffen Koch - University of Stuttgart, Stuttgart, Germany
Download camera-ready PDF
Room: Bayshore VI
2024-10-17T16:45:00Z GMT-0600 Change your timezone on the schedule page
2024-10-17T16:45:00Z

Fast forward
Keywords
Geographical projection, geo-referenced graph, degree-of-interest function, ego-perspective exploration.
Abstract
The visualization and interactive exploration of geo-referenced networks poses challenges if the network's nodes are not evenly distributed. Our approach proposes new ways of realizing animated transitions for exploring such networks from an ego-perspective. We aim to reduce the required screen estate while maintaining the viewers' mental map of distances and directions. A preliminary study provides first insights of the comprehensiveness of animated geographic transitions regarding directional relationships between start and end point in different projections. Two use cases showcase how ego-perspective graph exploration can be supported using less screen space than previous approaches.
Exploring the Capability of LLMs in Performing Low-Level Visual Analytic Tasks on SVG Data Visualizations
Zhongzheng Xu - Brown University, Providence, United States
Emily Wall - Emory University, Atlanta, United States
Screen-reader Accessible PDF
Download preprint PDF
Download camera-ready PDF
Download Supplemental Material
Room: Bayshore VI
2024-10-17T18:48:00Z GMT-0600 Change your timezone on the schedule page
2024-10-17T18:48:00Z

Fast forward
Keywords
Data Visualization, Large Language Models (LLM), Visual Analytics Tasks, Support Vector Graphics (SVG)
Abstract
Data visualizations help extract insights from datasets, but reaching these insights requires decomposing high level goals into low-level analytic tasks that can be complex due to varying degrees of data literacy and visualization experience. Recent advancements in large language models (LLMs) have shown promise for lowering barriers for users to achieve tasks such as writing code and may likewise facilitate visualization insight. Scalable Vector Graphics (SVG), a text-based image format common in data visualizations, matches well with the text sequence processing of transformer-based LLMs. In this paper, we explore the capability of LLMs to perform 10 low-level visual analytic tasks defined by Amar, Eagan, and Stasko directly on SVG-based visualizations. Using zero-shot prompts, we instruct the models to provide responses or modify the SVG code based on given visualizations. Our findings demonstrate that LLMs can effectively modify existing SVG visualizations for some tasks like Cluster but perform poorly on tasks requiring mathematical operations like Compute Derived Value. We also discovered that LLM performance can vary based on factors such as the number of data points, the presence of value labels, and the chart type. Our findings contribute to gauging the general capabilities of LLMs and highlight the need for further exploration and development to fully harness their potential in supporting visual analytic tasks.
Exploring the Capability of LLMs in Performing Low-Level Visual Analytic Tasks on SVG Data Visualizations
Zhongzheng Xu - Brown University, Providence, United States
Emily Wall - Emory University, Atlanta, United States
Screen-reader Accessible PDF
Download preprint PDF
Download camera-ready PDF
Download Supplemental Material
Room: Bayshore VI
2024-10-17T18:48:00Z GMT-0600 Change your timezone on the schedule page
2024-10-17T18:48:00Z

Fast forward
Keywords
Data Visualization, Large Language Models (LLM), Visual Analytics Tasks, Support Vector Graphics (SVG)
Abstract
Data visualizations help extract insights from datasets, but reaching these insights requires decomposing high level goals into low-level analytic tasks that can be complex due to varying degrees of data literacy and visualization experience. Recent advancements in large language models (LLMs) have shown promise for lowering barriers for users to achieve tasks such as writing code and may likewise facilitate visualization insight. Scalable Vector Graphics (SVG), a text-based image format common in data visualizations, matches well with the text sequence processing of transformer-based LLMs. In this paper, we explore the capability of LLMs to perform 10 low-level visual analytic tasks defined by Amar, Eagan, and Stasko directly on SVG-based visualizations. Using zero-shot prompts, we instruct the models to provide responses or modify the SVG code based on given visualizations. Our findings demonstrate that LLMs can effectively modify existing SVG visualizations for some tasks like Cluster but perform poorly on tasks requiring mathematical operations like Compute Derived Value. We also discovered that LLM performance can vary based on factors such as the number of data points, the presence of value labels, and the chart type. Our findings contribute to gauging the general capabilities of LLMs and highlight the need for further exploration and development to fully harness their potential in supporting visual analytic tasks.
Topological Separation of Vortices
Adeel Zafar - University of Houston, Houston, United States
Zahra Poorshayegh - University of Houston, Houston, United States
Di Yang - University of Houston, Houston, United States
Guoning Chen - University of Houston, Houston, United States
Download preprint PDF
Download camera-ready PDF
Download Supplemental Material
Room: Bayshore I
2024-10-17T15:15:00Z GMT-0600 Change your timezone on the schedule page
2024-10-17T15:15:00Z

Fast forward
Keywords
Fluid flow, vortices, vortex topology
Abstract
Vortices and their analysis play a critical role in the understanding of complex phenomena in turbulent flows. Traditional vortex extraction methods, notably region-based techniques, often overlook the entanglement phenomenon, resulting in the inclusion of multiple vortices within a single extracted region. Their separation is necessary for quantifying different types of vortices and their statistics. In this study, we propose a novel vortex separation method that extends the conventional contour tree-based segmentation approach with an additional step termed “layering”. Upon extracting a vortical region using specified vortex criteria (e.g., λ2), we initially establish topological segmentation based on the contour tree, followed by the layering process to allocate appropriate segmentation IDs to unsegmented cells, thus separating individual vortices within the region. However, these regions may still suffer from inaccurate splits, which we address statistically by leveraging the continuity of vorticity lines across the split boundaries. Our findings demonstrate a significant improvement in both the separation of vortices and the mitigation of inaccurate splits compared to prior methods.
Topological Separation of Vortices
Adeel Zafar - University of Houston, Houston, United States
Zahra Poorshayegh - University of Houston, Houston, United States
Di Yang - University of Houston, Houston, United States
Guoning Chen - University of Houston, Houston, United States
Download preprint PDF
Download camera-ready PDF
Download Supplemental Material
Room: Bayshore I
2024-10-17T15:15:00Z GMT-0600 Change your timezone on the schedule page
2024-10-17T15:15:00Z

Fast forward
Keywords
Fluid flow, vortices, vortex topology
Abstract
Vortices and their analysis play a critical role in the understanding of complex phenomena in turbulent flows. Traditional vortex extraction methods, notably region-based techniques, often overlook the entanglement phenomenon, resulting in the inclusion of multiple vortices within a single extracted region. Their separation is necessary for quantifying different types of vortices and their statistics. In this study, we propose a novel vortex separation method that extends the conventional contour tree-based segmentation approach with an additional step termed “layering”. Upon extracting a vortical region using specified vortex criteria (e.g., λ2), we initially establish topological segmentation based on the contour tree, followed by the layering process to allocate appropriate segmentation IDs to unsegmented cells, thus separating individual vortices within the region. However, these regions may still suffer from inaccurate splits, which we address statistically by leveraging the continuity of vorticity lines across the split boundaries. Our findings demonstrate a significant improvement in both the separation of vortices and the mitigation of inaccurate splits compared to prior methods.
Towards Reusable and Reactive Widgets for Information Visualization Research and Dissemination
John Alexis Guerra-Gomez - Northeastern University, San Francisco, United States
Screen-reader Accessible PDF
Download camera-ready PDF
Download Supplemental Material
Room: Bayshore I
2024-10-17T17:00:00Z GMT-0600 Change your timezone on the schedule page
2024-10-17T17:00:00Z
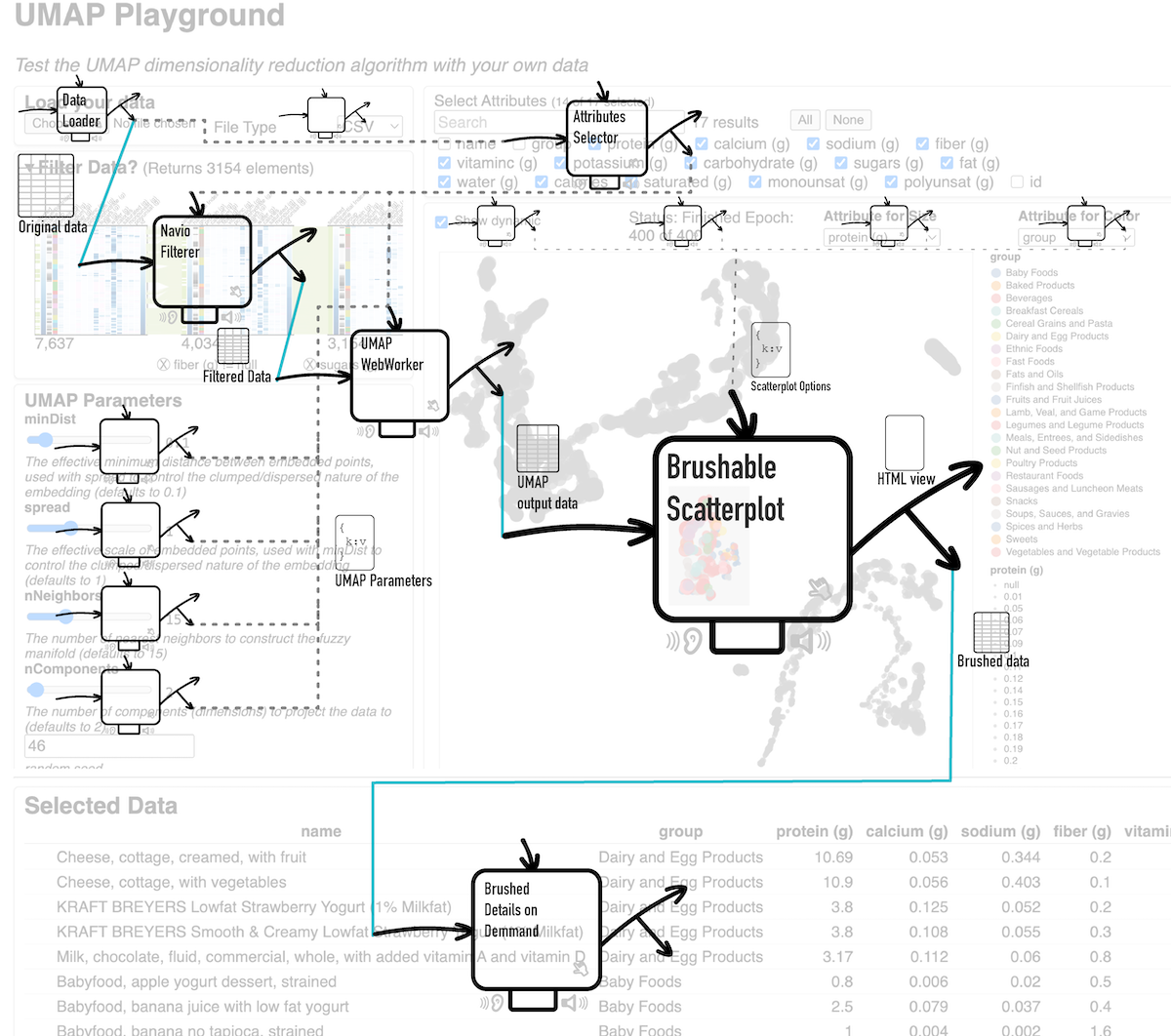
Fast forward
Keywords
Information Visualization, Software Components, Reactive Components, Notebook Programming, Direct Manipulation, Brush and Linking
Abstract
The information visualization research community commonly produces supporting software to demonstrate technical contributions to the field. However, developing this software tends to be an overwhelming task. The final product tends to be a research prototype without much thought for modularization and re-usability, which makes it harder to replicate and adopt. This paper presents a design pattern for facilitating the creation, dissemination, and re-utilization of visualization techniques using reactive widgets. The design pattern features basic concepts that leverage modern front-end development best practices and standards, which facilitate development and replication. The paper presents several usage examples of the pattern, templates for implementation, and even a wrapper for facilitating the conversion of any Vega [27,28] specification into a reactive widget.
Towards Reusable and Reactive Widgets for Information Visualization Research and Dissemination
John Alexis Guerra-Gomez - Northeastern University, San Francisco, United States
Screen-reader Accessible PDF
Download camera-ready PDF
Download Supplemental Material
Room: Bayshore I
2024-10-17T17:00:00Z GMT-0600 Change your timezone on the schedule page
2024-10-17T17:00:00Z
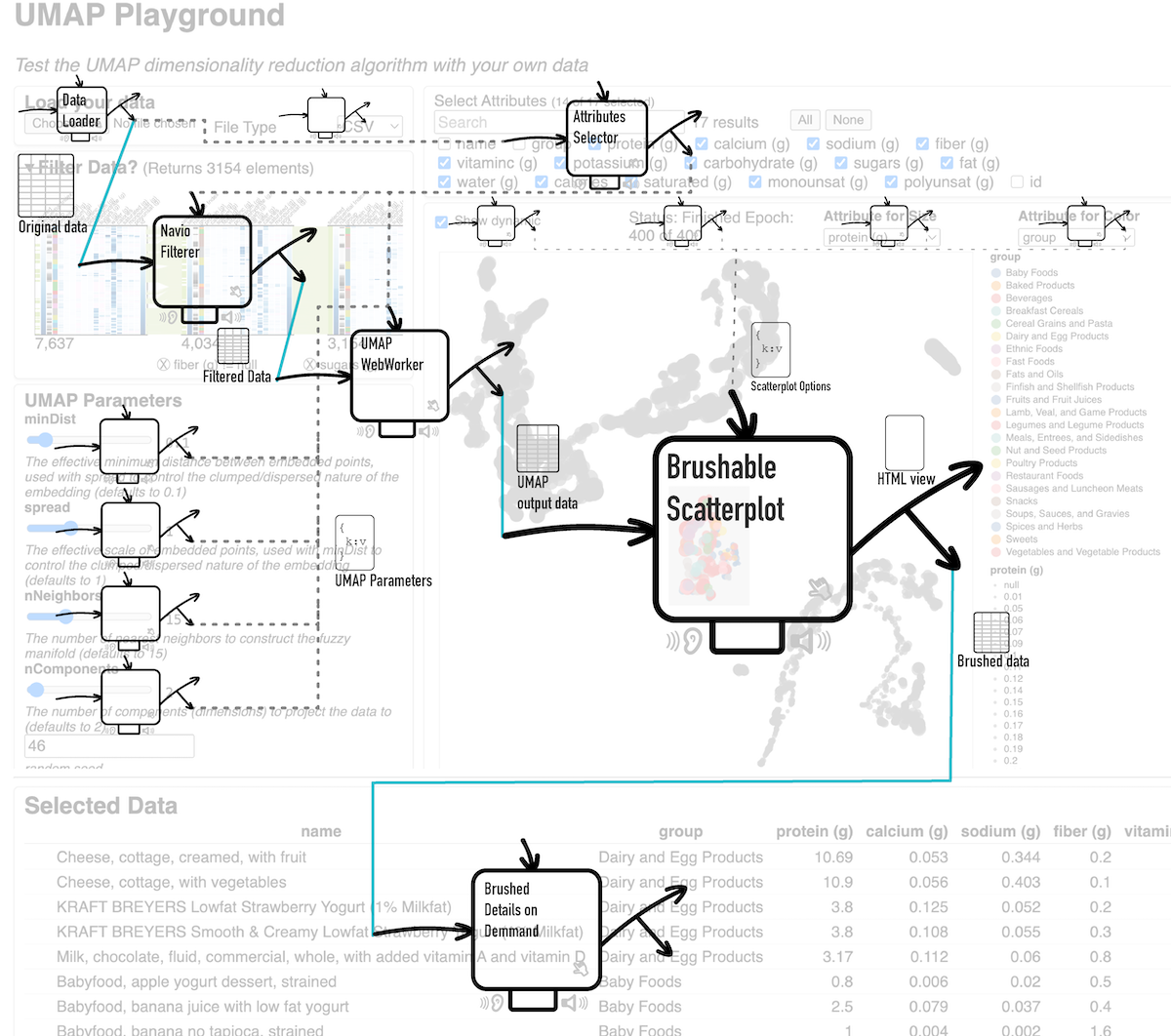
Fast forward
Keywords
Information Visualization, Software Components, Reactive Components, Notebook Programming, Direct Manipulation, Brush and Linking
Abstract
The information visualization research community commonly produces supporting software to demonstrate technical contributions to the field. However, developing this software tends to be an overwhelming task. The final product tends to be a research prototype without much thought for modularization and re-usability, which makes it harder to replicate and adopt. This paper presents a design pattern for facilitating the creation, dissemination, and re-utilization of visualization techniques using reactive widgets. The design pattern features basic concepts that leverage modern front-end development best practices and standards, which facilitate development and replication. The paper presents several usage examples of the pattern, templates for implementation, and even a wrapper for facilitating the conversion of any Vega [27,28] specification into a reactive widget.
Bringing Data into the Conversation: Adapting Content from Business Intelligence Dashboards for Threaded Collaboration Platforms
Hyeok Kim - Northwestern University, Evanston, United States
Arjun Srinivasan - Tableau Research, Seattle, United States
Matthew Brehmer - Tableau Research, Seattle, United States
Screen-reader Accessible PDF
Download preprint PDF
Download camera-ready PDF
Download Supplemental Material
Room: Bayshore VI
2024-10-17T16:54:00Z GMT-0600 Change your timezone on the schedule page
2024-10-17T16:54:00Z

Fast forward
Keywords
Collaboration visualization, visualization retargeting, responsive visualization design, business intelligence
Abstract
To enable data-driven decision-making across organizations, data professionals need to share insights with their colleagues in context-appropriate communication channels. Many of their colleagues rely on data but are not themselves analysts; furthermore, their colleagues are reluctant or unable to use dedicated analytical applications or dashboards, and they expect communication to take place within threaded collaboration platforms such as Slack or Microsoft Teams. In this paper, we introduce a set of six strategies for adapting content from business intelligence (BI) dashboards into appropriate formats for sharing on collaboration platforms, formats that we refer to as dashboard snapshots. Informed by prior studies of enterprise communication around data, these strategies go beyond redesigning or restyling by considering varying levels of data literacy across an organization, introducing affordances for self-service question-answering, and anticipating the post-sharing lifecycle of data artifacts. These strategies involve the use of templates that are matched to common communicative intents, serving to reduce the workload of data professionals. We contribute a formal representation of these strategies and demonstrate their applicability in a comprehensive enterprise communication scenario featuring multiple stakeholders that unfolds over the span of months.
Bringing Data into the Conversation: Adapting Content from Business Intelligence Dashboards for Threaded Collaboration Platforms
Hyeok Kim - Northwestern University, Evanston, United States
Arjun Srinivasan - Tableau Research, Seattle, United States
Matthew Brehmer - Tableau Research, Seattle, United States
Screen-reader Accessible PDF
Download preprint PDF
Download camera-ready PDF
Download Supplemental Material
Room: Bayshore VI
2024-10-17T16:54:00Z GMT-0600 Change your timezone on the schedule page
2024-10-17T16:54:00Z

Fast forward
Keywords
Collaboration visualization, visualization retargeting, responsive visualization design, business intelligence
Abstract
To enable data-driven decision-making across organizations, data professionals need to share insights with their colleagues in context-appropriate communication channels. Many of their colleagues rely on data but are not themselves analysts; furthermore, their colleagues are reluctant or unable to use dedicated analytical applications or dashboards, and they expect communication to take place within threaded collaboration platforms such as Slack or Microsoft Teams. In this paper, we introduce a set of six strategies for adapting content from business intelligence (BI) dashboards into appropriate formats for sharing on collaboration platforms, formats that we refer to as dashboard snapshots. Informed by prior studies of enterprise communication around data, these strategies go beyond redesigning or restyling by considering varying levels of data literacy across an organization, introducing affordances for self-service question-answering, and anticipating the post-sharing lifecycle of data artifacts. These strategies involve the use of templates that are matched to common communicative intents, serving to reduce the workload of data professionals. We contribute a formal representation of these strategies and demonstrate their applicability in a comprehensive enterprise communication scenario featuring multiple stakeholders that unfolds over the span of months.
Animating the Narrative: A Review of Animation Styles in Narrative Visualization
Vyri Junhan Yang - Louisiana State University, Baton Rouge, United States
Mahmood Jasim - Louisiana State University, Baton Rouge, United States
Download camera-ready PDF
Room: Bayshore III
2024-10-17T18:45:00Z GMT-0600 Change your timezone on the schedule page
2024-10-17T18:45:00Z
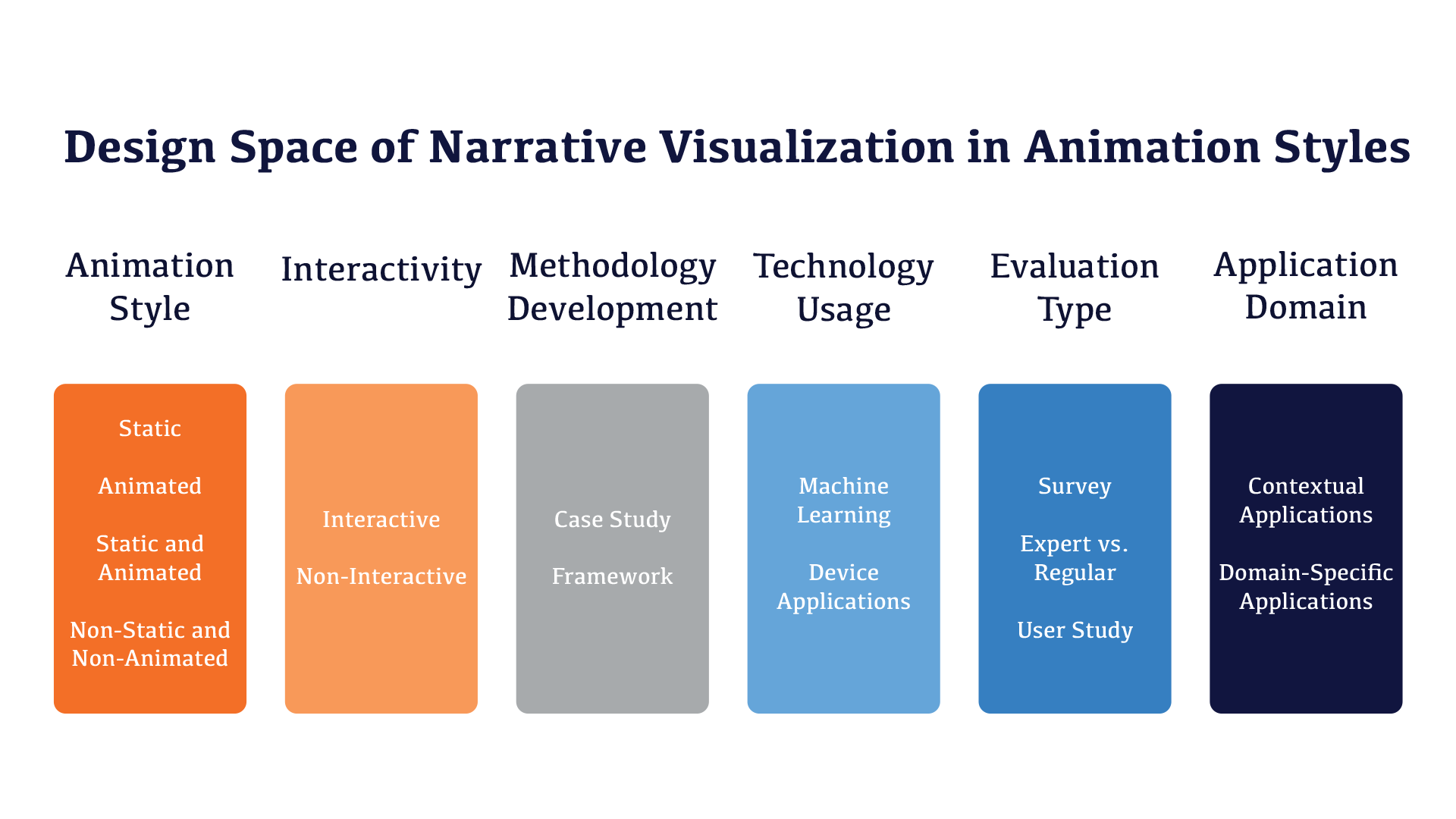
Fast forward
Keywords
Narrative visualizations, static and animated visualization, categorization, design space
Abstract
Narrative visualization has become a crucial tool in data presentation, merging storytelling with data visualization to convey complex information in an engaging and accessible manner. In this study, we review the design space for narrative visualizations, focusing on animation style, through a comprehensive analysis of 80 papers from key visualization venues. We categorize these papers into six broad themes: Animation Style, Interactivity, Technology Usage, Methodology Development, Evaluation Type, and Application Domain. Our findings reveal a significant evolution in the field, marked by a growing preference for animated and non-interactive techniques. This trend reflects a shift towards minimizing user interaction while enhancing the clarity and impact of data presentation. We also identified key trends and technologies shaping the field, highlighting the role of technologies, such as machine learning in driving these changes. We offer insights into the dynamic interrelations within the narrative visualization domains, and suggest future research directions, including exploring non-interactive techniques, examining the interplay between different visualization elements, and developing domain-specific visualizations.
Animating the Narrative: A Review of Animation Styles in Narrative Visualization
Vyri Junhan Yang - Louisiana State University, Baton Rouge, United States
Mahmood Jasim - Louisiana State University, Baton Rouge, United States
Download camera-ready PDF
Room: Bayshore III
2024-10-17T18:45:00Z GMT-0600 Change your timezone on the schedule page
2024-10-17T18:45:00Z
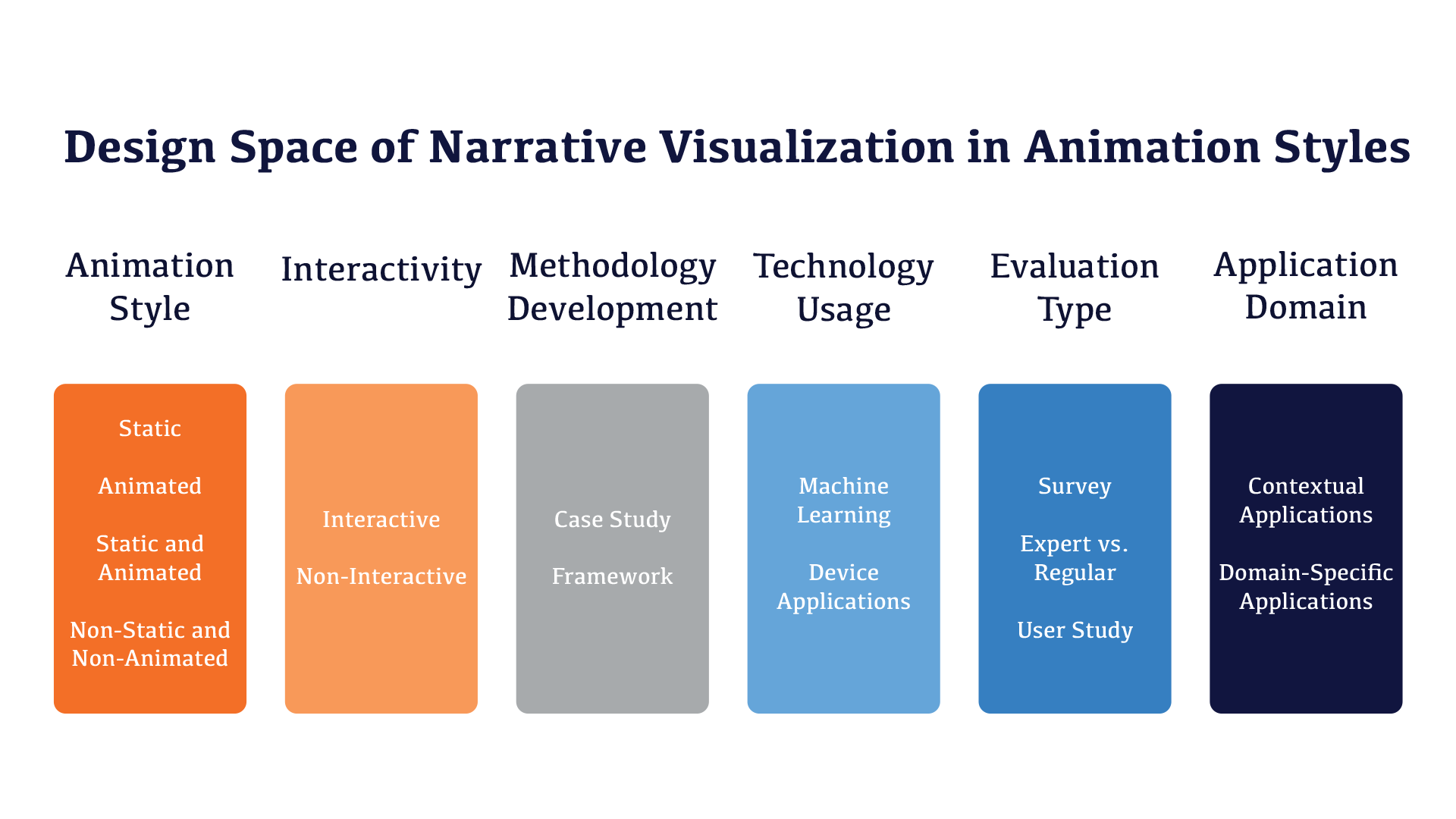
Fast forward
Keywords
Narrative visualizations, static and animated visualization, categorization, design space
Abstract
Narrative visualization has become a crucial tool in data presentation, merging storytelling with data visualization to convey complex information in an engaging and accessible manner. In this study, we review the design space for narrative visualizations, focusing on animation style, through a comprehensive analysis of 80 papers from key visualization venues. We categorize these papers into six broad themes: Animation Style, Interactivity, Technology Usage, Methodology Development, Evaluation Type, and Application Domain. Our findings reveal a significant evolution in the field, marked by a growing preference for animated and non-interactive techniques. This trend reflects a shift towards minimizing user interaction while enhancing the clarity and impact of data presentation. We also identified key trends and technologies shaping the field, highlighting the role of technologies, such as machine learning in driving these changes. We offer insights into the dynamic interrelations within the narrative visualization domains, and suggest future research directions, including exploring non-interactive techniques, examining the interplay between different visualization elements, and developing domain-specific visualizations.
LinkQ: An LLM-Assisted Visual Interface for Knowledge Graph Question-Answering
Harry Li - MIT Lincoln Laboratory, Lexington, United States
Gabriel Appleby - Tufts University, Medford, United States
Ashley Suh - MIT Lincoln Laboratory, Lexington, United States
Screen-reader Accessible PDF
Download preprint PDF
Download camera-ready PDF
Download Supplemental Material
Room: Bayshore VI
2024-10-17T18:30:00Z GMT-0600 Change your timezone on the schedule page
2024-10-17T18:30:00Z

Fast forward
Keywords
Knowledge graphs, large language models, query construction, question-answering, natural language interfaces.
Abstract
We present LinkQ, a system that leverages a large language model (LLM) to facilitate knowledge graph (KG) query construction through natural language question-answering. Traditional approaches often require detailed knowledge of a graph querying language, limiting the ability for users - even experts - to acquire valuable insights from KGs. LinkQ simplifies this process by implementing a multistep protocol in which the LLM interprets a user's question, then systematically converts it into a well-formed query. LinkQ helps users iteratively refine any open-ended questions into precise ones, supporting both targeted and exploratory analysis. Further, LinkQ guards against the LLM hallucinating outputs by ensuring users' questions are only ever answered from ground truth KG data. We demonstrate the efficacy of LinkQ through a qualitative study with five KG practitioners. Our results indicate that practitioners find LinkQ effective for KG question-answering, and desire future LLM-assisted exploratory data analysis systems.
LinkQ: An LLM-Assisted Visual Interface for Knowledge Graph Question-Answering
Harry Li - MIT Lincoln Laboratory, Lexington, United States
Gabriel Appleby - Tufts University, Medford, United States
Ashley Suh - MIT Lincoln Laboratory, Lexington, United States
Screen-reader Accessible PDF
Download preprint PDF
Download camera-ready PDF
Download Supplemental Material
Room: Bayshore VI
2024-10-17T18:30:00Z GMT-0600 Change your timezone on the schedule page
2024-10-17T18:30:00Z

Fast forward
Keywords
Knowledge graphs, large language models, query construction, question-answering, natural language interfaces.
Abstract
We present LinkQ, a system that leverages a large language model (LLM) to facilitate knowledge graph (KG) query construction through natural language question-answering. Traditional approaches often require detailed knowledge of a graph querying language, limiting the ability for users - even experts - to acquire valuable insights from KGs. LinkQ simplifies this process by implementing a multistep protocol in which the LLM interprets a user's question, then systematically converts it into a well-formed query. LinkQ helps users iteratively refine any open-ended questions into precise ones, supporting both targeted and exploratory analysis. Further, LinkQ guards against the LLM hallucinating outputs by ensuring users' questions are only ever answered from ground truth KG data. We demonstrate the efficacy of LinkQ through a qualitative study with five KG practitioners. Our results indicate that practitioners find LinkQ effective for KG question-answering, and desire future LLM-assisted exploratory data analysis systems.
From Graphs to Words: A Computer-Assisted Framework for the Production of Accessible Text Descriptions
Qiang Xu - Polytechnique Montréal, Montréal, Canada
Thomas Hurtut - Polytechnique Montreal, Montreal, Canada
Download camera-ready PDF
Room: Palma Ceia I
2024-10-16T13:03:00Z GMT-0600 Change your timezone on the schedule page
2024-10-16T13:03:00Z
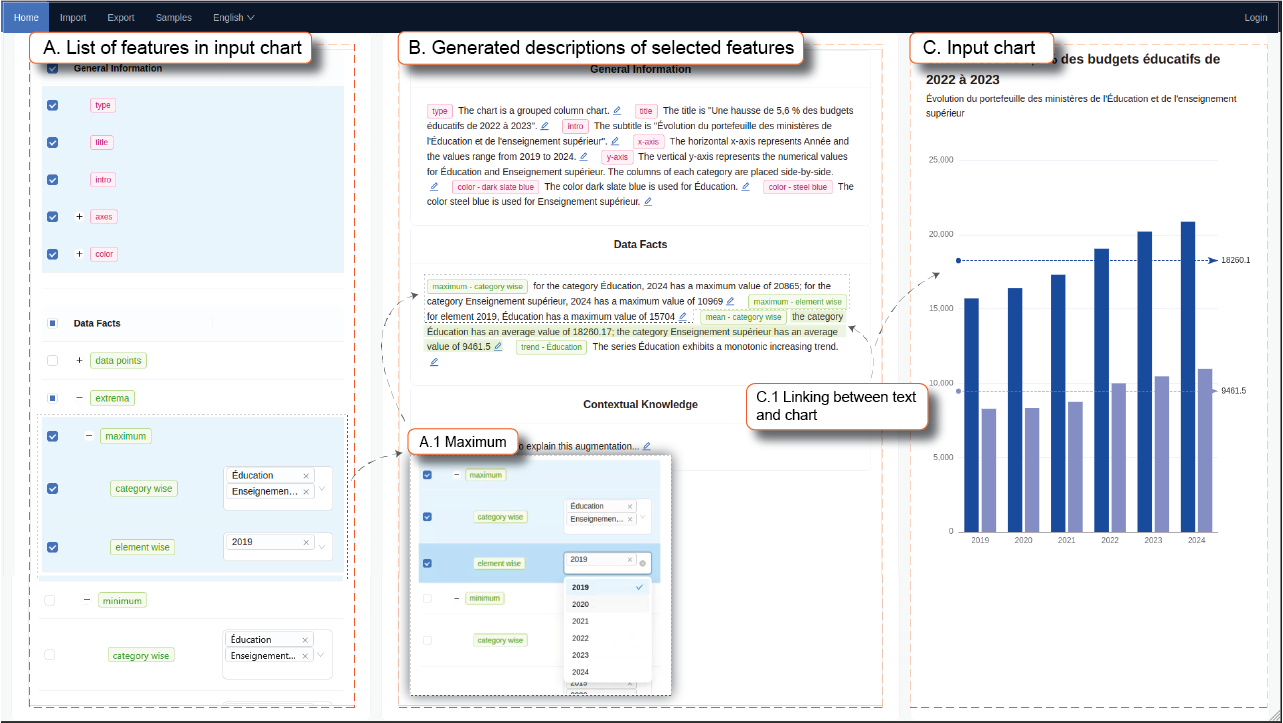
Fast forward
Keywords
Accessibility, chart text description.
Abstract
In the digital landscape, the ubiquity of data visualizations in media underscores the necessity for accessibility to ensure inclusivity for all users, including those with visual impairments. Current visual content often fails to cater to the needs of screen reader users due to the absence of comprehensive textual descriptions. To address this gap, we propose in this paper a framework designed to empower media content creators to transform charts into descriptive narratives. This tool not only facilitates the understanding of complex visual data through text but also fosters a broader awareness of accessibility in digital content creation. Through the application of this framework, users can interpret and convey the insights of data visualizations more effectively, accommodating a diverse audience. Our evaluations reveal that this tool not only enhances the comprehension of data visualizations but also promotes new perspectives on the represented data, thereby broadening the interpretative possibilities for all users.
From Graphs to Words: A Computer-Assisted Framework for the Production of Accessible Text Descriptions
Qiang Xu - Polytechnique Montréal, Montréal, Canada
Thomas Hurtut - Polytechnique Montreal, Montreal, Canada
Download camera-ready PDF
Room: Palma Ceia I
2024-10-16T13:03:00Z GMT-0600 Change your timezone on the schedule page
2024-10-16T13:03:00Z
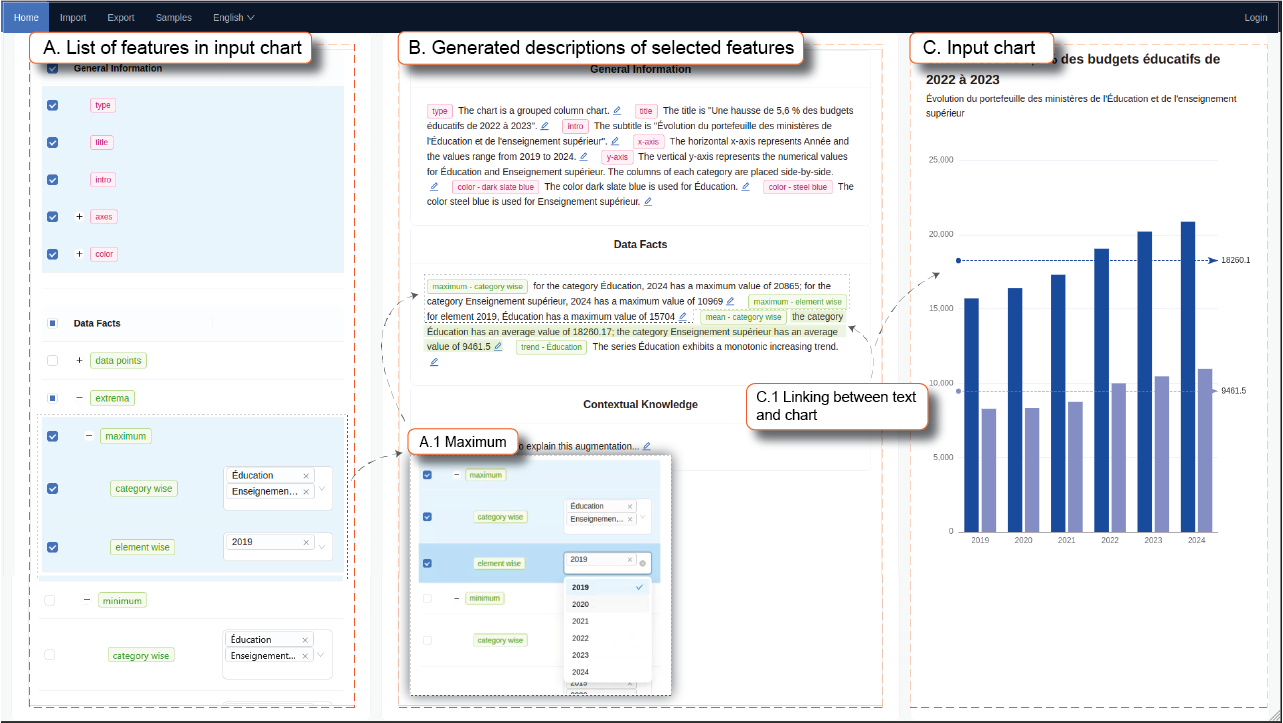
Fast forward
Keywords
Accessibility, chart text description.
Abstract
In the digital landscape, the ubiquity of data visualizations in media underscores the necessity for accessibility to ensure inclusivity for all users, including those with visual impairments. Current visual content often fails to cater to the needs of screen reader users due to the absence of comprehensive textual descriptions. To address this gap, we propose in this paper a framework designed to empower media content creators to transform charts into descriptive narratives. This tool not only facilitates the understanding of complex visual data through text but also fosters a broader awareness of accessibility in digital content creation. Through the application of this framework, users can interpret and convey the insights of data visualizations more effectively, accommodating a diverse audience. Our evaluations reveal that this tool not only enhances the comprehension of data visualizations but also promotes new perspectives on the represented data, thereby broadening the interpretative possibilities for all users.
Design of a Real-Time Visual Analytics Decision Support Interface to Manage Air Traffic Complexity
Elmira Zohrevandi - Linköping University, Norrköping, Sweden. Linköping University, Norrköping, Sweden
Katerina Vrotsou - Linköping University, Norrköping, Sweden. Linköping University, Norrköping, Sweden
Carl A. L. Westin - Institute of Science and Technology, Norrköping, Sweden. Institute of Science and Technology, Norrköping, Sweden
Jonas Lundberg - Linköping University, Norrköping, Sweden. Linköping University, Norrköping, Sweden
Anders Ynnerman - Linköping University, Norrköping, Sweden. Linköping University, Norrköping, Sweden
Download camera-ready PDF
Room: Palma Ceia I
2024-10-16T13:12:00Z GMT-0600 Change your timezone on the schedule page
2024-10-16T13:12:00Z

Fast forward
Keywords
Visual analytics, Visualization design, Safety-critical systems
Abstract
An essential task of an air traffic controller is to manage the traffic flow by predicting future trajectories. Complex traffic patterns are difficult to predict and manage and impose cognitive load on the air traffic controllers. In this work we present an interactive visual analytics interface which facilitates detection and resolution of complex traffic patterns for air traffic controllers. The interface supports air traffic controllers in detecting complex clusters of aircraft and further enables them to visualize and simultaneously compare how different re-routing strategies for each individual aircraft yield reduction of complexity in the entire sector for the next hour. The development of the concepts was supported by the domain-specific feedback we received from six fully licensed and operational air traffic controllers in an iterative design process over a period of 14 months.
Design of a Real-Time Visual Analytics Decision Support Interface to Manage Air Traffic Complexity
Elmira Zohrevandi - Linköping University, Norrköping, Sweden. Linköping University, Norrköping, Sweden
Katerina Vrotsou - Linköping University, Norrköping, Sweden. Linköping University, Norrköping, Sweden
Carl A. L. Westin - Institute of Science and Technology, Norrköping, Sweden. Institute of Science and Technology, Norrköping, Sweden
Jonas Lundberg - Linköping University, Norrköping, Sweden. Linköping University, Norrköping, Sweden
Anders Ynnerman - Linköping University, Norrköping, Sweden. Linköping University, Norrköping, Sweden
Download camera-ready PDF
Room: Palma Ceia I
2024-10-16T13:12:00Z GMT-0600 Change your timezone on the schedule page
2024-10-16T13:12:00Z

Fast forward
Keywords
Visual analytics, Visualization design, Safety-critical systems
Abstract
An essential task of an air traffic controller is to manage the traffic flow by predicting future trajectories. Complex traffic patterns are difficult to predict and manage and impose cognitive load on the air traffic controllers. In this work we present an interactive visual analytics interface which facilitates detection and resolution of complex traffic patterns for air traffic controllers. The interface supports air traffic controllers in detecting complex clusters of aircraft and further enables them to visualize and simultaneously compare how different re-routing strategies for each individual aircraft yield reduction of complexity in the entire sector for the next hour. The development of the concepts was supported by the domain-specific feedback we received from six fully licensed and operational air traffic controllers in an iterative design process over a period of 14 months.
Text-based transfer function design for semantic volume rendering
Sangwon Jeong - Vanderbilt University, Nashville, United States
Jixian Li - University of Utah, Salt Lake City, United States
Shusen Liu - Lawrence Livermore National Laboratory , Livermore, United States
Chris R. Johnson - University of Utah, Salt Lake City, United States
Matthew Berger - Vanderbilt University, Nashville, United States
Screen-reader Accessible PDF
Download preprint PDF
Download camera-ready PDF
Room: Bayshore VI
2024-10-16T16:45:00Z GMT-0600 Change your timezone on the schedule page
2024-10-16T16:45:00Z
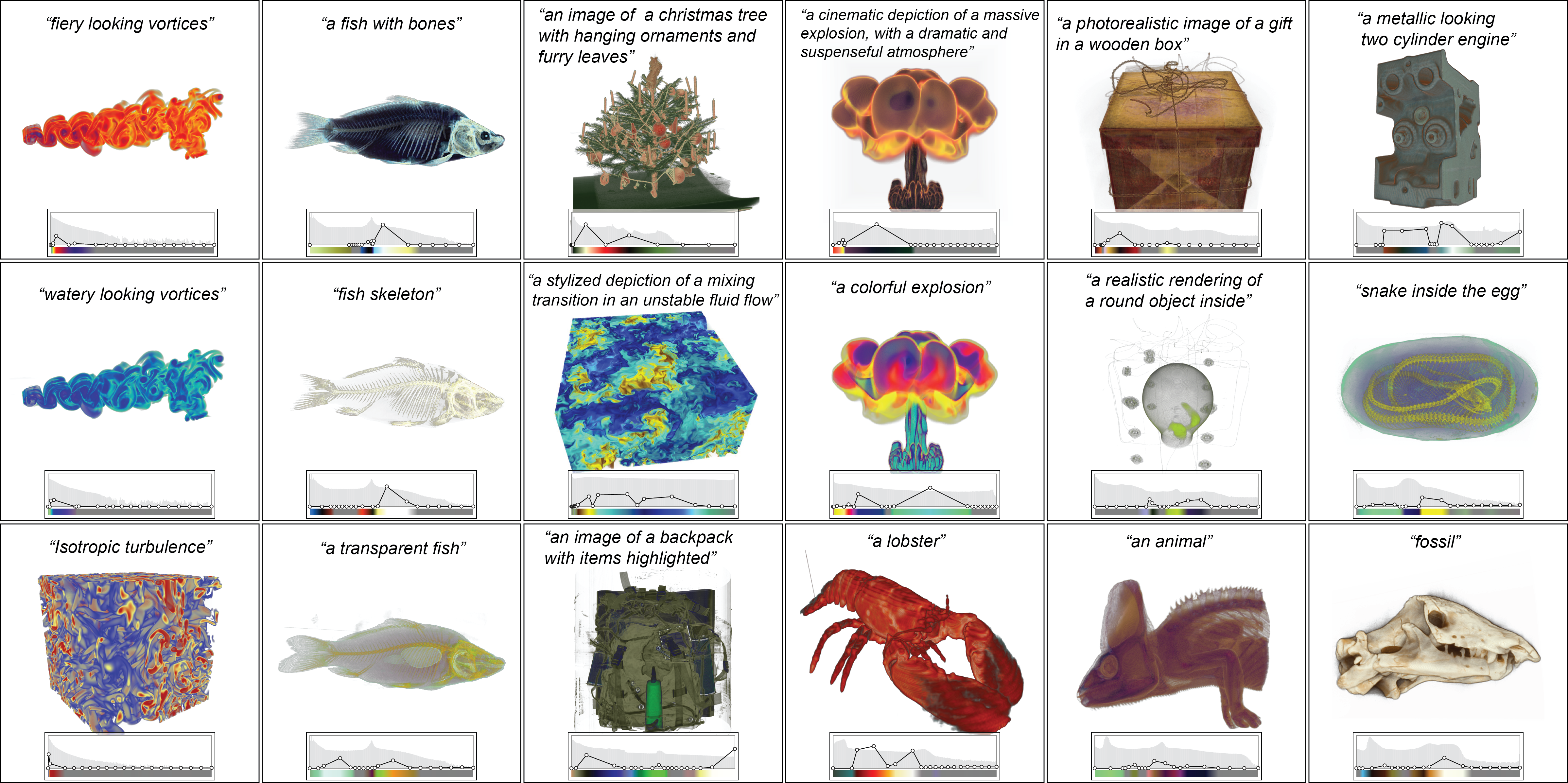
Fast forward
Keywords
Transfer function design, vision-language model
Abstract
Transfer function design is crucial in volume rendering, as it directly influences the visual representation and interpretation of volumetric data. However, creating effective transfer functions that align with users' visual objectives is often challenging due to the complex parameter space and the semantic gap between transfer function values and features of interest within the volume. In this work, we propose a novel approach that leverages recent advancements in language-vision models to bridge this semantic gap. By employing a fully differentiable rendering pipeline and an image-based loss function guided by language descriptions, our method generates transfer functions that yield volume-rendered images closely matching the user's intent. We demonstrate the effectiveness of our approach in creating meaningful transfer functions from simple descriptions, empowering users to intuitively express their desired visual outcomes with minimal effort. This advancement streamlines the transfer function design process and makes volume rendering more accessible to a wider range of users.
Text-based transfer function design for semantic volume rendering
Sangwon Jeong - Vanderbilt University, Nashville, United States
Jixian Li - University of Utah, Salt Lake City, United States
Shusen Liu - Lawrence Livermore National Laboratory , Livermore, United States
Chris R. Johnson - University of Utah, Salt Lake City, United States
Matthew Berger - Vanderbilt University, Nashville, United States
Screen-reader Accessible PDF
Download preprint PDF
Download camera-ready PDF
Room: Bayshore VI
2024-10-16T16:45:00Z GMT-0600 Change your timezone on the schedule page
2024-10-16T16:45:00Z
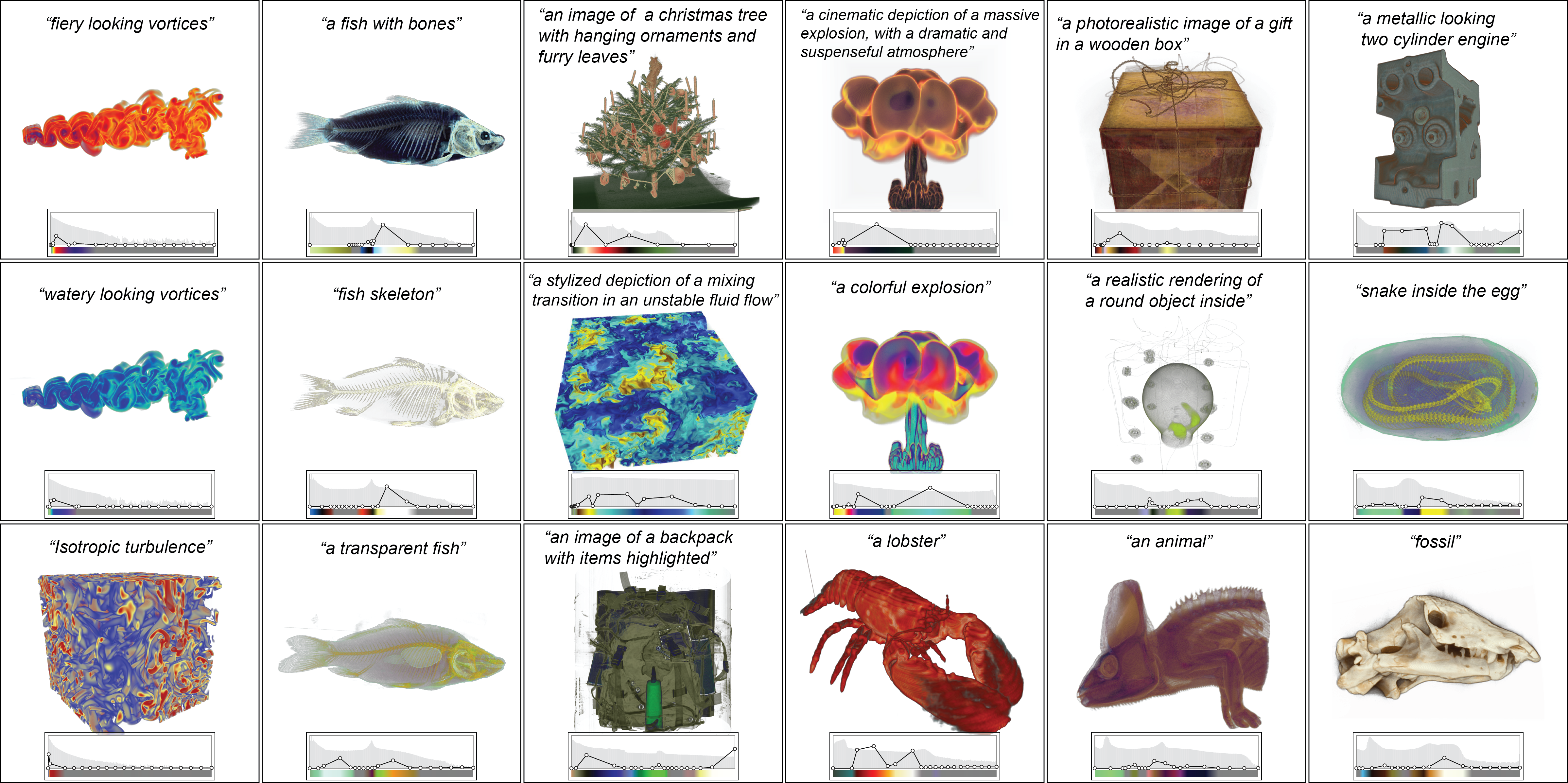
Fast forward
Keywords
Transfer function design, vision-language model
Abstract
Transfer function design is crucial in volume rendering, as it directly influences the visual representation and interpretation of volumetric data. However, creating effective transfer functions that align with users' visual objectives is often challenging due to the complex parameter space and the semantic gap between transfer function values and features of interest within the volume. In this work, we propose a novel approach that leverages recent advancements in language-vision models to bridge this semantic gap. By employing a fully differentiable rendering pipeline and an image-based loss function guided by language descriptions, our method generates transfer functions that yield volume-rendered images closely matching the user's intent. We demonstrate the effectiveness of our approach in creating meaningful transfer functions from simple descriptions, empowering users to intuitively express their desired visual outcomes with minimal effort. This advancement streamlines the transfer function design process and makes volume rendering more accessible to a wider range of users.
Diffusion Explainer: Visual Explanation for Text-to-image Stable Diffusion
Seongmin Lee - Georgia Tech, Atlanta, United States
Benjamin Hoover - GA Tech, Atlanta, United States. IBM Research AI, Cambridge, United States
Hendrik Strobelt - IBM Research AI, Cambridge, United States
Zijie J. Wang - Georgia Tech, Atlanta, United States
ShengYun Peng - Georgia Institute of Technology, Atlanta, United States
Austin P Wright - Georgia Institute of Technology , Atlanta , United States
Kevin Li - Georgia Institute of Technology, Atlanta, United States
Haekyu Park - Georgia Institute of Technology, Atlanta, United States
Haoyang Yang - Georgia Institute of Technology, Atlanta, United States
Duen Horng (Polo) Chau - Georgia Tech, Atlanta, United States
Screen-reader Accessible PDF
Download preprint PDF
Download camera-ready PDF
Room: Bayshore VI
2024-10-17T17:54:00Z GMT-0600 Change your timezone on the schedule page
2024-10-17T17:54:00Z
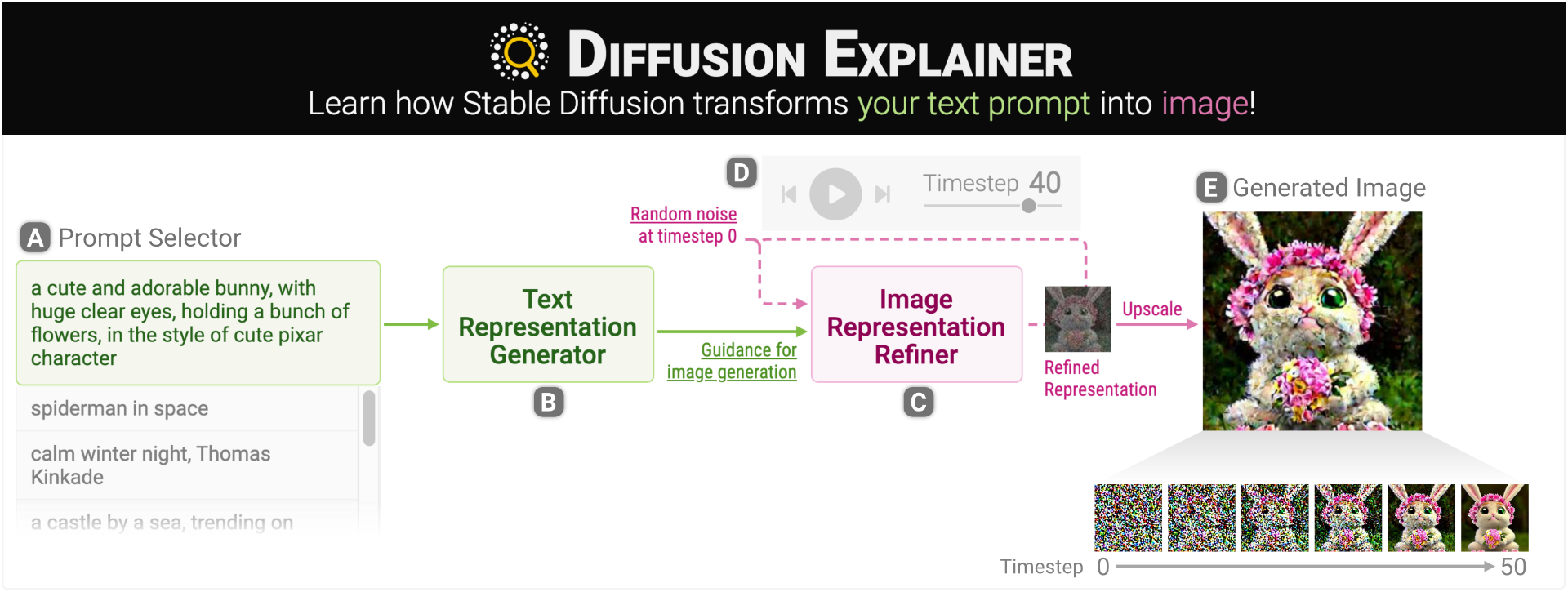
Fast forward
Keywords
Machine Learning, Statistics, Modelling, and Simulation Applications; Software Prototype
Abstract
Diffusion-based generative models’ impressive ability to create convincing images has garnered global attention. However, their complex structures and operations often pose challenges for non-experts to grasp. We present Diffusion Explainer, the first interactive visualization tool that explains how Stable Diffusion transforms text prompts into images. Diffusion Explainer tightly integrates a visual overview of Stable Diffusion’s complex structure with explanations of the underlying operations. By comparing image generation of prompt variants, users can discover the impact of keyword changes on image generation. A 56-participant user study demonstrates that Diffusion Explainer offers substantial learning benefits to non-experts. Our tool has been used by over 10,300 users from 124 countries at https://poloclub.github.io/diffusion-explainer/.
Diffusion Explainer: Visual Explanation for Text-to-image Stable Diffusion
Seongmin Lee - Georgia Tech, Atlanta, United States
Benjamin Hoover - GA Tech, Atlanta, United States. IBM Research AI, Cambridge, United States
Hendrik Strobelt - IBM Research AI, Cambridge, United States
Zijie J. Wang - Georgia Tech, Atlanta, United States
ShengYun Peng - Georgia Institute of Technology, Atlanta, United States
Austin P Wright - Georgia Institute of Technology , Atlanta , United States
Kevin Li - Georgia Institute of Technology, Atlanta, United States
Haekyu Park - Georgia Institute of Technology, Atlanta, United States
Haoyang Yang - Georgia Institute of Technology, Atlanta, United States
Duen Horng (Polo) Chau - Georgia Tech, Atlanta, United States
Screen-reader Accessible PDF
Download preprint PDF
Download camera-ready PDF
Room: Bayshore VI
2024-10-17T17:54:00Z GMT-0600 Change your timezone on the schedule page
2024-10-17T17:54:00Z
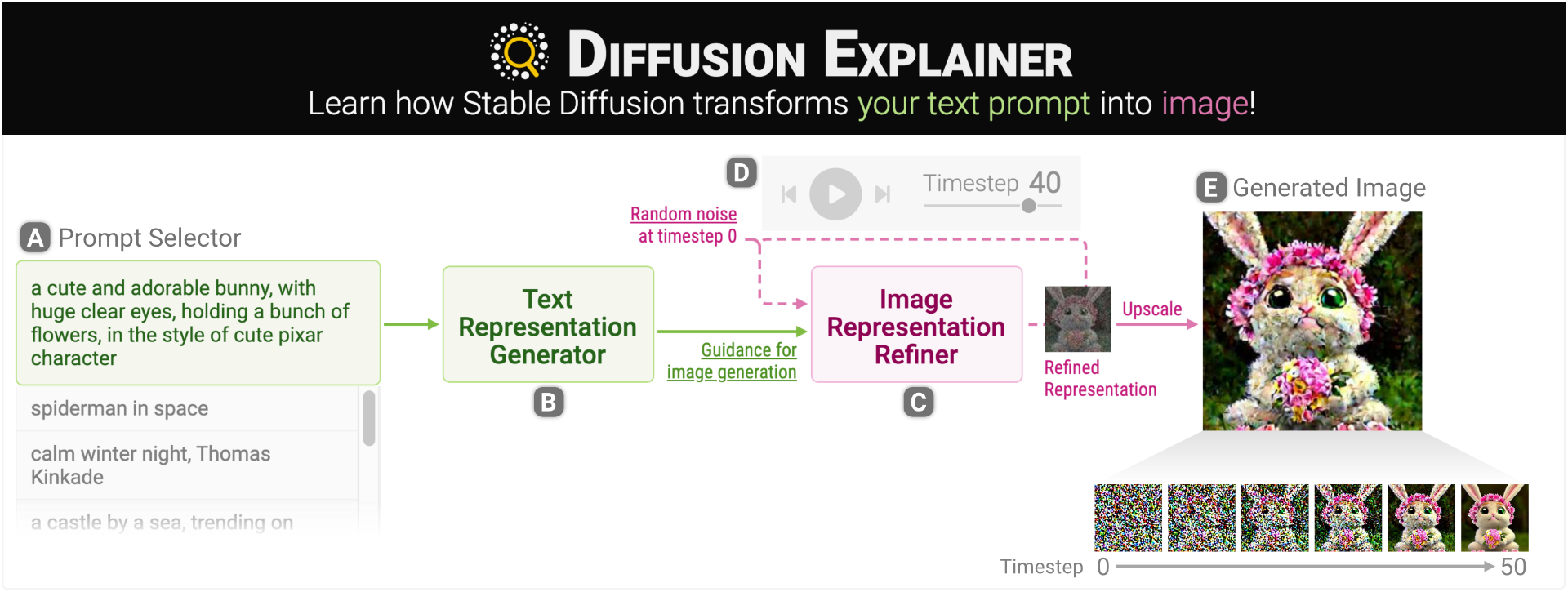
Fast forward
Keywords
Machine Learning, Statistics, Modelling, and Simulation Applications; Software Prototype
Abstract
Diffusion-based generative models’ impressive ability to create convincing images has garnered global attention. However, their complex structures and operations often pose challenges for non-experts to grasp. We present Diffusion Explainer, the first interactive visualization tool that explains how Stable Diffusion transforms text prompts into images. Diffusion Explainer tightly integrates a visual overview of Stable Diffusion’s complex structure with explanations of the underlying operations. By comparing image generation of prompt variants, users can discover the impact of keyword changes on image generation. A 56-participant user study demonstrates that Diffusion Explainer offers substantial learning benefits to non-experts. Our tool has been used by over 10,300 users from 124 countries at https://poloclub.github.io/diffusion-explainer/.
Uniform Sample Distribution in Scatterplots via Sector-based Transformation
Hennes Rave - University of Münster, Münster, Germany
Vladimir Molchanov - University of Münster, Münster, Germany
Lars Linsen - University of Münster, Münster, Germany
Download camera-ready PDF
Room: Bayshore VI
2024-10-16T13:15:00Z GMT-0600 Change your timezone on the schedule page
2024-10-16T13:15:00Z
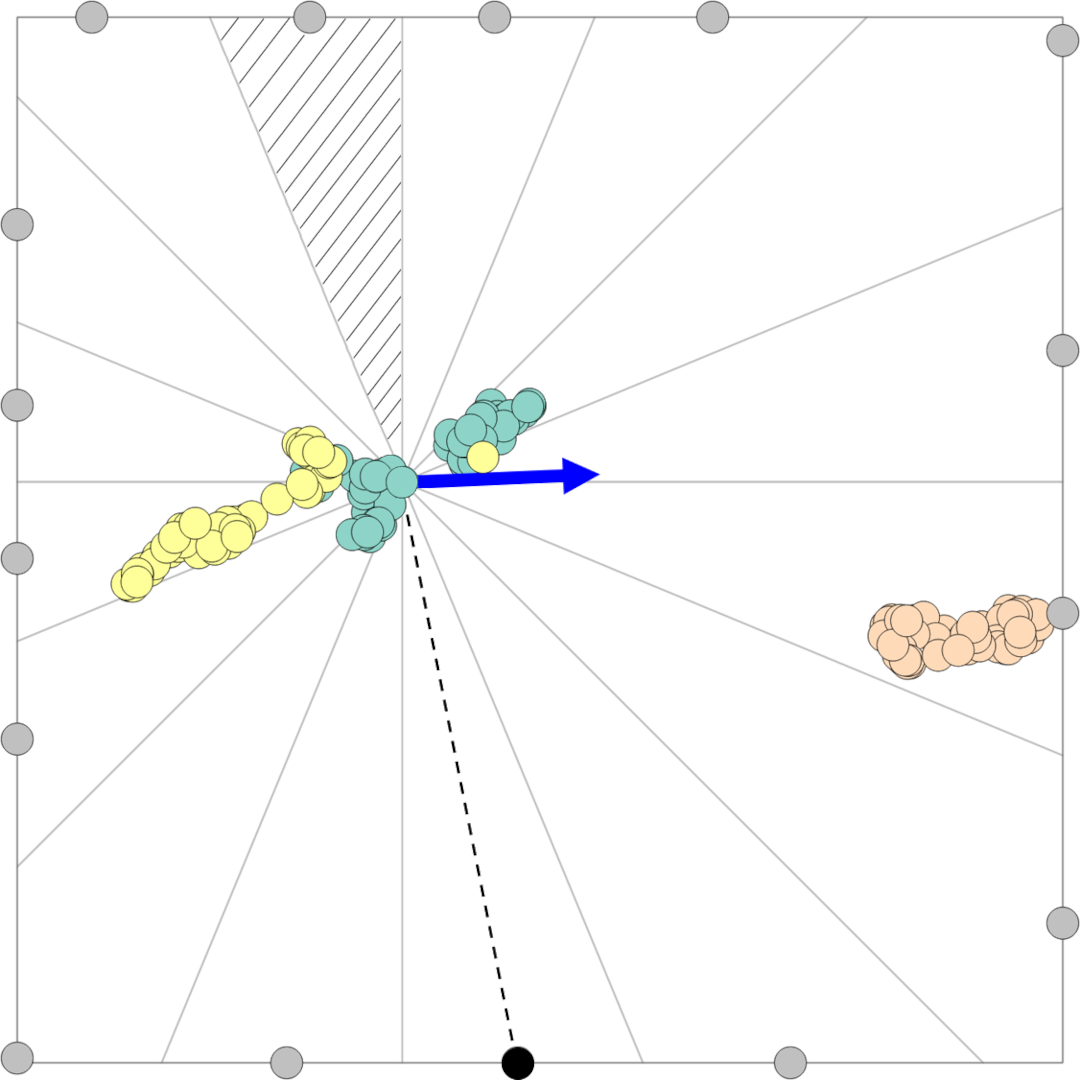
Fast forward
Keywords
Scatterplot de-cluttering, spatial transformation.
Abstract
A high number of samples often leads to occlusion in scatterplots, which hinders data perception and analysis. De-cluttering approaches based on spatial transformation reduce visual clutter by remapping samples using the entire available scatterplot domain. Such regularized scatterplots may still be used for data analysis tasks, if the spatial transformation is smooth and preserves the original neighborhood relations of samples. Recently, Rave et al. proposed an efficient regularization method based on integral images. We propose a generalization of their regularization scheme using sector-based transformations with the aim of increasing sample uniformity of the resulting scatterplot. We document the improvement of our approach using various uniformity measures.
Uniform Sample Distribution in Scatterplots via Sector-based Transformation
Hennes Rave - University of Münster, Münster, Germany
Vladimir Molchanov - University of Münster, Münster, Germany
Lars Linsen - University of Münster, Münster, Germany
Download camera-ready PDF
Room: Bayshore VI
2024-10-16T13:15:00Z GMT-0600 Change your timezone on the schedule page
2024-10-16T13:15:00Z
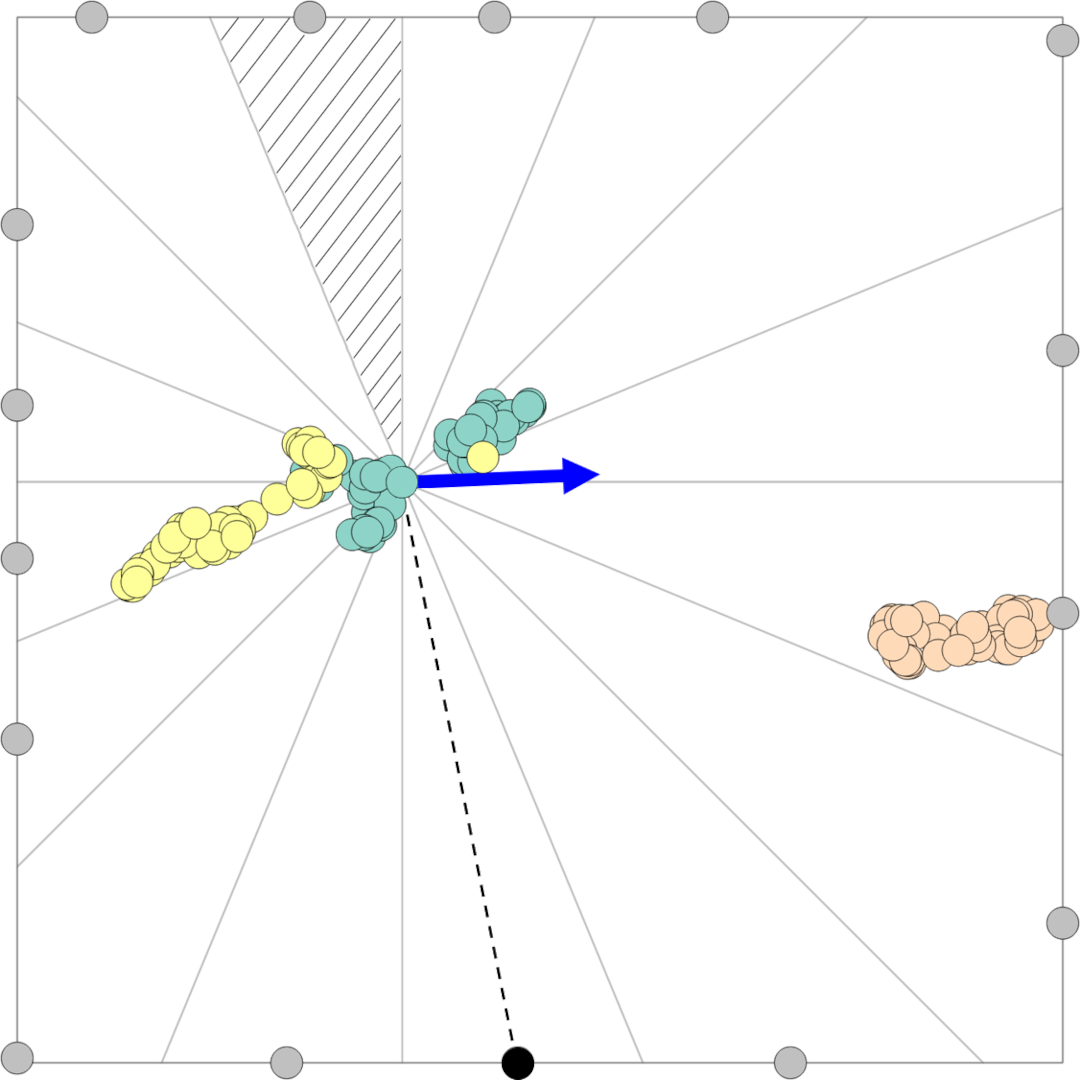
Fast forward
Keywords
Scatterplot de-cluttering, spatial transformation.
Abstract
A high number of samples often leads to occlusion in scatterplots, which hinders data perception and analysis. De-cluttering approaches based on spatial transformation reduce visual clutter by remapping samples using the entire available scatterplot domain. Such regularized scatterplots may still be used for data analysis tasks, if the spatial transformation is smooth and preserves the original neighborhood relations of samples. Recently, Rave et al. proposed an efficient regularization method based on integral images. We propose a generalization of their regularization scheme using sector-based transformations with the aim of increasing sample uniformity of the resulting scatterplot. We document the improvement of our approach using various uniformity measures.
Evaluating the Semantic Profiling Abilities of LLMs for Natural Language Utterances in Data Visualization
Hannah K. Bako - University of Maryland, College Park, United States
Arshnoor Bhutani - University of Maryland, College Park, United States
Xinyi Liu - The University of Texas at Austin, Austin, United States
Kwesi Adu Cobbina - University of Maryland, College Park, United States
Zhicheng Liu - University of Maryland, College Park, United States
Screen-reader Accessible PDF
Download preprint PDF
Download camera-ready PDF
Download Supplemental Material
Room: Bayshore VI
2024-10-17T14:33:00Z GMT-0600 Change your timezone on the schedule page
2024-10-17T14:33:00Z
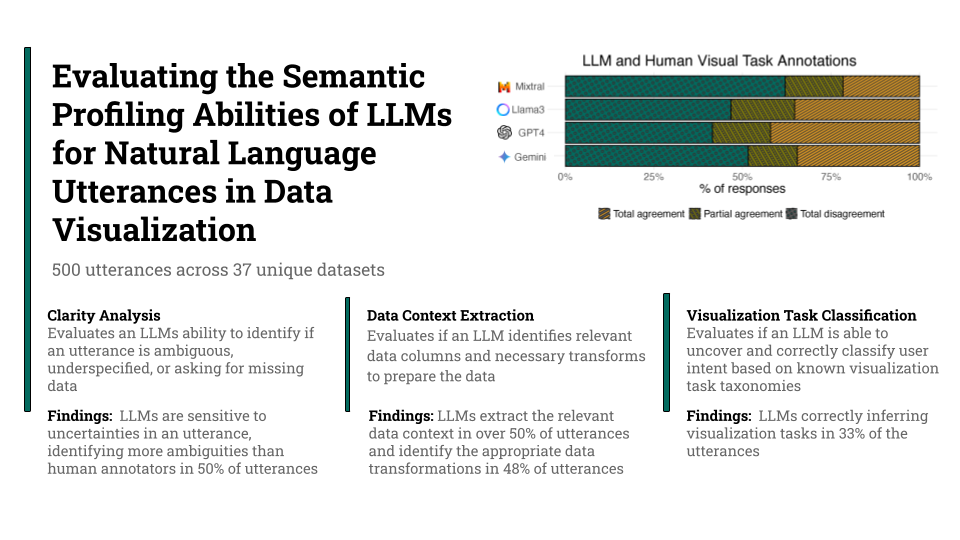
Fast forward
Keywords
Human-centered computing—Visualization—Empirical studies in visualization;
Abstract
Automatically generating data visualizations in response to human utterances on datasets necessitates a deep semantic understanding of the utterance, including implicit and explicit references to data attributes, visualization tasks, and necessary data preparation steps. Natural Language Interfaces (NLIs) for data visualization have explored ways to infer such information, yet challenges persist due to inherent uncertainty in human speech. Recent advances in Large Language Models (LLMs) provide an avenue to address these challenges, but their ability to extract the relevant semantic information remains unexplored. In this study, we evaluate four publicly available LLMs (GPT-4, Gemini-Pro, Llama3, and Mixtral), investigating their ability to comprehend utterances even in the presence of uncertainty and identify the relevant data context and visual tasks. Our findings reveal that LLMs are sensitive to uncertainties in utterances. Despite this sensitivity, they are able to extract the relevant data context. However, LLMs struggle with inferring visualization tasks. Based on these results, we highlight future research directions on using LLMs for visualization generation. Our supplementary materials have been shared on GitHub: https://github.com/hdi-umd/Semantic_Profiling_LLM_Evaluation.
Evaluating the Semantic Profiling Abilities of LLMs for Natural Language Utterances in Data Visualization
Hannah K. Bako - University of Maryland, College Park, United States
Arshnoor Bhutani - University of Maryland, College Park, United States
Xinyi Liu - The University of Texas at Austin, Austin, United States
Kwesi Adu Cobbina - University of Maryland, College Park, United States
Zhicheng Liu - University of Maryland, College Park, United States
Screen-reader Accessible PDF
Download preprint PDF
Download camera-ready PDF
Download Supplemental Material
Room: Bayshore VI
2024-10-17T14:33:00Z GMT-0600 Change your timezone on the schedule page
2024-10-17T14:33:00Z
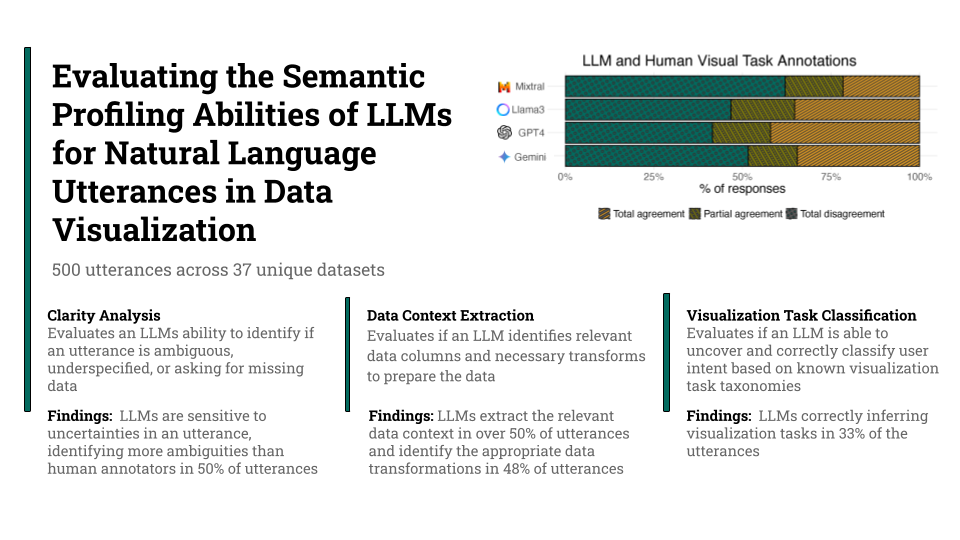
Fast forward
Keywords
Human-centered computing—Visualization—Empirical studies in visualization;
Abstract
Automatically generating data visualizations in response to human utterances on datasets necessitates a deep semantic understanding of the utterance, including implicit and explicit references to data attributes, visualization tasks, and necessary data preparation steps. Natural Language Interfaces (NLIs) for data visualization have explored ways to infer such information, yet challenges persist due to inherent uncertainty in human speech. Recent advances in Large Language Models (LLMs) provide an avenue to address these challenges, but their ability to extract the relevant semantic information remains unexplored. In this study, we evaluate four publicly available LLMs (GPT-4, Gemini-Pro, Llama3, and Mixtral), investigating their ability to comprehend utterances even in the presence of uncertainty and identify the relevant data context and visual tasks. Our findings reveal that LLMs are sensitive to uncertainties in utterances. Despite this sensitivity, they are able to extract the relevant data context. However, LLMs struggle with inferring visualization tasks. Based on these results, we highlight future research directions on using LLMs for visualization generation. Our supplementary materials have been shared on GitHub: https://github.com/hdi-umd/Semantic_Profiling_LLM_Evaluation.
Guided Statistical Workflows with Interactive Explanations and Assumption Checking
Yuqi Zhang - New York University, New York, United States
Adam Perer - Carnegie Mellon University, Pittsburgh, United States
Will Epperson - Carnegie Mellon University, Pittsburgh, United States
Screen-reader Accessible PDF
Download camera-ready PDF
Room: Bayshore VI
2024-10-16T18:12:00Z GMT-0600 Change your timezone on the schedule page
2024-10-16T18:12:00Z
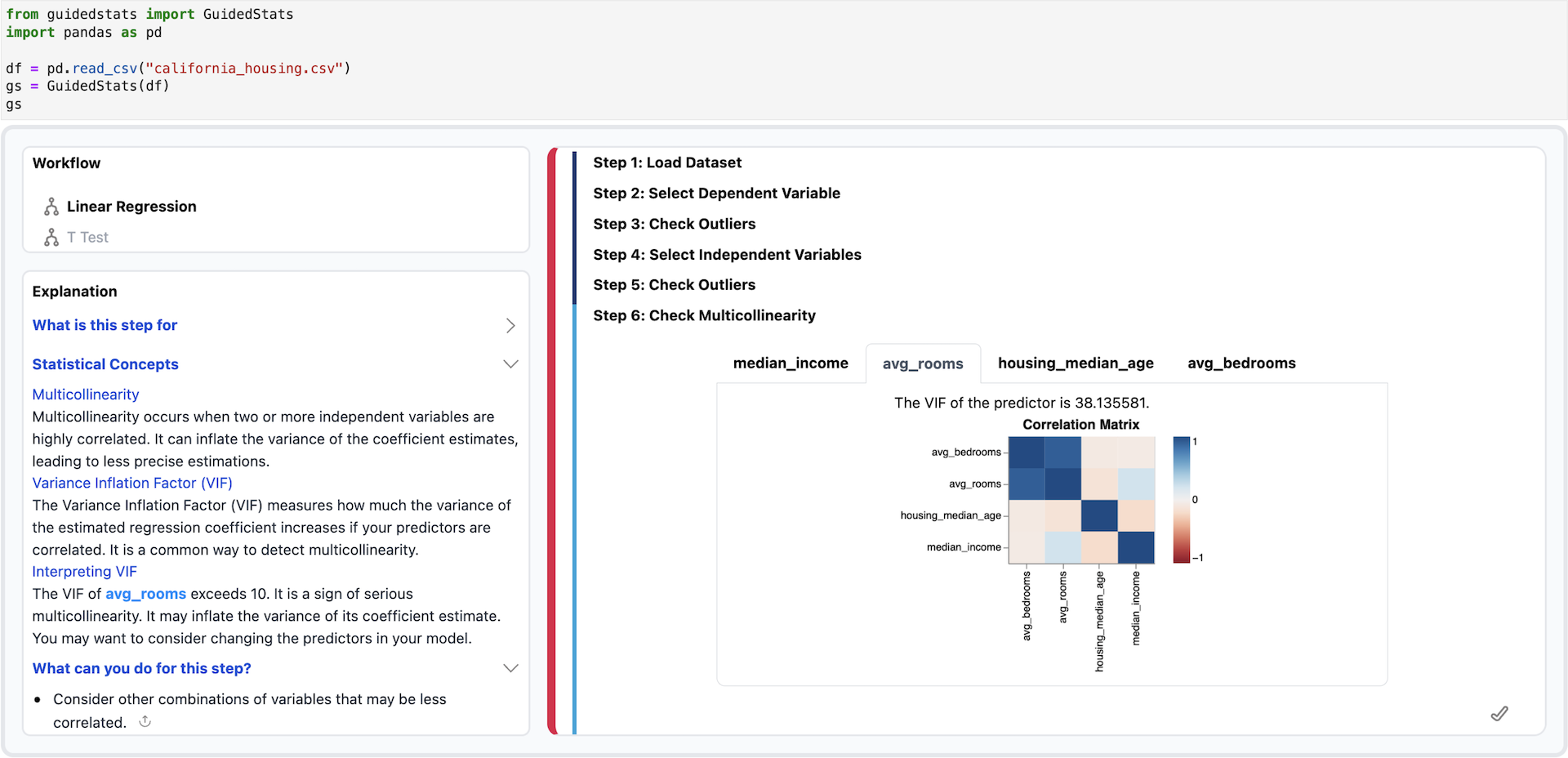
Fast forward
Keywords
Data science tools, computational notebooks, analytical guidance
Abstract
Statistical practices such as building regression models or running hypothesis tests rely on following rigorous procedures of steps and verifying assumptions on data to produce valid results. However, common statistical tools do not verify users’ decision choices and provide low-level statistical functions without instructions on the whole analysis practice. Users can easily misuse analysis methods, potentially decreasing the validity of results. To address this problem, we introduce GuidedStats, an interactive interface within computational notebooks that encapsulates guidance, models, visualization, and exportable results into interactive workflows. It breaks down typical analysis processes, such as linear regression and two-sample T-tests, into interactive steps supplemented with automatic visualizations and explanations for step-wise evaluation. Users can iterate on input choices to refine their models, while recommended actions and exports allow the user to continue their analysis in code. Case studies show how GuidedStats offers valuable instructions for conducting fluid statistical analyses while finding possible assumption violations in the underlying data, supporting flexible and accurate statistical analyses.
Guided Statistical Workflows with Interactive Explanations and Assumption Checking
Yuqi Zhang - New York University, New York, United States
Adam Perer - Carnegie Mellon University, Pittsburgh, United States
Will Epperson - Carnegie Mellon University, Pittsburgh, United States
Screen-reader Accessible PDF
Download camera-ready PDF
Room: Bayshore VI
2024-10-16T18:12:00Z GMT-0600 Change your timezone on the schedule page
2024-10-16T18:12:00Z
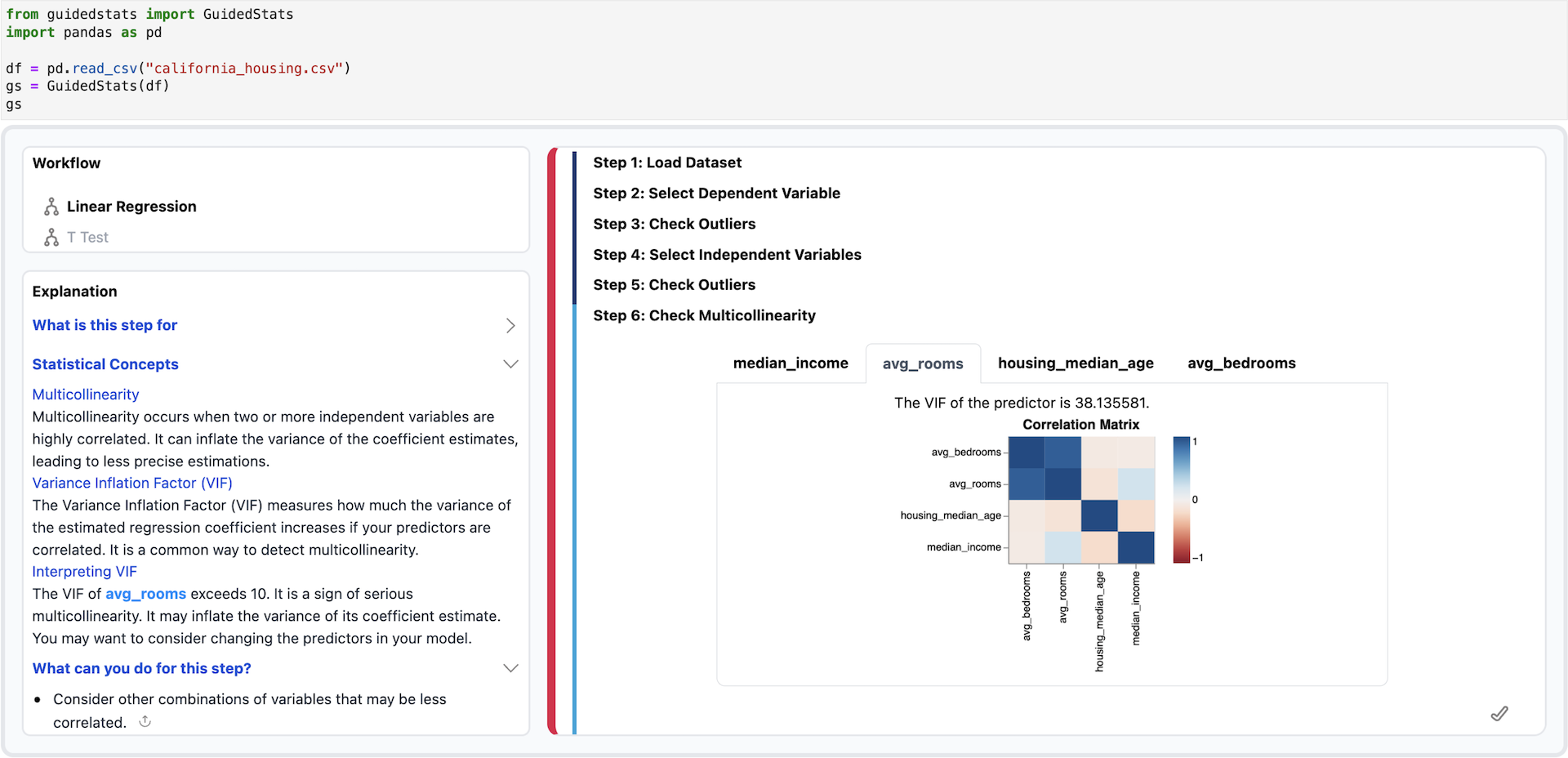
Fast forward
Keywords
Data science tools, computational notebooks, analytical guidance
Abstract
Statistical practices such as building regression models or running hypothesis tests rely on following rigorous procedures of steps and verifying assumptions on data to produce valid results. However, common statistical tools do not verify users’ decision choices and provide low-level statistical functions without instructions on the whole analysis practice. Users can easily misuse analysis methods, potentially decreasing the validity of results. To address this problem, we introduce GuidedStats, an interactive interface within computational notebooks that encapsulates guidance, models, visualization, and exportable results into interactive workflows. It breaks down typical analysis processes, such as linear regression and two-sample T-tests, into interactive steps supplemented with automatic visualizations and explanations for step-wise evaluation. Users can iterate on input choices to refine their models, while recommended actions and exports allow the user to continue their analysis in code. Case studies show how GuidedStats offers valuable instructions for conducting fluid statistical analyses while finding possible assumption violations in the underlying data, supporting flexible and accurate statistical analyses.
Demystifying Spatial Dependence: Interactive Visualizations for Interpreting Local Spatial Autocorrelation
Lee Mason - NIH, Rockville, United States. Queen's University, Belfast, United Kingdom
Blánaid Hicks - Queen's University Belfast , Belfast , United Kingdom
Jonas S Almeida - National Institutes of Health, Rockville, United States
Download preprint PDF
Download camera-ready PDF
Download Supplemental Material
Room: Bayshore VI
2024-10-17T16:36:00Z GMT-0600 Change your timezone on the schedule page
2024-10-17T16:36:00Z
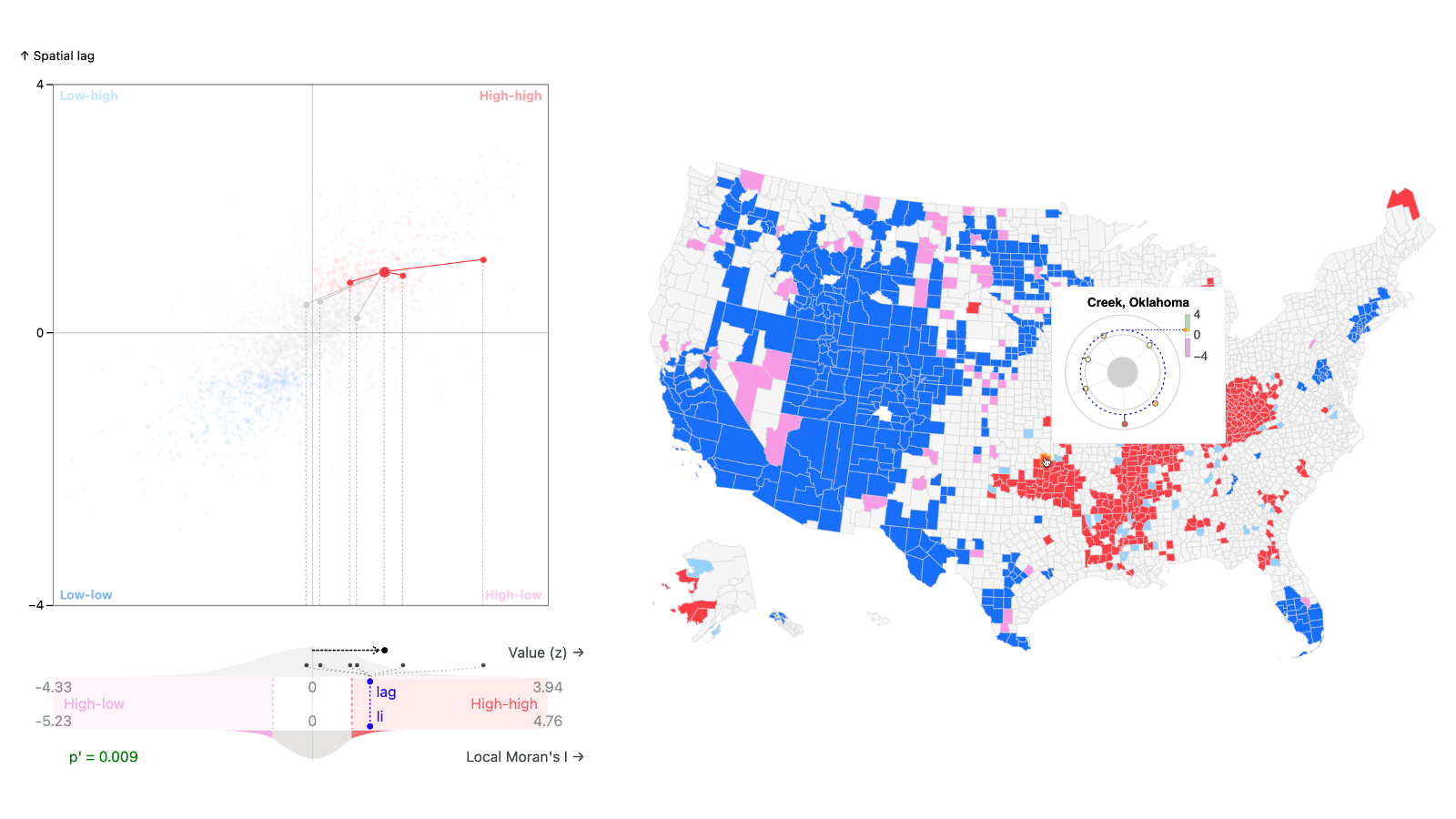
Fast forward
Keywords
Spatial, spatial clustering, spatial autocorrelation, geospatial, GIS, interactive visualization, visual analytics, Moran's I, local indicators of spatial association
Abstract
The Local Moran's I statistic is a valuable tool for identifying localized patterns of spatial autocorrelation. Understanding these patterns is crucial in spatial analysis, but interpreting the statistic can be difficult. To simplify this process, we introduce three novel visualizations that enhance the interpretation of Local Moran's I results. These visualizations can be interactively linked to one another, and to established visualizations, to offer a more holistic exploration of the results. We provide a JavaScript library with implementations of these new visual elements, along with a web dashboard that demonstrates their integrated use.
Demystifying Spatial Dependence: Interactive Visualizations for Interpreting Local Spatial Autocorrelation
Lee Mason - NIH, Rockville, United States. Queen's University, Belfast, United Kingdom
Blánaid Hicks - Queen's University Belfast , Belfast , United Kingdom
Jonas S Almeida - National Institutes of Health, Rockville, United States
Download preprint PDF
Download camera-ready PDF
Download Supplemental Material
Room: Bayshore VI
2024-10-17T16:36:00Z GMT-0600 Change your timezone on the schedule page
2024-10-17T16:36:00Z
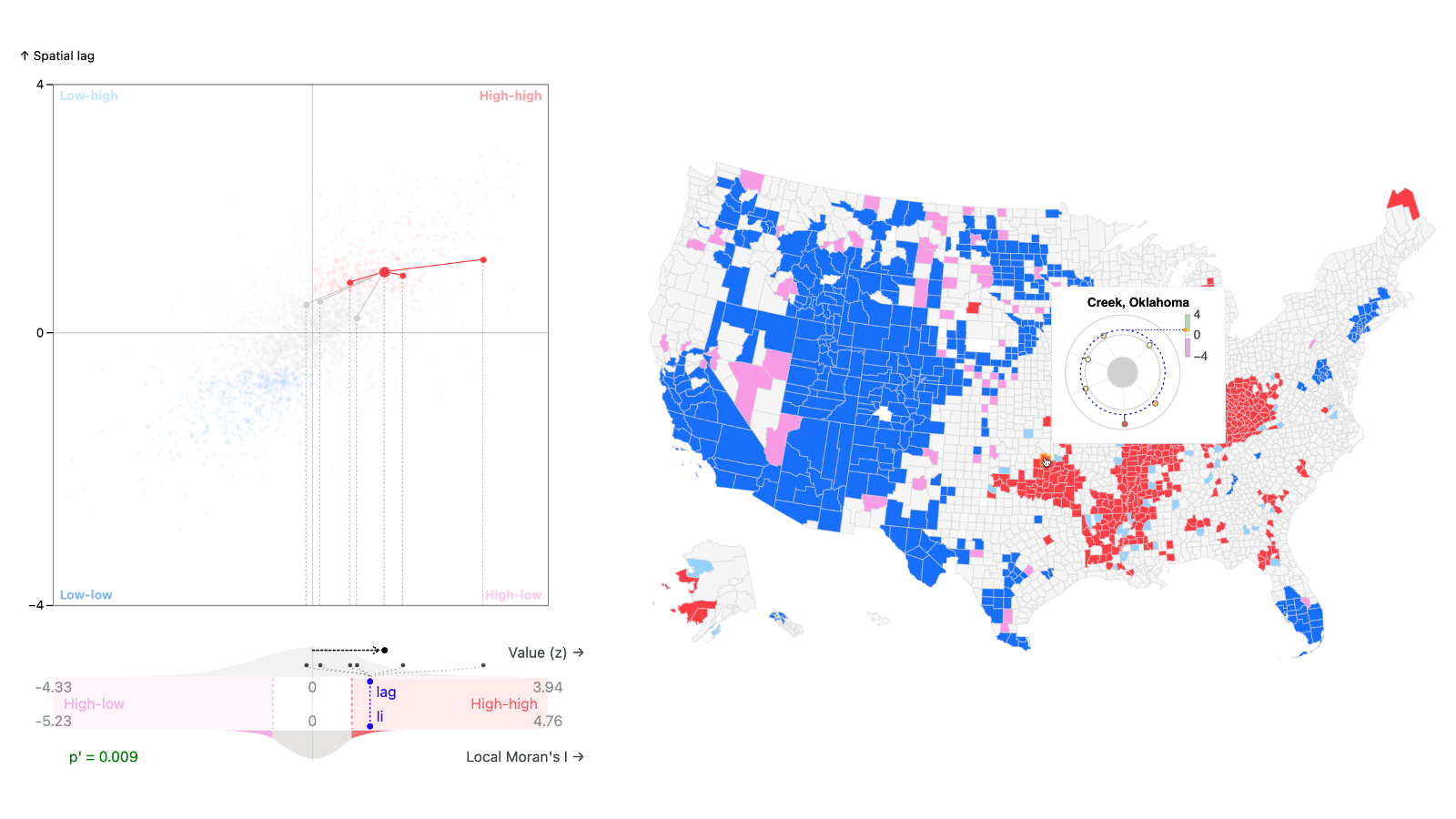
Fast forward
Keywords
Spatial, spatial clustering, spatial autocorrelation, geospatial, GIS, interactive visualization, visual analytics, Moran's I, local indicators of spatial association
Abstract
The Local Moran's I statistic is a valuable tool for identifying localized patterns of spatial autocorrelation. Understanding these patterns is crucial in spatial analysis, but interpreting the statistic can be difficult. To simplify this process, we introduce three novel visualizations that enhance the interpretation of Local Moran's I results. These visualizations can be interactively linked to one another, and to established visualizations, to offer a more holistic exploration of the results. We provide a JavaScript library with implementations of these new visual elements, along with a web dashboard that demonstrates their integrated use.
Dark Mode or Light Mode? Exploring the Impact of Contrast Polarity on Visualization Performance Between Age Groups
Zack While - University of Massachusetts Amherst, Amherst, United States
Ali Sarvghad - University of Massachusetts Amherst, Amherst, United States
Download camera-ready PDF
Download Supplemental Material
Room: Bayshore VI
2024-10-17T12:30:00Z GMT-0600 Change your timezone on the schedule page
2024-10-17T12:30:00Z
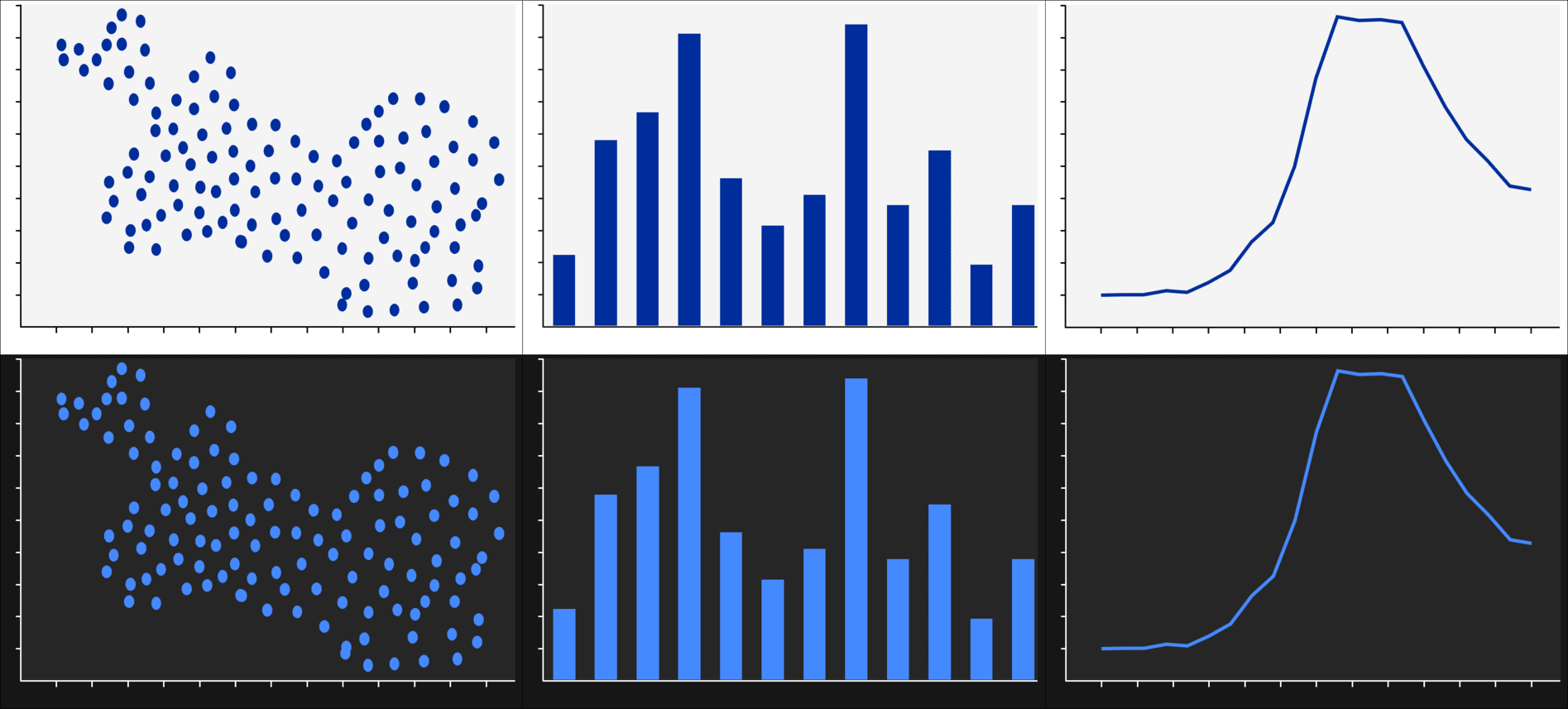
Fast forward
Keywords
people in late adulthood, GerontoVis, data visualization, contrast polarity
Abstract
This study examines the impact of positive and negative contrast polarities (i.e., light and dark modes) on the performance of younger adults and people in their late adulthood (PLA). In a crowdsourced study with 134 participants (69 below age 60, 66 aged 60 and above), we assessed their accuracy and time performing analysis tasks across three common visualization types (Bar, Line, Scatterplot) and two contrast polarities (positive and negative). We observed that, across both age groups, the polarity that led to better performance and the resulting amount of improvement varied on an individual basis, with each polarity benefiting comparable proportions of participants. However, the contrast polarity that led to better performance did not always match their preferred polarity. Additionally, we observed that the choice of contrast polarity can have an impact on time similar to that of the choice of visualization type, resulting in an average percent difference of around 36%. These findings indicate that, overall, the effects of contrast polarity on visual analysis performance do not noticeably change with age. Furthermore, they underscore the importance of making visualizations available in both contrast polarities to better-support a broad audience with differing needs. Supplementary materials for this work can be found at https://osf.io/539a4/.
Dark Mode or Light Mode? Exploring the Impact of Contrast Polarity on Visualization Performance Between Age Groups
Zack While - University of Massachusetts Amherst, Amherst, United States
Ali Sarvghad - University of Massachusetts Amherst, Amherst, United States
Download camera-ready PDF
Download Supplemental Material
Room: Bayshore VI
2024-10-17T12:30:00Z GMT-0600 Change your timezone on the schedule page
2024-10-17T12:30:00Z
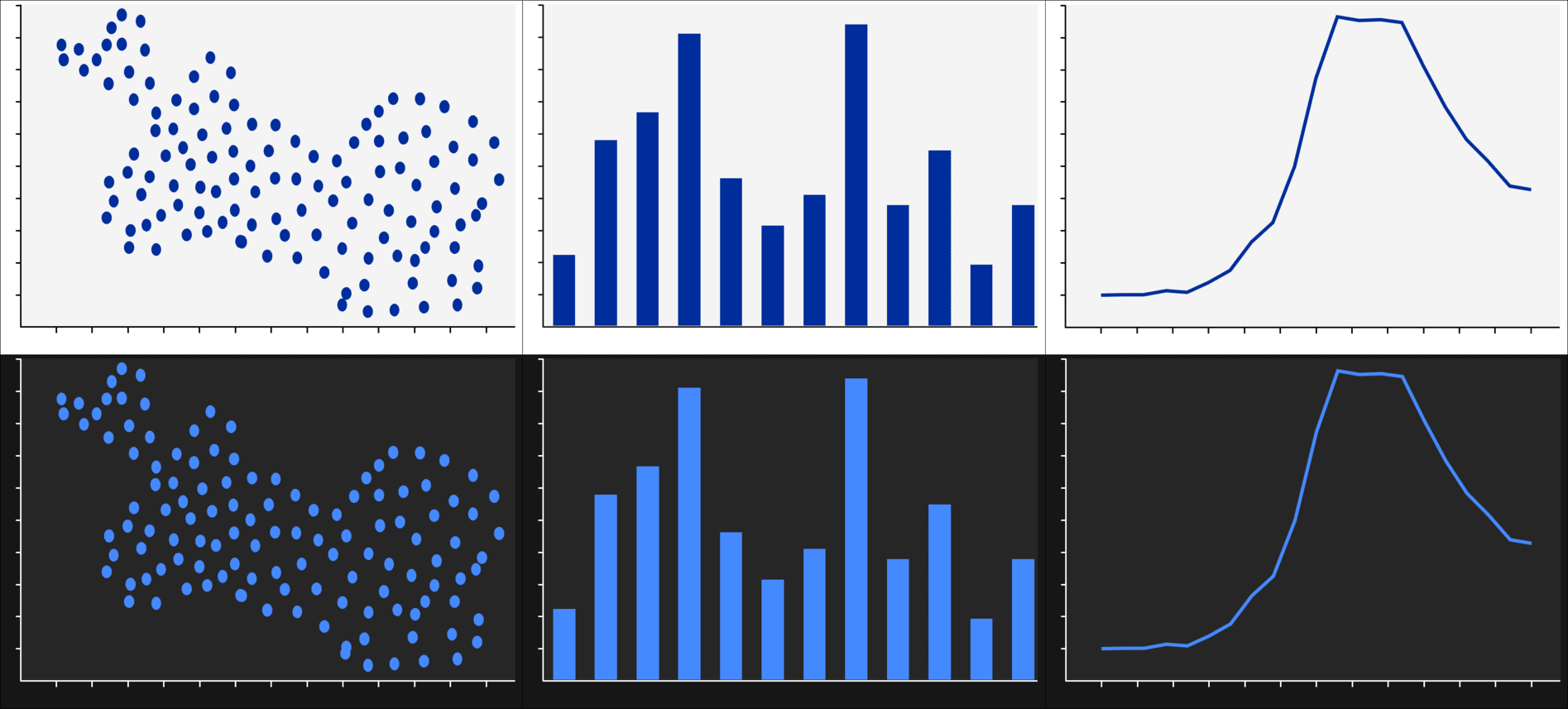
Fast forward
Keywords
people in late adulthood, GerontoVis, data visualization, contrast polarity
Abstract
This study examines the impact of positive and negative contrast polarities (i.e., light and dark modes) on the performance of younger adults and people in their late adulthood (PLA). In a crowdsourced study with 134 participants (69 below age 60, 66 aged 60 and above), we assessed their accuracy and time performing analysis tasks across three common visualization types (Bar, Line, Scatterplot) and two contrast polarities (positive and negative). We observed that, across both age groups, the polarity that led to better performance and the resulting amount of improvement varied on an individual basis, with each polarity benefiting comparable proportions of participants. However, the contrast polarity that led to better performance did not always match their preferred polarity. Additionally, we observed that the choice of contrast polarity can have an impact on time similar to that of the choice of visualization type, resulting in an average percent difference of around 36%. These findings indicate that, overall, the effects of contrast polarity on visual analysis performance do not noticeably change with age. Furthermore, they underscore the importance of making visualizations available in both contrast polarities to better-support a broad audience with differing needs. Supplementary materials for this work can be found at https://osf.io/539a4/.
Representing Charts as Text for Language Models: An In-Depth Study of Question Answering for Bar Charts
Victor S. Bursztyn - Adobe Research, San Jose, United States
Jane Hoffswell - Adobe Research, Seattle, United States
Shunan Guo - Adobe Research, San Jose, United States
Eunyee Koh - Adobe Research, San Jose, United States
Screen-reader Accessible PDF
Download camera-ready PDF
Download Supplemental Material
Room: Bayshore VI
2024-10-17T14:42:00Z GMT-0600 Change your timezone on the schedule page
2024-10-17T14:42:00Z

Fast forward
Keywords
Machine Learning Techniques; Charts, Diagrams, and Plots; Datasets; Computational Benchmark Studies
Abstract
Machine Learning models for chart-grounded Q&A (CQA) often treat charts as images, but performing CQA on pixel values has proven challenging. We thus investigate a resource overlooked by current ML-based approaches: the declarative documents describing how charts should visually encode data (i.e., chart specifications). In this work, we use chart specifications to enhance language models (LMs) for chart-reading tasks, such that the resulting system can robustly understand language for CQA. Through a case study with 359 bar charts, we test novel fine tuning schemes on both GPT-3 and T5 using a new dataset curated for two CQA tasks: question-answering and visual explanation generation. Our text-only approaches strongly outperform vision-based GPT-4 on explanation generation (99% vs. 63% accuracy), and show promising results for question-answering (57-67% accuracy). Through in-depth experiments, we also show that our text-only approaches are mostly robust to natural language variation.
Representing Charts as Text for Language Models: An In-Depth Study of Question Answering for Bar Charts
Victor S. Bursztyn - Adobe Research, San Jose, United States
Jane Hoffswell - Adobe Research, Seattle, United States
Shunan Guo - Adobe Research, San Jose, United States
Eunyee Koh - Adobe Research, San Jose, United States
Screen-reader Accessible PDF
Download camera-ready PDF
Download Supplemental Material
Room: Bayshore VI
2024-10-17T14:42:00Z GMT-0600 Change your timezone on the schedule page
2024-10-17T14:42:00Z

Fast forward
Keywords
Machine Learning Techniques; Charts, Diagrams, and Plots; Datasets; Computational Benchmark Studies
Abstract
Machine Learning models for chart-grounded Q&A (CQA) often treat charts as images, but performing CQA on pixel values has proven challenging. We thus investigate a resource overlooked by current ML-based approaches: the declarative documents describing how charts should visually encode data (i.e., chart specifications). In this work, we use chart specifications to enhance language models (LMs) for chart-reading tasks, such that the resulting system can robustly understand language for CQA. Through a case study with 359 bar charts, we test novel fine tuning schemes on both GPT-3 and T5 using a new dataset curated for two CQA tasks: question-answering and visual explanation generation. Our text-only approaches strongly outperform vision-based GPT-4 on explanation generation (99% vs. 63% accuracy), and show promising results for question-answering (57-67% accuracy). Through in-depth experiments, we also show that our text-only approaches are mostly robust to natural language variation.
Building and Eroding: Exogenous and Endogenous Factors that Influence Subjective Trust in Visualization
R. Jordan Crouser - Smith College, Northampton, United States
Syrine Matoussi - Smith College, Northampton, United States
Lan Kung - Smith College, Northampton, United States
Saugat Pandey - Washington University in St. Louis, St. Louis, United States
Oen G McKinley - Washington University in St. Louis, St. Louis, United States
Alvitta Ottley - Washington University in St. Louis, St. Louis, United States
Screen-reader Accessible PDF
Download preprint PDF
Download camera-ready PDF
Download Supplemental Material
Room: Palma Ceia I
2024-10-16T13:21:00Z GMT-0600 Change your timezone on the schedule page
2024-10-16T13:21:00Z

Fast forward
Keywords
Trust, data visualization, individual differences, personality
Abstract
Trust is a subjective yet fundamental component of human-computer interaction, and is a determining factor in shaping the efficacy of data visualizations. Prior research has identified five dimensions of trust assessment in visualizations (credibility, clarity, reliability, familiarity, and confidence), and observed that these dimensions tend to vary predictably along with certain features of the visualization being evaluated. This raises a further question: how do the design features driving viewers' trust assessment vary with the characteristics of the viewers themselves? By reanalyzing data from these studies through the lens of individual differences, we build a more detailed map of the relationships between design features, individual characteristics, and trust behaviors. In particular, we model the distinct contributions of endogenous design features (such as visualization type, or the use of color) and exogenous user characteristics (such as visualization literacy), as well as the interactions between them. We then use these findings to make recommendations for individualized and adaptive visualization design.
Building and Eroding: Exogenous and Endogenous Factors that Influence Subjective Trust in Visualization
R. Jordan Crouser - Smith College, Northampton, United States
Syrine Matoussi - Smith College, Northampton, United States
Lan Kung - Smith College, Northampton, United States
Saugat Pandey - Washington University in St. Louis, St. Louis, United States
Oen G McKinley - Washington University in St. Louis, St. Louis, United States
Alvitta Ottley - Washington University in St. Louis, St. Louis, United States
Screen-reader Accessible PDF
Download preprint PDF
Download camera-ready PDF
Download Supplemental Material
Room: Palma Ceia I
2024-10-16T13:21:00Z GMT-0600 Change your timezone on the schedule page
2024-10-16T13:21:00Z

Fast forward
Keywords
Trust, data visualization, individual differences, personality
Abstract
Trust is a subjective yet fundamental component of human-computer interaction, and is a determining factor in shaping the efficacy of data visualizations. Prior research has identified five dimensions of trust assessment in visualizations (credibility, clarity, reliability, familiarity, and confidence), and observed that these dimensions tend to vary predictably along with certain features of the visualization being evaluated. This raises a further question: how do the design features driving viewers' trust assessment vary with the characteristics of the viewers themselves? By reanalyzing data from these studies through the lens of individual differences, we build a more detailed map of the relationships between design features, individual characteristics, and trust behaviors. In particular, we model the distinct contributions of endogenous design features (such as visualization type, or the use of color) and exogenous user characteristics (such as visualization literacy), as well as the interactions between them. We then use these findings to make recommendations for individualized and adaptive visualization design.
"Must Be a Tuesday": Affect, Attribution, and Geographic Variability in Equity-Oriented Visualizations of Population Health Disparities
Eli Holder - 3iap, Raleigh, United States
Lace M. Padilla - Northeastern University, Boston, United States. University of California Merced, Merced, United States
Download camera-ready PDF
Download Supplemental Material
Room: Bayshore VI
2024-10-17T16:27:00Z GMT-0600 Change your timezone on the schedule page
2024-10-17T16:27:00Z
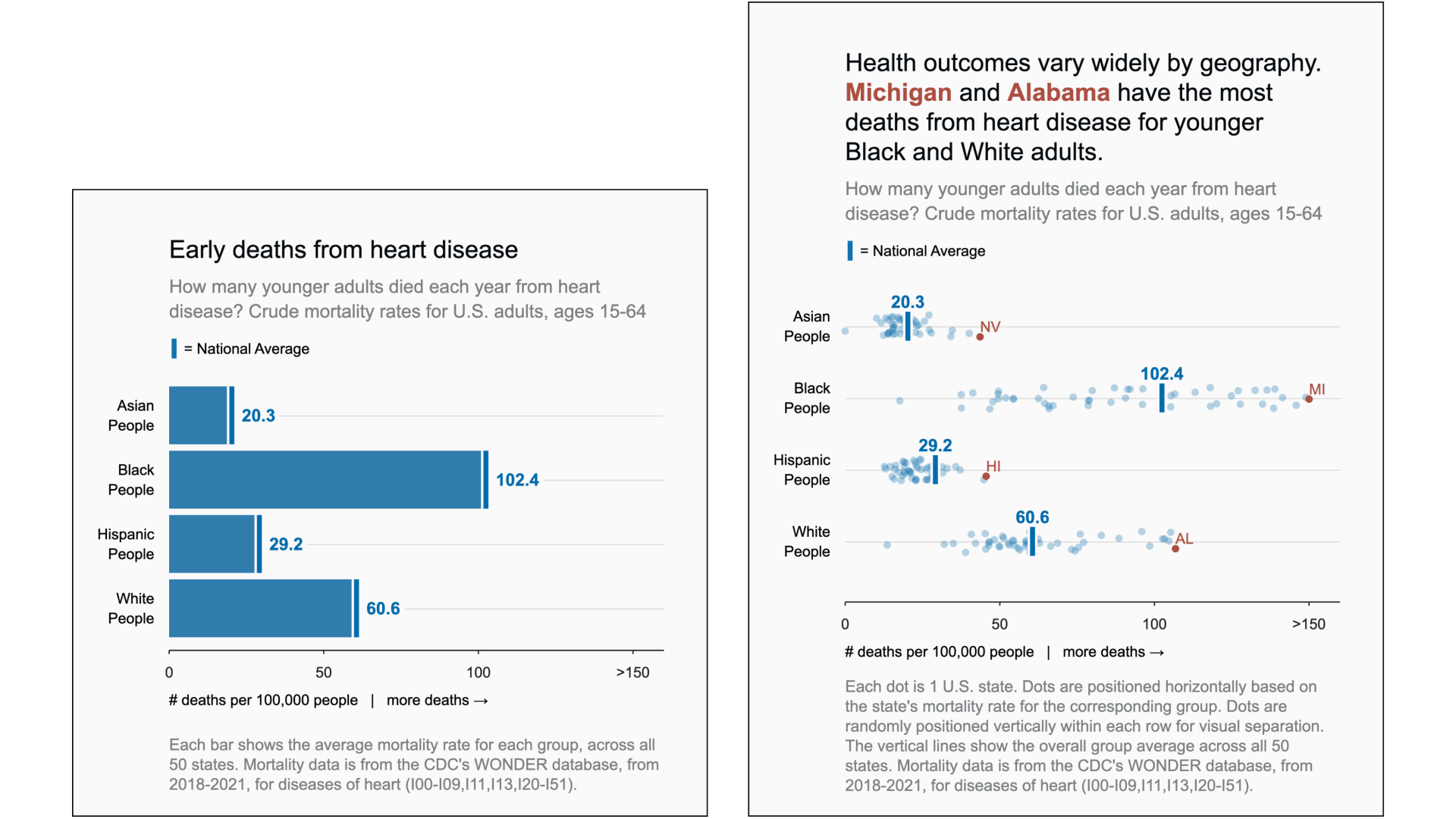
Fast forward
Keywords
Health Equity, Public Health Communication
Abstract
This study examines the impacts of public health communications visualizing risk disparities between racial and other social groups. It compares the effects of traditional bar charts to an alternative design emphasizing geographic variability with differing annotations and jitter plots. Whereas both visualization designs increased perceived vulnerability, behavioral intent, and policy support, the geo-emphasized charts were significantly more effective in reducing personal attribution biases. The findings also reveal emotionally taxing experiences for chart viewers from marginalized communities. This work suggests a need for strategic reevaluation of visual communication tools in public health to enhance understanding and engagement without reinforcing stereotypes or emotional distress.
"Must Be a Tuesday": Affect, Attribution, and Geographic Variability in Equity-Oriented Visualizations of Population Health Disparities
Eli Holder - 3iap, Raleigh, United States
Lace M. Padilla - Northeastern University, Boston, United States. University of California Merced, Merced, United States
Download camera-ready PDF
Download Supplemental Material
Room: Bayshore VI
2024-10-17T16:27:00Z GMT-0600 Change your timezone on the schedule page
2024-10-17T16:27:00Z
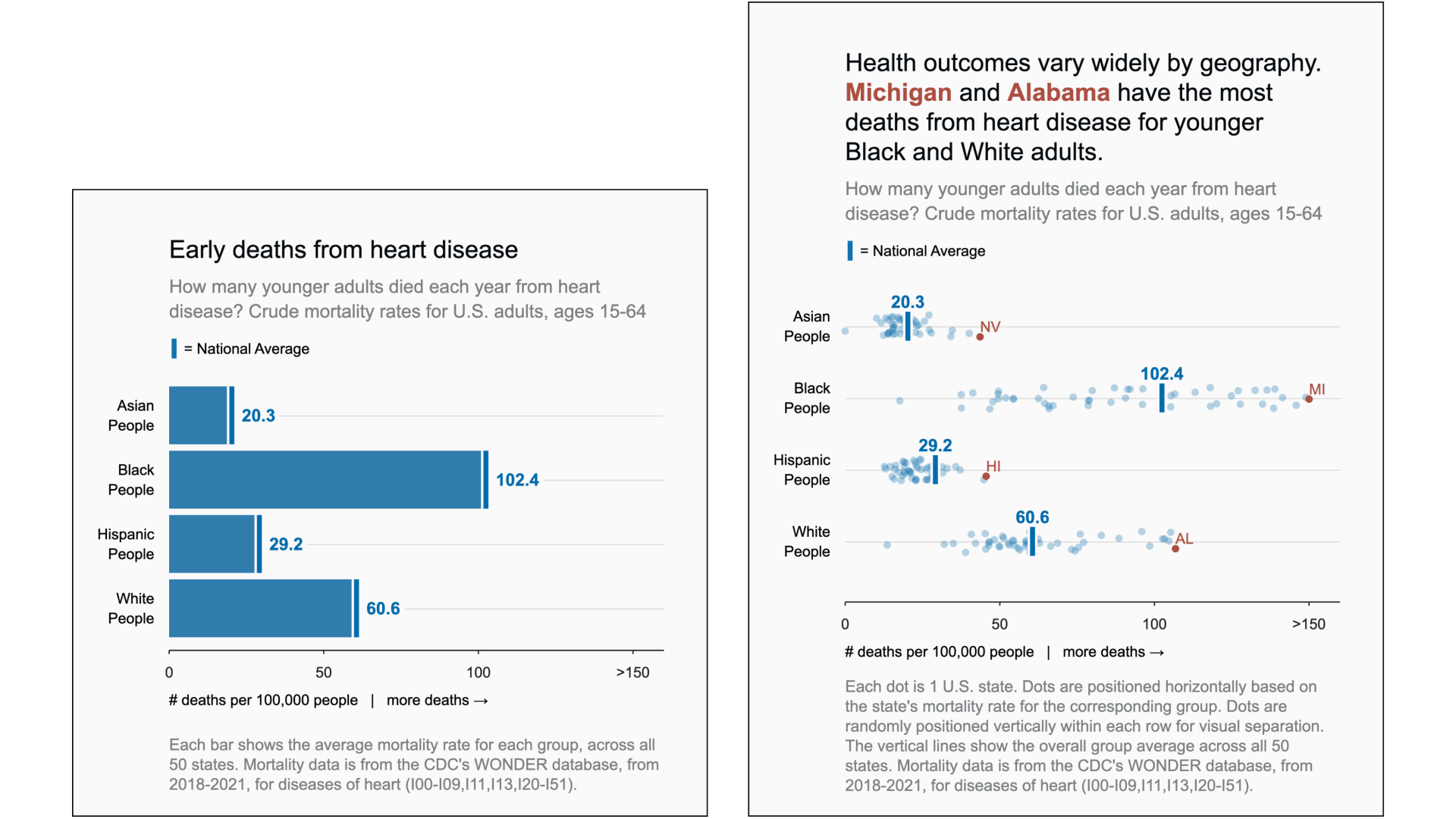
Fast forward
Keywords
Health Equity, Public Health Communication
Abstract
This study examines the impacts of public health communications visualizing risk disparities between racial and other social groups. It compares the effects of traditional bar charts to an alternative design emphasizing geographic variability with differing annotations and jitter plots. Whereas both visualization designs increased perceived vulnerability, behavioral intent, and policy support, the geo-emphasized charts were significantly more effective in reducing personal attribution biases. The findings also reveal emotionally taxing experiences for chart viewers from marginalized communities. This work suggests a need for strategic reevaluation of visual communication tools in public health to enhance understanding and engagement without reinforcing stereotypes or emotional distress.
Multi-User Mobile Augmented Reality for Cardiovascular Surgical Planning
Pratham Darrpan Mehta - Georgia Tech, Atlanta, United States
Rahul Ozhur Narayanan - Georgia Tech, Atlanta, United States
Harsha Karanth - Georgia Tech, Atlanta, United States
Haoyang Yang - Georgia Institute of Technology, Atlanta, United States
Timothy C Slesnick - Emory University, Atlanta, United States
Fawwaz Shaw - Emory University/Children's Healthcare of Atlanta, Atlanta, United States
Duen Horng (Polo) Chau - Georgia Tech, Atlanta, United States
Screen-reader Accessible PDF
Download preprint PDF
Download camera-ready PDF
Download Supplemental Material
Room: Bayshore VI
2024-10-16T16:54:00Z GMT-0600 Change your timezone on the schedule page
2024-10-16T16:54:00Z
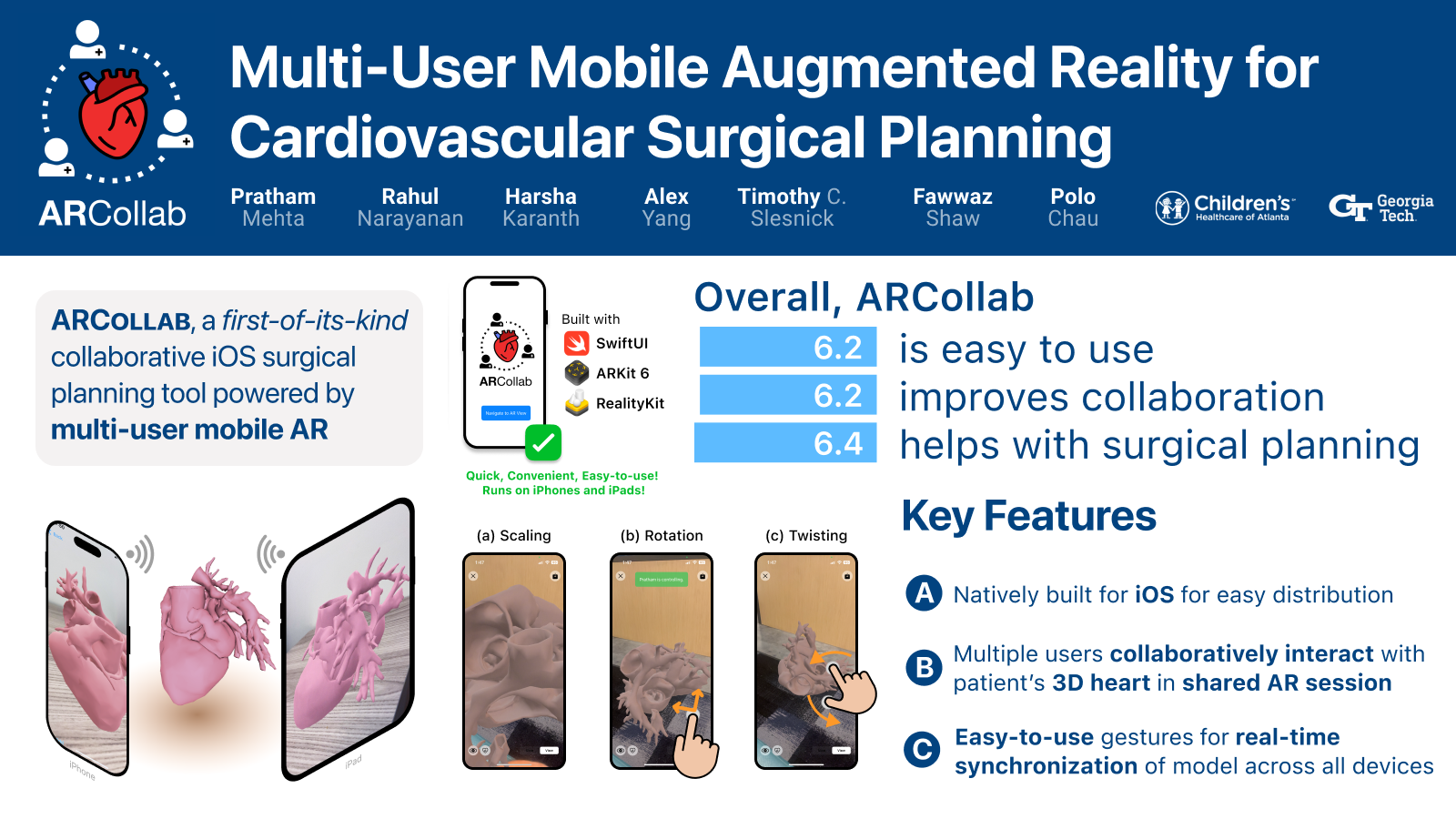
Fast forward
Keywords
Augmented Reality, Mobile Collaboration, Surgical Planning
Abstract
Collaborative planning for congenital heart diseases typically involves creating physical heart models through 3D printing, which are then examined by both surgeons and cardiologists. Recent developments in mobile augmented reality (AR) technologies have presented a viable alternative, known for their ease of use and portability. However, there is still a lack of research examining the utilization of multi-user mobile AR environments to support collaborative planning for cardiovascular surgeries. We created ARCollab, an iOS AR app designed for enabling multiple surgeons and cardiologists to interact with a patient's 3D heart model in a shared environment. ARCollab enables surgeons and cardiologists to import heart models, manipulate them through gestures and collaborate with other users, eliminating the need for fabricating physical heart models. Our evaluation of ARCollab's usability and usefulnessin enhancing collaboration, conducted with three cardiothoracic surgeons and two cardiologists, marks the first human evaluation of a multi-user mobile AR tool for surgical planning. ARCollab is open-source, available at https://github.com/poloclub/arcollab.
Multi-User Mobile Augmented Reality for Cardiovascular Surgical Planning
Pratham Darrpan Mehta - Georgia Tech, Atlanta, United States
Rahul Ozhur Narayanan - Georgia Tech, Atlanta, United States
Harsha Karanth - Georgia Tech, Atlanta, United States
Haoyang Yang - Georgia Institute of Technology, Atlanta, United States
Timothy C Slesnick - Emory University, Atlanta, United States
Fawwaz Shaw - Emory University/Children's Healthcare of Atlanta, Atlanta, United States
Duen Horng (Polo) Chau - Georgia Tech, Atlanta, United States
Screen-reader Accessible PDF
Download preprint PDF
Download camera-ready PDF
Download Supplemental Material
Room: Bayshore VI
2024-10-16T16:54:00Z GMT-0600 Change your timezone on the schedule page
2024-10-16T16:54:00Z
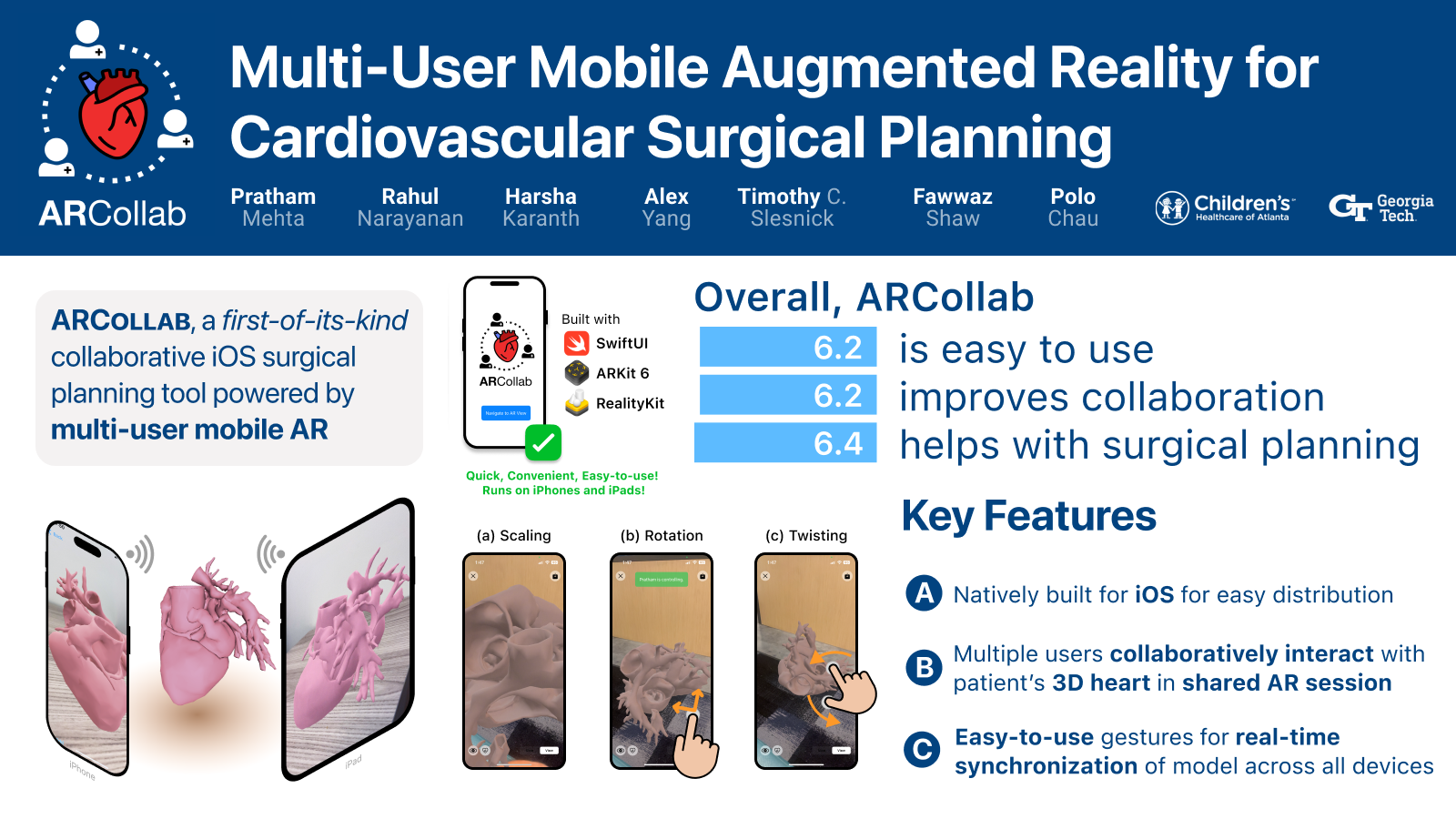
Fast forward
Keywords
Augmented Reality, Mobile Collaboration, Surgical Planning
Abstract
Collaborative planning for congenital heart diseases typically involves creating physical heart models through 3D printing, which are then examined by both surgeons and cardiologists. Recent developments in mobile augmented reality (AR) technologies have presented a viable alternative, known for their ease of use and portability. However, there is still a lack of research examining the utilization of multi-user mobile AR environments to support collaborative planning for cardiovascular surgeries. We created ARCollab, an iOS AR app designed for enabling multiple surgeons and cardiologists to interact with a patient's 3D heart model in a shared environment. ARCollab enables surgeons and cardiologists to import heart models, manipulate them through gestures and collaborate with other users, eliminating the need for fabricating physical heart models. Our evaluation of ARCollab's usability and usefulnessin enhancing collaboration, conducted with three cardiothoracic surgeons and two cardiologists, marks the first human evaluation of a multi-user mobile AR tool for surgical planning. ARCollab is open-source, available at https://github.com/poloclub/arcollab.
Zoomable Level-of-Detail ChartTables for Interpreting Probabilistic Model Outputs for Reactionary Train Delays
Aidan Slingsby - City, University of London, London, United Kingdom
Jonathan Hyde - Risk Solutions, Warrington, United Kingdom
Download preprint PDF
Download camera-ready PDF
Download Supplemental Material
Room: Bayshore VI
2024-10-17T13:24:00Z GMT-0600 Change your timezone on the schedule page
2024-10-17T13:24:00Z
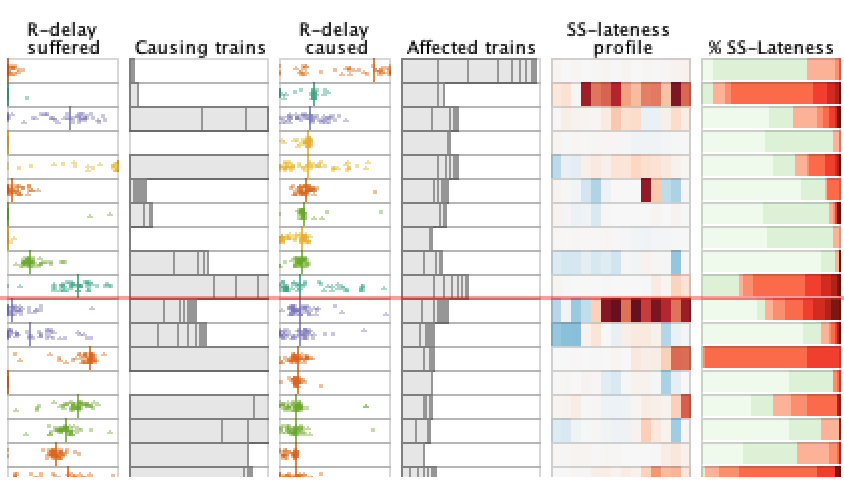
Fast forward
Keywords
Level-of-detail, mini-charts, distributions, stochastic modelling.
Abstract
"Reactionary delay" is a result of the accumulated cascading effects of knock-on train delays which is increasing on UK railways due to increasing utilisation of the railway infrastructure. The chaotic nature of its effects on train lateness is notoriously hard to predict. We use a stochastic Monte-Carto-style simulation of reactionary delay that produces whole distributions of likely reactionary delay and delays this causes. We demonstrate how Zoomable Level-of-Detail ChartTables - case-by-variable tables where cases are rows, variables are columns, variables are complex composite metrics that incorporate distributions, and cells contain mini-charts that depict these as different levels of detail through zoom interaction - help interpret whole distributions of model outputs to help understand the causes and effects of reactionary delay, how they inform timetable robustness testing, and how they could be used in other contexts.
Zoomable Level-of-Detail ChartTables for Interpreting Probabilistic Model Outputs for Reactionary Train Delays
Aidan Slingsby - City, University of London, London, United Kingdom
Jonathan Hyde - Risk Solutions, Warrington, United Kingdom
Download preprint PDF
Download camera-ready PDF
Download Supplemental Material
Room: Bayshore VI
2024-10-17T13:24:00Z GMT-0600 Change your timezone on the schedule page
2024-10-17T13:24:00Z
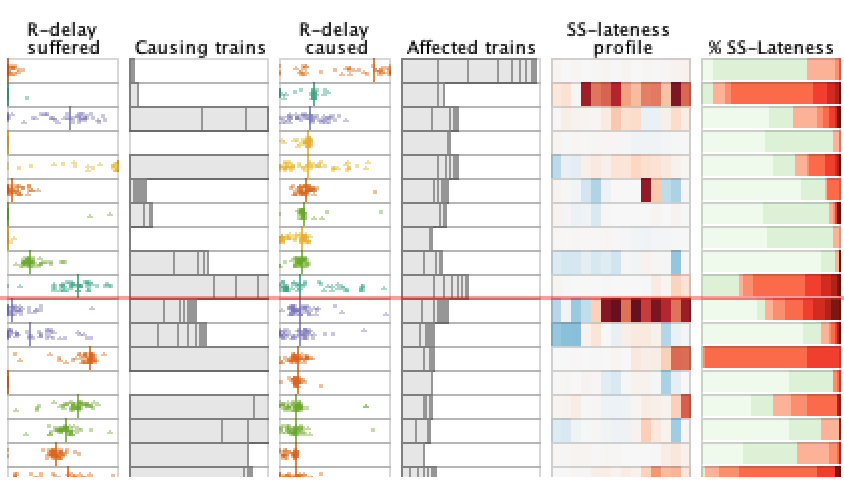
Fast forward
Keywords
Level-of-detail, mini-charts, distributions, stochastic modelling.
Abstract
"Reactionary delay" is a result of the accumulated cascading effects of knock-on train delays which is increasing on UK railways due to increasing utilisation of the railway infrastructure. The chaotic nature of its effects on train lateness is notoriously hard to predict. We use a stochastic Monte-Carto-style simulation of reactionary delay that produces whole distributions of likely reactionary delay and delays this causes. We demonstrate how Zoomable Level-of-Detail ChartTables - case-by-variable tables where cases are rows, variables are columns, variables are complex composite metrics that incorporate distributions, and cells contain mini-charts that depict these as different levels of detail through zoom interaction - help interpret whole distributions of model outputs to help understand the causes and effects of reactionary delay, how they inform timetable robustness testing, and how they could be used in other contexts.
Evaluating Graphical Perception of Visual Motion for Quantitative Data Encoding
Shaghayegh Esmaeili -
Samia Kabir -
Anthony M. Colas -
Rhema P. Linder -
Eric D. Ragan -
Screen-reader Accessible PDF
DOI: 10.1109/TVCG.2022.3193756
Room: Bayshore III
2024-10-17T17:57:00Z GMT-0600 Change your timezone on the schedule page
2024-10-17T17:57:00Z
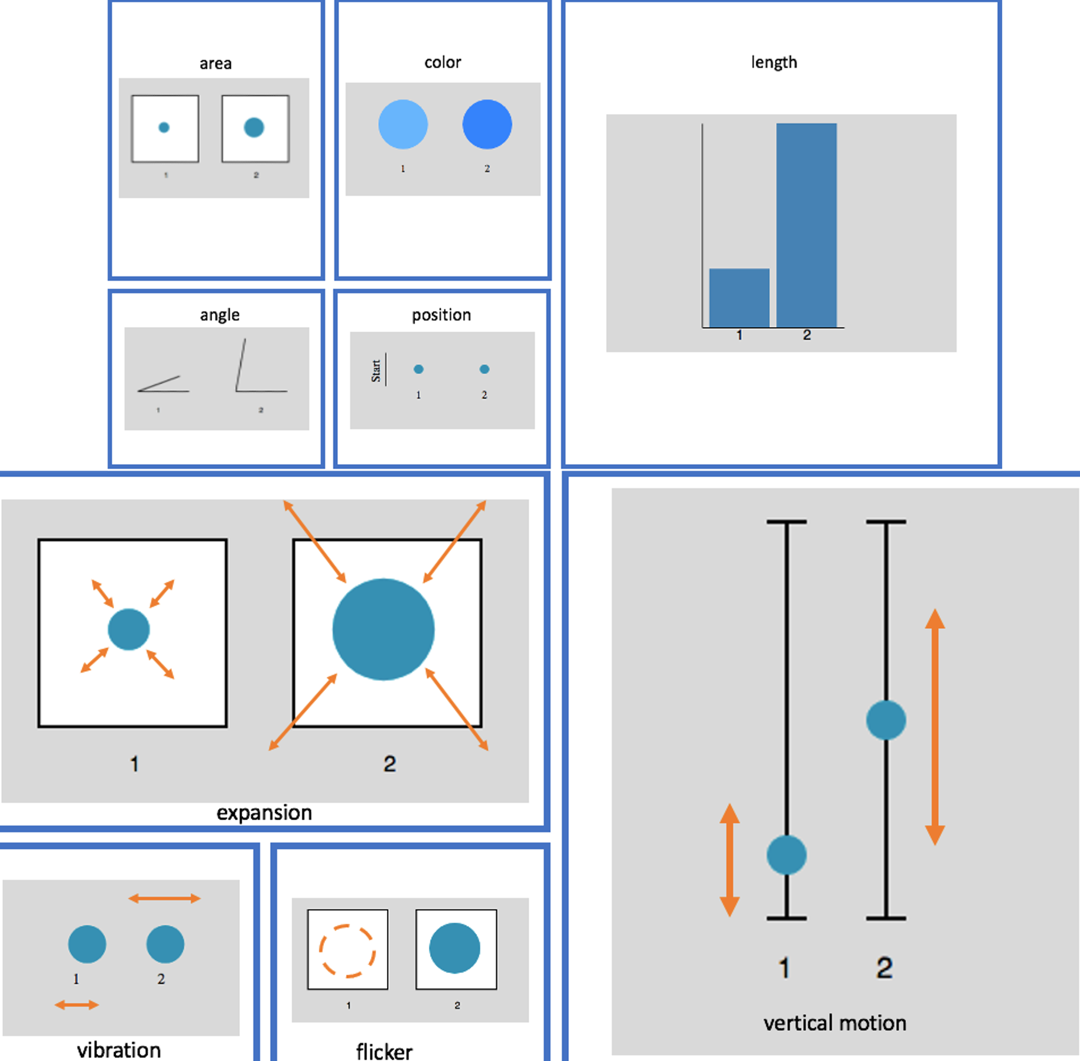
Fast forward
Keywords
Information visualization, animation and motion-related techniques, empirical study, graphical perception, evaluation.
Abstract
Information visualization uses various types of representations to encode data into graphical formats. Prior work on visualization techniques has evaluated the accuracy of perceived numerical data values from visual data encodings such as graphical position, length, orientation, size, and color. Our work aims to extend the research of graphical perception to the use of motion as data encodings for quantitative values. We present two experiments implementing multiple fundamental aspects of motion such as type, speed, and synchronicity that can be used for numerical value encoding as well as comparing motion to static visual encodings in terms of user perception and accuracy. We studied how well users can assess the differences between several types of motion and static visual encodings and present an updated ranking of accuracy for quantitative judgments. Our results indicate that non-synchronized motion can be interpreted more quickly and more accurately than synchronized motion. Moreover, our ranking of static and motion visual representations shows that motion, especially expansion and translational types, has great potential as a data encoding technique for quantitative value. Finally, we discuss the implications for the use of animation and motion for numerical representations in data visualization.
Evaluating Graphical Perception of Visual Motion for Quantitative Data Encoding
Shaghayegh Esmaeili -
Samia Kabir -
Anthony M. Colas -
Rhema P. Linder -
Eric D. Ragan -
Screen-reader Accessible PDF
DOI: 10.1109/TVCG.2022.3193756
Room: Bayshore III
2024-10-17T17:57:00Z GMT-0600 Change your timezone on the schedule page
2024-10-17T17:57:00Z
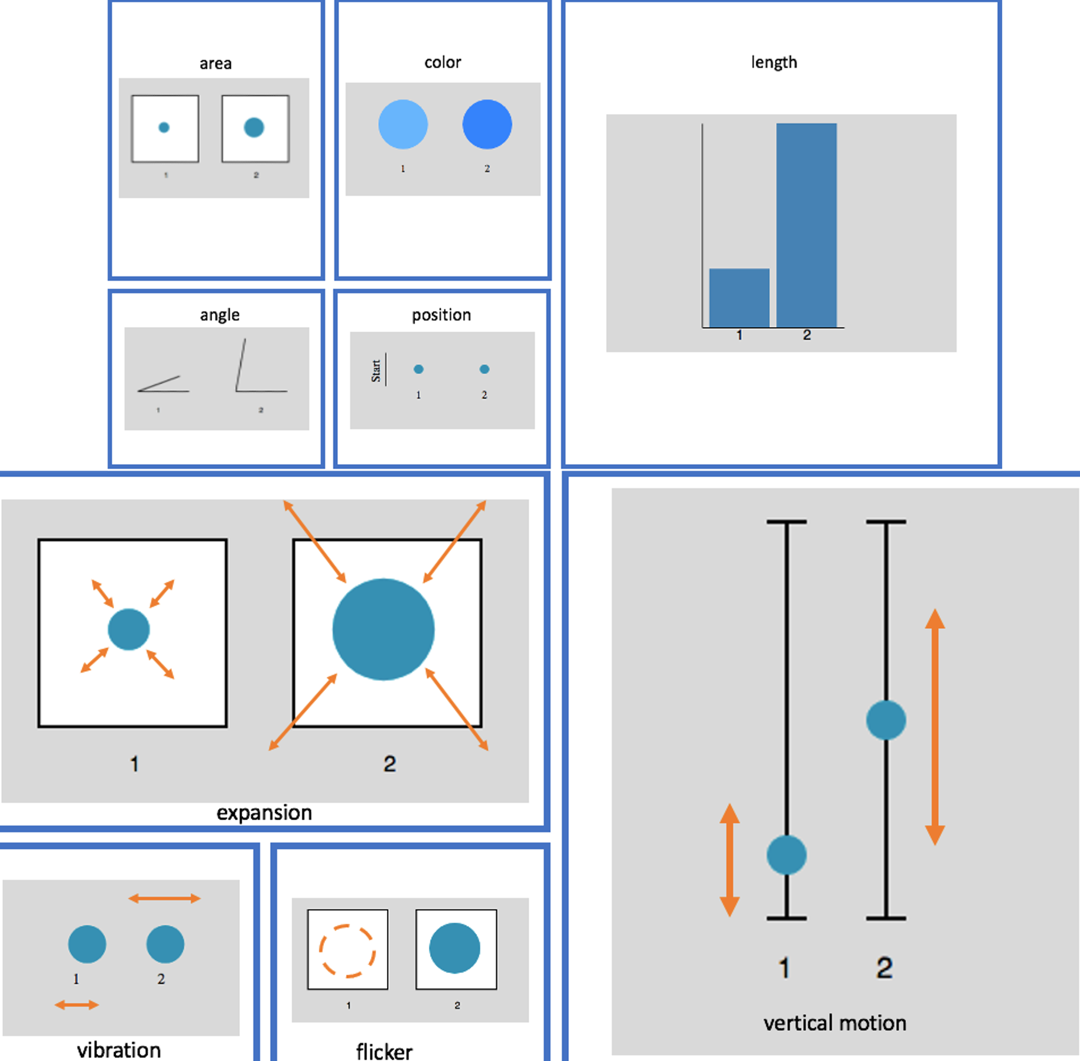
Fast forward
Keywords
Information visualization, animation and motion-related techniques, empirical study, graphical perception, evaluation.
Abstract
Information visualization uses various types of representations to encode data into graphical formats. Prior work on visualization techniques has evaluated the accuracy of perceived numerical data values from visual data encodings such as graphical position, length, orientation, size, and color. Our work aims to extend the research of graphical perception to the use of motion as data encodings for quantitative values. We present two experiments implementing multiple fundamental aspects of motion such as type, speed, and synchronicity that can be used for numerical value encoding as well as comparing motion to static visual encodings in terms of user perception and accuracy. We studied how well users can assess the differences between several types of motion and static visual encodings and present an updated ranking of accuracy for quantitative judgments. Our results indicate that non-synchronized motion can be interpreted more quickly and more accurately than synchronized motion. Moreover, our ranking of static and motion visual representations shows that motion, especially expansion and translational types, has great potential as a data encoding technique for quantitative value. Finally, we discuss the implications for the use of animation and motion for numerical representations in data visualization.
V-Mail: 3D-Enabled Correspondence about Spatial Data on (Almost) All Your Devices
Jung Who Nam -
Tobias Isenberg -
Daniel F. Keefe -
Download preprint PDF
DOI: 10.1109/TVCG.2022.3229017
Room: Bayshore V
2024-10-16T16:24:00Z GMT-0600 Change your timezone on the schedule page
2024-10-16T16:24:00Z

Fast forward
Keywords
Human-computer interaction, visualization of scientific 3D data, communication, storytelling, immersive analytics
Abstract
We present V-Mail, a framework of cross-platform applications, interactive techniques, and communication protocols for improved multi-person correspondence about spatial 3D datasets. Inspired by the daily use of e-mail, V-Mail seeks to enable a similar style of rapid, multi-person communication accessible on any device; however, it aims to do this in the new context of spatial 3D communication, where limited access to 3D graphics hardware typically prevents such communication. The approach integrates visual data storytelling with data exploration, spatial annotations, and animated transitions. V-Mail ``data stories'' are exported in a standard video file format to establish a common baseline level of access on (almost) any device. The V-Mail framework also includes a series of complementary client applications and plugins that enable different degrees of story co-authoring and data exploration, adjusted automatically to match the capabilities of various devices. A lightweight, phone-based V-Mail app makes it possible to annotate data by adding captions to the video. These spatial annotations are then immediately accessible to team members running high-end 3D graphics visualization systems that also include a V-Mail client, implemented as a plugin. Results and evaluation from applying V-Mail to assist communication within an interdisciplinary science team studying Antarctic ice sheets confirm the utility of the asynchronous, cross-platform collaborative framework while also highlighting some current limitations and opportunities for future work.
V-Mail: 3D-Enabled Correspondence about Spatial Data on (Almost) All Your Devices
Jung Who Nam -
Tobias Isenberg -
Daniel F. Keefe -
Download preprint PDF
DOI: 10.1109/TVCG.2022.3229017
Room: Bayshore V
2024-10-16T16:24:00Z GMT-0600 Change your timezone on the schedule page
2024-10-16T16:24:00Z

Fast forward
Keywords
Human-computer interaction, visualization of scientific 3D data, communication, storytelling, immersive analytics
Abstract
We present V-Mail, a framework of cross-platform applications, interactive techniques, and communication protocols for improved multi-person correspondence about spatial 3D datasets. Inspired by the daily use of e-mail, V-Mail seeks to enable a similar style of rapid, multi-person communication accessible on any device; however, it aims to do this in the new context of spatial 3D communication, where limited access to 3D graphics hardware typically prevents such communication. The approach integrates visual data storytelling with data exploration, spatial annotations, and animated transitions. V-Mail ``data stories'' are exported in a standard video file format to establish a common baseline level of access on (almost) any device. The V-Mail framework also includes a series of complementary client applications and plugins that enable different degrees of story co-authoring and data exploration, adjusted automatically to match the capabilities of various devices. A lightweight, phone-based V-Mail app makes it possible to annotate data by adding captions to the video. These spatial annotations are then immediately accessible to team members running high-end 3D graphics visualization systems that also include a V-Mail client, implemented as a plugin. Results and evaluation from applying V-Mail to assist communication within an interdisciplinary science team studying Antarctic ice sheets confirm the utility of the asynchronous, cross-platform collaborative framework while also highlighting some current limitations and opportunities for future work.
How Does Automation Shape the Process of Narrative Visualization: A Survey of Tools
Qing Chen -
Shixiong Cao -
Jiazhe Wang -
Nan Cao -
Download preprint PDF
DOI: 10.1109/TVCG.2023.3261320
Room: Bayshore I
2024-10-17T16:36:00Z GMT-0600 Change your timezone on the schedule page
2024-10-17T16:36:00Z
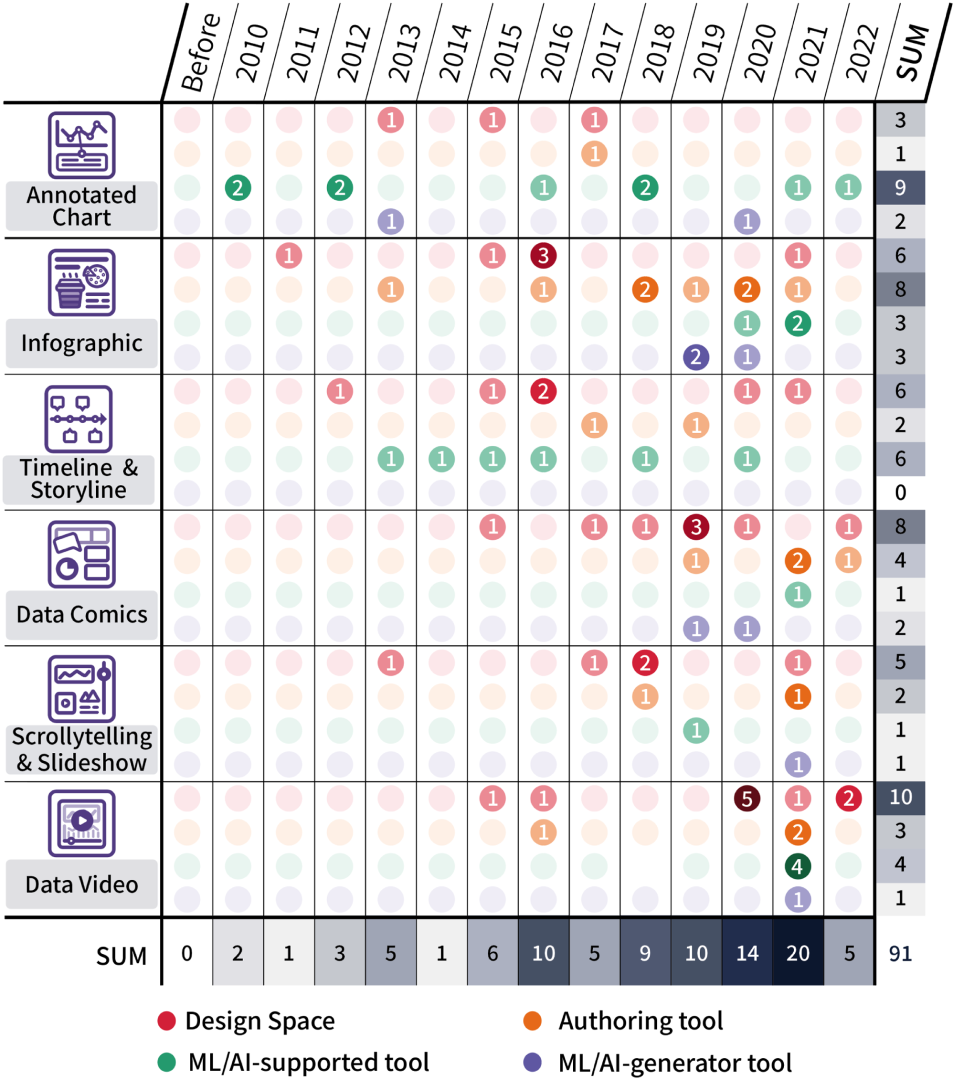
Fast forward
Keywords
Data Visualization, Automatic Visualization, Narrative Visualization, Design Space, Authoring Tools, Survey
Abstract
In recent years, narrative visualization has gained much attention. Researchers have proposed different design spaces for various narrative visualization genres and scenarios to facilitate the creation process. As users' needs grow and automation technologies advance, increasingly more tools have been designed and developed. In this study, we summarized six genres of narrative visualization (annotated charts, infographics, timelines & storylines, data comics, scrollytelling & slideshow, and data videos) based on previous research and four types of tools (design spaces, authoring tools, ML/AI-supported tools and ML/AI-generator tools) based on the intelligence and automation level of the tools. We surveyed 105 papers and tools to study how automation can progressively engage in visualization design and narrative processes to help users easily create narrative visualizations. This research aims to provide an overview of current research and development in the automation involvement of narrative visualization tools. We discuss key research problems in each category and suggest new opportunities to encourage further research in the related domain.
How Does Automation Shape the Process of Narrative Visualization: A Survey of Tools
Qing Chen -
Shixiong Cao -
Jiazhe Wang -
Nan Cao -
Download preprint PDF
DOI: 10.1109/TVCG.2023.3261320
Room: Bayshore I
2024-10-17T16:36:00Z GMT-0600 Change your timezone on the schedule page
2024-10-17T16:36:00Z
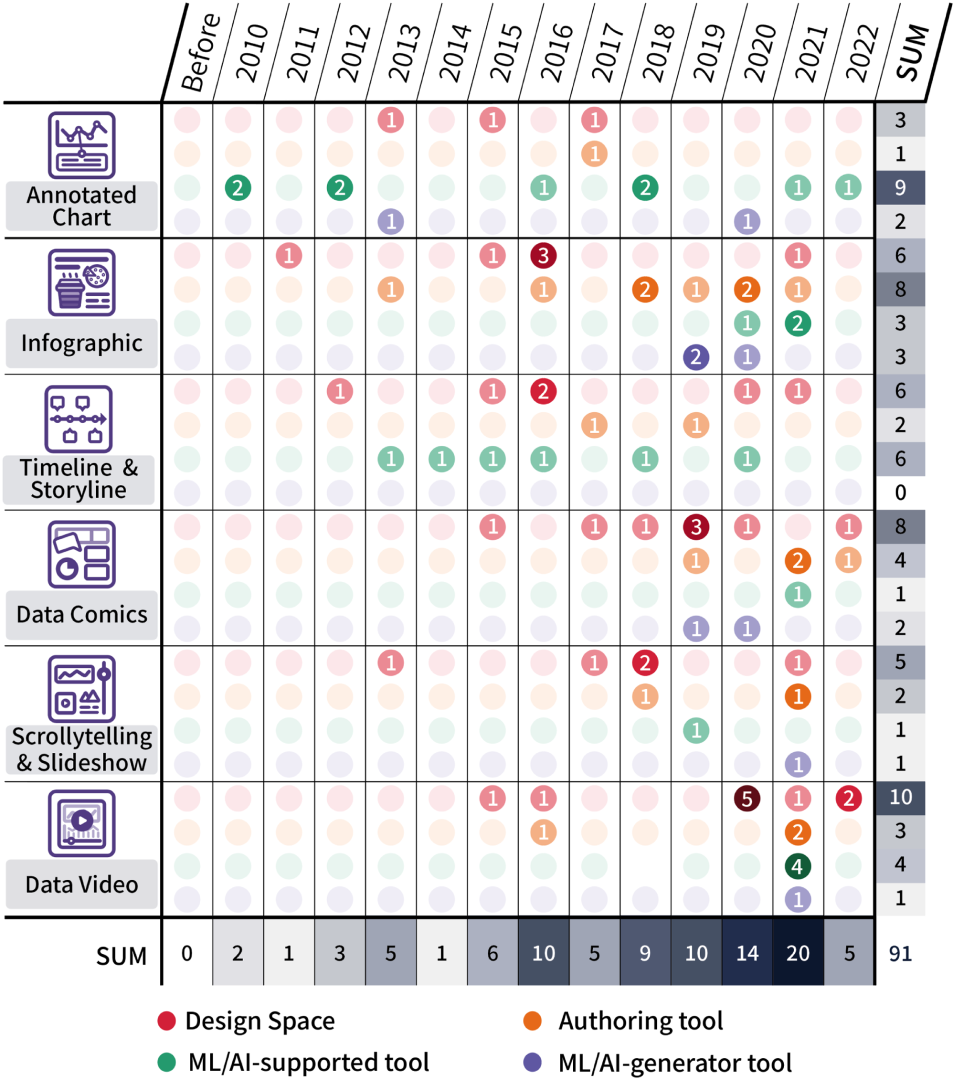
Fast forward
Keywords
Data Visualization, Automatic Visualization, Narrative Visualization, Design Space, Authoring Tools, Survey
Abstract
In recent years, narrative visualization has gained much attention. Researchers have proposed different design spaces for various narrative visualization genres and scenarios to facilitate the creation process. As users' needs grow and automation technologies advance, increasingly more tools have been designed and developed. In this study, we summarized six genres of narrative visualization (annotated charts, infographics, timelines & storylines, data comics, scrollytelling & slideshow, and data videos) based on previous research and four types of tools (design spaces, authoring tools, ML/AI-supported tools and ML/AI-generator tools) based on the intelligence and automation level of the tools. We surveyed 105 papers and tools to study how automation can progressively engage in visualization design and narrative processes to help users easily create narrative visualizations. This research aims to provide an overview of current research and development in the automation involvement of narrative visualization tools. We discuss key research problems in each category and suggest new opportunities to encourage further research in the related domain.
Effectiveness of Area-to-Value Legends and Grid Lines in Contiguous Area Cartograms
Kelvin L. T. Fung -
Simon T. Perrault -
Michael T. Gastner -
DOI: 10.1109/TVCG.2023.3275925
Room: Bayshore II
2024-10-16T18:33:00Z GMT-0600 Change your timezone on the schedule page
2024-10-16T18:33:00Z
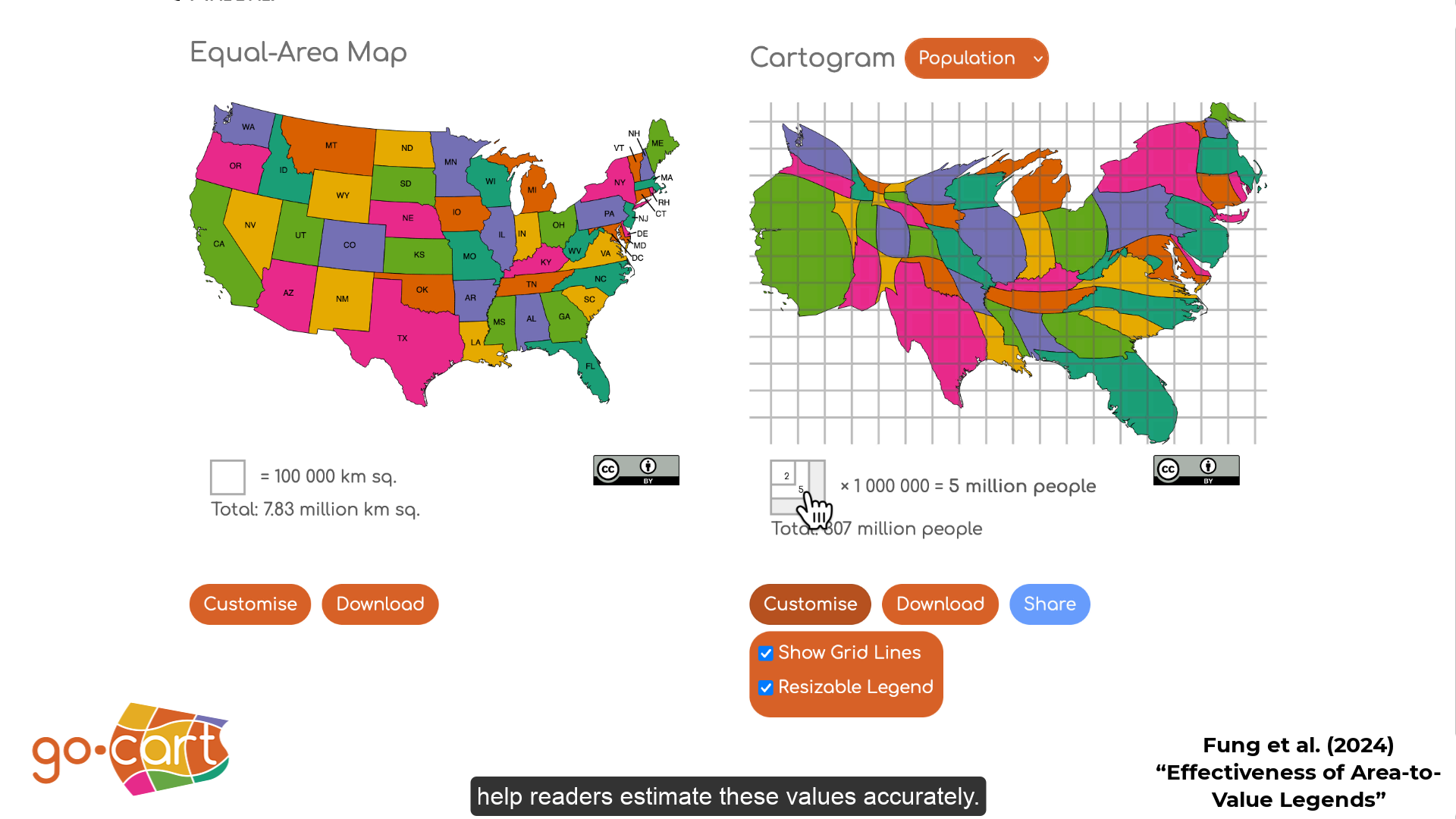
Fast forward
Keywords
Task Analysis, Symbols, Data Visualization, Sociology, Visualization, Switches, Mice, Cartogram, Geovisualization, Interactive Data Exploration, Quantitative Evaluation
Abstract
A contiguous area cartogram is a geographic map in which the area of each region is proportional to numerical data (e.g., population size) while keeping neighboring regions connected. In this study, we investigated whether value-to-area legends (square symbols next to the values represented by the squares' areas) and grid lines aid map readers in making better area judgments. We conducted an experiment to determine the accuracy, speed, and confidence with which readers infer numerical data values for the mapped regions. We found that, when only informed about the total numerical value represented by the whole cartogram without any legend, the distribution of estimates for individual regions was centered near the true value with substantial spread. Legends with grid lines significantly reduced the spread but led to a tendency to underestimate the values. Comparing differences between regions or between cartograms revealed that legends and grid lines slowed the estimation without improving accuracy. However, participants were more likely to complete the tasks when legends and grid lines were present, particularly when the area units represented by these features could be interactively selected. We recommend considering the cartogram's use case and purpose before deciding whether to include grid lines or an interactive legend.
Effectiveness of Area-to-Value Legends and Grid Lines in Contiguous Area Cartograms
Kelvin L. T. Fung -
Simon T. Perrault -
Michael T. Gastner -
DOI: 10.1109/TVCG.2023.3275925
Room: Bayshore II
2024-10-16T18:33:00Z GMT-0600 Change your timezone on the schedule page
2024-10-16T18:33:00Z
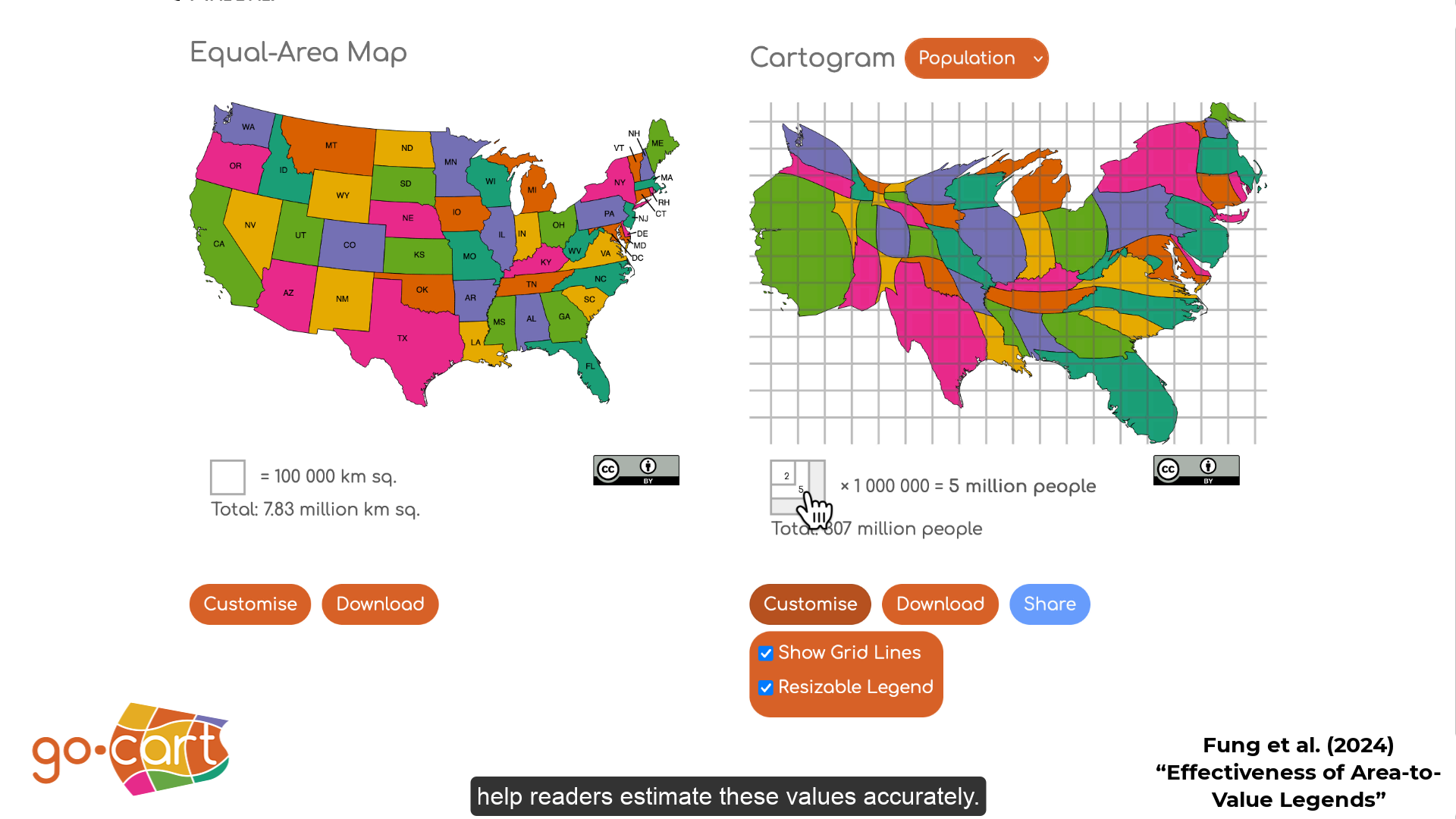
Fast forward
Keywords
Task Analysis, Symbols, Data Visualization, Sociology, Visualization, Switches, Mice, Cartogram, Geovisualization, Interactive Data Exploration, Quantitative Evaluation
Abstract
A contiguous area cartogram is a geographic map in which the area of each region is proportional to numerical data (e.g., population size) while keeping neighboring regions connected. In this study, we investigated whether value-to-area legends (square symbols next to the values represented by the squares' areas) and grid lines aid map readers in making better area judgments. We conducted an experiment to determine the accuracy, speed, and confidence with which readers infer numerical data values for the mapped regions. We found that, when only informed about the total numerical value represented by the whole cartogram without any legend, the distribution of estimates for individual regions was centered near the true value with substantial spread. Legends with grid lines significantly reduced the spread but led to a tendency to underestimate the values. Comparing differences between regions or between cartograms revealed that legends and grid lines slowed the estimation without improving accuracy. However, participants were more likely to complete the tasks when legends and grid lines were present, particularly when the area units represented by these features could be interactively selected. We recommend considering the cartogram's use case and purpose before deciding whether to include grid lines or an interactive legend.
More Than Data Stories: Broadening the Role of Visualization in Contemporary Journalism
Yu Fu -
John Stasko -
DOI: 10.1109/TVCG.2023.3287585
Room: Bayshore II
2024-10-17T17:45:00Z GMT-0600 Change your timezone on the schedule page
2024-10-17T17:45:00Z

Fast forward
Keywords
Computational journalism,data visualization,data-driven storytelling, journalism
Abstract
Data visualization and journalism are deeply connected. From early infographics to recent data-driven storytelling, visualization has become an integrated part of contemporary journalism, primarily as a communication artifact to inform the general public. Data journalism, harnessing the power of data visualization, has emerged as a bridge between the growing volume of data and our society. Visualization research that centers around data storytelling has sought to understand and facilitate such journalistic endeavors. However, a recent metamorphosis in journalism has brought broader challenges and opportunities that extend beyond mere communication of data. We present this article to enhance our understanding of such transformations and thus broaden visualization research's scope and practical contribution to this evolving field. We first survey recent significant shifts, emerging challenges, and computational practices in journalism. We then summarize six roles of computing in journalism and their implications. Based on these implications, we provide propositions for visualization research concerning each role. Ultimately, by mapping the roles and propositions onto a proposed ecological model and contextualizing existing visualization research, we surface seven general topics and a series of research agendas that can guide future visualization research at this intersection.
More Than Data Stories: Broadening the Role of Visualization in Contemporary Journalism
Yu Fu -
John Stasko -
DOI: 10.1109/TVCG.2023.3287585
Room: Bayshore II
2024-10-17T17:45:00Z GMT-0600 Change your timezone on the schedule page
2024-10-17T17:45:00Z

Fast forward
Keywords
Computational journalism,data visualization,data-driven storytelling, journalism
Abstract
Data visualization and journalism are deeply connected. From early infographics to recent data-driven storytelling, visualization has become an integrated part of contemporary journalism, primarily as a communication artifact to inform the general public. Data journalism, harnessing the power of data visualization, has emerged as a bridge between the growing volume of data and our society. Visualization research that centers around data storytelling has sought to understand and facilitate such journalistic endeavors. However, a recent metamorphosis in journalism has brought broader challenges and opportunities that extend beyond mere communication of data. We present this article to enhance our understanding of such transformations and thus broaden visualization research's scope and practical contribution to this evolving field. We first survey recent significant shifts, emerging challenges, and computational practices in journalism. We then summarize six roles of computing in journalism and their implications. Based on these implications, we provide propositions for visualization research concerning each role. Ultimately, by mapping the roles and propositions onto a proposed ecological model and contextualizing existing visualization research, we surface seven general topics and a series of research agendas that can guide future visualization research at this intersection.
What Does the Chart Say? Grouping Cues Guide Viewer Comparisons and Conclusions in Bar Charts
Cindy Xiong Bearfield -
Chase Stokes -
Andrew Lovett -
Steven Franconeri -
DOI: 10.1109/TVCG.2023.3289292
Room: Bayshore II
2024-10-16T18:45:00Z GMT-0600 Change your timezone on the schedule page
2024-10-16T18:45:00Z

Fast forward
Keywords
comparison, perception, visual grouping, bar charts, verbal conclusions.
Abstract
Reading a visualization is like reading a paragraph. Each sentence is a comparison: the mean of these is higher than those; this difference is smaller than that. What determines which comparisons are made first? The viewer's goals and expertise matter, but the way that values are visually grouped together within the chart also impacts those comparisons. Research from psychology suggests that comparisons involve multiple steps. First, the viewer divides the visualization into a set of units. This might include a single bar or a grouped set of bars. Then the viewer selects and compares two of these units, perhaps noting that one pair of bars is longer than another. Viewers might take an additional third step and perform a second-order comparison, perhaps determining that the difference between one pair of bars is greater than the difference between another pair. We create a visual comparison taxonomy that allows us to develop and test a sequence of hypotheses about which comparisons people are more likely to make when reading a visualization. We find that people tend to compare two groups before comparing two individual bars and that second-order comparisons are rare. Visual cues like spatial proximity and color can influence which elements are grouped together and selected for comparison, with spatial proximity being a stronger grouping cue. Interestingly, once the viewer grouped together and compared a set of bars, regardless of whether the group is formed by spatial proximity or color similarity, they no longer consider other possible groupings in their comparisons.
What Does the Chart Say? Grouping Cues Guide Viewer Comparisons and Conclusions in Bar Charts
Cindy Xiong Bearfield -
Chase Stokes -
Andrew Lovett -
Steven Franconeri -
DOI: 10.1109/TVCG.2023.3289292
Room: Bayshore II
2024-10-16T18:45:00Z GMT-0600 Change your timezone on the schedule page
2024-10-16T18:45:00Z

Fast forward
Keywords
comparison, perception, visual grouping, bar charts, verbal conclusions.
Abstract
Reading a visualization is like reading a paragraph. Each sentence is a comparison: the mean of these is higher than those; this difference is smaller than that. What determines which comparisons are made first? The viewer's goals and expertise matter, but the way that values are visually grouped together within the chart also impacts those comparisons. Research from psychology suggests that comparisons involve multiple steps. First, the viewer divides the visualization into a set of units. This might include a single bar or a grouped set of bars. Then the viewer selects and compares two of these units, perhaps noting that one pair of bars is longer than another. Viewers might take an additional third step and perform a second-order comparison, perhaps determining that the difference between one pair of bars is greater than the difference between another pair. We create a visual comparison taxonomy that allows us to develop and test a sequence of hypotheses about which comparisons people are more likely to make when reading a visualization. We find that people tend to compare two groups before comparing two individual bars and that second-order comparisons are rare. Visual cues like spatial proximity and color can influence which elements are grouped together and selected for comparison, with spatial proximity being a stronger grouping cue. Interestingly, once the viewer grouped together and compared a set of bars, regardless of whether the group is formed by spatial proximity or color similarity, they no longer consider other possible groupings in their comparisons.
This is the Table I Want! Interactive Data Transformation on Desktop and in Virtual Reality
Sungwon In -
Tica Lin -
Chris North -
Hanspeter Pfister -
Yalong Yang -
Screen-reader Accessible PDF
Download preprint PDF
DOI: 10.1109/TVCG.2023.3299602
Room: Bayshore II
2024-10-16T12:42:00Z GMT-0600 Change your timezone on the schedule page
2024-10-16T12:42:00Z

Fast forward
Keywords
Immersive Analytics, Data Transformation, Data Science, Interaction, Empirical Study, Virtual/Augmented/Mixed Reality
Abstract
Data transformation is an essential step in data science. While experts primarily use programming to transform their data, there is an increasing need to support non-programmers with user interface-based tools. With the rapid development in interaction techniques and computing environments, we report our empirical findings about the effects of interaction techniques and environments on performing data transformation tasks. Specifically, we studied the potential benefits of direct interaction and virtual reality (VR) for data transformation. We compared gesture interaction versus a standard WIMP user interface, each on the desktop and in VR. With the tested data and tasks, we found time performance was similar between desktop and VR. Meanwhile, VR demonstrates preliminary evidence to better support provenance and sense-making throughout the data transformation process. Our exploration of performing data transformation in VR also provides initial affirmation for enabling an iterative and fully immersive data science workflow.
This is the Table I Want! Interactive Data Transformation on Desktop and in Virtual Reality
Sungwon In -
Tica Lin -
Chris North -
Hanspeter Pfister -
Yalong Yang -
Screen-reader Accessible PDF
Download preprint PDF
DOI: 10.1109/TVCG.2023.3299602
Room: Bayshore II
2024-10-16T12:42:00Z GMT-0600 Change your timezone on the schedule page
2024-10-16T12:42:00Z

Fast forward
Keywords
Immersive Analytics, Data Transformation, Data Science, Interaction, Empirical Study, Virtual/Augmented/Mixed Reality
Abstract
Data transformation is an essential step in data science. While experts primarily use programming to transform their data, there is an increasing need to support non-programmers with user interface-based tools. With the rapid development in interaction techniques and computing environments, we report our empirical findings about the effects of interaction techniques and environments on performing data transformation tasks. Specifically, we studied the potential benefits of direct interaction and virtual reality (VR) for data transformation. We compared gesture interaction versus a standard WIMP user interface, each on the desktop and in VR. With the tested data and tasks, we found time performance was similar between desktop and VR. Meanwhile, VR demonstrates preliminary evidence to better support provenance and sense-making throughout the data transformation process. Our exploration of performing data transformation in VR also provides initial affirmation for enabling an iterative and fully immersive data science workflow.
Visualizing and Comparing Machine Learning Predictions to Improve Human-AI Teaming on the Example of Cell Lineage
Jiayi Hong -
Ross Maciejewski -
Alain Trubuil -
Tobias Isenberg -
Screen-reader Accessible PDF
Download preprint PDF
DOI: 10.1109/TVCG.2023.3302308
Room: Bayshore V
2024-10-17T13:06:00Z GMT-0600 Change your timezone on the schedule page
2024-10-17T13:06:00Z
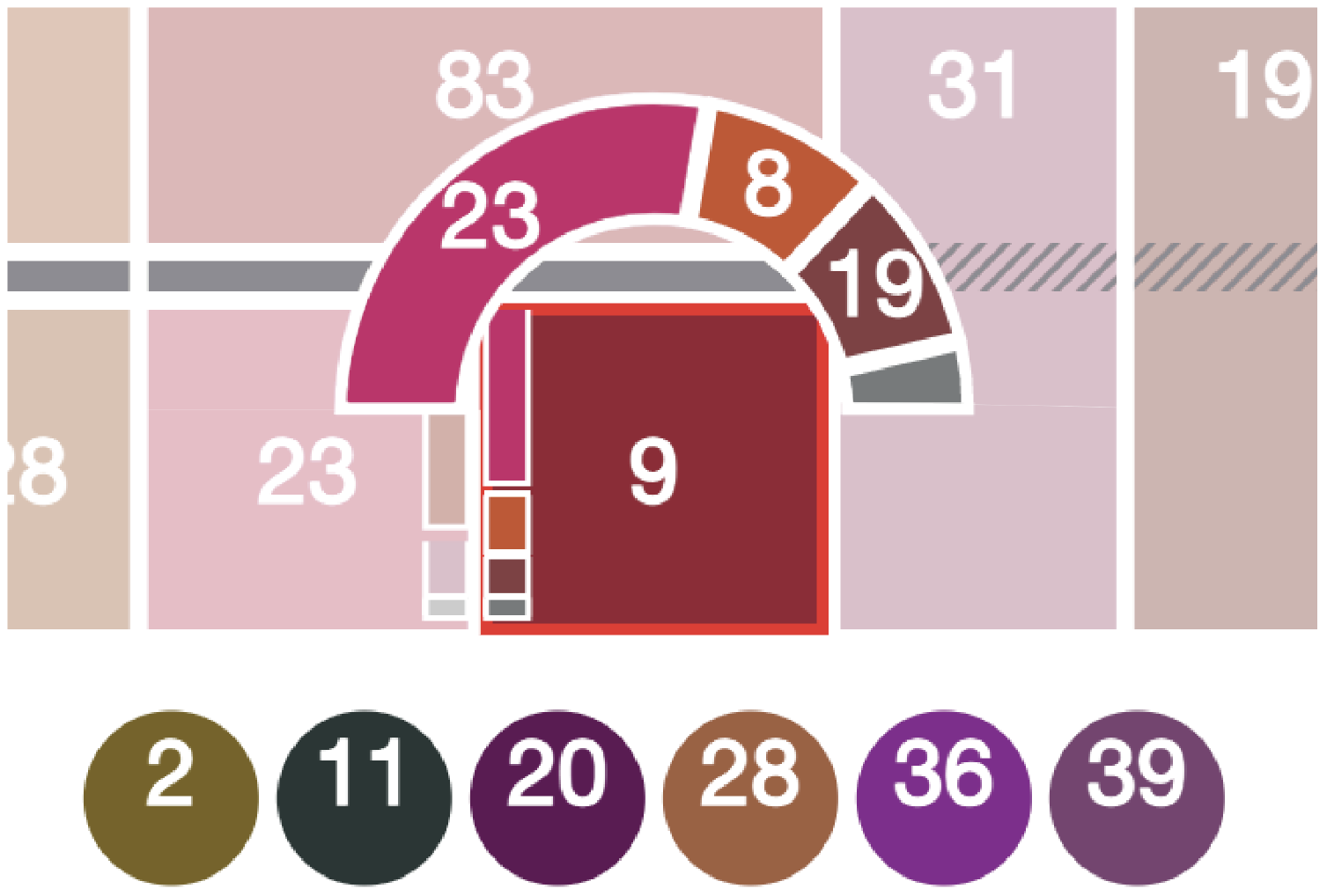
Fast forward
Keywords
Visualization, visual analytics, machine learning, comparing ML predictions, human-AI teaming, plant biology, cell lineage
Abstract
We visualize the predictions of multiple machine learning models to help biologists as they interactively make decisions about cell lineage---the development of a (plant) embryo from a single ovum cell. Based on a confocal microscopy dataset, traditionally biologists manually constructed the cell lineage, starting from this observation and reasoning backward in time to establish their inheritance. To speed up this tedious process, we make use of machine learning (ML) models trained on a database of manually established cell lineages to assist the biologist in cell assignment. Most biologists, however, are not familiar with ML, nor is it clear to them which model best predicts the embryo's development. We thus have developed a visualization system that is designed to support biologists in exploring and comparing ML models, checking the model predictions, detecting possible ML model mistakes, and deciding on the most likely embryo development. To evaluate our proposed system, we deployed our interface with six biologists in an observational study. Our results show that the visual representations of machine learning are easily understandable, and our tool, LineageD+, could potentially increase biologists' working efficiency and enhance the understanding of embryos.
Visualizing and Comparing Machine Learning Predictions to Improve Human-AI Teaming on the Example of Cell Lineage
Jiayi Hong -
Ross Maciejewski -
Alain Trubuil -
Tobias Isenberg -
Screen-reader Accessible PDF
Download preprint PDF
DOI: 10.1109/TVCG.2023.3302308
Room: Bayshore V
2024-10-17T13:06:00Z GMT-0600 Change your timezone on the schedule page
2024-10-17T13:06:00Z
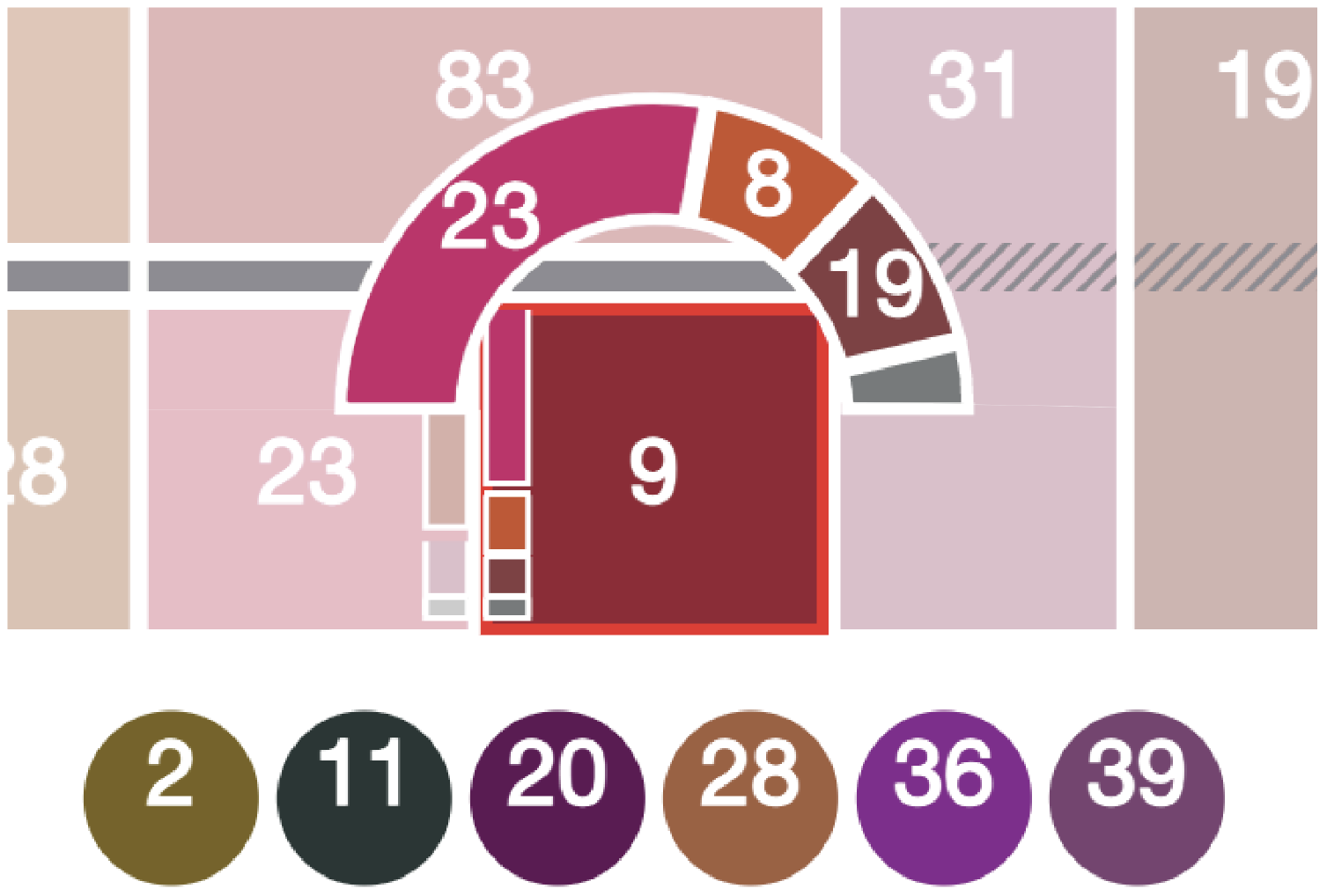
Fast forward
Keywords
Visualization, visual analytics, machine learning, comparing ML predictions, human-AI teaming, plant biology, cell lineage
Abstract
We visualize the predictions of multiple machine learning models to help biologists as they interactively make decisions about cell lineage---the development of a (plant) embryo from a single ovum cell. Based on a confocal microscopy dataset, traditionally biologists manually constructed the cell lineage, starting from this observation and reasoning backward in time to establish their inheritance. To speed up this tedious process, we make use of machine learning (ML) models trained on a database of manually established cell lineages to assist the biologist in cell assignment. Most biologists, however, are not familiar with ML, nor is it clear to them which model best predicts the embryo's development. We thus have developed a visualization system that is designed to support biologists in exploring and comparing ML models, checking the model predictions, detecting possible ML model mistakes, and deciding on the most likely embryo development. To evaluate our proposed system, we deployed our interface with six biologists in an observational study. Our results show that the visual representations of machine learning are easily understandable, and our tool, LineageD+, could potentially increase biologists' working efficiency and enhance the understanding of embryos.
SmartGD: A GAN-Based Graph Drawing Framework for Diverse Aesthetic Goals
Xiaoqi Wang -
Kevin Yen -
Yifan Hu -
Han-Wei Shen -
DOI: 10.1109/TVCG.2023.3306356
Room: Bayshore VII
2024-10-18T13:06:00Z GMT-0600 Change your timezone on the schedule page
2024-10-18T13:06:00Z

Fast forward
Abstract
A multitude of studies have been conducted on graph drawing, but many existing methods only focus on optimizing a single aesthetic aspect of graph layouts. There are a few existing methods that attempt to develop a flexible solution for optimizing different aesthetic aspects measured by different aesthetic criteria. Furthermore, thanks to the significant advance in deep learning techniques, several deep learning-based layout methods were proposed recently, which have demonstrated the advantages of the deep learning approaches for graph drawing. However, none of these existing methods can be directly applied to optimizing non-differentiable criteria without special accommodation. In this work, we propose a novel Generative Adversarial Network (GAN) based deep learning framework for graph drawing, called SmartGD, which can optimize any quantitative aesthetic goals even though they are non-differentiable. In the cases where the aesthetic goal is too abstract to be described mathematically, SmartGD can draw graphs in a similar style as a collection of good layout examples, which might be selected by humans based on the abstract aesthetic goal. To demonstrate the effectiveness and efficiency of SmartGD, we conduct experiments on minimizing stress, minimizing edge crossing, maximizing crossing angle, and a combination of multiple aesthetics. Compared with several popular graph drawing algorithms, the experimental results show that SmartGD achieves good performance both quantitatively and qualitatively.
SmartGD: A GAN-Based Graph Drawing Framework for Diverse Aesthetic Goals
Xiaoqi Wang -
Kevin Yen -
Yifan Hu -
Han-Wei Shen -
DOI: 10.1109/TVCG.2023.3306356
Room: Bayshore VII
2024-10-18T13:06:00Z GMT-0600 Change your timezone on the schedule page
2024-10-18T13:06:00Z

Fast forward
Abstract
A multitude of studies have been conducted on graph drawing, but many existing methods only focus on optimizing a single aesthetic aspect of graph layouts. There are a few existing methods that attempt to develop a flexible solution for optimizing different aesthetic aspects measured by different aesthetic criteria. Furthermore, thanks to the significant advance in deep learning techniques, several deep learning-based layout methods were proposed recently, which have demonstrated the advantages of the deep learning approaches for graph drawing. However, none of these existing methods can be directly applied to optimizing non-differentiable criteria without special accommodation. In this work, we propose a novel Generative Adversarial Network (GAN) based deep learning framework for graph drawing, called SmartGD, which can optimize any quantitative aesthetic goals even though they are non-differentiable. In the cases where the aesthetic goal is too abstract to be described mathematically, SmartGD can draw graphs in a similar style as a collection of good layout examples, which might be selected by humans based on the abstract aesthetic goal. To demonstrate the effectiveness and efficiency of SmartGD, we conduct experiments on minimizing stress, minimizing edge crossing, maximizing crossing angle, and a combination of multiple aesthetics. Compared with several popular graph drawing algorithms, the experimental results show that SmartGD achieves good performance both quantitatively and qualitatively.
On Network Structural and Temporal Encodings: A Space and Time Odyssey
Velitchko Filipov -
Alessio Arleo -
Markus Bögl -
Silvia Miksch -
Screen-reader Accessible PDF
DOI: 10.1109/TVCG.2023.3310019
Room: Bayshore I
2024-10-16T18:21:00Z GMT-0600 Change your timezone on the schedule page
2024-10-16T18:21:00Z
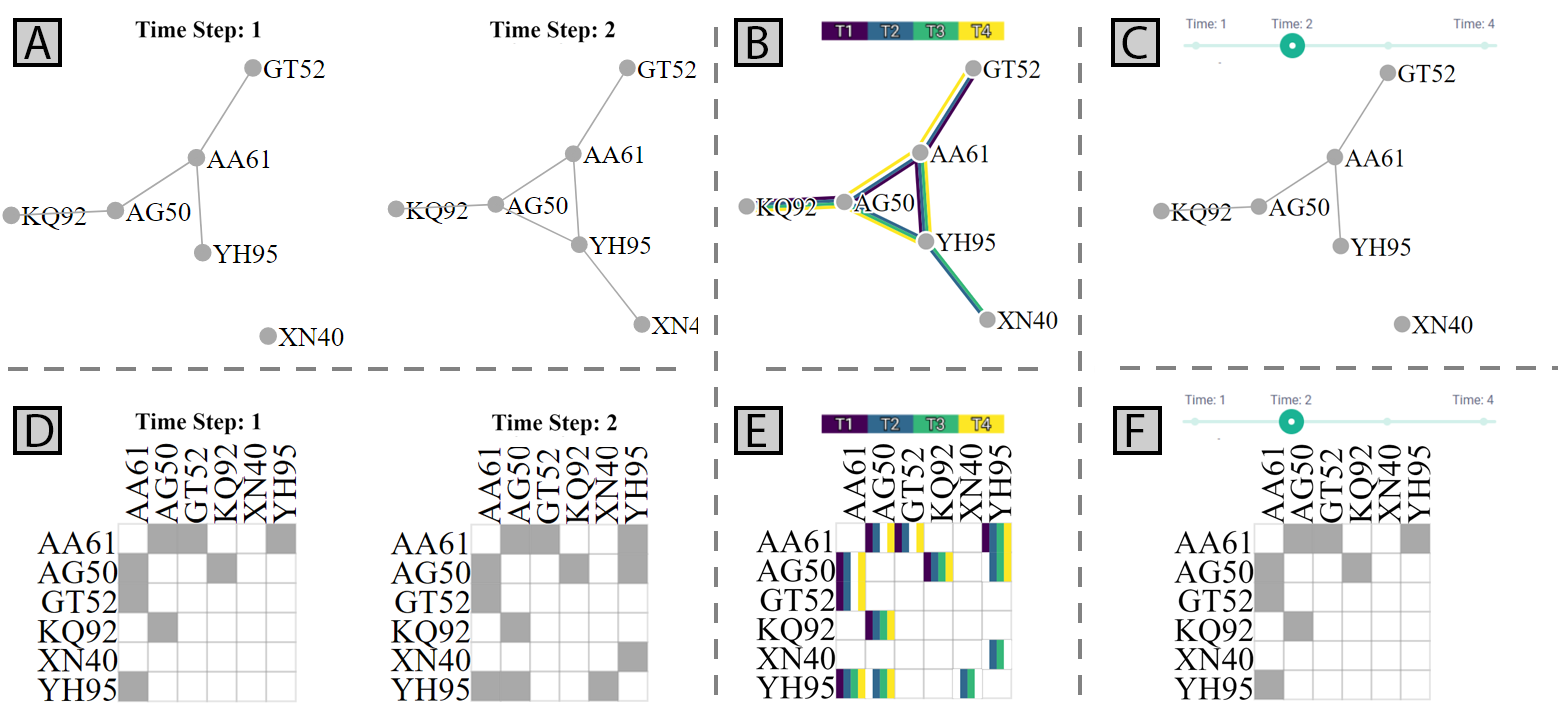
Fast forward
Abstract
The dynamic network visualization design space consists of two major dimensions: network structural and temporal representation. As more techniques are developed and published, a clear need for evaluation and experimental comparisons between them emerges. Most studies explore the temporal dimension and diverse interaction techniques supporting the participants, focusing on a single structural representation. Empirical evidence about performance and preference for different visualization approaches is scattered over different studies, experimental settings, and tasks. This paper aims to comprehensively investigate the dynamic network visualization design space in two evaluations. First, a controlled study assessing participants' response times, accuracy, and preferences for different combinations of network structural and temporal representations on typical dynamic network exploration tasks, with and without the support of standard interaction methods. Second, the best-performing combinations from the first study are enhanced based on participants' feedback and evaluated in a heuristic-based qualitative study with visualization experts on a real-world network. Our results highlight node-link with animation and playback controls as the best-performing combination and the most preferred based on ratings. Matrices achieve similar performance to node-link in the first study but have considerably lower scores in our second evaluation. Similarly, juxtaposition exhibits evident scalability issues in more realistic analysis contexts.
On Network Structural and Temporal Encodings: A Space and Time Odyssey
Velitchko Filipov -
Alessio Arleo -
Markus Bögl -
Silvia Miksch -
Screen-reader Accessible PDF
DOI: 10.1109/TVCG.2023.3310019
Room: Bayshore I
2024-10-16T18:21:00Z GMT-0600 Change your timezone on the schedule page
2024-10-16T18:21:00Z
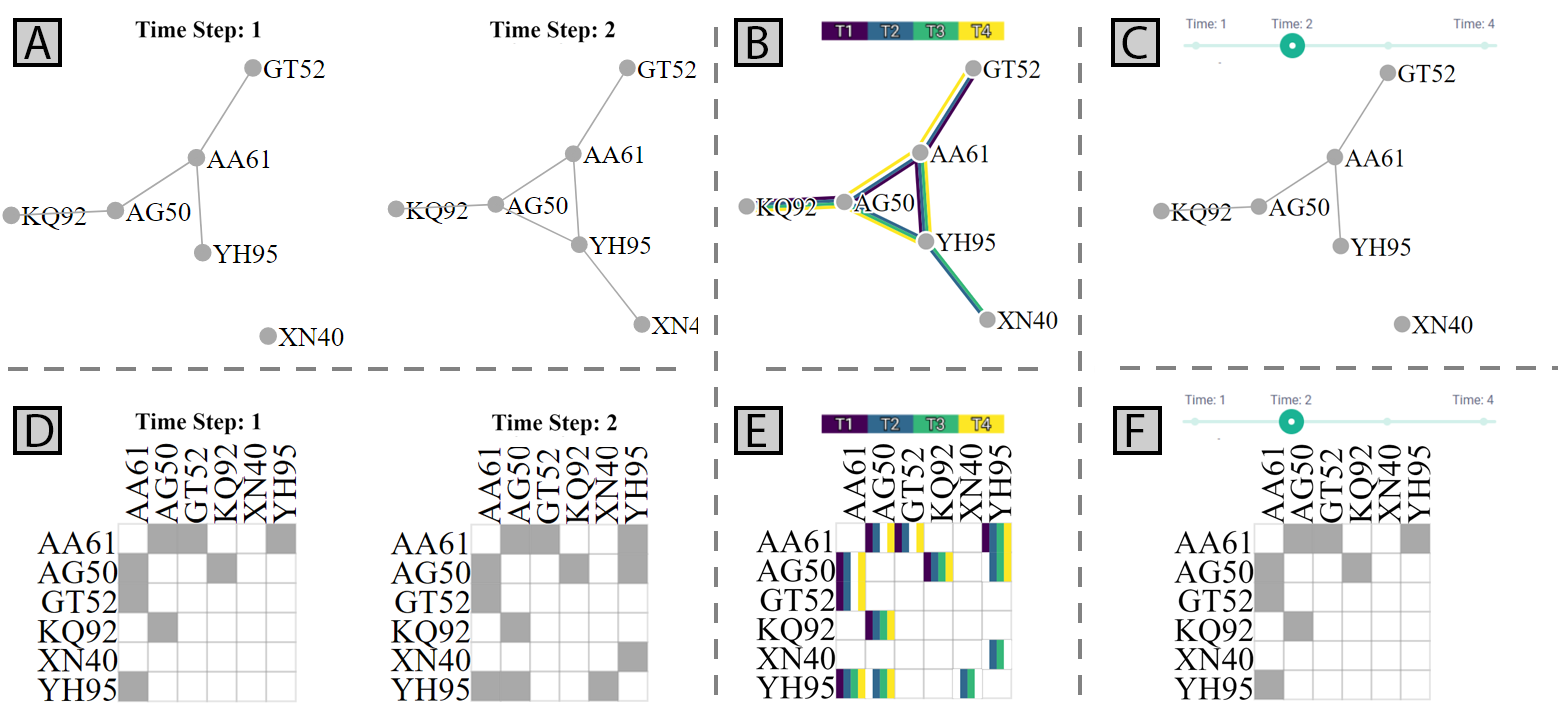
Fast forward
Abstract
The dynamic network visualization design space consists of two major dimensions: network structural and temporal representation. As more techniques are developed and published, a clear need for evaluation and experimental comparisons between them emerges. Most studies explore the temporal dimension and diverse interaction techniques supporting the participants, focusing on a single structural representation. Empirical evidence about performance and preference for different visualization approaches is scattered over different studies, experimental settings, and tasks. This paper aims to comprehensively investigate the dynamic network visualization design space in two evaluations. First, a controlled study assessing participants' response times, accuracy, and preferences for different combinations of network structural and temporal representations on typical dynamic network exploration tasks, with and without the support of standard interaction methods. Second, the best-performing combinations from the first study are enhanced based on participants' feedback and evaluated in a heuristic-based qualitative study with visualization experts on a real-world network. Our results highlight node-link with animation and playback controls as the best-performing combination and the most preferred based on ratings. Matrices achieve similar performance to node-link in the first study but have considerably lower scores in our second evaluation. Similarly, juxtaposition exhibits evident scalability issues in more realistic analysis contexts.
AdaVis: Adaptive and Explainable Visualization Recommendation for Tabular Data'
Songheng Zhang -
Yong Wang -
Haotian Li -
Huamin Qu -
DOI: 10.1109/TVCG.2023.3316469
Room: Bayshore II
2024-10-17T12:30:00Z GMT-0600 Change your timezone on the schedule page
2024-10-17T12:30:00Z

Fast forward
Keywords
Visualization Recommendation, Logical Reasoning, Data Visualization, Knowledge Graph
Abstract
Automated visualization recommendation facilitates the rapid creation of effective visualizations, which is especially beneficial for users with limited time and limited knowledge of data visualization. There is an increasing trend in leveraging machine learning (ML) techniques to achieve an end-to-end visualization recommendation. However, existing ML-based approaches implicitly assume that there is only one appropriate visualization for a specific dataset, which is often not true for real applications. Also, they often work like a black box, and are difficult for users to understand the reasons for recommending specific visualizations. To fill the research gap, we propose AdaVis, an adaptive and explainable approach to recommend one or multiple appropriate visualizations for a tabular dataset. It leverages a box embedding-based knowledge graph to well model the possible one-to-many mapping relations among different entities (i.e., data features, dataset columns, datasets, and visualization choices). The embeddings of the entities and relations can be learned from dataset-visualization pairs. Also, AdaVis incorporates the attention mechanism into the inference framework. Attention can indicate the relative importance of data features for a dataset and provide fine-grained explainability. Our extensive evaluations through quantitative metric evaluations, case studies, and user interviews demonstrate the effectiveness of AdaVis.
AdaVis: Adaptive and Explainable Visualization Recommendation for Tabular Data'
Songheng Zhang -
Yong Wang -
Haotian Li -
Huamin Qu -
DOI: 10.1109/TVCG.2023.3316469
Room: Bayshore II
2024-10-17T12:30:00Z GMT-0600 Change your timezone on the schedule page
2024-10-17T12:30:00Z

Fast forward
Keywords
Visualization Recommendation, Logical Reasoning, Data Visualization, Knowledge Graph
Abstract
Automated visualization recommendation facilitates the rapid creation of effective visualizations, which is especially beneficial for users with limited time and limited knowledge of data visualization. There is an increasing trend in leveraging machine learning (ML) techniques to achieve an end-to-end visualization recommendation. However, existing ML-based approaches implicitly assume that there is only one appropriate visualization for a specific dataset, which is often not true for real applications. Also, they often work like a black box, and are difficult for users to understand the reasons for recommending specific visualizations. To fill the research gap, we propose AdaVis, an adaptive and explainable approach to recommend one or multiple appropriate visualizations for a tabular dataset. It leverages a box embedding-based knowledge graph to well model the possible one-to-many mapping relations among different entities (i.e., data features, dataset columns, datasets, and visualization choices). The embeddings of the entities and relations can be learned from dataset-visualization pairs. Also, AdaVis incorporates the attention mechanism into the inference framework. Attention can indicate the relative importance of data features for a dataset and provide fine-grained explainability. Our extensive evaluations through quantitative metric evaluations, case studies, and user interviews demonstrate the effectiveness of AdaVis.
GeoLinter: A Linting Framework for Choropleth Maps
Fan Lei -
Arlen Fan -
Alan M. MacEachren -
Ross Maciejewski -
Screen-reader Accessible PDF
Download preprint PDF
DOI: 10.1109/TVCG.2023.3322372
Room: Bayshore II
2024-10-16T17:45:00Z GMT-0600 Change your timezone on the schedule page
2024-10-16T17:45:00Z
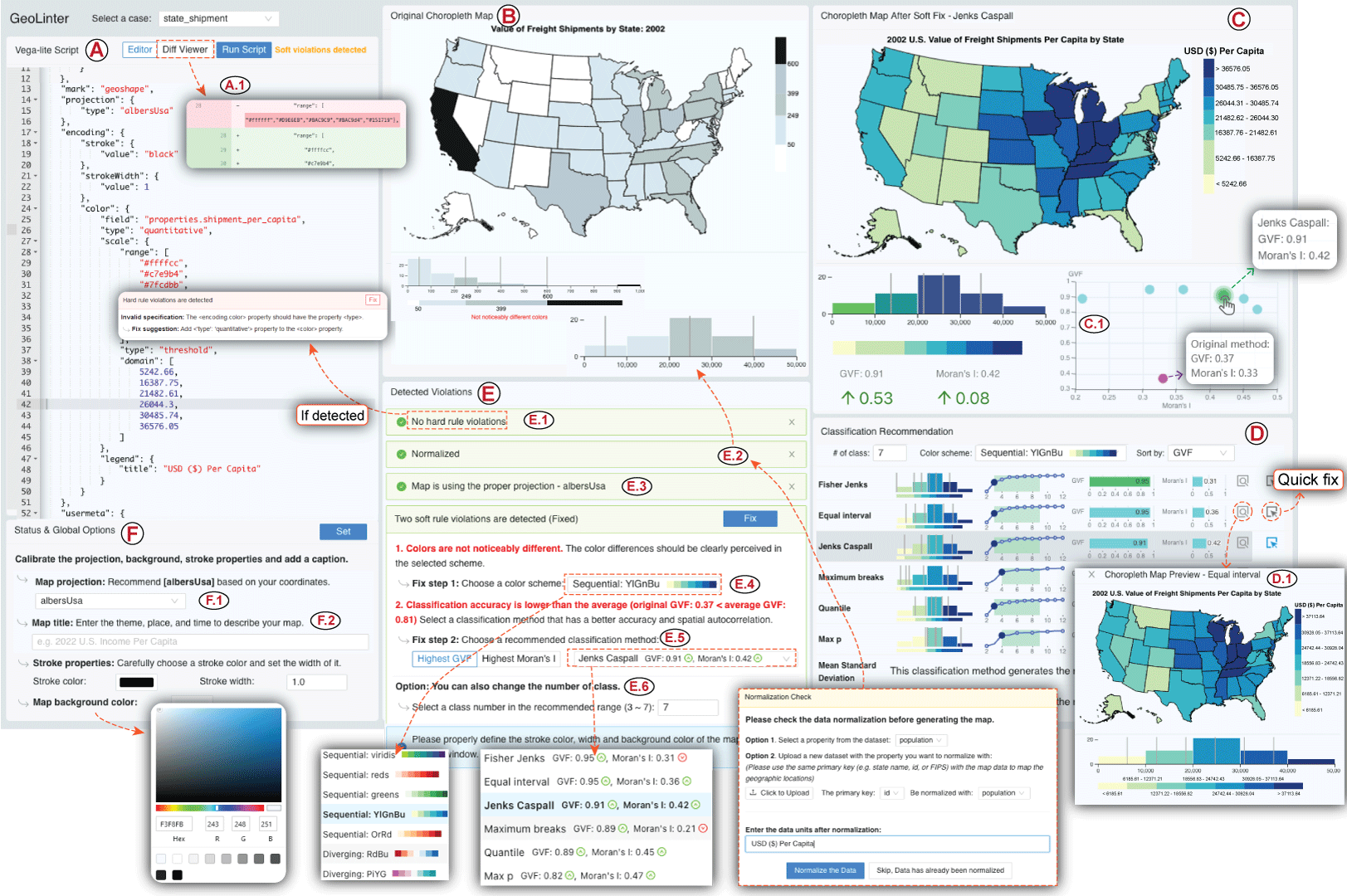
Fast forward
Keywords
Data visualization , Image color analysis , Geology , Recommender systems , Guidelines , Bars , Visualization Author Keywords: Automated visualization design , choropleth maps , visualization linting , visualization recommendation
Abstract
Visualization linting is a proven effective tool in assisting users to follow established visualization guidelines. Despite its success, visualization linting for choropleth maps, one of the most popular visualizations on the internet, has yet to be investigated. In this paper, we present GeoLinter, a linting framework for choropleth maps that assists in creating accurate and robust maps. Based on a set of design guidelines and metrics drawing upon a collection of best practices from the cartographic literature, GeoLinter detects potentially suboptimal design decisions and provides further recommendations on design improvement with explanations at each step of the design process. We perform a validation study to evaluate the proposed framework's functionality with respect to identifying and fixing errors and apply its results to improve the robustness of GeoLinter. Finally, we demonstrate the effectiveness of the GeoLinter - validated through empirical studies - by applying it to a series of case studies using real-world datasets.
GeoLinter: A Linting Framework for Choropleth Maps
Fan Lei -
Arlen Fan -
Alan M. MacEachren -
Ross Maciejewski -
Screen-reader Accessible PDF
Download preprint PDF
DOI: 10.1109/TVCG.2023.3322372
Room: Bayshore II
2024-10-16T17:45:00Z GMT-0600 Change your timezone on the schedule page
2024-10-16T17:45:00Z
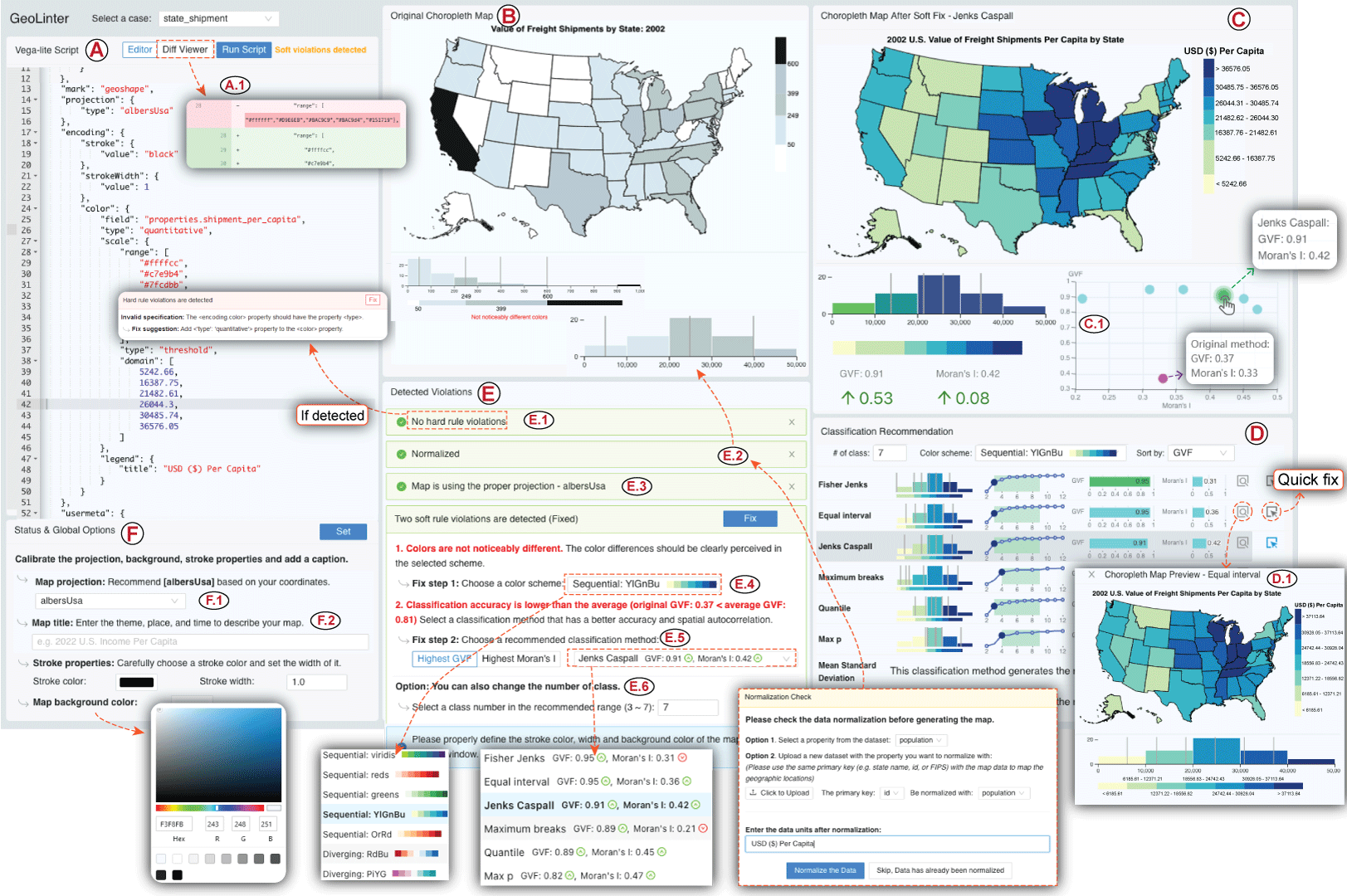
Fast forward
Keywords
Data visualization , Image color analysis , Geology , Recommender systems , Guidelines , Bars , Visualization Author Keywords: Automated visualization design , choropleth maps , visualization linting , visualization recommendation
Abstract
Visualization linting is a proven effective tool in assisting users to follow established visualization guidelines. Despite its success, visualization linting for choropleth maps, one of the most popular visualizations on the internet, has yet to be investigated. In this paper, we present GeoLinter, a linting framework for choropleth maps that assists in creating accurate and robust maps. Based on a set of design guidelines and metrics drawing upon a collection of best practices from the cartographic literature, GeoLinter detects potentially suboptimal design decisions and provides further recommendations on design improvement with explanations at each step of the design process. We perform a validation study to evaluate the proposed framework's functionality with respect to identifying and fixing errors and apply its results to improve the robustness of GeoLinter. Finally, we demonstrate the effectiveness of the GeoLinter - validated through empirical studies - by applying it to a series of case studies using real-world datasets.
Eliciting Multimodal and Collaborative Interactions for Data Exploration on Large Vertical Displays
Gabriela Molina León -
Petra Isenberg -
Andreas Breiter -
Download preprint PDF
DOI: 10.1109/TVCG.2023.3323150
Room: Bayshore V
2024-10-16T16:48:00Z GMT-0600 Change your timezone on the schedule page
2024-10-16T16:48:00Z
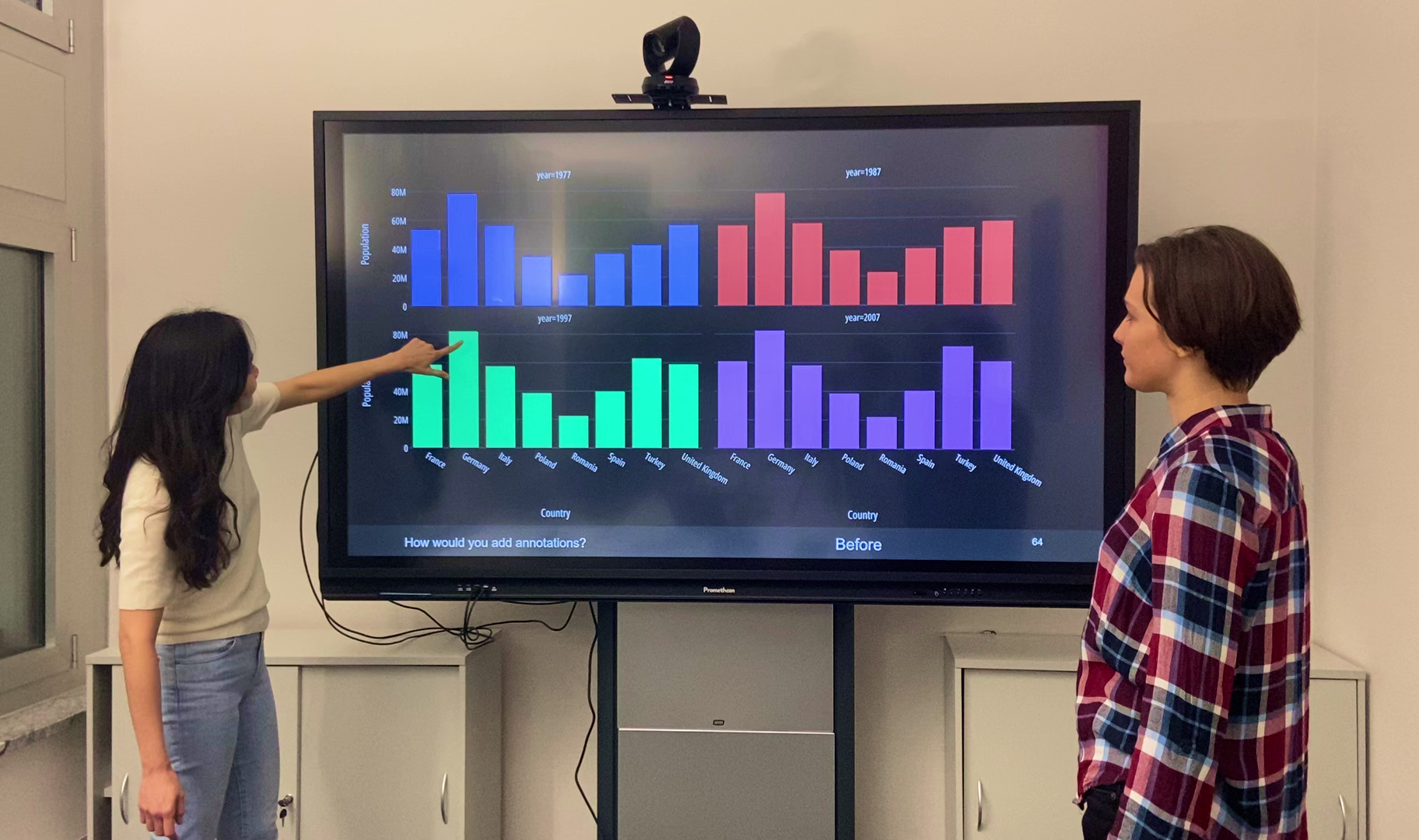
Fast forward
Keywords
Multimodal interaction, collaborative work, large vertical displays, elicitation study, spatio-temporal data
Abstract
We examined user preferences to combine multiple interaction modalities for collaborative interaction with data shown on large vertical displays. Large vertical displays facilitate visual data exploration and allow the use of diverse interaction modalities by multiple users at different distances from the screen. Yet, how to offer multiple interaction modalities is a non-trivial problem. We conducted an elicitation study with 20 participants that generated 1015 interaction proposals combining touch, speech, pen, and mid-air gestures. Given the opportunity to interact using these four 13 modalities, participants preferred speech interaction in 10 of 15 14 low-level tasks and direct manipulation for straightforward tasks 15 such as showing a tooltip or selecting. In contrast to previous work, 16 participants most favored unimodal and personal interactions. We 17 identified what we call collaborative synonyms among their interaction proposals and found that pairs of users collaborated either unimodally and simultaneously or multimodally and sequentially. We provide insights into how end-users associate visual exploration tasks with certain modalities and how they collaborate at different interaction distances using specific interaction modalities. The supplemental material is available at https://osf.io/m8zuh.
Eliciting Multimodal and Collaborative Interactions for Data Exploration on Large Vertical Displays
Gabriela Molina León -
Petra Isenberg -
Andreas Breiter -
Download preprint PDF
DOI: 10.1109/TVCG.2023.3323150
Room: Bayshore V
2024-10-16T16:48:00Z GMT-0600 Change your timezone on the schedule page
2024-10-16T16:48:00Z
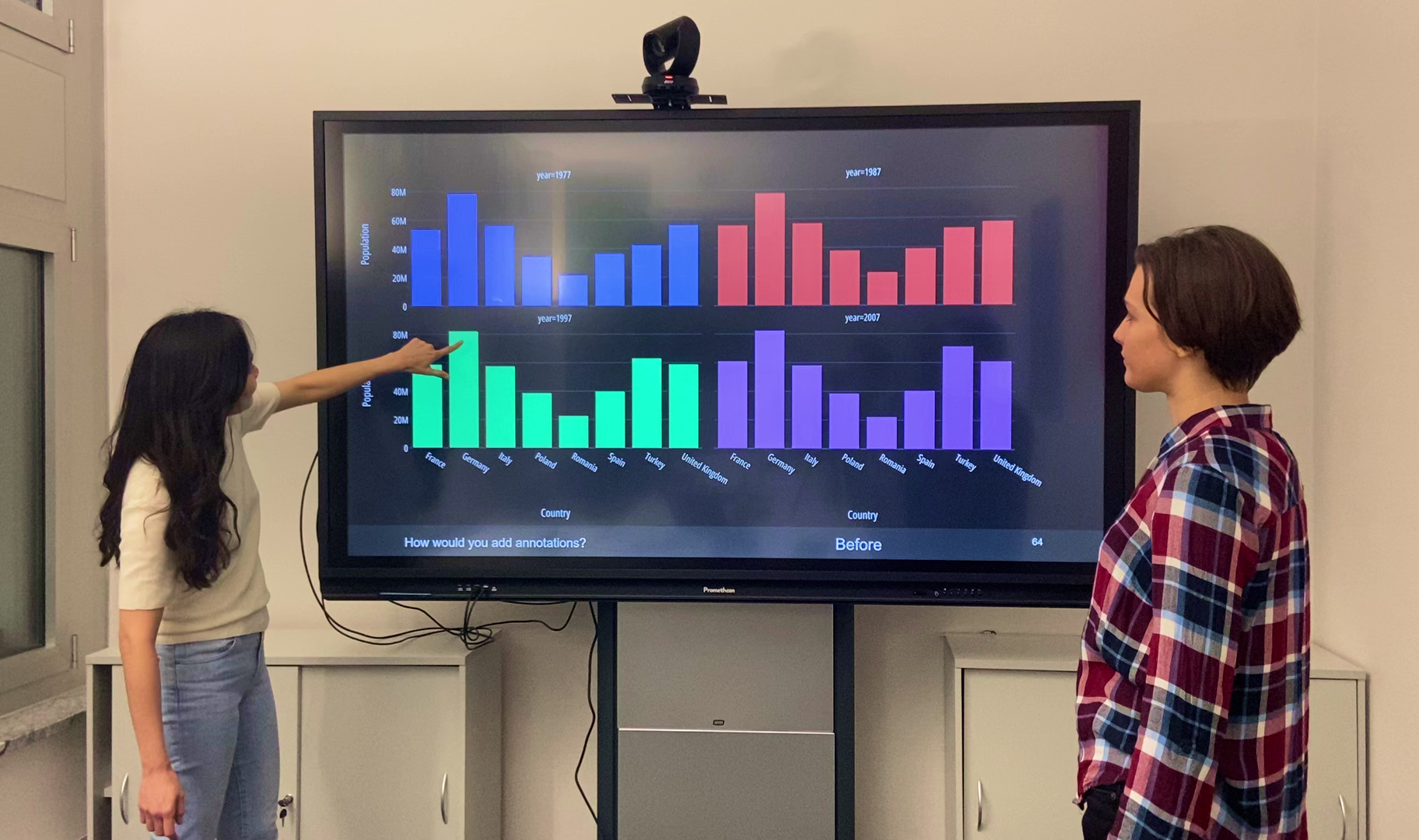
Fast forward
Keywords
Multimodal interaction, collaborative work, large vertical displays, elicitation study, spatio-temporal data
Abstract
We examined user preferences to combine multiple interaction modalities for collaborative interaction with data shown on large vertical displays. Large vertical displays facilitate visual data exploration and allow the use of diverse interaction modalities by multiple users at different distances from the screen. Yet, how to offer multiple interaction modalities is a non-trivial problem. We conducted an elicitation study with 20 participants that generated 1015 interaction proposals combining touch, speech, pen, and mid-air gestures. Given the opportunity to interact using these four 13 modalities, participants preferred speech interaction in 10 of 15 14 low-level tasks and direct manipulation for straightforward tasks 15 such as showing a tooltip or selecting. In contrast to previous work, 16 participants most favored unimodal and personal interactions. We 17 identified what we call collaborative synonyms among their interaction proposals and found that pairs of users collaborated either unimodally and simultaneously or multimodally and sequentially. We provide insights into how end-users associate visual exploration tasks with certain modalities and how they collaborate at different interaction distances using specific interaction modalities. The supplemental material is available at https://osf.io/m8zuh.
Interpreting High-Dimensional Projections With Capacity
Yang Zhang -
Jisheng Liu -
Chufan Lai -
Yuan Zhou -
Siming Chen -
DOI: 10.1109/TVCG.2023.3324851
Room: Bayshore V
2024-10-16T14:27:00Z GMT-0600 Change your timezone on the schedule page
2024-10-16T14:27:00Z

Fast forward
Abstract
Dimensionality reduction (DR) algorithms are diverse and widely used for analyzing high-dimensional data. Various metrics and tools have been proposed to evaluate and interpret the DR results. However, most metrics and methods fail to be well generalized to measure any DR results from the perspective of original distribution fidelity or lack interactive exploration of DR results. There is still a need for more intuitive and quantitative analysis to interactively explore high-dimensional data and improve interpretability. We propose a metric and a generalized algorithm-agnostic approach based on the concept of capacity to evaluate and analyze the DR results. Based on our approach, we develop a visual analytic system HiLow for exploring high-dimensional data and projections. We also propose a mixed-initiative recommendation algorithm that assists users in interactively DR results manipulation. Users can compare the differences in data distribution after the interaction through HiLow. Furthermore, we propose a novel visualization design focusing on quantitative analysis of differences between high and low-dimensional data distributions. Finally, through user study and case studies, we validate the effectiveness of our approach and system in enhancing the interpretability of projections and analyzing the distribution of high and low-dimensional data.
Interpreting High-Dimensional Projections With Capacity
Yang Zhang -
Jisheng Liu -
Chufan Lai -
Yuan Zhou -
Siming Chen -
DOI: 10.1109/TVCG.2023.3324851
Room: Bayshore V
2024-10-16T14:27:00Z GMT-0600 Change your timezone on the schedule page
2024-10-16T14:27:00Z

Fast forward
Abstract
Dimensionality reduction (DR) algorithms are diverse and widely used for analyzing high-dimensional data. Various metrics and tools have been proposed to evaluate and interpret the DR results. However, most metrics and methods fail to be well generalized to measure any DR results from the perspective of original distribution fidelity or lack interactive exploration of DR results. There is still a need for more intuitive and quantitative analysis to interactively explore high-dimensional data and improve interpretability. We propose a metric and a generalized algorithm-agnostic approach based on the concept of capacity to evaluate and analyze the DR results. Based on our approach, we develop a visual analytic system HiLow for exploring high-dimensional data and projections. We also propose a mixed-initiative recommendation algorithm that assists users in interactively DR results manipulation. Users can compare the differences in data distribution after the interaction through HiLow. Furthermore, we propose a novel visualization design focusing on quantitative analysis of differences between high and low-dimensional data distributions. Finally, through user study and case studies, we validate the effectiveness of our approach and system in enhancing the interpretability of projections and analyzing the distribution of high and low-dimensional data.
What Do We Mean When We Say “Insight”? A Formal Synthesis of Existing Theory
Leilani Battle -
Alvitta Ottley -
DOI: 10.1109/TVCG.2023.3326698
Room: Bayshore II
2024-10-16T15:15:00Z GMT-0600 Change your timezone on the schedule page
2024-10-16T15:15:00Z
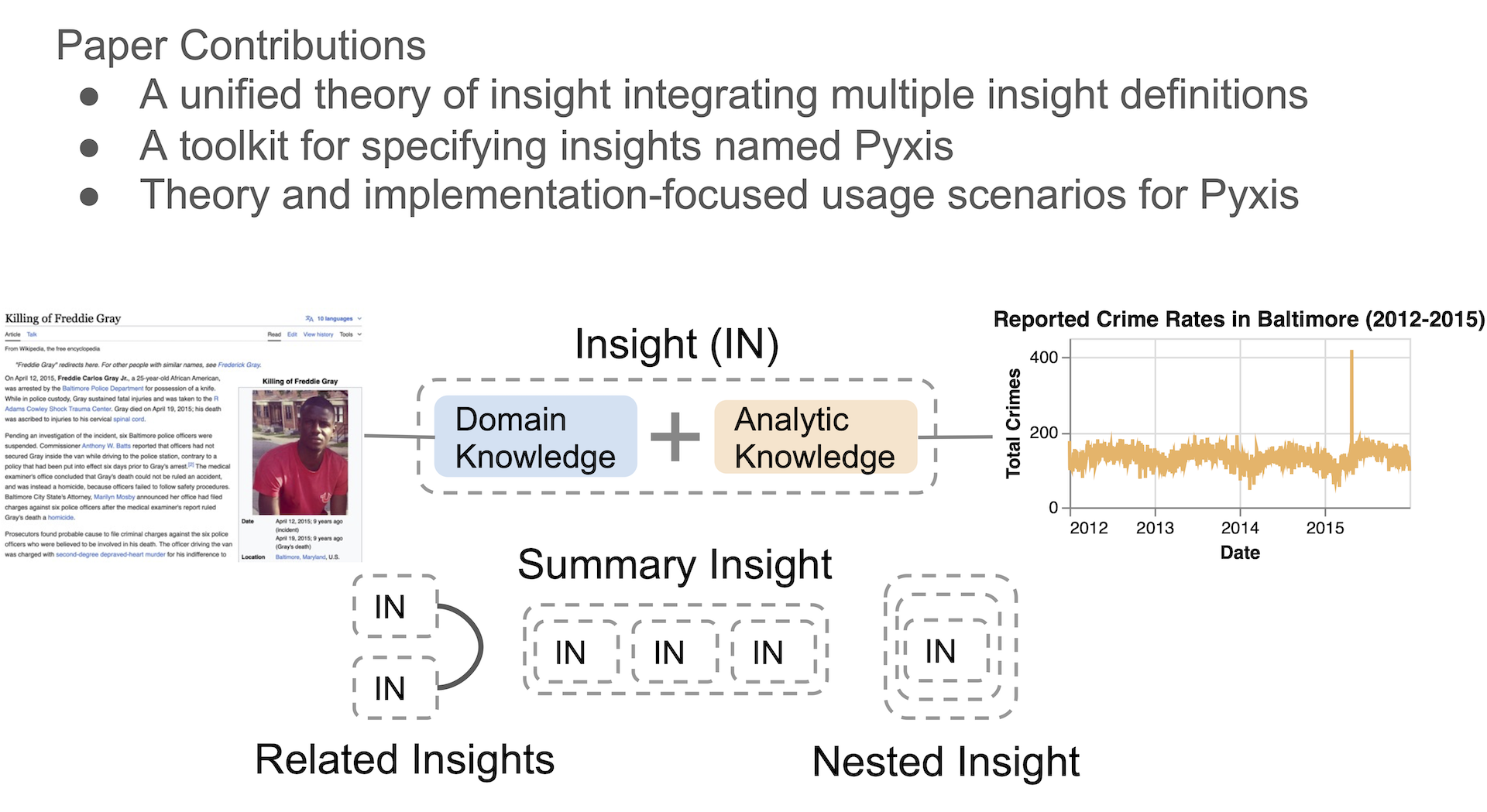
Fast forward
Abstract
Researchers have derived many theoretical models for specifying users’ insights as they interact with a visualization system. These representations are essential for understanding the insight discovery process, such as when inferring user interaction patterns that lead to insight or assessing the rigor of reported insights. However, theoretical models can be difficult to apply to existing tools and user studies, often due to discrepancies in how insight and its constituent parts are defined. This paper calls attention to the consistent structures that recur across the visualization literature and describes how they connect multiple theoretical representations of insight. We synthesize a unified formalism for insights using these structures, enabling a wider audience of researchers and developers to adopt the corresponding models. Through a series of theoretical case studies, we use our formalism to compare and contrast existing theories, revealing interesting research challenges in reasoning about a user's domain knowledge and leveraging synergistic approaches in data mining and data management research.
What Do We Mean When We Say “Insight”? A Formal Synthesis of Existing Theory
Leilani Battle -
Alvitta Ottley -
DOI: 10.1109/TVCG.2023.3326698
Room: Bayshore II
2024-10-16T15:15:00Z GMT-0600 Change your timezone on the schedule page
2024-10-16T15:15:00Z
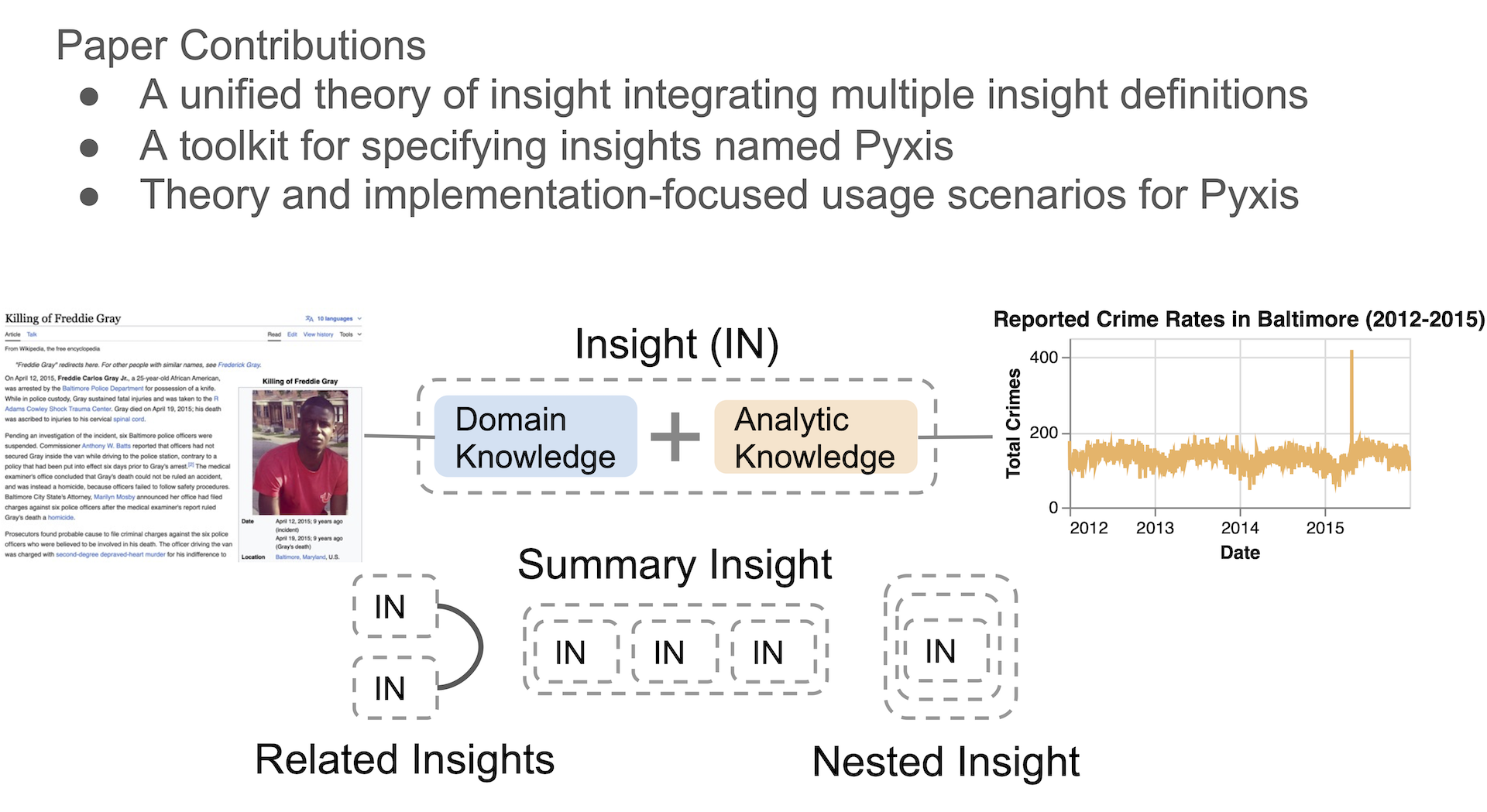
Fast forward
Abstract
Researchers have derived many theoretical models for specifying users’ insights as they interact with a visualization system. These representations are essential for understanding the insight discovery process, such as when inferring user interaction patterns that lead to insight or assessing the rigor of reported insights. However, theoretical models can be difficult to apply to existing tools and user studies, often due to discrepancies in how insight and its constituent parts are defined. This paper calls attention to the consistent structures that recur across the visualization literature and describes how they connect multiple theoretical representations of insight. We synthesize a unified formalism for insights using these structures, enabling a wider audience of researchers and developers to adopt the corresponding models. Through a series of theoretical case studies, we use our formalism to compare and contrast existing theories, revealing interesting research challenges in reasoning about a user's domain knowledge and leveraging synergistic approaches in data mining and data management research.
Wasserstein Dictionaries of Persistence Diagrams
Keanu Sisouk -
Julie Delon -
Julien Tierny -
Download preprint PDF
DOI: 10.1109/TVCG.2023.3330262
Room: Bayshore I
2024-10-17T14:51:00Z GMT-0600 Change your timezone on the schedule page
2024-10-17T14:51:00Z

Fast forward
Keywords
Topological data analysis, ensemble data, persistence diagrams
Abstract
This paper presents a computational framework for the concise encoding of an ensemble of persistence diagrams, in the form of weighted Wasserstein barycenters [100], [102] of a dictionary of atom diagrams. We introduce a multi-scale gradient descent approach for the efficient resolution of the corresponding minimization problem, which interleaves the optimization of the barycenter weights with the optimization of the atom diagrams. Our approach leverages the analytic expressions for the gradient of both sub-problems to ensure fast iterations and it additionally exploits shared-memory parallelism. Extensive experiments on public ensembles demonstrate the efficiency of our approach, with Wasserstein dictionary computations in the orders of minutes for the largest examples. We show the utility of our contributions in two applications. First, we apply Wassserstein dictionaries to data reduction and reliably compress persistence diagrams by concisely representing them with their weights in the dictionary. Second, we present a dimensionality reduction framework based on a Wasserstein dictionary defined with a small number of atoms (typically three) and encode the dictionary as a low dimensional simplex embedded in a visual space (typically in 2D). In both applications, quantitative experiments assess the relevance of our framework. Finally, we provide a C++ implementation that can be used to reproduce our results.
Wasserstein Dictionaries of Persistence Diagrams
Keanu Sisouk -
Julie Delon -
Julien Tierny -
Download preprint PDF
DOI: 10.1109/TVCG.2023.3330262
Room: Bayshore I
2024-10-17T14:51:00Z GMT-0600 Change your timezone on the schedule page
2024-10-17T14:51:00Z

Fast forward
Keywords
Topological data analysis, ensemble data, persistence diagrams
Abstract
This paper presents a computational framework for the concise encoding of an ensemble of persistence diagrams, in the form of weighted Wasserstein barycenters [100], [102] of a dictionary of atom diagrams. We introduce a multi-scale gradient descent approach for the efficient resolution of the corresponding minimization problem, which interleaves the optimization of the barycenter weights with the optimization of the atom diagrams. Our approach leverages the analytic expressions for the gradient of both sub-problems to ensure fast iterations and it additionally exploits shared-memory parallelism. Extensive experiments on public ensembles demonstrate the efficiency of our approach, with Wasserstein dictionary computations in the orders of minutes for the largest examples. We show the utility of our contributions in two applications. First, we apply Wassserstein dictionaries to data reduction and reliably compress persistence diagrams by concisely representing them with their weights in the dictionary. Second, we present a dimensionality reduction framework based on a Wasserstein dictionary defined with a small number of atoms (typically three) and encode the dictionary as a low dimensional simplex embedded in a visual space (typically in 2D). In both applications, quantitative experiments assess the relevance of our framework. Finally, we provide a C++ implementation that can be used to reproduce our results.
Submerse: Visualizing Storm Surge Flooding Simulations in Immersive Display Ecologies
Saeed Boorboor -
Yoonsang Kim -
Ping Hu -
Josef Moses -
Brian Colle -
Arie E. Kaufman -
DOI: 10.1109/TVCG.2023.3332511
Room: Bayshore VII
2024-10-16T14:15:00Z GMT-0600 Change your timezone on the schedule page
2024-10-16T14:15:00Z
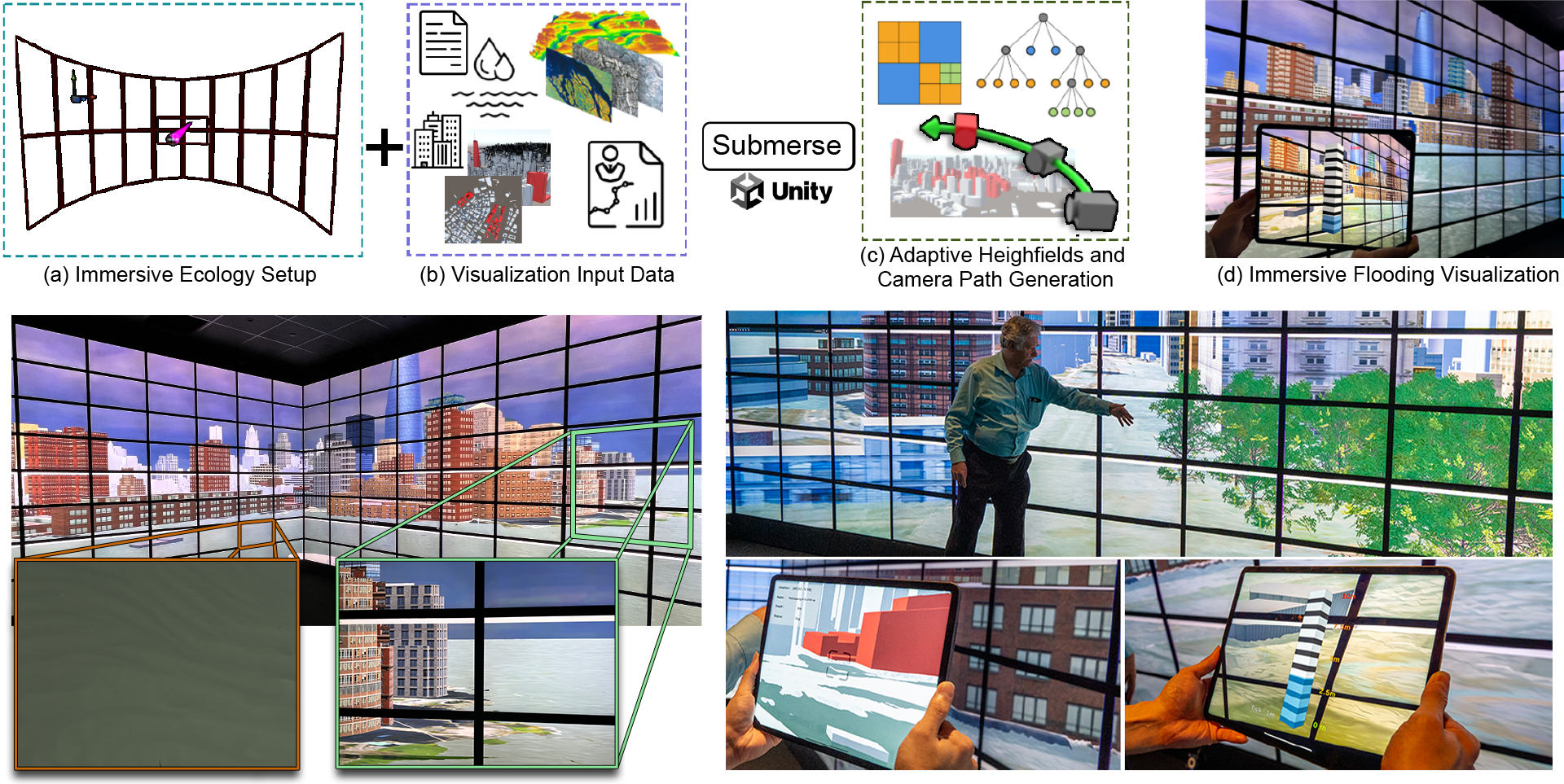
Fast forward
Keywords
Camera navigation, flooding simulation visualization, immersive visualization, mixed reality
Abstract
We present Submerse, an end-to-end framework for visualizing flooding scenarios on large and immersive display ecologies. Specifically, we reconstruct a surface mesh from input flood simulation data and generate a to-scale 3D virtual scene by incorporating geographical data such as terrain, textures, buildings, and additional scene objects. To optimize computation and memory performance for large simulation datasets, we discretize the data on an adaptive grid using dynamic quadtrees and support level-of-detail based rendering. Moreover, to provide a perception of flooding direction for a time instance, we animate the surface mesh by synthesizing water waves. As interaction is key for effective decision-making and analysis, we introduce two novel techniques for flood visualization in immersive systems: (1) an automatic scene-navigation method using optimal camera viewpoints generated for marked points-of-interest based on the display layout, and (2) an AR-based focus+context technique using an aux display system. Submerse is developed in collaboration between computer scientists and atmospheric scientists. We evaluate the effectiveness of our system and application by conducting workshops with emergency managers, domain experts, and concerned stakeholders in the Stony Brook Reality Deck, an immersive gigapixel facility, to visualize a superstorm flooding scenario in New York City.
Submerse: Visualizing Storm Surge Flooding Simulations in Immersive Display Ecologies
Saeed Boorboor -
Yoonsang Kim -
Ping Hu -
Josef Moses -
Brian Colle -
Arie E. Kaufman -
DOI: 10.1109/TVCG.2023.3332511
Room: Bayshore VII
2024-10-16T14:15:00Z GMT-0600 Change your timezone on the schedule page
2024-10-16T14:15:00Z
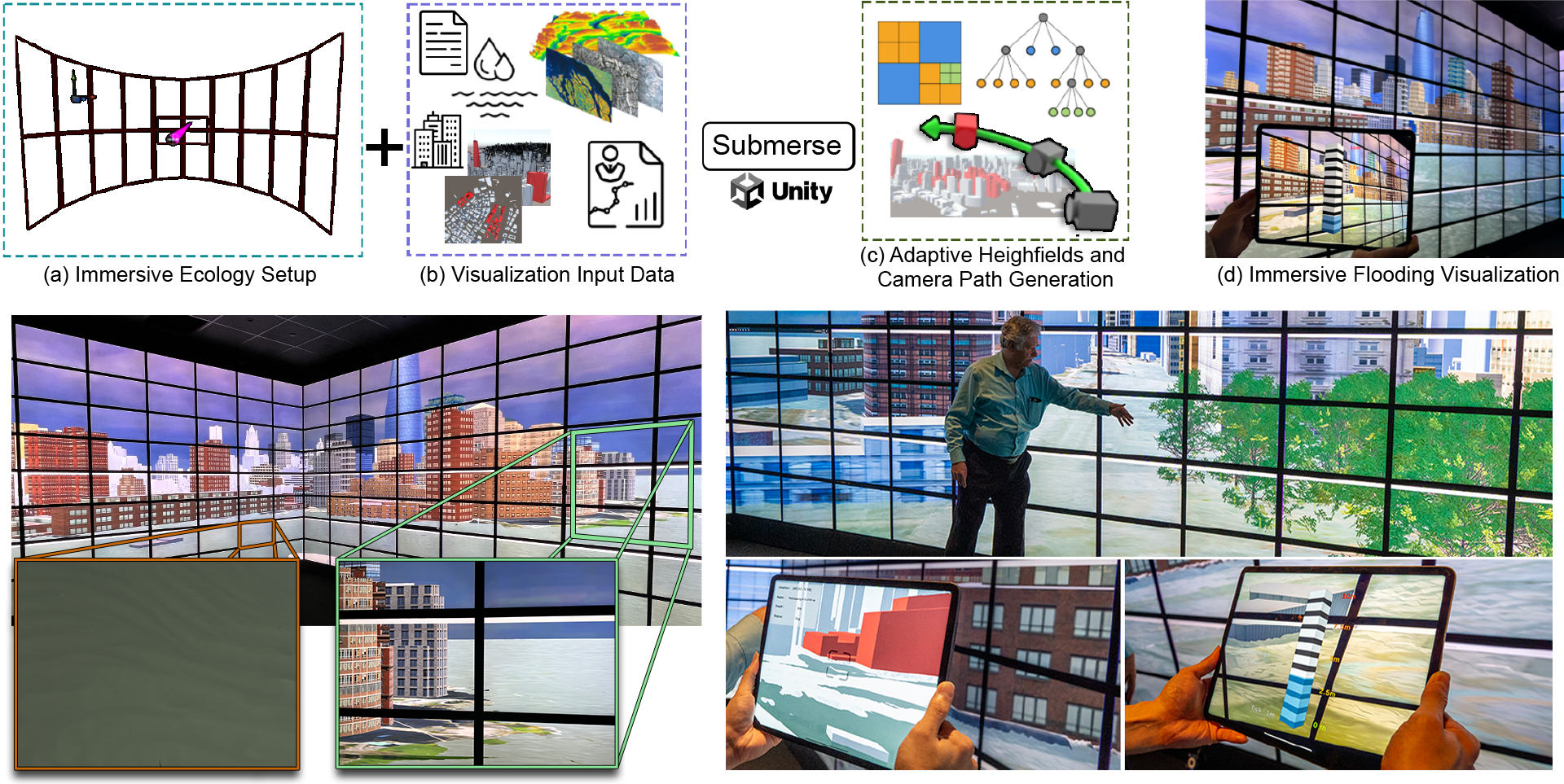
Fast forward
Keywords
Camera navigation, flooding simulation visualization, immersive visualization, mixed reality
Abstract
We present Submerse, an end-to-end framework for visualizing flooding scenarios on large and immersive display ecologies. Specifically, we reconstruct a surface mesh from input flood simulation data and generate a to-scale 3D virtual scene by incorporating geographical data such as terrain, textures, buildings, and additional scene objects. To optimize computation and memory performance for large simulation datasets, we discretize the data on an adaptive grid using dynamic quadtrees and support level-of-detail based rendering. Moreover, to provide a perception of flooding direction for a time instance, we animate the surface mesh by synthesizing water waves. As interaction is key for effective decision-making and analysis, we introduce two novel techniques for flood visualization in immersive systems: (1) an automatic scene-navigation method using optimal camera viewpoints generated for marked points-of-interest based on the display layout, and (2) an AR-based focus+context technique using an aux display system. Submerse is developed in collaboration between computer scientists and atmospheric scientists. We evaluate the effectiveness of our system and application by conducting workshops with emergency managers, domain experts, and concerned stakeholders in the Stony Brook Reality Deck, an immersive gigapixel facility, to visualize a superstorm flooding scenario in New York City.
QuantumEyes: Towards Better Interpretability of Quantum Circuits
Shaolun Ruan -
Qiang Guan -
Paul Griffin -
Ying Mao -
Yong Wang -
Screen-reader Accessible PDF
Download preprint PDF
DOI: 10.1109/TVCG.2023.3332999
Room: Bayshore V
2024-10-17T17:57:00Z GMT-0600 Change your timezone on the schedule page
2024-10-17T17:57:00Z

Fast forward
Keywords
Data visualization, design study, interpretability, quantum computing.
Abstract
Quantum computing offers significant speedup compared to classical computing, which has led to a growing interest among users in learning and applying quantum computing across various applications. However, quantum circuits, which are fundamental for implementing quantum algorithms, can be challenging for users to understand due to their underlying logic, such as the temporal evolution of quantum states and the effect of quantum amplitudes on the probability of basis quantum states. To fill this research gap, we propose QuantumEyes, an interactive visual analytics system to enhance the interpretability of quantum circuits through both global and local levels. For the global-level analysis, we present three coupled visualizations to delineate the changes of quantum states and the underlying reasons: a Probability Summary View to overview the probability evolution of quantum states; a State Evolution View to enable an in-depth analysis of the influence of quantum gates on the quantum states; a Gate Explanation View to show the individual qubit states and facilitate a better understanding of the effect of quantum gates. For the local-level analysis, we design a novel geometrical visualization dandelion chart to explicitly reveal how the quantum amplitudes affect the probability of the quantum state. We thoroughly evaluated QuantumEyes as well as the novel dandelion chart integrated into it through two case studies on different types of quantum algorithms and in-depth expert interviews with 12 domain experts. The results demonstrate the effectiveness and usability of our approach in enhancing the interpretability of quantum circuits.
QuantumEyes: Towards Better Interpretability of Quantum Circuits
Shaolun Ruan -
Qiang Guan -
Paul Griffin -
Ying Mao -
Yong Wang -
Screen-reader Accessible PDF
Download preprint PDF
DOI: 10.1109/TVCG.2023.3332999
Room: Bayshore V
2024-10-17T17:57:00Z GMT-0600 Change your timezone on the schedule page
2024-10-17T17:57:00Z

Fast forward
Keywords
Data visualization, design study, interpretability, quantum computing.
Abstract
Quantum computing offers significant speedup compared to classical computing, which has led to a growing interest among users in learning and applying quantum computing across various applications. However, quantum circuits, which are fundamental for implementing quantum algorithms, can be challenging for users to understand due to their underlying logic, such as the temporal evolution of quantum states and the effect of quantum amplitudes on the probability of basis quantum states. To fill this research gap, we propose QuantumEyes, an interactive visual analytics system to enhance the interpretability of quantum circuits through both global and local levels. For the global-level analysis, we present three coupled visualizations to delineate the changes of quantum states and the underlying reasons: a Probability Summary View to overview the probability evolution of quantum states; a State Evolution View to enable an in-depth analysis of the influence of quantum gates on the quantum states; a Gate Explanation View to show the individual qubit states and facilitate a better understanding of the effect of quantum gates. For the local-level analysis, we design a novel geometrical visualization dandelion chart to explicitly reveal how the quantum amplitudes affect the probability of the quantum state. We thoroughly evaluated QuantumEyes as well as the novel dandelion chart integrated into it through two case studies on different types of quantum algorithms and in-depth expert interviews with 12 domain experts. The results demonstrate the effectiveness and usability of our approach in enhancing the interpretability of quantum circuits.
SenseMap: Urban Performance Visualization and Analytics via Semantic Textual Similarity
Juntong Chen -
Qiaoyun Huang -
Changbo Wang -
Chenhui Li -
DOI: 10.1109/TVCG.2023.3333356
Room: Bayshore VII
2024-10-16T14:51:00Z GMT-0600 Change your timezone on the schedule page
2024-10-16T14:51:00Z
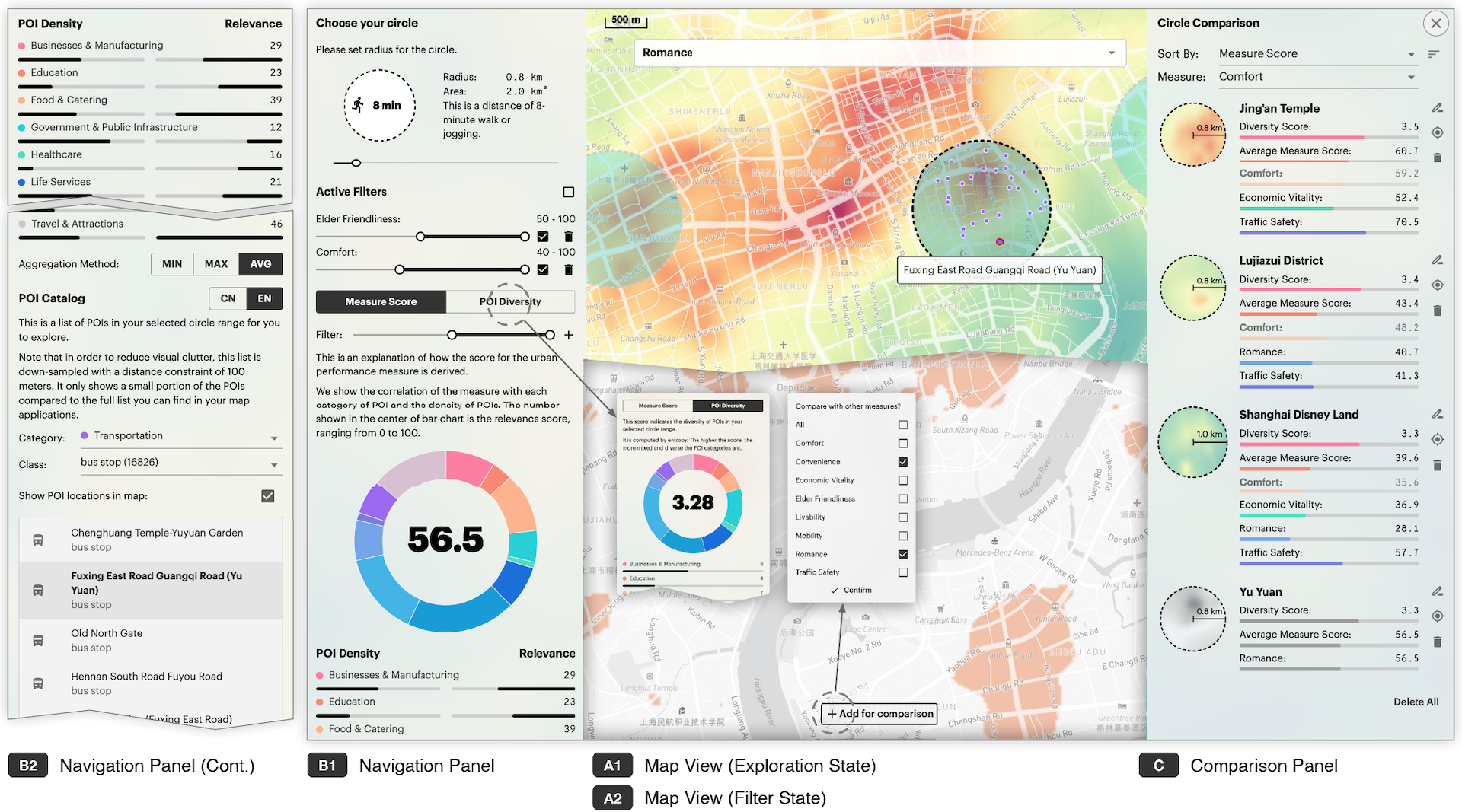
Fast forward
Keywords
Urban data, semantic textual similarity, point of interest, density map, visual analytics, visualization design
Abstract
As urban populations grow, effectively accessing urban performance measures such as livability and comfort becomes increasingly important due to their significant socioeconomic impacts. While Point of Interest (POI) data has been utilized for various applications in location-based services, its potential for urban performance analytics remains unexplored. In this paper, we present SenseMap, a novel approach for analyzing urban performance by leveraging POI data as a semantic representation of urban functions. We quantify the contribution of POIs to different urban performance measures by calculating semantic textual similarities on our constructed corpus. We propose Semantic-adaptive Kernel Density Estimation which takes into account POIs’ influential areas across different Traffic Analysis Zones and semantic contributions to generate semantic density maps for measures. We design and implement a feature-rich, real-time visual analytics system for users to explore the urban performance of their surroundings. Evaluations with human judgment and reference data demonstrate the feasibility and validity of our method. Usage scenarios and user studies demonstrate the capability, usability, and explainability of our system.
SenseMap: Urban Performance Visualization and Analytics via Semantic Textual Similarity
Juntong Chen -
Qiaoyun Huang -
Changbo Wang -
Chenhui Li -
DOI: 10.1109/TVCG.2023.3333356
Room: Bayshore VII
2024-10-16T14:51:00Z GMT-0600 Change your timezone on the schedule page
2024-10-16T14:51:00Z
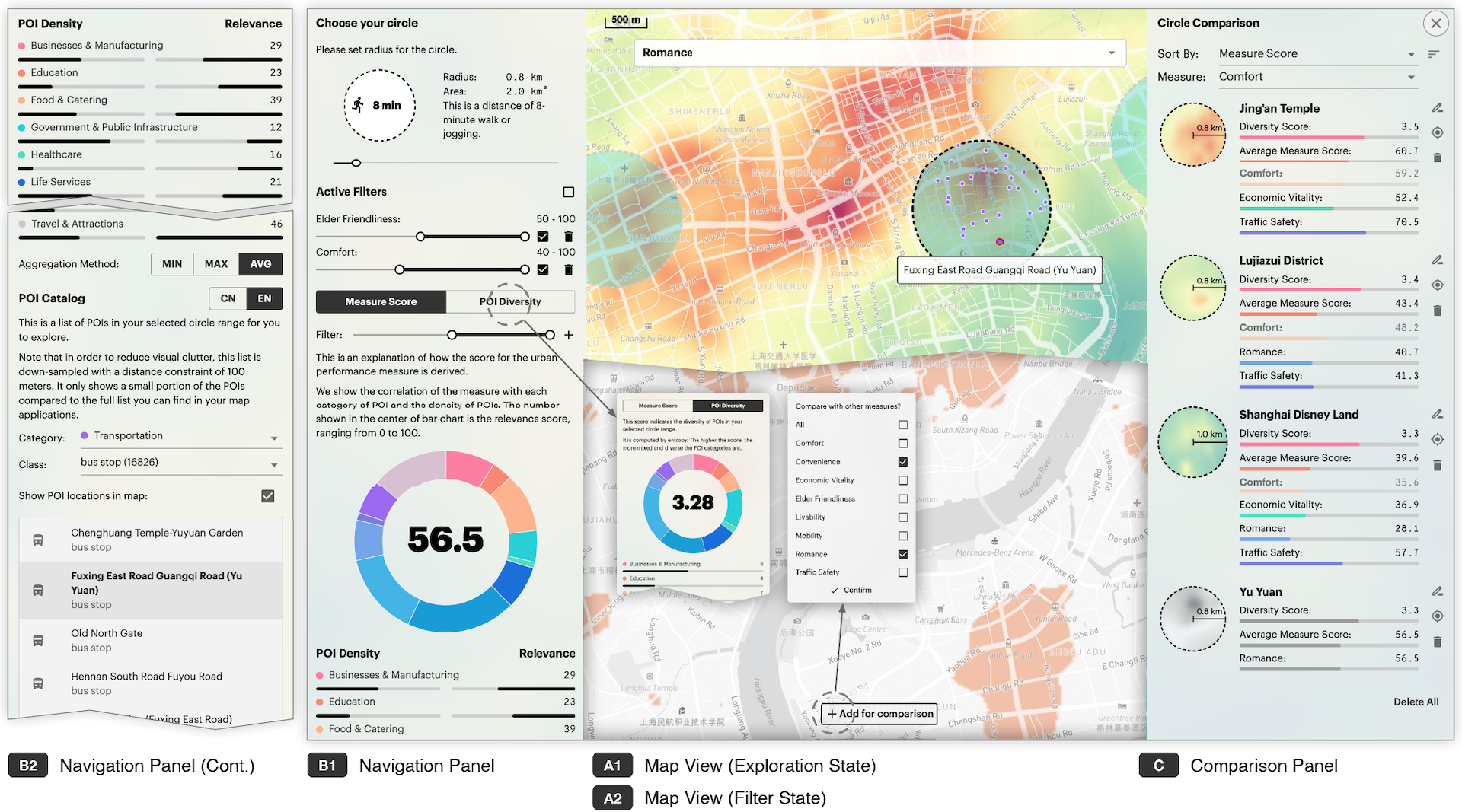
Fast forward
Keywords
Urban data, semantic textual similarity, point of interest, density map, visual analytics, visualization design
Abstract
As urban populations grow, effectively accessing urban performance measures such as livability and comfort becomes increasingly important due to their significant socioeconomic impacts. While Point of Interest (POI) data has been utilized for various applications in location-based services, its potential for urban performance analytics remains unexplored. In this paper, we present SenseMap, a novel approach for analyzing urban performance by leveraging POI data as a semantic representation of urban functions. We quantify the contribution of POIs to different urban performance measures by calculating semantic textual similarities on our constructed corpus. We propose Semantic-adaptive Kernel Density Estimation which takes into account POIs’ influential areas across different Traffic Analysis Zones and semantic contributions to generate semantic density maps for measures. We design and implement a feature-rich, real-time visual analytics system for users to explore the urban performance of their surroundings. Evaluations with human judgment and reference data demonstrate the feasibility and validity of our method. Usage scenarios and user studies demonstrate the capability, usability, and explainability of our system.
Preliminary Guidelines For Combining Data Integration and Visual Data Analysis
Adam Coscia -
Ashley Suh -
Remco Chang -
Alex Endert -
Screen-reader Accessible PDF
Download preprint PDF
DOI: 10.1109/TVCG.2023.3334513
Room: Bayshore II
2024-10-16T13:18:00Z GMT-0600 Change your timezone on the schedule page
2024-10-16T13:18:00Z
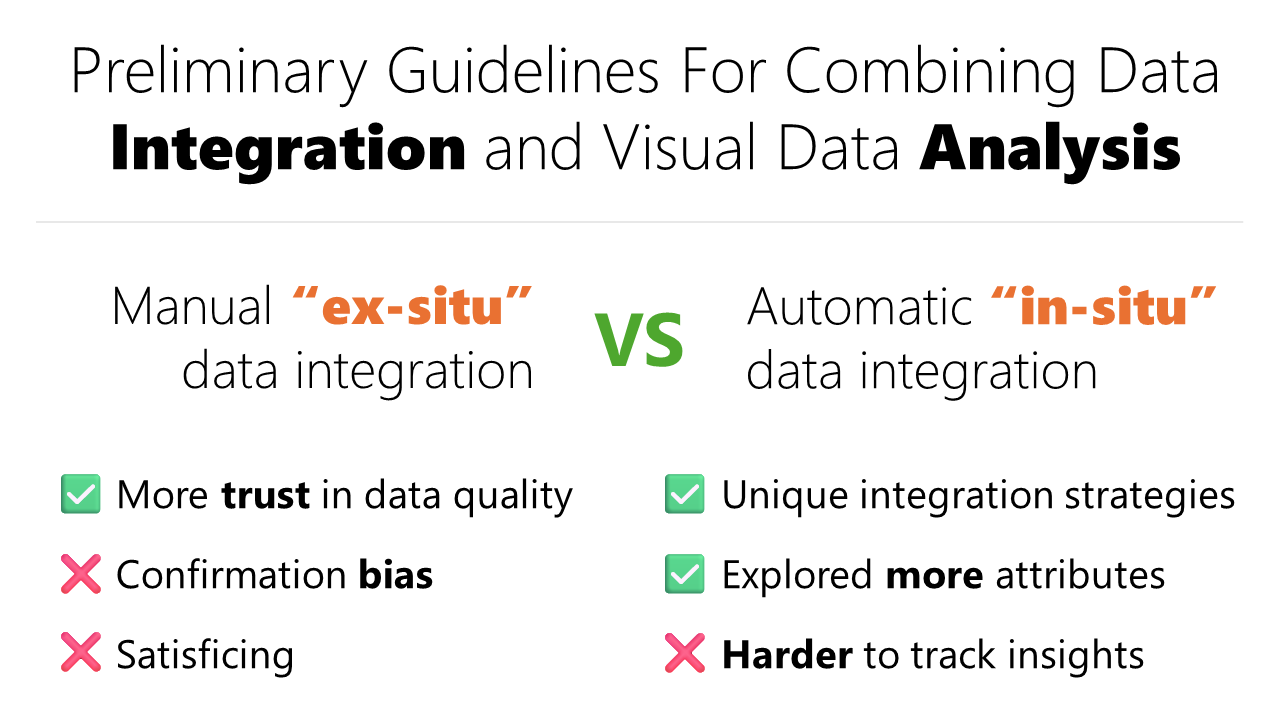
Fast forward
Keywords
Visual analytics, Data integration, User interface design, Integration strategies, Analytical behaviors.
Abstract
Data integration is often performed to consolidate information from multiple disparate data sources during visual data analysis. However, integration operations are usually separate from visual analytics operations such as encode and filter in both interface design and empirical research. We conducted a preliminary user study to investigate whether and how data integration should be incorporated directly into the visual analytics process. We used two interface alternatives featuring contrasting approaches to the data preparation and analysis workflow: manual file-based ex-situ integration as a separate step from visual analytics operations; and automatic UI-based in-situ integration merged with visual analytics operations. Participants were asked to complete specific and free-form tasks with each interface, browsing for patterns, generating insights, and summarizing relationships between attributes distributed across multiple files. Analyzing participants' interactions and feedback, we found both task completion time and total interactions to be similar across interfaces and tasks, as well as unique integration strategies between interfaces and emergent behaviors related to satisficing and cognitive bias. Participants' time spent and interactions revealed that in-situ integration enabled users to spend more time on analysis tasks compared with ex-situ integration. Participants' integration strategies and analytical behaviors revealed differences in interface usage for generating and tracking hypotheses and insights. With these results, we synthesized preliminary guidelines for designing future visual analytics interfaces that can support integrating attributes throughout an active analysis process.
Preliminary Guidelines For Combining Data Integration and Visual Data Analysis
Adam Coscia -
Ashley Suh -
Remco Chang -
Alex Endert -
Screen-reader Accessible PDF
Download preprint PDF
DOI: 10.1109/TVCG.2023.3334513
Room: Bayshore II
2024-10-16T13:18:00Z GMT-0600 Change your timezone on the schedule page
2024-10-16T13:18:00Z
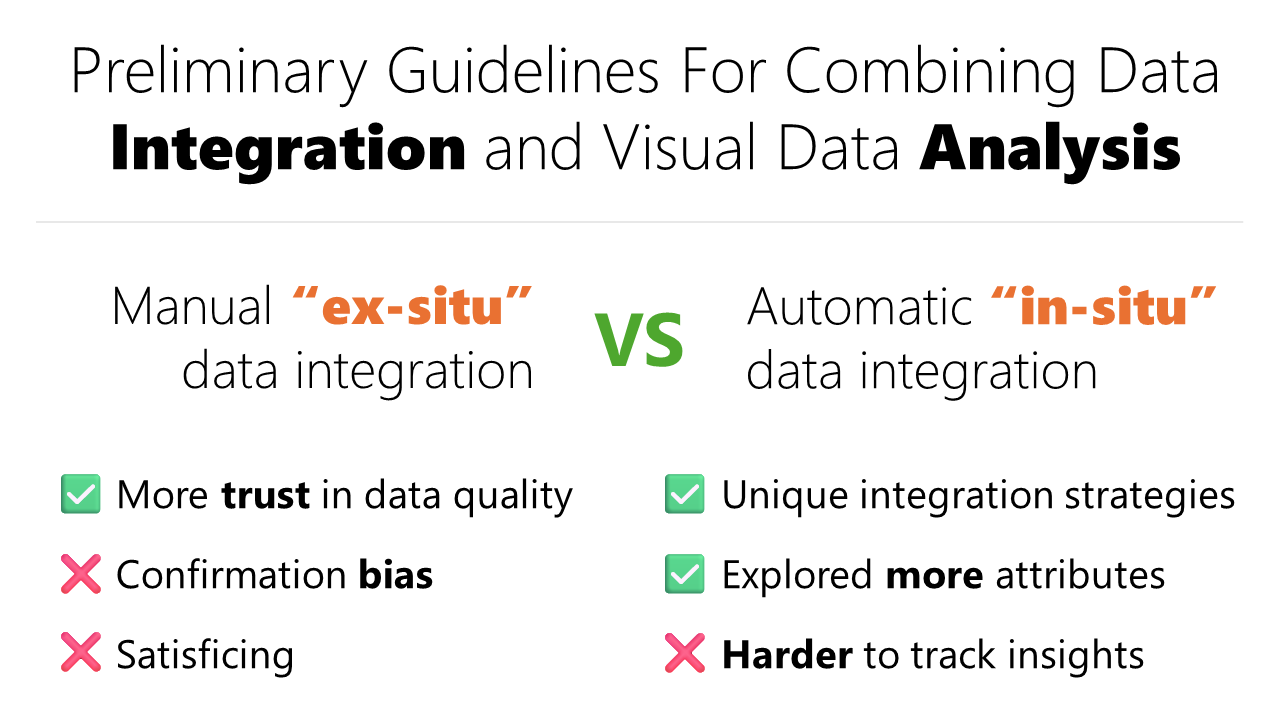
Fast forward
Keywords
Visual analytics, Data integration, User interface design, Integration strategies, Analytical behaviors.
Abstract
Data integration is often performed to consolidate information from multiple disparate data sources during visual data analysis. However, integration operations are usually separate from visual analytics operations such as encode and filter in both interface design and empirical research. We conducted a preliminary user study to investigate whether and how data integration should be incorporated directly into the visual analytics process. We used two interface alternatives featuring contrasting approaches to the data preparation and analysis workflow: manual file-based ex-situ integration as a separate step from visual analytics operations; and automatic UI-based in-situ integration merged with visual analytics operations. Participants were asked to complete specific and free-form tasks with each interface, browsing for patterns, generating insights, and summarizing relationships between attributes distributed across multiple files. Analyzing participants' interactions and feedback, we found both task completion time and total interactions to be similar across interfaces and tasks, as well as unique integration strategies between interfaces and emergent behaviors related to satisficing and cognitive bias. Participants' time spent and interactions revealed that in-situ integration enabled users to spend more time on analysis tasks compared with ex-situ integration. Participants' integration strategies and analytical behaviors revealed differences in interface usage for generating and tracking hypotheses and insights. With these results, we synthesized preliminary guidelines for designing future visual analytics interfaces that can support integrating attributes throughout an active analysis process.
Wasserstein Auto-Encoders of Merge Trees (and Persistence Diagrams)
Mathieu Pont -
Julien Tierny -
DOI: 10.1109/TVCG.2023.3334755
Room: Bayshore I
2024-10-17T15:03:00Z GMT-0600 Change your timezone on the schedule page
2024-10-17T15:03:00Z
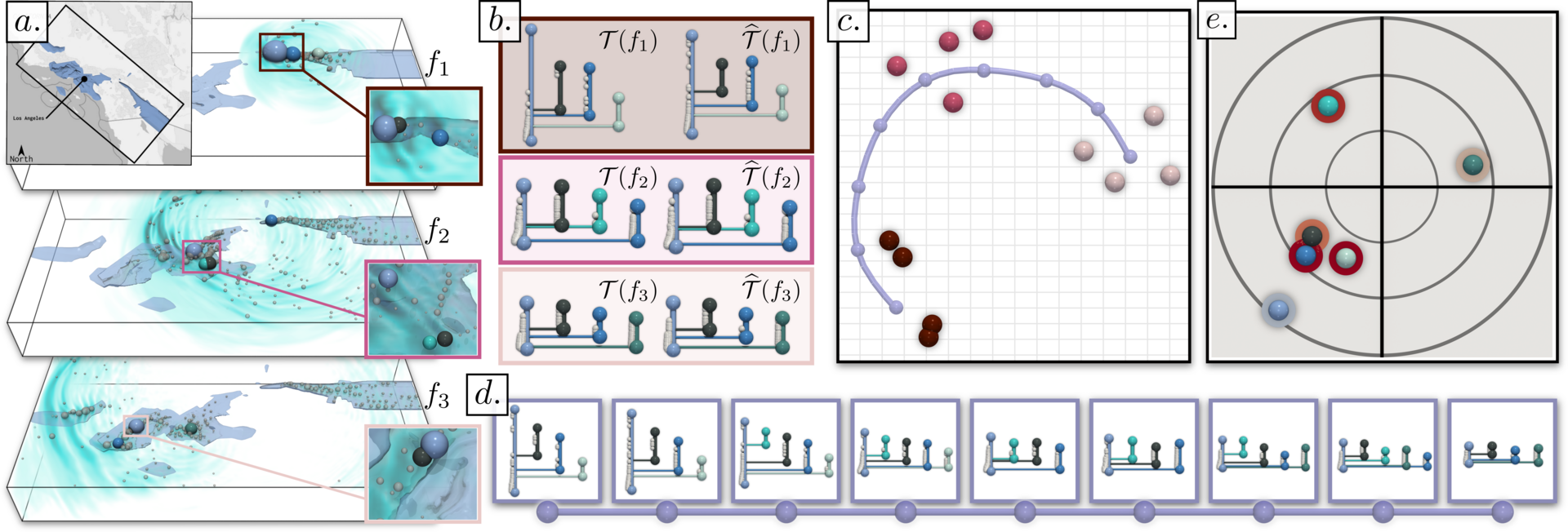
Fast forward
Keywords
Topological data analysis, ensemble data, persistence diagrams, merge trees, auto-encoders, neural networks
Abstract
This paper presents a computational framework for the Wasserstein auto-encoding of merge trees (MT-WAE), a novel extension of the classical auto-encoder neural network architecture to the Wasserstein metric space of merge trees. In contrast to traditional auto-encoders which operate on vectorized data, our formulation explicitly manipulates merge trees on their associated metric space at each layer of the network, resulting in superior accuracy and interpretability. Our novel neural network approach can be interpreted as a non-linear generalization of previous linear attempts [79] at merge tree encoding. It also trivially extends to persistence diagrams. Extensive experiments on public ensembles demonstrate the efficiency of our algorithms, with MT-WAE computations in the orders of minutes on average. We show the utility of our contributions in two applications adapted from previous work on merge tree encoding [79]. First, we apply MT-WAE to merge tree compression, by concisely representing them with their coordinates in the final layer of our auto-encoder. Second, we document an application to dimensionality reduction, by exploiting the latent space of our auto-encoder, for the visual analysis of ensemble data. We illustrate the versatility of our framework by introducing two penalty terms, to help preserve in the latent space both the Wasserstein distances between merge trees, as well as their clusters. In both applications, quantitative experiments assess the relevance of our framework. Finally, we provide a C++ implementation that can be used for reproducibility.