diff --git a/.Rbuildignore b/.Rbuildignore
new file mode 100644
index 0000000..91114bf
--- /dev/null
+++ b/.Rbuildignore
@@ -0,0 +1,2 @@
+^.*\.Rproj$
+^\.Rproj\.user$
diff --git a/.gitattributes b/.gitattributes
new file mode 100644
index 0000000..8bce350
--- /dev/null
+++ b/.gitattributes
@@ -0,0 +1 @@
+*.qmd linguist-language=RMarkdown
diff --git a/.github/dependabot.yml b/.github/dependabot.yml
new file mode 100644
index 0000000..90e05c4
--- /dev/null
+++ b/.github/dependabot.yml
@@ -0,0 +1,11 @@
+# To get started with Dependabot version updates, you'll need to specify which
+# package ecosystems to update and where the package manifests are located.
+# Please see the documentation for all configuration options:
+# https://docs.github.com/github/administering-a-repository/configuration-options-for-dependency-updates
+
+version: 2
+updates:
+ - package-ecosystem: "github-actions" # See documentation for possible values
+ directory: "/" # Location of package manifests
+ schedule:
+ interval: "weekly"
diff --git a/.github/workflows/deploy.yaml b/.github/workflows/deploy.yaml
new file mode 100644
index 0000000..4c1f48a
--- /dev/null
+++ b/.github/workflows/deploy.yaml
@@ -0,0 +1,64 @@
+# Workflow derived from https://github.com/r-lib/actions/tree/master/examples
+# Need help debugging build failures? Start at https://github.com/r-lib/actions#where-to-find-help
+on:
+ workflow_dispatch:
+ schedule:
+ - cron: '0 0 1 * *' # Run at 00:00 on the 1st of every month
+ push:
+ branches: [main]
+ pull_request:
+ merge_group:
+
+name: deploy
+
+jobs:
+ deploy:
+ runs-on: ubuntu-latest
+ env:
+ GITHUB_PAT: ${{ secrets.GITHUB_TOKEN }}
+ LANG: "en_US.UTF-8"
+ LC_ALL: "en_US.UTF-8"
+ steps:
+ - uses: actions/checkout@v4
+
+ - uses: quarto-dev/quarto-actions/setup@v2
+
+ - uses: r-lib/actions/setup-r@v2
+ with:
+ use-public-rspm: true
+ extra-repositories: 'https://stan-dev.r-universe.dev'
+
+ - name: Install dependencies
+ uses: r-lib/actions/setup-r-dependencies@v2
+ with:
+ extra-packages:
+ any::gh,
+ any::knitr,
+ any::rmarkdown,
+ local::.,
+
+ - name: Install cmdstan
+ uses: epinowcast/actions/install-cmdstan@v1
+ with:
+ cmdstan-version: 'latest'
+ num-cores: 2
+
+ - name: Render site
+ run: quarto render
+
+ - name: Upload website
+ uses: actions/upload-artifact@v4
+ if: github.event_name == 'pull_request'
+ with:
+ name: site
+ retention-days: 5
+ path: _site
+
+ - name: Deploy to GitHub pages 🚀
+ if: github.ref_name == 'main'
+ uses: JamesIves/github-pages-deploy-action@v4.6.8
+ with:
+ branch: gh-pages
+ folder: _site
+ single-commit: true
+ clean: true
diff --git a/.github/workflows/render-readme.yaml b/.github/workflows/render-readme.yaml
new file mode 100644
index 0000000..05ec6aa
--- /dev/null
+++ b/.github/workflows/render-readme.yaml
@@ -0,0 +1,64 @@
+name: render-readme
+
+concurrency:
+ group: ${{ github.workflow }}-${{ github.head_ref }}
+ cancel-in-progress: true
+
+on:
+ workflow_dispatch:
+ push:
+ branches:
+ - main
+
+jobs:
+ render-readme:
+ runs-on: macos-latest
+ env:
+ GITHUB_PAT: ${{ secrets.GITHUB_TOKEN }}
+ steps:
+ - name: Checkout repos
+ uses: actions/checkout@v4
+
+ - name: Setup R
+ uses: r-lib/actions/setup-r@v2
+ with:
+ use-public-rspm: true
+ extra-repositories: 'https://stan-dev.r-universe.dev'
+
+ - name: Setup pandoc
+ uses: r-lib/actions/setup-pandoc@v2
+
+ - name: Install dependencies
+ uses: r-lib/actions/setup-r-dependencies@v2
+ with:
+ extra-packages: any::rmarkdown, any::allcontributors
+
+ - name: Update contributors
+ if: github.ref == 'refs/heads/main'
+ run: allcontributors::add_contributors(format = "text", check_urls = FALSE)
+ shell: Rscript {0}
+
+ - name: Compile the readme
+ run: |
+ rmarkdown::render("README.Rmd")
+ shell: Rscript {0}
+
+ - name: Upload README.md as an artifact
+ uses: actions/upload-artifact@v4
+ with:
+ name: readme
+ path: README.md
+
+ - name: Create Pull Request
+ if: github.ref == 'refs/heads/main'
+ uses: peter-evans/create-pull-request@v7
+ with:
+ commit-message: "Automatic README update"
+ title: "Update README"
+ body: "This is an automated pull request to update the README."
+ branch: "update-readme-${{ github.run_number }}"
+ labels: "documentation"
+ add-paths: |
+ README.Rmd
+ README.md
+ token: ${{ secrets.GITHUB_TOKEN }}
diff --git a/.gitignore b/.gitignore
new file mode 100644
index 0000000..fefa29a
--- /dev/null
+++ b/.gitignore
@@ -0,0 +1,16 @@
+.Rproj.user
+.Rhistory
+.RData
+.Ruserdata
+_site
+.DS_Store
+*_files/
+/.quarto/
+inst/stan/simple-nowcast
+inst/stan/*
+!inst/stan/functions
+!inst/stan/*.stan
+*.html
+*.rmarkdown
+.vscode/
+*pdf
diff --git a/.nojekyll b/.nojekyll
new file mode 100644
index 0000000..e69de29
diff --git a/DESCRIPTION b/DESCRIPTION
new file mode 100644
index 0000000..e258c46
--- /dev/null
+++ b/DESCRIPTION
@@ -0,0 +1,42 @@
+Package: nfidd
+Title: Material to support course on nowcasting and forecasting of infectious
+ disease dynamics
+Version: 0.1.0.9000
+Authors@R: c(
+ person(
+ given = "NFIDD course contributors",
+ family = "",
+ role = c("cre", "aut"),
+ email = "epiforecasts@gmail.com"
+ )
+ )
+Description: Resources to support a short course on nowcasting and forecasting
+ of infectious disease dynamics.
+License: MIT + file LICENSE
+URL: https://github.com/nfidd/nfidd
+BugReports: https://github.com/nfidd/nfidd/issues
+Depends:
+ R (>= 4.2.0)
+Imports:
+ dplyr,
+ tidyr
+Suggests:
+ bayesplot,
+ cmdstanr,
+ ggplot2,
+ lubridate,
+ posterior,
+ purrr,
+ rmarkdown,
+ scoringutils,
+ tidybayes,
+ qra
+Remotes:
+ epiforecasts/scoringutils@b370557,
+ epiforecasts/qra@v0.1.0
+Additional_repositories:
+ https://stan-dev.r-universe.dev
+Encoding: UTF-8
+Language: en-GB
+RoxygenNote: 7.3.2
+LazyData: true
diff --git a/LICENSE b/LICENSE
new file mode 100644
index 0000000..f8eaaaf
--- /dev/null
+++ b/LICENSE
@@ -0,0 +1,21 @@
+MIT License
+
+Copyright (c) 2024 NFIDD contributors
+
+Permission is hereby granted, free of charge, to any person obtaining a copy
+of this software and associated documentation files (the "Software"), to deal
+in the Software without restriction, including without limitation the rights
+to use, copy, modify, merge, publish, distribute, sublicense, and/or sell
+copies of the Software, and to permit persons to whom the Software is
+furnished to do so, subject to the following conditions:
+
+The above copyright notice and this permission notice shall be included in all
+copies or substantial portions of the Software.
+
+THE SOFTWARE IS PROVIDED "AS IS", WITHOUT WARRANTY OF ANY KIND, EXPRESS OR
+IMPLIED, INCLUDING BUT NOT LIMITED TO THE WARRANTIES OF MERCHANTABILITY,
+FITNESS FOR A PARTICULAR PURPOSE AND NONINFRINGEMENT. IN NO EVENT SHALL THE
+AUTHORS OR COPYRIGHT HOLDERS BE LIABLE FOR ANY CLAIM, DAMAGES OR OTHER
+LIABILITY, WHETHER IN AN ACTION OF CONTRACT, TORT OR OTHERWISE, ARISING FROM,
+OUT OF OR IN CONNECTION WITH THE SOFTWARE OR THE USE OR OTHER DEALINGS IN THE
+SOFTWARE.
diff --git a/NAMESPACE b/NAMESPACE
new file mode 100644
index 0000000..b87ba6c
--- /dev/null
+++ b/NAMESPACE
@@ -0,0 +1,34 @@
+# Generated by roxygen2: do not edit by hand
+
+export(add_delays)
+export(censored_delay_pmf)
+export(convolve_with_delay)
+export(geometric_diff_ar)
+export(geometric_random_walk)
+export(make_daily_infections)
+export(make_gen_time_pmf)
+export(make_ip_pmf)
+export(nfidd_cmdstan_model)
+export(nfidd_load_stan_functions)
+export(nfidd_stan_function_files)
+export(nfidd_stan_functions)
+export(nfidd_stan_models)
+export(nfidd_stan_path)
+export(renewal)
+export(simulate_onsets)
+importFrom(dplyr,count)
+importFrom(dplyr,full_join)
+importFrom(dplyr,if_else)
+importFrom(dplyr,join_by)
+importFrom(dplyr,left_join)
+importFrom(dplyr,mutate)
+importFrom(dplyr,n)
+importFrom(dplyr,select)
+importFrom(dplyr,tibble)
+importFrom(dplyr,transmute)
+importFrom(stats,rbinom)
+importFrom(stats,rgamma)
+importFrom(stats,rlnorm)
+importFrom(stats,rpois)
+importFrom(tidyr,expand)
+importFrom(tidyr,replace_na)
diff --git a/NEWS.md b/NEWS.md
new file mode 100644
index 0000000..12ab8fc
--- /dev/null
+++ b/NEWS.md
@@ -0,0 +1,7 @@
+# nfidd 0.1.0.9000
+
+In development version of the package and teaching material for teaching in Bangkok in November 2024.
+
+# nfidd 0.1.0
+
+Initial release of the `nfidd` package and teaching material for teaching in June 2024 in Stockholm.
\ No newline at end of file
diff --git a/R/add_delays.r b/R/add_delays.r
new file mode 100644
index 0000000..05db107
--- /dev/null
+++ b/R/add_delays.r
@@ -0,0 +1,37 @@
+#' Simulate symptom onset and hospitalization times from infection times
+#'
+#' @param infection_times A data frame containing a column of infection times
+#'
+#' @return A data frame with columns for infection time, onset time, and
+#' hospitalization time (with 70% of hospitalizations set to NA)
+#'
+#' @importFrom dplyr mutate n if_else
+#' @importFrom stats rgamma rlnorm rbinom
+#'
+#' @export
+#'
+#' @examples
+#' add_delays(infection_times)
+add_delays <- function(infection_times) {
+ # Add random delays from appropriate probability distributions
+ df <- infection_times |>
+ mutate(
+ # Gamma distribution for onset times
+ onset_time = infection_time + rgamma(n(), shape = 5, rate = 1),
+ # Lognormal distribution for hospitalisation times
+ hosp_time = onset_time + rlnorm(n(), meanlog = 1.75, sdlog = 0.5)
+ )
+
+ # Set random 70% of hospitalization dates to NA (30% hospitalized)
+ df <- df |>
+ mutate(
+ hosp_time = if_else(
+ # use the binomial distribution for random binary outcomes
+ rbinom(n = n(), size = 1, prob = 0.3) == 1,
+ hosp_time,
+ NA_real_
+ )
+ )
+
+ return(df)
+}
diff --git a/R/censored-delay-pmf.r b/R/censored-delay-pmf.r
new file mode 100644
index 0000000..debe94f
--- /dev/null
+++ b/R/censored-delay-pmf.r
@@ -0,0 +1,33 @@
+#' Discretise a Continuous Delay Distribution
+#'
+#' This function discretises a continuous delay distribution into a probability
+#' mass function (PMF) over discrete days.
+#'
+#' @param rgen A function that generates random delays, e.g., `rgamma`,
+#' `rlnorm`.
+#' @param max The maximum delay.
+#' @param n The number of replicates to simulate. Defaults to `1e+6`.
+#' @param ... Additional parameters of the delay distribution.
+#'
+#' @return A vector of probabilities corresponding to discrete indices from
+#' `0` to `max`, representing the discretised delay distribution.
+#'
+#' @export
+#'
+#' @examples
+#' censored_delay_pmf(rgen = rgamma, max = 14, shape = 5, rate = 1)
+censored_delay_pmf <- function(rgen, max, n = 1e+6, ...) {
+ ## first, simulate exact time of first event (given by day), uniformly
+ ## between 0 and 1
+ first <- runif(n, min = 0, max = 1)
+ ## now, simulate the exact time of the second event
+ second <- first + rgen(n, ...)
+ ## round down to get the delay in days
+ delay <- floor(second)
+ ## get vector of counts
+ counts <- table(factor(delay, levels = seq(0, max)))
+ ## normalise to get pmf
+ pmf <- counts / sum(counts)
+ ## return
+ return(pmf)
+}
diff --git a/R/convolve-with-delay.r b/R/convolve-with-delay.r
new file mode 100644
index 0000000..ffca294
--- /dev/null
+++ b/R/convolve-with-delay.r
@@ -0,0 +1,30 @@
+#' Convolve a Time Series with a Delay Distribution
+#'
+#' This function convolves a time series with a delay distribution given as a
+#' probability mass function (PMF).
+#'
+#' @param ts A vector of the time series to convolve.
+#' @param delay_pmf The probability mass function of the delay, given as a
+#' vector of probabilities, corresponding to discrete indices 0, 1, 2 of the
+#' discretised delay distribution.
+#'
+#' @return A vector of the convolved time series.
+#'
+#' @export
+#'
+#' @examples
+#' convolve_with_delay(ts = c(10, 14, 10, 10), delay_pmf = c(0.1, 0.6, 0.3))
+convolve_with_delay <- function(ts, delay_pmf) {
+ max_delay <- length(delay_pmf) - 1 ## subtract one because zero-indexed
+ convolved <- vapply(seq_along(ts), \(i) {
+ ## get vector of infections over the possible window of the delay period
+ first_index <- max(1, i - max_delay)
+ ts_segment <- ts[seq(first_index, i)]
+ ## take reverse of pmf and cut if needed
+ pmf <- rev(delay_pmf)[seq_len(i - first_index + 1)]
+ ## convolve with delay distribution
+ ret <- sum(ts_segment * pmf)
+ return(ret)
+ }, numeric(1))
+ return(convolved)
+}
diff --git a/R/geometric-diff-ar.r b/R/geometric-diff-ar.r
new file mode 100644
index 0000000..1c15032
--- /dev/null
+++ b/R/geometric-diff-ar.r
@@ -0,0 +1,31 @@
+#' Geometric Differenced Autoregressive Process
+#'
+#' This function generates a geometric differenced autoregressive process.
+#'
+#' @param init The initial value.
+#' @param noise A vector of steps (on the standard normal scale).
+#' @param std The step size of the random walk.
+#' @param damp A damping parameter.
+#'
+#' @return A vector of the generated geometric differenced autoregressive
+#' process.
+#'
+#' @export
+#'
+#' @examples
+#' geometric_diff_ar(init = 1, noise = rnorm(100), phi = 0.1, std = 0.1)
+geometric_diff_ar <- function(init, noise, std, damp) {
+ ## number of values: initial and each of the steps
+ n <- length(noise) + 1
+ ## declare vector
+ x <- numeric(n)
+ ## initial value
+ x[1] <- init
+ ## second value (no difference yet available for use)
+ x[2] <- x[1] + noise[1] * std
+ ## random walk
+ for (i in 3:n) {
+ x[i] <- x[i - 1] + damp * (x[i - 1] - x[i - 2]) + noise[i - 1] * std
+ }
+ return(exp(x))
+}
diff --git a/R/geometric-random-walk.r b/R/geometric-random-walk.r
new file mode 100644
index 0000000..b2ec343
--- /dev/null
+++ b/R/geometric-random-walk.r
@@ -0,0 +1,27 @@
+#' Geometric Random Walk
+#'
+#' This function generates a geometric random walk.
+#'
+#' @param init The initial value.
+#' @param noise A vector of steps (on the standard normal scale).
+#' @param std The step size of the random walk.
+#'
+#' @return A vector of the generated geometric random walk.
+#'
+#' @export
+#'
+#' @examples
+#' geometric_random_walk(init = 1, noise = rnorm(100), std = 0.1)
+geometric_random_walk <- function(init, noise, std) {
+ ## number of values: initial and each of the steps
+ n <- length(noise) + 1
+ ## declare vector
+ x <- numeric(n)
+ ## initial value
+ x[1] <- init
+ ## random walk
+ for (i in 2:n) {
+ x[i] <- x[i - 1] + noise[i - 1] * std
+ }
+ return(exp(x))
+}
diff --git a/R/make-daily-infections.r b/R/make-daily-infections.r
new file mode 100644
index 0000000..96158b0
--- /dev/null
+++ b/R/make-daily-infections.r
@@ -0,0 +1,33 @@
+#' Convert infection times to a daily time series
+#'
+#' @param infection_times A data frame containing a column of infection times
+#'
+#' @return A data frame with columns infection_day and infections, containing
+#' the daily count of infections
+#'
+#' @importFrom dplyr transmute count full_join join_by
+#' @importFrom tidyr expand replace_na
+#'
+#' @export
+#'
+#' @examples
+#' make_daily_infections(infection_times)
+make_daily_infections <- function(infection_times) {
+ ## Censor infection times to days
+ df <- infection_times |>
+ transmute(infection_day = floor(infection_time))
+
+ ## Summarise infections as a time series by day
+ inf_ts <- df |>
+ count(infection_day, name = "infections")
+
+ ## fill data frame with zeroes for days without infection
+ all_days <- expand(
+ df, infection_day = seq(min(infection_day), max(infection_day))
+ )
+ inf_ts <- all_days |>
+ full_join(inf_ts, by = join_by(infection_day)) |>
+ replace_na(list(infections = 0))
+
+ return(inf_ts)
+}
diff --git a/R/nfidd-stan-tools.r b/R/nfidd-stan-tools.r
new file mode 100644
index 0000000..f1b048a
--- /dev/null
+++ b/R/nfidd-stan-tools.r
@@ -0,0 +1,304 @@
+#' Get the path to Stan code
+#'
+#' @return A character string with the path to the Stan code
+#'
+#' @family stantools
+#'
+#' @export
+nfidd_stan_path <- function() {
+ system.file("stan", package = "nfidd")
+}
+
+#' Count the number of unmatched braces in a line
+#' @noRd
+.unmatched_braces <- function(line) {
+ ifelse(
+ grepl("{", line, fixed = TRUE),
+ length(gregexpr("{", line, fixed = TRUE)), 0
+ ) -
+ ifelse(
+ grepl("}", line, fixed = TRUE),
+ length(gregexpr("}", line, fixed = TRUE)), 0
+ )
+}
+
+#' Extract function names or content from Stan code
+#'
+#' @param content Character vector containing Stan code
+#'
+#' @param names_only Logical, if TRUE extract function names, otherwise
+#' extract function content.
+#'
+#' @param functions Optional, character vector of function names to extract
+#' content for.
+#' @return Character vector of function names or content
+#' @keywords internal
+.extract_stan_functions <- function(
+ content, names_only = FALSE, functions = NULL) {
+ def_pattern <- "^(real|vector|matrix|void|int|array\\s*<\\s*(real|vector|matrix|int)\\s*>|tuple\\s*<\\s*.*\\s*>)\\s+" # nolint
+ func_pattern <- paste0(
+ def_pattern, "(\\w+)\\s*\\("
+ )
+ func_lines <- grep(func_pattern, content, value = TRUE)
+ # remove the func_pattern
+ func_lines <- sub(def_pattern, "", func_lines)
+ # get the next complete word after the pattern until the first (
+ func_names <- sub("\\s*\\(.*$", "", func_lines)
+ if (!is.null(functions)) {
+ func_names <- intersect(func_names, functions)
+ }
+ if (names_only) {
+ return(func_names)
+ } else {
+ func_content <- character(0)
+ for (func_name in func_names) {
+ start_line <- grep(paste0(def_pattern, func_name, "\\("), content)
+ if (length(start_line) == 0) next
+ end_line <- start_line
+ brace_count <- 0
+ # Ensure we find the first opening brace
+ repeat {
+ line <- content[end_line]
+ brace_count <- brace_count + .unmatched_braces(line)
+ end_line <- end_line + 1
+ if (brace_count > 0) break
+ }
+ # Continue until all braces are closed
+ repeat {
+ line <- content[end_line]
+ brace_count <- brace_count + .unmatched_braces(line)
+ if (brace_count == 0) break
+ end_line <- end_line + 1
+ }
+ func_content <- c(
+ func_content, paste(content[start_line:end_line], collapse = "\n")
+ )
+ }
+ return(func_content)
+ }
+}
+
+#' Get Stan function names from Stan files
+#'
+#' This function reads all Stan files in the specified directory and extracts
+#' the names of all functions defined in those files.
+#'
+#' @param stan_path Character string specifying the path to the directory
+#' containing Stan files. Defaults to the Stan path of the nfidd
+#' package.
+#'
+#' @return A character vector containing unique names of all functions found in
+#' the Stan files.
+#'
+#' @export
+#'
+#' @family stantools
+nfidd_stan_functions <- function(
+ stan_path = nfidd::nfidd_stan_path()) {
+ stan_files <- list.files(
+ file.path(stan_path, "functions"),
+ pattern = "\\.stan$", full.names = TRUE,
+ recursive = TRUE
+ )
+ functions <- character(0)
+ for (file in stan_files) {
+ content <- readLines(file)
+ functions <- c(
+ functions, .extract_stan_functions(content, names_only = TRUE)
+ )
+ }
+ unique(functions)
+}
+
+#' Get Stan files containing specified functions
+#'
+#' This function retrieves Stan files from a specified directory, optionally
+#' filtering for files that contain specific functions.
+#'
+#' @param functions Character vector of function names to search for. If NULL,
+#' all Stan files are returned.
+#' @inheritParams nfidd_stan_functions
+#'
+#' @return A character vector of file paths to Stan files.
+#'
+#' @export
+#'
+#' @family stantools
+nfidd_stan_function_files <- function(
+ functions = NULL,
+ stan_path = nfidd::nfidd_stan_path()) {
+ # List all Stan files in the directory
+ all_files <- list.files(
+ file.path(stan_path, "functions"),
+ pattern = "\\.stan$",
+ full.names = TRUE,
+ recursive = TRUE
+ )
+
+ if (is.null(functions)) {
+ return(all_files)
+ } else {
+ # Initialize an empty vector to store matching files
+ matching_files <- character(0)
+
+ for (file in all_files) {
+ content <- readLines(file)
+ extracted_functions <- .extract_stan_functions(content, names_only = TRUE)
+
+ if (any(functions %in% extracted_functions)) {
+ matching_files <- c(matching_files, file)
+ }
+ }
+
+ # remove the path from the file names
+ matching_files <- sub(
+ paste0(stan_path, "/"), "", matching_files
+ )
+ return(matching_files)
+ }
+}
+
+#' Load Stan functions as a string
+#'
+#' @param functions Character vector of function names to load. Defaults to all
+#' functions.
+#'
+#' @param stan_path Character string, the path to the Stan code. Defaults to the
+#' path to the Stan code in the nfidd package.
+#'
+#' @param wrap_in_block Logical, whether to wrap the functions in a
+#' `functions{}` block. Default is FALSE.
+#'
+#' @param write_to_file Logical, whether to write the output to a file. Default
+#' is FALSE.
+#'
+#' @param output_file Character string, the path to write the output file if
+#' write_to_file is TRUE. Defaults to "nfidd_functions.stan".
+#'
+#' @return A character string containing the requested Stan functions
+#'
+#' @family stantools
+#'
+#' @export
+nfidd_load_stan_functions <- function(
+ functions = NULL, stan_path = nfidd::nfidd_stan_path(),
+ wrap_in_block = FALSE, write_to_file = FALSE,
+ output_file = "nfidd_functions.stan") {
+ stan_files <- list.files(
+ file.path(stan_path, "functions"),
+ pattern = "\\.stan$", full.names = TRUE,
+ recursive = TRUE
+ )
+ all_content <- character(0)
+
+ for (file in stan_files) {
+ content <- readLines(file)
+ if (is.null(functions)) {
+ all_content <- c(all_content, content)
+ } else {
+ for (func in functions) {
+ func_content <- .extract_stan_functions(
+ content,
+ names_only = FALSE,
+ functions = func
+ )
+ all_content <- c(all_content, func_content)
+ }
+ }
+ }
+
+ # Add version comment
+ version_comment <- paste(
+ "// Stan functions from nfidd version",
+ utils::packageVersion("nfidd")
+ )
+ all_content <- c(version_comment, all_content)
+
+ if (wrap_in_block) {
+ all_content <- c("functions {", all_content, "}")
+ }
+
+ result <- paste(all_content, collapse = "\n")
+
+ if (write_to_file) {
+ writeLines(result, output_file)
+ message("Stan functions written to: ", output_file, "\n")
+ }
+
+ return(result)
+}
+
+#' List Available Stan Models in NFIDD
+#'
+#' This function finds all available Stan models in the NFIDD package and
+#' returns their names without the .stan extension.
+#'
+#' @param stan_path Character string specifying the path to Stan files. Defaults
+#' to the result of `nfidd_stan_path()`.
+#'
+#' @return A character vector of available Stan model names.
+#'
+#' @export
+#'
+#' @examples
+#' nfidd_list_stan_models()
+nfidd_stan_models <- function(
+ stan_path = nfidd::nfidd_stan_path()
+ ) {
+ stan_files <- list.files(
+ stan_path,
+ pattern = "\\.stan$", full.names = FALSE,
+ recursive = FALSE
+ )
+
+ # Remove .stan extension
+ model_names <- tools::file_path_sans_ext(stan_files)
+
+ return(model_names)
+}
+
+#' Create a CmdStanModel with NFIDD Stan functions
+#'
+#' This function creates a CmdStanModel object using a specified Stan model from
+#' the NFIDD package and optionally includes additional user-specified Stan
+#' files.
+#'
+#' @param model_name Character string specifying which Stan model to use.
+#' @param include_paths Character vector of paths to include for Stan
+#' compilation. Defaults to the result of `nfidd_stan_path()`.
+#' @param ... Additional arguments passed to cmdstanr::cmdstan_model().
+#'
+#' @return A CmdStanModel object.
+#'
+#' @export
+#'
+#' @family modelhelpers
+#'
+#' @examplesIf requireNamespace("cmdstanr", quietly = TRUE)
+#' if (!is.null(cmdstanr::cmdstan_version(error_on_NA = FALSE))) {
+#' model <- nfidd_cmdstan_model("simple-nowcast", compile = FALSE)
+#' model
+#' }
+nfidd_cmdstan_model <- function(
+ model_name,
+ include_paths = nfidd::nfidd_stan_path(),
+ ...) {
+ if (!requireNamespace("cmdstanr", quietly = TRUE)) {
+ stop("Package 'cmdstanr' is required but not installed for this function.")
+ }
+
+ stan_model <- system.file(
+ "stan", paste0(model_name, ".stan"),
+ package = "nfidd"
+ )
+
+ if (stan_model == "") {
+ stop(sprintf("Model '%s.stan' not found in NFIDD package", model_name))
+ }
+
+ cmdstanr::cmdstan_model(
+ stan_model,
+ include_paths = include_paths,
+ ...
+ )
+}
\ No newline at end of file
diff --git a/R/renewal.r b/R/renewal.r
new file mode 100644
index 0000000..bc29f89
--- /dev/null
+++ b/R/renewal.r
@@ -0,0 +1,40 @@
+#' Simulate Infections using the Renewal Equation
+#'
+#' This function simulates infections using the renewal equation.
+#'
+#' @param I0 The initial number of infections.
+#' @param R The reproduction number, given as a vector with one entry per time
+#' point.
+#' @param gen_time The generation time distribution, given as a vector with one
+#' entry per day after infection (the first element corresponding to one day
+#' after infection).
+#'
+#' @return A vector of simulated infections over time.
+#'
+#' @export
+#'
+#' @examples
+#' renewal(
+#' I0 = 5,
+#' R = c(rep(3, 4), rep(0.5, 5)),
+#' gen_time = c(0.1, 0.2, 0.3, 0.2, 0.1)
+#' )
+renewal <- function(I0, R, gen_time) {
+ ## set the maximum generation time
+ max_gen_time <- length(gen_time)
+ ## number of time points
+ times <- length(R)
+ I <- c(I0, rep(0, times)) ## set up vector holding number of infected
+ ## iterate over time points
+ for (t in 1:times) {
+ ## calculate convolution
+ first_index <- max(1, t - max_gen_time + 1)
+ I_segment <- I[seq(first_index, t)]
+ ## iterate over generation times
+ ## take reverse of pmf and reverse if needed
+ gen_pmf <- rev(gen_time[seq_len(t - first_index + 1)])
+ ## convolve infections with generation time
+ I[t + 1] <- sum(I_segment * gen_pmf) * R[t]
+ }
+ return(I[-1]) ## remove I0 from time series
+}
diff --git a/R/simulate_onsets.r b/R/simulate_onsets.r
new file mode 100644
index 0000000..9f715db
--- /dev/null
+++ b/R/simulate_onsets.r
@@ -0,0 +1,70 @@
+#' Generate a probability mass function for the generation time
+#'
+#' @param max Maximum delay to consider
+#' @param shape Shape parameter for the gamma distribution
+#' @param rate Rate parameter for the gamma distribution
+#'
+#' @return A vector of probabilities representing the generation time PMF
+#'
+#' @export
+#'
+#' @importFrom stats rgamma
+make_gen_time_pmf <- function(max = 14, shape = 4, rate = 1) {
+ pmf <- censored_delay_pmf(rgamma, max = max, shape = shape, rate = rate)
+ pmf <- pmf[-1] # remove first element
+ pmf <- pmf / sum(pmf) # renormalise
+ return(pmf)
+}
+
+#' Generate a probability mass function for the incubation period
+#'
+#' @param max Maximum delay to consider
+#' @param shape Shape parameter for the gamma distribution
+#' @param rate Rate parameter for the gamma distribution
+#'
+#' @return A vector of probabilities representing the incubation period PMF
+#'
+#' @export
+#'
+#' @importFrom stats rgamma
+make_ip_pmf <- function(max = 14, shape = 5, rate = 1) {
+ censored_delay_pmf(rgamma, max = max, shape = shape, rate = rate)
+}
+
+#' Simulate symptom onsets from daily infection counts
+#'
+#' @param inf_ts A data frame containing columns infection_day and infections,
+#' as returned by `make_daily_infections()`.
+#'
+#' @param gen_time_pmf A vector of probabilities representing the generation
+#' time PMF, as returned by `make_gen_time_pmf()`.
+#'
+#' @param ip_pmf A vector of probabilities representing the incubation period
+#' PMF, as returned by `make_ip_pmf()`.
+#'
+#' @return A data frame with columns day, onsets, and infections containing
+#' the daily count of symptom onsets and infections
+#'
+#' @importFrom stats rgamma rpois
+#' @importFrom dplyr tibble left_join select
+#' @importFrom tidyr replace_na
+#'
+#' @export
+#'
+#' @examples
+#' gt_pmf <- make_gen_time_pmf()
+#' ip_pmf <- make_ip_pmf()
+#' simulate_onsets(make_daily_infections(infection_times), gt_pmf, ip_pmf)
+simulate_onsets <- function(inf_ts, gen_time_pmf = make_gen_time_pmf(),
+ ip_pmf = make_ip_pmf()) {
+ onsets <- convolve_with_delay(inf_ts$infections, ip_pmf)
+ onsets <- rpois(n = length(onsets), lambda = onsets)
+ onset_df <- tibble(day = seq_along(onsets), onsets = onsets) |>
+ left_join(
+ inf_ts |> select(day = infection_day, infections),
+ by = "day"
+ ) |>
+ replace_na(list(infections = 0))
+
+ return(onset_df)
+}
diff --git a/README.Rmd b/README.Rmd
new file mode 100644
index 0000000..890dbc0
--- /dev/null
+++ b/README.Rmd
@@ -0,0 +1,73 @@
+---
+output: github_document
+---
+
+# Nowcasting and forecasting infectious disease dynamics
+
+This repository contains the material to create the nfidd course page.
+
+All the raw material is in the folder `sessions/` and is written in
+`quarto`. Any changes to the quarto files are automatically
+updated on the web site once committed to the `main` branch.
+
+To add a lesson, add a `.qmd` file in the `sessions` folder with a YAML field `order:` corresponding to where it fits in, and edit `sessions.qmd` to add it to the schedule.
+
+## Local testing
+
+The `html` pages can be generated locally using the function
+
+```{r render, eval = FALSE}
+quarto::quarto_render()
+```
+
+## Contributors
+
+
+
+
+
+
+
+
+
+
+
+
+
+
+All contributions to this project are gratefully acknowledged using the [`allcontributors` package](https://github.com/ropenscilabs/allcontributors) following the [all-contributors](https://allcontributors.org) specification. Contributions of any kind are welcome!
+
+### Code
+
+
+sbfnk,
+seabbs,
+kathsherratt,
+github-actions[bot],
+dependabot[bot],
+jamesmbaazam,
+ManuelStapper,
+zsusswein
+
+
+
+### Issues
+
+
+toshiakiasakura,
+jcken95
+
+
+
+
+
+
+
+
+
+
+
+
+
+
+
diff --git a/README.md b/README.md
new file mode 100644
index 0000000..1f351e3
--- /dev/null
+++ b/README.md
@@ -0,0 +1,52 @@
+
+# Nowcasting and forecasting infectious disease dynamics
+
+This repository contains the material to create the nfidd course page.
+
+All the raw material is in the folder `sessions/` and is written in
+`quarto`. Any changes to the quarto files are automatically updated on
+the web site once committed to the `main` branch.
+
+To add a lesson, add a `.qmd` file in the `sessions` folder with a YAML
+field `order:` corresponding to where it fits in, and edit
+`sessions.qmd` to add it to the schedule.
+
+## Local testing
+
+The `html` pages can be generated locally using the function
+
+``` r
+quarto::quarto_render()
+```
+
+## Contributors
+
+
+
+
+
+All contributions to this project are gratefully acknowledged using the
+[`allcontributors`
+package](https://github.com/ropenscilabs/allcontributors) following the
+[all-contributors](https://allcontributors.org) specification.
+Contributions of any kind are welcome!
+
+### Code
+
+sbfnk,
+seabbs,
+kathsherratt,
+github-actions\[bot\],
+dependabot\[bot\],
+jamesmbaazam,
+ManuelStapper,
+zsusswein
+
+### Issues
+
+toshiakiasakura,
+jcken95
+
+
+
+
diff --git a/_data/elsevier-harvard.csl b/_data/elsevier-harvard.csl
new file mode 100644
index 0000000..afcfaef
--- /dev/null
+++ b/_data/elsevier-harvard.csl
@@ -0,0 +1,237 @@
+
+
diff --git a/_data/papers.bib b/_data/papers.bib
new file mode 100644
index 0000000..e69de29
diff --git a/_quarto.yml b/_quarto.yml
new file mode 100644
index 0000000..a998455
--- /dev/null
+++ b/_quarto.yml
@@ -0,0 +1,40 @@
+project:
+ type: website
+ render:
+ - "*.qmd"
+
+website:
+ title: "NFIDD"
+ site-url: https://nfidd.github.io/nfidd/
+ description: "Nowcasting and forecasting infectious disease dynamics"
+ body-footer: ''
+ open-graph: true
+ navbar:
+ left:
+ - sidebar:sessions
+ - text: "Learning objectives"
+ href: learning_objectives.qmd
+ - text: "Getting set up for the course"
+ href: getting-set-up.qmd
+ - text: "Getting help"
+ href: help.qmd
+ - text: "Timetable"
+ href: sessions.qmd
+ right:
+ - icon: github
+ href: https://github.com/nfidd/nfidd
+ sidebar:
+ - id: sessions
+ title: "Sessions"
+ style: "docked"
+ contents: "sessions/*.qmd"
+
+format:
+ html:
+ theme:
+ light: cosmo
+ dark: solar
+ css: styles.css
+ link-external-icon: true
+ link-external-newwindow: true
+ toc: true
diff --git a/blank.html b/blank.html
new file mode 100644
index 0000000..ec18481
--- /dev/null
+++ b/blank.html
@@ -0,0 +1,10 @@
+
diff --git a/data-raw/epicurve.r b/data-raw/epicurve.r
new file mode 100644
index 0000000..329fe41
--- /dev/null
+++ b/data-raw/epicurve.r
@@ -0,0 +1,14 @@
+library("epichains")
+library("usethis")
+library("dplyr")
+library("ggplot2")
+
+set.seed(12345)
+infection_times <- simulate_chains(
+ index_cases = 1, offspring_dist = rpois, statistic = "size", lambda = 1.5,
+ pop = 10000, generation_time = function(n) rgamma(n, shape = 4)
+) |>
+ select(infection_time = time) |>
+ as.data.frame()
+
+usethis::use_data(infection_times, overwrite = TRUE)
diff --git a/data-raw/generate-example-forecasts.r b/data-raw/generate-example-forecasts.r
new file mode 100644
index 0000000..9c35a72
--- /dev/null
+++ b/data-raw/generate-example-forecasts.r
@@ -0,0 +1,120 @@
+library("dplyr")
+library("tidyr")
+library("cmdstanr")
+library("tidybayes")
+library("purrr")
+library("usethis")
+library("nfidd")
+
+set.seed(12345)
+
+# simulate data
+gen_time_pmf <- make_gen_time_pmf()
+ip_pmf <- make_ip_pmf()
+onset_df <- simulate_onsets(
+ make_daily_infections(infection_times), gen_time_pmf, ip_pmf
+)
+
+# define a function to fit and forecast for a single date
+forecast_target_day <- function(
+ mod, onset_df, target_day, horizon, gen_time_pmf, ip_pmf, data_to_list, ...
+) {
+
+ message("Fitting and forecasting for target day: ", target_day)
+
+ train_df <- onset_df |>
+ filter(day <= target_day)
+
+ test_df <- onset_df |>
+ filter(day > target_day, day <= target_day + horizon)
+
+ data <- data_to_list(train_df, horizon, gen_time_pmf, ip_pmf)
+
+ fit <- mod$sample(
+ data = data, chains = 4, parallel_chains = 4, adapt_delta = 0.95,
+ max_treedepth = 15, iter_warmup = 1000, iter_sampling = 500, ...)
+
+ forecast <- fit |>
+ gather_draws(forecast[day]) |>
+ filter(!is.na(.value)) |>
+ slice_head(n = 1000) |>
+ mutate(horizon = day) |>
+ mutate(day = day + target_day) |>
+ mutate(target_day = target_day) |>
+ mutate(.draw = 1:n()) |>
+ select(-.chain, -.iteration)
+ return(forecast)
+}
+
+# define a forecast horizon
+horizon <- 14
+
+target_days <- onset_df |>
+ filter(day <= max(day) - horizon) |>
+ filter(day > 21) |>
+ pull(day)
+
+# create forecasts every 7 days
+target_days <- target_days[seq(1, length(target_days), 7)]
+
+# load the model
+rw_mod <- nfidd_cmdstan_model("estimate-inf-and-r-rw-forecast")
+
+data_to_list_rw <- function(train_df, horizon, gen_time_pmf, ip_pmf) {
+ data <- list(
+ n = nrow(train_df),
+ I0 = 1,
+ obs = train_df$onsets,
+ gen_time_max = length(gen_time_pmf),
+ gen_time_pmf = gen_time_pmf,
+ ip_max = length(ip_pmf) - 1,
+ ip_pmf = ip_pmf,
+ h = horizon
+ )
+ return(data)
+}
+
+rw_forecasts <- target_days |>
+ map_dfr(
+ \(x) forecast_target_day(
+ rw_mod, onset_df, x, horizon, gen_time_pmf, ip_pmf,
+ data_to_list_rw, init = \() list(init_R = 0, rw_sd = 0.01)
+ )
+ ) |>
+ mutate(model = "Random walk")
+
+usethis::use_data(rw_forecasts, overwrite = TRUE)
+
+# AR model forecass
+stat_mod <- nfidd_cmdstan_model("statistical-r")
+
+stat_forecasts <- target_days |>
+ map_dfr(
+ \(x) forecast_target_day(
+ stat_mod, onset_df, x, horizon, gen_time_pmf, ip_pmf, data_to_list_rw,
+ init = \() list(init_R = 0, rw_sd = 0.01)
+ )
+ ) |>
+ mutate(model = "Statistical")
+
+usethis::use_data(stat_forecasts, overwrite = TRUE)
+
+# Mechanistic model forecasts
+
+mech_mod <- nfidd_cmdstan_model("mechanistic-r")
+
+data_to_list_mech <- function(train_df, horizon, gen_time_pmf, ip_pmf) {
+ data <- data_to_list_rw(train_df, horizon, gen_time_pmf, ip_pmf)
+ data$N_prior <- c(10000, 2000)
+ return(data)
+}
+
+mech_forecasts <- target_days |>
+ map_dfr(
+ \(x) forecast_target_day(
+ mech_mod, onset_df, x, horizon, gen_time_pmf, ip_pmf, data_to_list_mech
+ )
+ ) |>
+ mutate(model = "Mechanistic")
+
+usethis::use_data(mech_forecasts, overwrite = TRUE)
diff --git a/data/infection_times.rda b/data/infection_times.rda
new file mode 100644
index 0000000..a4d31c5
Binary files /dev/null and b/data/infection_times.rda differ
diff --git a/data/mech_forecasts.rda b/data/mech_forecasts.rda
new file mode 100644
index 0000000..6e44a5e
Binary files /dev/null and b/data/mech_forecasts.rda differ
diff --git a/data/rw_forecasts.rda b/data/rw_forecasts.rda
new file mode 100644
index 0000000..feb7b99
Binary files /dev/null and b/data/rw_forecasts.rda differ
diff --git a/data/stat_forecasts.rda b/data/stat_forecasts.rda
new file mode 100644
index 0000000..1d83e5b
Binary files /dev/null and b/data/stat_forecasts.rda differ
diff --git a/footer.html b/footer.html
new file mode 100644
index 0000000..01d2ee9
--- /dev/null
+++ b/footer.html
@@ -0,0 +1,20 @@
+
+
+
+
diff --git a/getting-set-up.qmd b/getting-set-up.qmd
new file mode 100644
index 0000000..7d86e39
--- /dev/null
+++ b/getting-set-up.qmd
@@ -0,0 +1,84 @@
+Each session in this course uses R code for demonstration.
+All the content is self-contained within a software package designed for the course.
+To get the most out of this course, you will need to use R and the following instructions to interact with the course material.
+
+# Getting R
+
+- [R](http://cran.r-project.org) is used as the main programming language. Please install at least version: R-4.2.0.
+- [RStudio](http://www.rstudio.com/products/rstudio/download/) is a popular graphic user interface (GUI). Its Visual Editor provides the best experience of going through this course. Please make sure you update RStudio to the latest version.
+
+# Installing additional requirements
+
+Before you get started with the course, you will first need to install the following software.
+
+## Installation of the `nfidd` package
+
+To install the packages needed in the course, including the `nfidd` package that contains data files and helper functions used throughout, you can use the `pak` package:
+
+```{r install, eval=FALSE}
+install.packages("pak")
+pak::pak("nfidd/nfidd", dependencies = "all", upgrade = TRUE)
+```
+
+Then you can check that the installation completed successfully by loading the package into your **R** session:
+
+```{r load, eval=FALSE}
+library("nfidd")
+```
+
+## Installing `cmdstan`
+
+The course relies on running stan through the `cmdstanr` **R** package, which itself uses the `cmdstan` software.
+This requires a separate installation step:
+
+```{r cmdstan_install, eval = FALSE}
+cmdstanr::install_cmdstan()
+```
+
+::: callout-note
+This may take a few minutes.
+Also you're likely to see lots of warnings and other messages printed to your screen - don't worry, this is normal and doesn't mean there is a problem.
+:::
+
+If there are any problems with this, you can try (on Windows) to fix them using
+
+```{r cmdstan_toolchain, eval = FALSE}
+cmdstanr::check_cmdstan_toolchain(fix = TRUE)
+```
+
+You can test that you have a working `cmdstanr` setup using
+
+```{r cmdstan_test}
+cmdstanr::cmdstan_version()
+```
+
+For more details, and for links to resources in case something goes wrong, see the [Getting Started with CmdStanr](https://mc-stan.org/cmdstanr/articles/cmdstanr.html) vignette of the package.
+
+# Accessing the course
+
+To be able to use the code in each session, you will need a local copy of the course material.
+
+- Directly download the course material:
+
+ ::: callout-tip
+ [[**Download**]{.underline}](https://github.com/nfidd/nfidd/archive/refs/heads/main.zip)
+ :::
+
+- Alternatively, if you are familiar with git you can clone the [repo](https://github.com/nfidd/nfidd).
+
+### Interacting with the course
+
+In this course, all content is written using [R Notebooks](https://bookdown.org/yihui/rmarkdown/notebook.html).
+This means that we can combine text with code and see the output directly.
+The notebooks are then directly reproduced on the course website (for example, this page).
+
+To interact with each session in the course, we recommend opening the RStudio Project file (`nfidd.RProj`) in the `nfidd` folder you have just downloaded.
+Then you can choose to:
+
+- View each session on the website, and copy-paste the code into your own R script.
+ - Tip: if you hover over each code chunk on the website you can use a "Copy" button at the top right corner.
+- Open the R Notebook for each session.
+ - Each notebook is saved in `nfidd/sessions/` as a `.qmd` file.
+ - Execute code with the single green "play" button at the top-right corner of each code chunk ("Run current chunk"). You can also execute code line-by-line using `Ctrl/Cmd + Enter`.
+ - We suggest using "Visual" view for a better experience (top-left of the RStudio pane).
+
diff --git a/header.html b/header.html
new file mode 100644
index 0000000..b1c7fac
--- /dev/null
+++ b/header.html
@@ -0,0 +1,4 @@
+
+
+
diff --git a/help.qmd b/help.qmd
new file mode 100644
index 0000000..30b285f
--- /dev/null
+++ b/help.qmd
@@ -0,0 +1,7 @@
+---
+title: "Getting help"
+comments: false
+---
+
+For any questions about the course or its content, feel free to use the Discussion
+ board
diff --git a/index.qmd b/index.qmd
new file mode 100644
index 0000000..d1bed31
--- /dev/null
+++ b/index.qmd
@@ -0,0 +1,24 @@
+---
+title: "Nowcasting and forecasting infectious disease dynamics"
+site: distill::distill_website
+comments: false
+about:
+ template: jolla
+ links:
+ - icon: chat-fill
+ text: Dive into the course
+ href: nfidd/sessions/introduction-and-course-overview.html
+ - icon: github
+ text: Visit us on GitHub
+ href: https://github.com/nfidd/nfidd
+---
+
+```{r setup, include=FALSE}
+knitr::opts_chunk$set(echo = FALSE)
+```
+
+A course and living resource for learning about nowcasting and forecasting infectious disease dynamics.
+
+We invite anyone to use the materials here in their own teaching and learning. For questions and discussion of the course or its content, we welcome all users to the [Discussion board](https://github.com/nfidd/nfidd/discussions). We welcome all forms of [contributions, additions and suggestions](https://github.com/nfidd/nfidd/issues) for improving the materials.
+
+All materials here are provided under the permissive [MIT License](https://github.com/nfidd/nfidd/blob/main/LICENSE).
diff --git a/inst/stan/censored-delay-model.stan b/inst/stan/censored-delay-model.stan
new file mode 100644
index 0000000..acebd23
--- /dev/null
+++ b/inst/stan/censored-delay-model.stan
@@ -0,0 +1,28 @@
+data {
+ int n;
+ array[n] int onset_to_hosp;
+}
+
+parameters {
+ real meanlog;
+ real sdlog;
+ array[n] real onset_day_time;
+ array[n] real hosp_day_time;
+}
+
+transformed parameters {
+ array[n] real true_onset_to_hosp;
+ for (i in 1:n) {
+ true_onset_to_hosp[i] =
+ onset_to_hosp[i] + hosp_day_time[i] - onset_day_time[i];
+ }
+}
+
+model {
+ meanlog ~ normal(0, 10);
+ sdlog ~ normal(0, 10) T[0, ];
+ onset_day_time ~ uniform(0, 1);
+ hosp_day_time ~ uniform(0, 1);
+
+ true_onset_to_hosp ~ lognormal(meanlog, sdlog);
+}
diff --git a/inst/stan/coin.stan b/inst/stan/coin.stan
new file mode 100644
index 0000000..bfde5e3
--- /dev/null
+++ b/inst/stan/coin.stan
@@ -0,0 +1,15 @@
+data {
+ int N; // integer, minimum 1
+ int x; // integer, minimum 0
+}
+
+parameters {
+ real theta; // real, between 0 and 1
+}
+
+model {
+ // Uniform prior
+ theta ~ uniform(0, 1);
+ // Binomial likelihood
+ x ~ binomial(N, theta);
+}
diff --git a/inst/stan/estimate-inf-and-r-rw-forecast.stan b/inst/stan/estimate-inf-and-r-rw-forecast.stan
new file mode 100644
index 0000000..66fbf3f
--- /dev/null
+++ b/inst/stan/estimate-inf-and-r-rw-forecast.stan
@@ -0,0 +1,49 @@
+functions {
+ #include "functions/convolve_with_delay.stan"
+ #include "functions/renewal.stan"
+ #include "functions/geometric_random_walk.stan"
+}
+
+data {
+ int n; // number of days
+ int I0; // number initially infected
+ array[n] int obs; // observed symptom onsets
+ int gen_time_max; // maximum generation time
+ array[gen_time_max] real gen_time_pmf; // pmf of generation time distribution
+ int ip_max; // max incubation period
+ array[ip_max + 1] real ip_pmf;
+ int h; // number of days to forecast
+}
+
+transformed data {
+ int m = n + h;
+}
+
+parameters {
+ real init_R; // initial reproduction number
+ array[m-1] real rw_noise; // random walk noise
+ real rw_sd; // random walk standard deviation
+}
+
+transformed parameters {
+ array[m] real R = geometric_random_walk(init_R, rw_noise, rw_sd);
+ array[m] real infections = renewal(I0, R, gen_time_pmf);
+ array[m] real onsets = convolve_with_delay(infections, ip_pmf);
+}
+
+model {
+ // priors
+ init_R ~ normal(-.1, 0.5); // Approximately Normal(1, 0.5)
+ rw_noise ~ std_normal();
+ rw_sd ~ normal(0, 0.05) T[0,];
+ obs ~ poisson(onsets[1:n]);
+}
+
+generated quantities {
+ array[h] real forecast;
+ if (h > 0) {
+ for (i in 1:h) {
+ forecast[i] = poisson_rng(onsets[n + i]);
+ }
+ }
+}
diff --git a/inst/stan/estimate-inf-and-r-rw.stan b/inst/stan/estimate-inf-and-r-rw.stan
new file mode 100644
index 0000000..8db6868
--- /dev/null
+++ b/inst/stan/estimate-inf-and-r-rw.stan
@@ -0,0 +1,35 @@
+functions {
+ #include "functions/convolve_with_delay.stan"
+ #include "functions/renewal.stan"
+ #include "functions/geometric_random_walk.stan"
+}
+
+data {
+ int n; // number of days
+ int I0; // number initially infected
+ array[n] int obs; // observed symptom onsets
+ int gen_time_max; // maximum generation time
+ array[gen_time_max] real gen_time_pmf; // pmf of generation time distribution
+ int ip_max; // max incubation period
+ array[ip_max + 1] real ip_pmf;
+}
+
+parameters {
+ real init_R; // initial reproduction number
+ array[n-1] real rw_noise; // random walk noise
+ real rw_sd; // random walk standard deviation
+}
+
+transformed parameters {
+ array[n] real R = geometric_random_walk(init_R, rw_noise, rw_sd);
+ array[n] real infections = renewal(I0, R, gen_time_pmf);
+ array[n] real onsets = convolve_with_delay(infections, ip_pmf);
+}
+
+model {
+ // priors
+ init_R ~ normal(-.1, 0.5); // Approximately Normal(1, 0.5)
+ rw_noise ~ std_normal();
+ rw_sd ~ normal(0, 0.05) T[0,];
+ obs ~ poisson(onsets);
+}
diff --git a/inst/stan/estimate-inf-and-r.stan b/inst/stan/estimate-inf-and-r.stan
new file mode 100644
index 0000000..0691cd0
--- /dev/null
+++ b/inst/stan/estimate-inf-and-r.stan
@@ -0,0 +1,29 @@
+functions {
+ #include "functions/convolve_with_delay.stan"
+ #include "functions/renewal.stan"
+}
+
+data {
+ int n; // number of days
+ int I0; // number initially infected
+ array[n] int obs; // observed symptom onsets
+ int gen_time_max; // maximum generation time
+ array[gen_time_max] real gen_time_pmf; // pmf of generation time distribution
+ int ip_max; // max incubation period
+ array[ip_max + 1] real ip_pmf;
+}
+
+parameters {
+ array[n] real R;
+}
+
+transformed parameters {
+ array[n] real infections = renewal(I0, R, gen_time_pmf);
+ array[n] real onsets = convolve_with_delay(infections, ip_pmf);
+}
+
+model {
+ // priors
+ R ~ normal(1, 1) T[0, ];
+ obs ~ poisson(onsets);
+}
diff --git a/inst/stan/estimate-infections.stan b/inst/stan/estimate-infections.stan
new file mode 100644
index 0000000..11fd78f
--- /dev/null
+++ b/inst/stan/estimate-infections.stan
@@ -0,0 +1,25 @@
+functions {
+ #include "functions/convolve_with_delay.stan"
+}
+
+data {
+ int n; // number of time days
+ array[n] int obs; // observed onsets
+ int ip_max; // max incubation period
+ // probability mass function of incubation period distribution (first index zero)
+ array[ip_max + 1] real ip_pmf;
+}
+
+parameters {
+ array[n] real infections;
+}
+
+transformed parameters {
+ array[n] real onsets = convolve_with_delay(infections, ip_pmf);
+}
+
+model {
+ // priors
+ infections ~ normal(0, 10) T[0, ];
+ obs ~ poisson(onsets);
+}
diff --git a/inst/stan/estimate-r.stan b/inst/stan/estimate-r.stan
new file mode 100644
index 0000000..f576326
--- /dev/null
+++ b/inst/stan/estimate-r.stan
@@ -0,0 +1,25 @@
+functions {
+ #include "functions/renewal.stan"
+}
+
+data {
+ int n; // number of days
+ int I0; // number initially infected
+ array[n] int obs; // observed infections
+ int gen_time_max; // maximum generation time
+ array[gen_time_max] real gen_time_pmf; // pmf of generation time distribution
+}
+
+parameters {
+ array[n] real R;
+}
+
+transformed parameters {
+ array[n] real infections = renewal(I0, R, gen_time_pmf);
+}
+
+model {
+ // priors
+ R ~ normal(1, 1) T[0, ];
+ obs ~ poisson(infections);
+}
diff --git a/inst/stan/functions/combine_obs_with_predicted_obs_rng.stan b/inst/stan/functions/combine_obs_with_predicted_obs_rng.stan
new file mode 100644
index 0000000..7ae78be
--- /dev/null
+++ b/inst/stan/functions/combine_obs_with_predicted_obs_rng.stan
@@ -0,0 +1,25 @@
+array[] int combine_obs_with_predicted_obs_rng(array[] int obs, array[] real complete_onsets_by_report, array[] int P, array[] int p, int d, array[] int D) {
+ int n = num_elements(p);
+ array[n] int reported_onsets = rep_array(0, n);
+ for (i in 1:n) {
+ int missing_reports = d - p[i];
+ int P_index = 0;
+ int D_index = 0;
+ if (i != 1) {
+ P_index = P[i - 1];
+ D_index = D[i - 1];
+ }
+ if (missing_reports != d) {
+ for (j in 1:p[i]) {
+ reported_onsets[i] += obs[P_index + j];
+ }
+ }
+ if (missing_reports > 0) {
+ for (j in 1:missing_reports) {
+ reported_onsets[i] += poisson_rng(complete_onsets_by_report[D_index + p[i] + j]);
+ }
+ }
+ }
+ return reported_onsets;
+ }
+
\ No newline at end of file
diff --git a/inst/stan/functions/condition_onsets_by_report.stan b/inst/stan/functions/condition_onsets_by_report.stan
new file mode 100644
index 0000000..4713a4d
--- /dev/null
+++ b/inst/stan/functions/condition_onsets_by_report.stan
@@ -0,0 +1,13 @@
+array[] real condition_onsets_by_report(array[] real onsets, array[] real delay) {
+ // length of time series
+ int n = num_elements(onsets);
+ // length of delay
+ int p = num_elements(delay);
+
+ array[n] real onsets_with_delay = onsets;
+
+ for (i in 1:min(p, n)) {
+ onsets_with_delay[n - i + 1] *= delay[i];
+ }
+ return onsets_with_delay;
+}
\ No newline at end of file
diff --git a/inst/stan/functions/convolve_with_delay.stan b/inst/stan/functions/convolve_with_delay.stan
new file mode 100644
index 0000000..5599b5a
--- /dev/null
+++ b/inst/stan/functions/convolve_with_delay.stan
@@ -0,0 +1,14 @@
+array[] real convolve_with_delay(array[] real ts, array[] real delay) {
+ // length of time series
+ int n = num_elements(ts);
+ int max_delay = num_elements(delay) - 1;
+ array[n] real ret;
+ for (i in 1:n) {
+ int first_index = max(1, i - max_delay);
+ int len = i - first_index + 1;
+ array[len] real pmf = reverse(delay)[1:len];
+ array[i - first_index + 1] real ts_segment = ts[first_index:i];
+ ret[i] = dot_product(ts_segment, pmf);
+ }
+ return(ret);
+}
diff --git a/inst/stan/functions/geometric_diff_ar.stan b/inst/stan/functions/geometric_diff_ar.stan
new file mode 100644
index 0000000..7ad449e
--- /dev/null
+++ b/inst/stan/functions/geometric_diff_ar.stan
@@ -0,0 +1,10 @@
+array[] real geometric_diff_ar(real init, array[] real noise, real std, real damp) {
+ int n = num_elements(noise) + 1;
+ array[n] real x;
+ x[1] = init;
+ x[2] = init + noise[1] * std;
+ for (i in 3:n) {
+ x[i] = x[i - 1] + damp * (x[i -1] - x[i- 2]) + noise[i - 1] * std;
+ }
+ return exp(x);
+}
diff --git a/inst/stan/functions/geometric_random_walk.stan b/inst/stan/functions/geometric_random_walk.stan
new file mode 100644
index 0000000..3ae4d84
--- /dev/null
+++ b/inst/stan/functions/geometric_random_walk.stan
@@ -0,0 +1,9 @@
+array[] real geometric_random_walk(real init, array[] real noise, real std) {
+ int n = num_elements(noise) + 1;
+ array[n] real x;
+ x[1] = init;
+ for (i in 2:n) {
+ x[i] = x[i-1] + noise[i-1] * std;
+ }
+ return exp(x);
+}
diff --git a/inst/stan/functions/observe_onsets_with_delay.stan b/inst/stan/functions/observe_onsets_with_delay.stan
new file mode 100644
index 0000000..5d7b7a5
--- /dev/null
+++ b/inst/stan/functions/observe_onsets_with_delay.stan
@@ -0,0 +1,17 @@
+array[] real observe_onsets_with_delay(array[] real onsets, vector reporting_delay, array[] int P, array[] int p) {
+ int n = num_elements(onsets);
+ int m = sum(p);
+ array[m] real onsets_by_report;
+ for (i in 1:n) {
+ int obs_index;
+ if (i == 1) {
+ obs_index = 0;
+ } else{
+ obs_index = P[i - 1];
+ }
+ for (j in 1:p[i]) {
+ onsets_by_report[obs_index + j] = onsets[i] * reporting_delay[j];
+ }
+ }
+ return onsets_by_report;
+}
diff --git a/inst/stan/functions/pop_bounded_renewal.stan b/inst/stan/functions/pop_bounded_renewal.stan
new file mode 100644
index 0000000..92c18ee
--- /dev/null
+++ b/inst/stan/functions/pop_bounded_renewal.stan
@@ -0,0 +1,16 @@
+array[] real pop_bounded_renewal(real I0, real R, array[] real gen_time, real N, int n) {
+ int max_gen_time = num_elements(gen_time);
+ array[n + 1] real I;
+ I[1] = I0;
+ real S = N - I0;
+
+ for (i in 1:n) {
+ int first_index = max(1, i - max_gen_time + 1);
+ int len = i - first_index + 1;
+ array[len] real I_segment = I[first_index:i];
+ array[len] real gen_pmf = reverse(gen_time[1:len]);
+ I[i + 1] = S / N * dot_product(I_segment, gen_pmf) * R;
+ S = S - I[i + 1];
+ }
+ return(I[2:(n + 1)]);
+}
diff --git a/inst/stan/functions/renewal.stan b/inst/stan/functions/renewal.stan
new file mode 100644
index 0000000..c1ff7ce
--- /dev/null
+++ b/inst/stan/functions/renewal.stan
@@ -0,0 +1,15 @@
+array[] real renewal(real I0, array[] real R, array[] real gen_time) {
+ // length of time series
+ int n = num_elements(R);
+ int max_gen_time = num_elements(gen_time);
+ array[n + 1] real I;
+ I[1] = I0;
+ for (i in 1:n) {
+ int first_index = max(1, i - max_gen_time + 1);
+ int len = i - first_index + 1;
+ array[len] real I_segment = I[first_index:i];
+ array[len] real gen_pmf = reverse(gen_time[1:len]);
+ I[i + 1] = dot_product(I_segment, gen_pmf) * R[i];
+ }
+ return(I[2:(n + 1)]);
+}
diff --git a/inst/stan/gamma.stan b/inst/stan/gamma.stan
new file mode 100644
index 0000000..6acd54f
--- /dev/null
+++ b/inst/stan/gamma.stan
@@ -0,0 +1,16 @@
+// gamma_model.stan
+data {
+ int N;
+ array[N] real y;
+}
+
+parameters {
+ real alpha;
+ real beta;
+}
+
+model {
+ alpha ~ normal(0, 10) T[0,];
+ beta ~ normal(0, 10) T[0,];
+ y ~ gamma(alpha, beta);
+}
diff --git a/inst/stan/joint-nowcast-with-r.stan b/inst/stan/joint-nowcast-with-r.stan
new file mode 100644
index 0000000..7dd11b3
--- /dev/null
+++ b/inst/stan/joint-nowcast-with-r.stan
@@ -0,0 +1,56 @@
+functions {
+ #include "functions/geometric_random_walk.stan"
+ #include "functions/renewal.stan"
+ #include "functions/convolve_with_delay.stan"
+ #include "functions/observe_onsets_with_delay.stan"
+ #include "functions/combine_obs_with_predicted_obs_rng.stan"
+}
+
+data {
+ int n; // number of days
+ int m; // number of reports
+ array[n] int p; // number of observations per day
+ array[m] int obs; // observed symptom onsets
+ int d; // number of reporting delays
+ int gen_time_max; // maximum generation time
+ array[gen_time_max] real gen_time_pmf; // pmf of generation time distribution
+ int ip_max; // max incubation period
+ array[ip_max + 1] real ip_pmf;
+}
+
+transformed data{
+ array[n] int P = to_int(cumulative_sum(p));
+ array[n] int D = to_int(cumulative_sum(rep_array(d, n)));
+}
+
+parameters {
+ real init_I; // initial number of infected
+ real init_R; // initial reproduction number
+ array[n-1] real rw_noise; // random walk noise
+ real rw_sd; // random walk standard deviation
+ simplex[d] reporting_delay; // reporting delay distribution
+}
+
+transformed parameters {
+ array[n] real R = geometric_random_walk(init_R, rw_noise, rw_sd);
+ array[n] real infections = renewal(init_I, R, gen_time_pmf);
+ array[n] real onsets = convolve_with_delay(infections, ip_pmf);
+ array[m] real onsets_by_report = observe_onsets_with_delay(onsets, reporting_delay, P, p);
+}
+
+model {
+ // Prior
+ init_I ~ lognormal(-1, 1);
+ init_R ~ normal(-.1, 0.5); // Approximately Normal(1, 0.5)
+ rw_noise ~ std_normal();
+ rw_sd ~ normal(0, 0.05) T[0,];
+ reporting_delay ~ dirichlet(rep_vector(1, d));
+ // Likelihood
+ obs ~ poisson(onsets_by_report);
+}
+
+generated quantities {
+ array[d*n] real complete_onsets_by_report = observe_onsets_with_delay(onsets, reporting_delay, D, rep_array(d, n));
+ array[n] int nowcast = combine_obs_with_predicted_obs_rng(obs, complete_onsets_by_report, P, p, d, D);
+}
+
diff --git a/inst/stan/joint-nowcast.stan b/inst/stan/joint-nowcast.stan
new file mode 100644
index 0000000..b5c91eb
--- /dev/null
+++ b/inst/stan/joint-nowcast.stan
@@ -0,0 +1,46 @@
+functions {
+ #include "functions/geometric_random_walk.stan"
+ #include "functions/observe_onsets_with_delay.stan"
+ #include "functions/combine_obs_with_predicted_obs_rng.stan"
+}
+
+data {
+ int n; // number of days
+ int m; // number of reports
+ array[n] int p; // number of observations per day
+ array[m] int obs; // observed symptom onsets
+ int d; // number of reporting delays
+}
+
+transformed data{
+ array[n] int P = to_int(cumulative_sum(p));
+ array[n] int D = to_int(cumulative_sum(rep_array(d, n)));
+}
+
+parameters {
+ real init_onsets;
+ array[n-1] real rw_noise;
+ real rw_sd;
+ simplex[d] reporting_delay; // reporting delay distribution
+}
+
+transformed parameters {
+ array[n] real onsets = geometric_random_walk(init_onsets, rw_noise, rw_sd);
+ array[m] real onsets_by_report = observe_onsets_with_delay(onsets, reporting_delay, P, p);
+}
+
+model {
+ // Prior
+ init_onsets ~ normal(1, 1) T[0,];
+ rw_noise ~ std_normal();
+ rw_sd ~ normal(0, 0.1) T[0,];
+ reporting_delay ~ dirichlet(rep_vector(1, d));
+ // Likelihood
+ obs ~ poisson(onsets_by_report);
+}
+
+generated quantities {
+ array[d*n] real complete_onsets_by_report = observe_onsets_with_delay(onsets, reporting_delay, D, rep_array(d, n));
+ array[n] int nowcast = combine_obs_with_predicted_obs_rng(obs, complete_onsets_by_report, P, p, d, D);
+}
+
diff --git a/inst/stan/lognormal.stan b/inst/stan/lognormal.stan
new file mode 100644
index 0000000..58f83e3
--- /dev/null
+++ b/inst/stan/lognormal.stan
@@ -0,0 +1,17 @@
+// lognormal_model.stan
+data {
+ int n; // number of data points
+ array[n] real y; // data
+}
+
+parameters {
+ real meanlog;
+ real sdlog;
+}
+
+model {
+ meanlog ~ normal(0, 10); // prior distribution
+ sdlog ~ normal(0, 10) T[0, ]; // prior distribution
+
+ y ~ lognormal(meanlog, sdlog);
+}
diff --git a/inst/stan/mechanistic-r.stan b/inst/stan/mechanistic-r.stan
new file mode 100644
index 0000000..076d2b9
--- /dev/null
+++ b/inst/stan/mechanistic-r.stan
@@ -0,0 +1,46 @@
+functions {
+ #include "functions/convolve_with_delay.stan"
+ #include "functions/pop_bounded_renewal.stan"
+}
+
+data {
+ int n; // number of days
+ int I0; // number initially infected
+ array[n] int obs; // observed symptom onsets
+ int gen_time_max; // maximum generation time
+ array[gen_time_max] real gen_time_pmf; // pmf of generation time distribution
+ int ip_max; // max incubation period
+ array[ip_max + 1] real ip_pmf;
+ int h; // number of days to forecast
+ array[2] real N_prior; // prior for total population
+}
+
+transformed data {
+ int m = n + h;
+}
+
+parameters {
+ real R; // initial reproduction number
+ real N; // total population
+}
+
+transformed parameters {
+ array[m] real infections = pop_bounded_renewal(I0, R, gen_time_pmf, N, m);
+ array[m] real onsets = convolve_with_delay(infections, ip_pmf);
+}
+
+model {
+ // priors
+ R ~ normal(1, 0.5) T[0,];
+ N ~ normal(N_prior[1], N_prior[2]) T[0,];
+ obs ~ poisson(onsets[1:n]);
+}
+
+generated quantities {
+ array[h] real forecast;
+ if (h > 0) {
+ for (i in 1:h) {
+ forecast[i] = poisson_rng(onsets[n + i]);
+ }
+ }
+}
diff --git a/inst/stan/simple-nowcast-rw.stan b/inst/stan/simple-nowcast-rw.stan
new file mode 100644
index 0000000..18bdc58
--- /dev/null
+++ b/inst/stan/simple-nowcast-rw.stan
@@ -0,0 +1,30 @@
+functions {
+ #include "functions/geometric_random_walk.stan"
+ #include "functions/condition_onsets_by_report.stan"
+}
+
+data {
+ int n; // number of days
+ array[n] int obs; // observed symptom onsets
+ int report_max; // max reporting delay
+ array[report_max + 1] real report_cdf;
+}
+
+parameters {
+ real init_onsets;
+ array[n-1] real rw_noise;
+ real rw_sd;
+}
+
+transformed parameters {
+ array[n] real onsets = geometric_random_walk(init_onsets, rw_noise, rw_sd);
+ array[n] real reported_onsets = condition_onsets_by_report(onsets, report_cdf);
+}
+
+model {
+ init_onsets ~ lognormal(0, 1) T[0,];
+ rw_noise ~ std_normal();
+ rw_sd ~ normal(0, 5) T[0,];
+ //Likelihood
+ obs ~ poisson(reported_onsets);
+}
diff --git a/inst/stan/simple-nowcast.stan b/inst/stan/simple-nowcast.stan
new file mode 100644
index 0000000..8e00c99
--- /dev/null
+++ b/inst/stan/simple-nowcast.stan
@@ -0,0 +1,24 @@
+functions {
+ #include "functions/condition_onsets_by_report.stan"
+}
+
+data {
+ int n; // number of days
+ array[n] int obs; // observed symptom onsets
+ int report_max; // max reporting delay
+ array[report_max + 1] real report_cdf;
+}
+
+parameters {
+ array[n] real onsets;
+}
+
+transformed parameters {
+ array[n] real reported_onsets = condition_onsets_by_report(onsets, report_cdf);
+}
+
+model {
+ onsets ~ normal(5, 20) T[0,];
+ // Likelihood
+ obs ~ poisson(reported_onsets);
+}
diff --git a/inst/stan/statistical-r.stan b/inst/stan/statistical-r.stan
new file mode 100644
index 0000000..a90fda6
--- /dev/null
+++ b/inst/stan/statistical-r.stan
@@ -0,0 +1,51 @@
+functions {
+ #include "functions/convolve_with_delay.stan"
+ #include "functions/renewal.stan"
+ #include "functions/geometric_diff_ar.stan"
+}
+
+data {
+ int n; // number of days
+ int I0; // number initially infected
+ array[n] int obs; // observed symptom onsets
+ int gen_time_max; // maximum generation time
+ array[gen_time_max] real gen_time_pmf; // pmf of generation time distribution
+ int ip_max; // max incubation period
+ array[ip_max + 1] real ip_pmf;
+ int h; // number of days to forecast
+}
+
+transformed data {
+ int m = n + h;
+}
+
+parameters {
+ real init_R; // initial reproduction number
+ array[m-1] real rw_noise; // random walk noise
+ real rw_sd; // random walk standard deviation
+ real damp; // damping
+}
+
+transformed parameters {
+ array[m] real R = geometric_diff_ar(init_R, rw_noise, rw_sd, damp);
+ array[m] real infections = renewal(I0, R, gen_time_pmf);
+ array[m] real onsets = convolve_with_delay(infections, ip_pmf);
+}
+
+model {
+ // priors
+ init_R ~ normal(-.1, 0.5); // Approximately Normal(1, 0.5)
+ rw_noise ~ std_normal();
+ rw_sd ~ normal(0, 0.01) T[0,];
+ damp ~ normal(1, 0.05) T[0, 1];
+ obs ~ poisson(onsets[1:n]);
+}
+
+generated quantities {
+ array[h] real forecast;
+ if (h > 0) {
+ for (i in 1:h) {
+ forecast[i] = poisson_rng(onsets[n + i]);
+ }
+ }
+}
diff --git a/inst/stan/truncated-delay-model.stan b/inst/stan/truncated-delay-model.stan
new file mode 100644
index 0000000..8f13558
--- /dev/null
+++ b/inst/stan/truncated-delay-model.stan
@@ -0,0 +1,19 @@
+data {
+ int n;
+ array[n] real onset_to_hosp;
+ array[n] real time_since_onset;
+}
+
+parameters {
+ real meanlog;
+ real sdlog;
+}
+
+model {
+ meanlog ~ normal(0, 10);
+ sdlog ~ normal(0, 10) T[0, ];
+
+ for (i in 1:n) {
+ onset_to_hosp[i] ~ lognormal(meanlog, sdlog) T[0, time_since_onset[i]];
+ }
+}
diff --git a/learning_objectives.qmd b/learning_objectives.qmd
new file mode 100644
index 0000000..1030ee7
--- /dev/null
+++ b/learning_objectives.qmd
@@ -0,0 +1,58 @@
+---
+title: "Independent learning outcomes"
+comments: false
+---
+
+## R and statistical concepts used
+
+- familiarity with R concepts used in the course
+ - to be completed once the course has been fully written but likely includes functions, accessing documentation, etc.
+- understanding of statistical concepts used in the course
+ - to be completed once the course has been fully written but likely includes discrete and continuous probability distributions
+
+## Delay distributions
+
+- understanding of the ubiquity of delays in epidemiological data
+- understanding of how delays affect population-level epidemiological data via discrete convolutions
+- ability to apply convolutions of discrete probability distributions to epidemiological data in R
+
+## Biases in delay distributions
+
+- understanding of how censoring affects the estimation and interpretation of epidemiological delay distributions
+- ability to estimate parameters of probability distributions from observed delays, taking into account censoring, using R
+- understanding of right truncation in epidemiolgical data
+- ability to estimate parameters of probability distributions from observed delays, taking into account truncation, in R
+
+## $R_t$ estimation and the renewal equation
+
+- understanding of the reproduction number and challenges in its estimation
+- awareness of broad categories of methods for estimating the reproduction number, including estimation from population-level data
+- understanding of the renewal equation as an epidemiological model
+- awareness of connections of the renewal equation with other epidemiological models
+- familiarity with the generation time as a particular type of delay distributions
+- ability to estimate static and time-varying reproduction numbers from time-series data in R
+
+## Nowcasting
+
+- understanding of nowcasting as a particular right truncation problem
+- Ability to perform a simple nowcast in R
+- awareness of the breadth of methods to perform nowcasting
+- $R_t$ estimation as a nowcasting problem
+
+## Forecasting
+
+- understanding of forecasting as an epidemiological problem, and its relationship with nowcasting and $R_t$ estimation
+- understanding of the difference between forecasts, projections and scenarios
+- familiarity with common forecasting models and their properties, and applicability in epidemiology
+- ability to use a common forecasting model on an epidemiological time series in R
+- ability to use a semi-mechanistic model for forecasting an epidemiological time series in R
+
+## Ensemble models
+
+- understanding of predictive ensembles and their properties
+- ability to create a predictive ensemble of forecasts in R
+
+## Evaluating forecasts (and nowcasts)
+
+- familiarity with metrics for evaluating probabilistic forecasts and their properties
+- ability to score probabilistic forecasts in R
diff --git a/man/add_delays.Rd b/man/add_delays.Rd
new file mode 100644
index 0000000..e3f46bd
--- /dev/null
+++ b/man/add_delays.Rd
@@ -0,0 +1,21 @@
+% Generated by roxygen2: do not edit by hand
+% Please edit documentation in R/add_delays.r
+\name{add_delays}
+\alias{add_delays}
+\title{Simulate symptom onset and hospitalization times from infection times}
+\usage{
+add_delays(infection_times)
+}
+\arguments{
+\item{infection_times}{A data frame containing a column of infection times}
+}
+\value{
+A data frame with columns for infection time, onset time, and
+ hospitalization time (with 70% of hospitalizations set to NA)
+}
+\description{
+Simulate symptom onset and hospitalization times from infection times
+}
+\examples{
+add_delays(infection_times)
+}
diff --git a/man/censored_delay_pmf.Rd b/man/censored_delay_pmf.Rd
new file mode 100644
index 0000000..92d63ee
--- /dev/null
+++ b/man/censored_delay_pmf.Rd
@@ -0,0 +1,29 @@
+% Generated by roxygen2: do not edit by hand
+% Please edit documentation in R/censored-delay-pmf.r
+\name{censored_delay_pmf}
+\alias{censored_delay_pmf}
+\title{Discretise a Continuous Delay Distribution}
+\usage{
+censored_delay_pmf(rgen, max, n = 1e+06, ...)
+}
+\arguments{
+\item{rgen}{A function that generates random delays, e.g., `rgamma`,
+`rlnorm`.}
+
+\item{max}{The maximum delay.}
+
+\item{n}{The number of replicates to simulate. Defaults to `1e+6`.}
+
+\item{...}{Additional parameters of the delay distribution.}
+}
+\value{
+A vector of probabilities corresponding to discrete indices from
+ `0` to `max`, representing the discretised delay distribution.
+}
+\description{
+This function discretises a continuous delay distribution into a probability
+mass function (PMF) over discrete days.
+}
+\examples{
+censored_delay_pmf(rgen = rgamma, max = 14, shape = 5, rate = 1)
+}
diff --git a/man/convolve_with_delay.Rd b/man/convolve_with_delay.Rd
new file mode 100644
index 0000000..7e63e6a
--- /dev/null
+++ b/man/convolve_with_delay.Rd
@@ -0,0 +1,25 @@
+% Generated by roxygen2: do not edit by hand
+% Please edit documentation in R/convolve-with-delay.r
+\name{convolve_with_delay}
+\alias{convolve_with_delay}
+\title{Convolve a Time Series with a Delay Distribution}
+\usage{
+convolve_with_delay(ts, delay_pmf)
+}
+\arguments{
+\item{ts}{A vector of the time series to convolve.}
+
+\item{delay_pmf}{The probability mass function of the delay, given as a
+vector of probabilities, corresponding to discrete indices 0, 1, 2 of the
+discretised delay distribution.}
+}
+\value{
+A vector of the convolved time series.
+}
+\description{
+This function convolves a time series with a delay distribution given as a
+probability mass function (PMF).
+}
+\examples{
+convolve_with_delay(ts = c(10, 14, 10, 10), delay_pmf = c(0.1, 0.6, 0.3))
+}
diff --git a/man/dot-extract_stan_functions.Rd b/man/dot-extract_stan_functions.Rd
new file mode 100644
index 0000000..d43411f
--- /dev/null
+++ b/man/dot-extract_stan_functions.Rd
@@ -0,0 +1,24 @@
+% Generated by roxygen2: do not edit by hand
+% Please edit documentation in R/nfidd-stan-tools.r
+\name{.extract_stan_functions}
+\alias{.extract_stan_functions}
+\title{Extract function names or content from Stan code}
+\usage{
+.extract_stan_functions(content, names_only = FALSE, functions = NULL)
+}
+\arguments{
+\item{content}{Character vector containing Stan code}
+
+\item{names_only}{Logical, if TRUE extract function names, otherwise
+extract function content.}
+
+\item{functions}{Optional, character vector of function names to extract
+content for.}
+}
+\value{
+Character vector of function names or content
+}
+\description{
+Extract function names or content from Stan code
+}
+\keyword{internal}
diff --git a/man/geometric_diff_ar.Rd b/man/geometric_diff_ar.Rd
new file mode 100644
index 0000000..647204a
--- /dev/null
+++ b/man/geometric_diff_ar.Rd
@@ -0,0 +1,27 @@
+% Generated by roxygen2: do not edit by hand
+% Please edit documentation in R/geometric-diff-ar.r
+\name{geometric_diff_ar}
+\alias{geometric_diff_ar}
+\title{Geometric Differenced Autoregressive Process}
+\usage{
+geometric_diff_ar(init, noise, std, damp)
+}
+\arguments{
+\item{init}{The initial value.}
+
+\item{noise}{A vector of steps (on the standard normal scale).}
+
+\item{std}{The step size of the random walk.}
+
+\item{damp}{A damping parameter.}
+}
+\value{
+A vector of the generated geometric differenced autoregressive
+process.
+}
+\description{
+This function generates a geometric differenced autoregressive process.
+}
+\examples{
+geometric_diff_ar(init = 1, noise = rnorm(100), phi = 0.1, std = 0.1)
+}
diff --git a/man/geometric_random_walk.Rd b/man/geometric_random_walk.Rd
new file mode 100644
index 0000000..cb6bf2c
--- /dev/null
+++ b/man/geometric_random_walk.Rd
@@ -0,0 +1,24 @@
+% Generated by roxygen2: do not edit by hand
+% Please edit documentation in R/geometric-random-walk.r
+\name{geometric_random_walk}
+\alias{geometric_random_walk}
+\title{Geometric Random Walk}
+\usage{
+geometric_random_walk(init, noise, std)
+}
+\arguments{
+\item{init}{The initial value.}
+
+\item{noise}{A vector of steps (on the standard normal scale).}
+
+\item{std}{The step size of the random walk.}
+}
+\value{
+A vector of the generated geometric random walk.
+}
+\description{
+This function generates a geometric random walk.
+}
+\examples{
+geometric_random_walk(init = 1, noise = rnorm(100), std = 0.1)
+}
diff --git a/man/make_daily_infections.Rd b/man/make_daily_infections.Rd
new file mode 100644
index 0000000..0ccfb24
--- /dev/null
+++ b/man/make_daily_infections.Rd
@@ -0,0 +1,21 @@
+% Generated by roxygen2: do not edit by hand
+% Please edit documentation in R/make-daily-infections.r
+\name{make_daily_infections}
+\alias{make_daily_infections}
+\title{Convert infection times to a daily time series}
+\usage{
+make_daily_infections(infection_times)
+}
+\arguments{
+\item{infection_times}{A data frame containing a column of infection times}
+}
+\value{
+A data frame with columns infection_day and infections, containing
+ the daily count of infections
+}
+\description{
+Convert infection times to a daily time series
+}
+\examples{
+make_daily_infections(infection_times)
+}
diff --git a/man/make_gen_time_pmf.Rd b/man/make_gen_time_pmf.Rd
new file mode 100644
index 0000000..493d8c1
--- /dev/null
+++ b/man/make_gen_time_pmf.Rd
@@ -0,0 +1,21 @@
+% Generated by roxygen2: do not edit by hand
+% Please edit documentation in R/simulate_onsets.r
+\name{make_gen_time_pmf}
+\alias{make_gen_time_pmf}
+\title{Generate a probability mass function for the generation time}
+\usage{
+make_gen_time_pmf(max = 14, shape = 4, rate = 1)
+}
+\arguments{
+\item{max}{Maximum delay to consider}
+
+\item{shape}{Shape parameter for the gamma distribution}
+
+\item{rate}{Rate parameter for the gamma distribution}
+}
+\value{
+A vector of probabilities representing the generation time PMF
+}
+\description{
+Generate a probability mass function for the generation time
+}
diff --git a/man/make_ip_pmf.Rd b/man/make_ip_pmf.Rd
new file mode 100644
index 0000000..6d85d27
--- /dev/null
+++ b/man/make_ip_pmf.Rd
@@ -0,0 +1,21 @@
+% Generated by roxygen2: do not edit by hand
+% Please edit documentation in R/simulate_onsets.r
+\name{make_ip_pmf}
+\alias{make_ip_pmf}
+\title{Generate a probability mass function for the incubation period}
+\usage{
+make_ip_pmf(max = 14, shape = 5, rate = 1)
+}
+\arguments{
+\item{max}{Maximum delay to consider}
+
+\item{shape}{Shape parameter for the gamma distribution}
+
+\item{rate}{Rate parameter for the gamma distribution}
+}
+\value{
+A vector of probabilities representing the incubation period PMF
+}
+\description{
+Generate a probability mass function for the incubation period
+}
diff --git a/man/nfidd_cmdstan_model.Rd b/man/nfidd_cmdstan_model.Rd
new file mode 100644
index 0000000..d255261
--- /dev/null
+++ b/man/nfidd_cmdstan_model.Rd
@@ -0,0 +1,33 @@
+% Generated by roxygen2: do not edit by hand
+% Please edit documentation in R/nfidd-stan-tools.r
+\name{nfidd_cmdstan_model}
+\alias{nfidd_cmdstan_model}
+\title{Create a CmdStanModel with NFIDD Stan functions}
+\usage{
+nfidd_cmdstan_model(model_name, include_paths = nfidd::nfidd_stan_path(), ...)
+}
+\arguments{
+\item{model_name}{Character string specifying which Stan model to use.}
+
+\item{include_paths}{Character vector of paths to include for Stan
+compilation. Defaults to the result of `nfidd_stan_path()`.}
+
+\item{...}{Additional arguments passed to cmdstanr::cmdstan_model().}
+}
+\value{
+A CmdStanModel object.
+}
+\description{
+This function creates a CmdStanModel object using a specified Stan model from
+the NFIDD package and optionally includes additional user-specified Stan
+files.
+}
+\examples{
+\dontshow{if (requireNamespace("cmdstanr", quietly = TRUE)) (if (getRversion() >= "3.4") withAutoprint else force)(\{ # examplesIf}
+if (!is.null(cmdstanr::cmdstan_version(error_on_NA = FALSE))) {
+ model <- nfidd_cmdstan_model("renewal", compile = FALSE)
+ model
+}
+\dontshow{\}) # examplesIf}
+}
+\concept{modelhelpers}
diff --git a/man/nfidd_load_stan_functions.Rd b/man/nfidd_load_stan_functions.Rd
new file mode 100644
index 0000000..6e8ec1b
--- /dev/null
+++ b/man/nfidd_load_stan_functions.Rd
@@ -0,0 +1,43 @@
+% Generated by roxygen2: do not edit by hand
+% Please edit documentation in R/nfidd-stan-tools.r
+\name{nfidd_load_stan_functions}
+\alias{nfidd_load_stan_functions}
+\title{Load Stan functions as a string}
+\usage{
+nfidd_load_stan_functions(
+ functions = NULL,
+ stan_path = nfidd::nfidd_stan_path(),
+ wrap_in_block = FALSE,
+ write_to_file = FALSE,
+ output_file = "nfidd_functions.stan"
+)
+}
+\arguments{
+\item{functions}{Character vector of function names to load. Defaults to all
+functions.}
+
+\item{stan_path}{Character string, the path to the Stan code. Defaults to the
+path to the Stan code in the nfidd package.}
+
+\item{wrap_in_block}{Logical, whether to wrap the functions in a
+`functions{}` block. Default is FALSE.}
+
+\item{write_to_file}{Logical, whether to write the output to a file. Default
+is FALSE.}
+
+\item{output_file}{Character string, the path to write the output file if
+write_to_file is TRUE. Defaults to "nfidd_functions.stan".}
+}
+\value{
+A character string containing the requested Stan functions
+}
+\description{
+Load Stan functions as a string
+}
+\seealso{
+Other stantools:
+\code{\link{nfidd_stan_function_files}()},
+\code{\link{nfidd_stan_functions}()},
+\code{\link{nfidd_stan_path}()}
+}
+\concept{stantools}
diff --git a/man/nfidd_stan_function_files.Rd b/man/nfidd_stan_function_files.Rd
new file mode 100644
index 0000000..5c16713
--- /dev/null
+++ b/man/nfidd_stan_function_files.Rd
@@ -0,0 +1,33 @@
+% Generated by roxygen2: do not edit by hand
+% Please edit documentation in R/nfidd-stan-tools.r
+\name{nfidd_stan_function_files}
+\alias{nfidd_stan_function_files}
+\title{Get Stan files containing specified functions}
+\usage{
+nfidd_stan_function_files(
+ functions = NULL,
+ stan_path = nfidd::nfidd_stan_path()
+)
+}
+\arguments{
+\item{functions}{Character vector of function names to search for. If NULL,
+all Stan files are returned.}
+
+\item{stan_path}{Character string specifying the path to the directory
+containing Stan files. Defaults to the Stan path of the nfidd
+package.}
+}
+\value{
+A character vector of file paths to Stan files.
+}
+\description{
+This function retrieves Stan files from a specified directory, optionally
+filtering for files that contain specific functions.
+}
+\seealso{
+Other stantools:
+\code{\link{nfidd_load_stan_functions}()},
+\code{\link{nfidd_stan_functions}()},
+\code{\link{nfidd_stan_path}()}
+}
+\concept{stantools}
diff --git a/man/nfidd_stan_functions.Rd b/man/nfidd_stan_functions.Rd
new file mode 100644
index 0000000..e873bb5
--- /dev/null
+++ b/man/nfidd_stan_functions.Rd
@@ -0,0 +1,28 @@
+% Generated by roxygen2: do not edit by hand
+% Please edit documentation in R/nfidd-stan-tools.r
+\name{nfidd_stan_functions}
+\alias{nfidd_stan_functions}
+\title{Get Stan function names from Stan files}
+\usage{
+nfidd_stan_functions(stan_path = nfidd::nfidd_stan_path())
+}
+\arguments{
+\item{stan_path}{Character string specifying the path to the directory
+containing Stan files. Defaults to the Stan path of the nfidd
+package.}
+}
+\value{
+A character vector containing unique names of all functions found in
+the Stan files.
+}
+\description{
+This function reads all Stan files in the specified directory and extracts
+the names of all functions defined in those files.
+}
+\seealso{
+Other stantools:
+\code{\link{nfidd_load_stan_functions}()},
+\code{\link{nfidd_stan_function_files}()},
+\code{\link{nfidd_stan_path}()}
+}
+\concept{stantools}
diff --git a/man/nfidd_stan_models.Rd b/man/nfidd_stan_models.Rd
new file mode 100644
index 0000000..4fe5f17
--- /dev/null
+++ b/man/nfidd_stan_models.Rd
@@ -0,0 +1,22 @@
+% Generated by roxygen2: do not edit by hand
+% Please edit documentation in R/nfidd-stan-tools.r
+\name{nfidd_stan_models}
+\alias{nfidd_stan_models}
+\title{List Available Stan Models in NFIDD}
+\usage{
+nfidd_stan_models(stan_path = nfidd::nfidd_stan_path())
+}
+\arguments{
+\item{stan_path}{Character string specifying the path to Stan files. Defaults
+to the result of `nfidd_stan_path()`.}
+}
+\value{
+A character vector of available Stan model names.
+}
+\description{
+This function finds all available Stan models in the NFIDD package and
+returns their names without the .stan extension.
+}
+\examples{
+nfidd_list_stan_models()
+}
diff --git a/man/nfidd_stan_path.Rd b/man/nfidd_stan_path.Rd
new file mode 100644
index 0000000..6d242bc
--- /dev/null
+++ b/man/nfidd_stan_path.Rd
@@ -0,0 +1,21 @@
+% Generated by roxygen2: do not edit by hand
+% Please edit documentation in R/nfidd-stan-tools.r
+\name{nfidd_stan_path}
+\alias{nfidd_stan_path}
+\title{Get the path to Stan code}
+\usage{
+nfidd_stan_path()
+}
+\value{
+A character string with the path to the Stan code
+}
+\description{
+Get the path to Stan code
+}
+\seealso{
+Other stantools:
+\code{\link{nfidd_load_stan_functions}()},
+\code{\link{nfidd_stan_function_files}()},
+\code{\link{nfidd_stan_functions}()}
+}
+\concept{stantools}
diff --git a/man/renewal.Rd b/man/renewal.Rd
new file mode 100644
index 0000000..e7447fa
--- /dev/null
+++ b/man/renewal.Rd
@@ -0,0 +1,31 @@
+% Generated by roxygen2: do not edit by hand
+% Please edit documentation in R/renewal.r
+\name{renewal}
+\alias{renewal}
+\title{Simulate Infections using the Renewal Equation}
+\usage{
+renewal(I0, R, gen_time)
+}
+\arguments{
+\item{I0}{The initial number of infections.}
+
+\item{R}{The reproduction number, given as a vector with one entry per time
+point.}
+
+\item{gen_time}{The generation time distribution, given as a vector with one
+entry per day after infection (the first element corresponding to one day
+after infection).}
+}
+\value{
+A vector of simulated infections over time.
+}
+\description{
+This function simulates infections using the renewal equation.
+}
+\examples{
+renewal(
+ I0 = 5,
+ R = c(rep(3, 4), rep(0.5, 5)),
+ gen_time = c(0.1, 0.2, 0.3, 0.2, 0.1)
+)
+}
diff --git a/man/simulate_onsets.Rd b/man/simulate_onsets.Rd
new file mode 100644
index 0000000..64769d6
--- /dev/null
+++ b/man/simulate_onsets.Rd
@@ -0,0 +1,34 @@
+% Generated by roxygen2: do not edit by hand
+% Please edit documentation in R/simulate_onsets.r
+\name{simulate_onsets}
+\alias{simulate_onsets}
+\title{Simulate symptom onsets from daily infection counts}
+\usage{
+simulate_onsets(
+ inf_ts,
+ gen_time_pmf = make_gen_time_pmf(),
+ ip_pmf = make_ip_pmf()
+)
+}
+\arguments{
+\item{inf_ts}{A data frame containing columns infection_day and infections,
+as returned by `make_daily_infections()`.}
+
+\item{gen_time_pmf}{A vector of probabilities representing the generation
+time PMF, as returned by `make_gen_time_pmf()`.}
+
+\item{ip_pmf}{A vector of probabilities representing the incubation period
+PMF, as returned by `make_ip_pmf()`.}
+}
+\value{
+A data frame with columns day, onsets, and infections containing
+ the daily count of symptom onsets and infections
+}
+\description{
+Simulate symptom onsets from daily infection counts
+}
+\examples{
+gt_pmf <- make_gen_time_pmf()
+ip_pmf <- make_ip_pmf()
+simulate_onsets(make_daily_infections(infection_times), gt_pmf, ip_pmf)
+}
diff --git a/nfidd.Rproj b/nfidd.Rproj
new file mode 100644
index 0000000..7301b00
--- /dev/null
+++ b/nfidd.Rproj
@@ -0,0 +1,22 @@
+Version: 1.0
+
+RestoreWorkspace: Default
+SaveWorkspace: Default
+AlwaysSaveHistory: Default
+
+EnableCodeIndexing: Yes
+UseSpacesForTab: Yes
+NumSpacesForTab: 2
+Encoding: UTF-8
+
+RnwWeave: Sweave
+LaTeX: pdfLaTeX
+
+AutoAppendNewline: Yes
+StripTrailingWhitespace: Yes
+
+BuildType: Package
+PackageUseDevtools: Yes
+PackageInstallArgs: --no-multiarch --with-keep.source
+
+MarkdownWrap: Sentence
diff --git a/sessions.qmd b/sessions.qmd
new file mode 100644
index 0000000..eef7a29
--- /dev/null
+++ b/sessions.qmd
@@ -0,0 +1,110 @@
+---
+title: "Sessions"
+comments: false
+---
+
+## Session 0: Introduction and course overview
+
+Monday June 24: 9.00-9.30
+
+- Introduction to the course and the instructors (10 mins)
+- Motivating the course: From an epidemiological line list to informing decisions in real-time (20 mins)
+
+## Session 1: R, Stan, and statistical concept background
+
+Monday June 24: 9.30-10.30
+
+- Introduction to statistical concepts used in the course (15 mins)
+- Introduction to stan concepts used in the course (15 mins)
+- Practice session: introduction to estimation in stan (30 mins)
+
+## Session 2: Delay distributions
+
+Monday June 24: 11.00-11.45
+
+- Introduction to epidemiological delays and how to represent them with probability distributions (10 mins)
+- Practice session: simulate and estimate epidemiological delays (30 mins)
+- Wrap up (5 mins)
+
+## Session 3: Biases in delay distributions
+
+Monday June 24: 11.45-12.30 and 14.00-14.45
+
+- Introduction to biases in delay distributions (10 mins)
+- Practice session: Simulating biases in delay distributions and estimating delays without adjustment on these data (35 mins)
+- Practice session: estimating delay distributions with adjustments for bias (35 mins)
+- Wrap up (10 mins)
+
+## Session 4: Using delay distributions to model the data generating process of an epidemic
+
+Monday June 24: 14.45-15.30
+
+- Using delay distributions to model the data generating process of an epidemic (15 mins)
+- Practice session: implementing a convolution model and identifying potential problems (30 mins)
+
+## Session 5: $R_t$ estimation and the renewal equation
+
+Monday June 24: 16.00-17.30
+
+- Introduction to the time-varying reproduction number (10 mins)
+- Practice session: using the renewal equation to estimate R (35 mins)
+- Practice session: combining $R_t$ estimation with delay distribution convolutions (35 mins)
+- Wrap up (10 mins)
+
+## Session 6: Nowcasting concepts
+
+Tuesday June 25: 9.00-10.30
+
+- Introduction to nowcasting as a right-truncation problem (10 mins)
+- Practice session: Simulating the delay distribution (35 mins)
+- Practice session: Nowcasting using pre-estimated delay distributions (35 mins)
+- Wrap up (10 mins)
+
+## Session 7: Nowcasting with an unknown reporting delay
+
+Tuesday June 25: 11.00-12.30
+
+- Introduction to joint estimation of delays and nowcasts (10 mins)
+- Practice session: Joint estimation of delays and nowcasts (35 mins)
+- Practice session: Joint estimation of delays, nowcasts and reproduction numbers (35 mins)
+- Wrap up (10 mins)
+
+## Session 8: Forecasting concepts
+
+Tuesday June 25: 14.00-15.30
+
+- Introduction to forecasting as an epidemiological problem, and its relationship with nowcasting and $R_t$ estimation (10 mins)
+- Practice session: extending a model into the future (35 minutes)
+- Practice session: evaluate your forecast (35 mins)
+- Wrap up (10 mins)
+
+## Session 9: Forecasting models
+
+Tuesday June 25: 16.00-17.30
+
+- An overview of forecasting models (10 mins)
+- Practice session: Evaluating forecasts from a range of models (60 mins)
+- Wrap up and discussion (20 mins)
+
+## Session 10: Examples of available tools
+
+Tuesday June 25: 9.00-10.30
+
+- Introduction to tools for $R_t$ estimation, nowcasting and forecasting (5 mins)
+- Group work: explore a tool and relate it to key concepts in the course (30 mins)
+- Discussion (15 mins)
+- Individual exploration of all packages (35 mins)
+- Wrap up (5 mins)
+
+## Session 11: Methods in the real world
+
+Wednesday June 26: 11.00-12.00
+
+- Presentations and Q&A on uses of nowcasts & forecasts in the real world (60 mins)
+
+## Session 12: End of course summary
+
+Wednesday June 26: 12.00-12.30
+
+- Summary of the course (10 mins)
+- Final discussion and closing (20 mins)
diff --git a/sessions/R-Stan-and-statistical-concepts.qmd b/sessions/R-Stan-and-statistical-concepts.qmd
new file mode 100644
index 0000000..d80542f
--- /dev/null
+++ b/sessions/R-Stan-and-statistical-concepts.qmd
@@ -0,0 +1,208 @@
+---
+title: "Probability distributions and parameter estimation"
+order: 1
+---
+
+# Introduction
+
+Many important characteristics, or parameters, of epidemiological processes are not fully observed - and are therefore uncertain. For example, in this course this might include time delays, reproduction numbers, or case numbers now and in the future. We can specify the shape of uncertainty around a specific parameter using a probability distribution.
+
+We'll want to correctly specify this uncertainty. We can do this best by combining our own prior understanding with the data that we have available. In this course, we'll use this Bayesian approach to modelling. A useful tool for creating these models is the `stan` probabilistic programming language.
+
+## Slides
+
+- [Introduction to statistical concepts used in the course](slides/introduction-to-statistical-concepts)
+- [Introduction to stan concepts used in the course](slides/introduction-to-stan)
+
+## Objectives
+
+The aim of this session is to introduce the concept of probability distributions and how to estimate their parameters using Bayesian inference with `stan`.
+
+::: {.callout-note collapse="true"}
+
+# Setup
+
+## Source file
+
+The source file of this session is located at `sessions/R-Stan-and-statistical-concepts.qmd`.
+
+## Libraries used
+
+In this session we will use the `ggplot2` library for plotting and the `nfidd` package to load the stan models.
+We will also use the `bayesplot` and `posterior` packages to investigate the results of the inference conducted with stan.
+
+```{r libraries, message = FALSE}
+library("nfidd")
+library("ggplot2")
+library("bayesplot")
+library("posterior")
+```
+
+::: {.callout-tip}
+The best way to interact with the material is via the [Visual Editor](https://docs.posit.co/ide/user/ide/guide/documents/visual-editor.html) of RStudio.
+:::
+
+## Initialisation
+
+We set a random seed for reproducibility.
+Setting this ensures that you should get exactly the same results on your computer as we do.
+We also set an option that makes `cmdstanr` show line numbers when printing model code.
+This is not strictly necessary but will help us talk about the models.
+
+```{r}
+set.seed(123)
+options(cmdstanr_print_line_numbers = TRUE)
+```
+
+:::
+
+# Simulating data from a probability distribution
+
+First let us simulate some data from a probability distribution.
+In R, this is usually done using a family of random number generation functions that start with `r`.
+For example, to simulate random numbers from a normal distribution you would use the `rnorm()` function.
+All these functions have a first argument `n`, the number of random replicates to generate, and then some further arguments that parameterise the probability distribution.
+
+```{r rnorm}
+rnorm(n = 10, mean = 0, sd = 1)
+```
+
+We will use probability distributions to characterise epidemiological delays.
+These are usually strictly positive because e.g. one cannot develop symptoms before becoming infected, and therefore the incubation period cannot be less than zero.
+Note that this does not necessarily apply to all distributions, e.g. serial intervals can be negative if person X infects Y but Y develops symptoms first.
+
+Probability distribution that are commonly used in this situation are the gamma or lognormal distributions.
+These are fairly similar, with one difference being that the lognormal commonly has a "heavier tail" on the right, i.e., gives more probability to occasional very large values, whereas the gamma distribution has more of a tail on the left, i.e., gives more probability to values lower than the mean.
+
+The gamma distribution is characterised by the `shape` ($\alpha$) and `rate` ($\beta$) parameters, with a mean of $\alpha/\beta$ and variance $\alpha/\beta^2$.
+A gamma distribution with mean 5 and variance 2, for example, has $\alpha = 12.5$ and $\beta = 2.5$.
+To simulate from such a distribution, we can use the following R code.
+
+```{r gammas}
+### simulate gamma with mean 5, variance 2
+gammas <- rgamma(100, shape = 12.5, rate = 2.5)
+head(gammas)
+mean(gammas)
+var(gammas)
+```
+
+The lognormal distribution is characterised by the `meanlog` ($\mu$) and `sdlog` ($\sigma$) parameters, with a mean of $e^{\mu + 0.5\sigma^2}$ and variance $(e^{\sigma^2} - 1) e^{2\mu + \sigma^2}$.
+A lognormal distribution with mean 5 and variance 2, for example, has (after a bit of calculation) $\mu = 1.57$ and $\sigma = 0.28$.
+
+```{r lognormals}
+### simulate lognormals with mean 5, variance 2
+lognormals <- rlnorm(100, meanlog = 1.57, sdlog = 0.28)
+head(lognormals)
+mean(lognormals)
+var(lognormals)
+```
+
+We can now plot the two distributions.
+
+```{r plot_randoms}
+df <- rbind(
+ data.frame(dist = "lognormal", randoms = lognormals),
+ data.frame(dist = "gamma", randoms = gammas)
+)
+ggplot(df, aes(x = randoms, fill = dist)) +
+ geom_density(alpha = 0.5)
+```
+
+We have used `geom_density()` to get smooth lines.
+Alternatively we could have used, e.g., `geom_histogram()` to plot the raw data.
+
+## Estimating the parameters of probability distributions
+
+We will now use stan to estimate the parameters of the probability distribution.
+To do so, we first load in the stan model.
+Normally, you would do this by saving the model code in a text file called `gamma_model.stan` and then loading this using `cmdstanr::cmdstan_model()`.
+For this course, we included all the models in the `nfidd` package, and they can be accessed using `nfidd_cmdstan_model()` which uses `cmdstanr::cmdstan_model()` to load the specified model included with the package.
+Afterwards, we can use this model exactly how we would if we had loaded it using `cmdstanr` directly.
+
+```{r load_gamma_model}
+### load gamma model from the nfidd package
+mod <- nfidd_cmdstan_model("gamma")
+### show model code
+mod
+```
+
+::: {.callout-tip}
+### Arrays in stan
+On line 4 there is a data declaration starting with `array[n]`.
+This declares an array of size `n` of the type given afterwards (here: `real`).
+Arrays work in a similar way as arrays or vectors in R and its elements be accessed with the bracket operator `[`.
+For example, to get the third element of the array `y` you would write `y[3]`.
+:::
+
+::: {.callout-tip}
+### Take 5 minutes
+Familarise yourself with the model above.
+Do you understand all the lines?
+Which line(s) define the parameter prior distribution(s), which one(s) the likelihood, and which one(s) the data that has to be supplied to the model?
+:::
+
+::: {.callout-note collapse="true"}
+### Solution
+Lines 13 and 14 define the parametric prior distributions (for parameters alpha and beta).
+Line 15 defines the likelihood.
+Lines 3 and 4 define the data that has to be supplied to the model.
+:::
+
+We use the model we have defined in conjunction with the gamma distributed random numbers generated earlier to see if we can recover the parameters of the gamma distribution used.
+Once you have familiarised yourself with the model, use the `sample()` function to fit the model.
+
+```{r gamma_inference, results='hide', message=FALSE}
+stan_data <- list(
+ N = length(gammas),
+ y = gammas
+)
+gamma_fit <- mod$sample(data = stan_data)
+```
+
+::: {.callout-note}
+### Passing data to the stan model
+The `stan_data` object is a list with elements that will is passed to the stan model as the `data` argument to `sample`.
+The names and types of the elements need to correspond to the data block in the model (see lines 3 and 4 in the model).
+Here, we pass the length of `gammas` as `N` and the vector `gammas` itself as `y`.
+:::
+
+::: {.callout-note}
+### Stan messages
+The`mod$sample` command will produce a lot of messages which we have suppressed above.
+This is fine and intended to keep the user informed about any issues as well as general progress with the inference.
+This will come in handy later in the course when we fit more complicated models that can take a little while to run.
+:::
+
+In order to view a summary of the posterior samples generated, use
+
+```{r gamma_summary}
+gamma_fit
+```
+
+You can see that the estimates are broadly consistent with the parameters we specified.
+To investigate this further, we will conduct a so-called *posterior predictive check* by comparing random numbers simulated using the estimated parameters to the ones we simulated earlier.
+
+```{r gamma_ppc}
+## Extract posterior draws
+gamma_posterior <- as_draws_df(gamma_fit$draws())
+head(gamma_posterior)
+
+## Generate posterior predictive samples
+gamma_ppc <- sapply(seq_along(gammas), function(i) {
+ rgamma(n = length(gammas),
+ shape = gamma_posterior$alpha[i],
+ rate = gamma_posterior$beta[i])
+})
+
+## Plot posterior predictive check
+ppc_dens_overlay(y = gammas, yrep = gamma_ppc)
+```
+
+We can see that the random numbers generated from the posterior samples are distributed relatively evenly around the data (in black), i.e., the samples generated earlier that we fitted to.
+
+## Going further
+
+- For the model above we chose truncated normal priors with a mode at 0 and standard deviation 10. If you change the parameters of the prior distributions, does it affect the results?
+- You could try the model included in `lognormal.stan` to estimate parameters of the lognormal distribution.
+
+## Wrap up
diff --git a/sessions/R-estimation-and-the-renewal-equation.qmd b/sessions/R-estimation-and-the-renewal-equation.qmd
new file mode 100644
index 0000000..81bf507
--- /dev/null
+++ b/sessions/R-estimation-and-the-renewal-equation.qmd
@@ -0,0 +1,497 @@
+---
+title: "$R_t$ estimation and the renewal equation"
+order: 5
+---
+
+# Introduction
+
+In the last session we used the idea of convolutions as a way to interpret individual time delays at a population level. In that session, we linked symptom onsets back to infections. Now we want to link infections themselves together over time, knowing that current infections were infected by past infections. Correctly capturing this transmission process is crucial to modelling infections in the present and future.
+
+## Slides
+
+- [Introduction to the reproduction number](slides/introduction-to-reproduction-number)
+
+## Objectives
+
+The aim of this session is to introduce the renewal equation as an infection generating process, and to show how it can be used to estimate a time-varying reproduction number.
+
+::: {.callout-note collapse="true"}
+
+# Setup
+
+## Source file
+
+The source file of this session is located at `sessions/R-estimation-and-the-renewal-equation.qmd`.
+
+## Libraries used
+
+In this session we will use the `nfidd` package to load a data set of infection times and access stan models and helper functions, the `dplyr` and `tidyr` packages for data wrangling, `ggplot2` library for plotting, and the `tidybayes` package for extracting results of the inference.
+
+```{r libraries, message = FALSE}
+library("nfidd")
+library("dplyr")
+library("tidyr")
+library("ggplot2")
+library("tidybayes")
+```
+
+::: {.callout-tip}
+The best way to interact with the material is via the [Visual Editor](https://docs.posit.co/ide/user/ide/guide/documents/visual-editor.html) of RStudio.
+:::
+
+## Initialisation
+
+We set a random seed for reproducibility.
+Setting this ensures that you should get exactly the same results on your computer as we do.
+We also set an option that makes `cmdstanr` show line numbers when printing model code.
+This is not strictly necessary but will help us talk about the models.
+
+```{r}
+set.seed(123)
+options(cmdstanr_print_line_numbers = TRUE)
+```
+
+:::
+
+# The renewal equation as a process model for infectious diseases
+
+In this session we introduce modelling the infection process itself, in addition to modelling observation processes.
+
+Recall that in the [session on convolutions](using-delay-distributions-to-model-the-data-generating-process-of-an-epidemic#estimating-a-time-series-of-infections) we tried to estimate the number of infections.
+In doing so we assumed that infections every day were independently identically distributed and determined only by the number of symptom onsets that they caused.
+In reality, however, we know that infections are not independent.
+Because infection is dependent on a pathogen being transmitted from one individual to another, we expect infections on any day to depend on existing infections, that is the number of individuals that became infectious in the recent past.
+We now express this relationship via the renewal equation, which links these recent infections to the number of new infections expected on any day via the reproduction number $R_t$.
+
+Remember that this is a more general concept than the _basic_ reproduction number $R_0$ which represents the average number of secondary infections caused by a single infectious individual in a completely susceptible population.
+
+The reproduction number $R_t$ (sometimes called the _effective_ reproduction number) more generally describes the average number of secondary infections caused by a single infectious individual and can vary in time and space as a function of differences in population level susceptibility, changes in behaviour, policy, seasonality etc.
+
+In most mechanistic models of infectious diseases (starting with the simplest SIR model), $R_t$ arises out of a combination of parameters and variables representing the system state, for example in a simple SIR model it can be calculated as $R_0 S/N$ where $S$ is the current number of susceptibles in the population of size $N$.
+By fitting such models to data it is then possible to calculate the value of $R_t$ at any point in time.
+
+The _renewal equation_ represents a more general model which includes the SIR model as a special case.
+In its basic form it makes no assumption about the specific processes that cause $R_t$ to have a certain value and/or change over time, but instead it only relates the number of infected people in the population, the current value of the reproduction number and a delay distribution that represents the timings of when individuals infect others relative to when they themselves became infected, the so-called generation time.
+Mathematically, it can be written as
+
+$$
+I_t = R_t \sum_{i=1}^{g_\mathrm{max}} I_{t-i} g_i
+$$
+
+Here, $I_t$ is the number of infected individuals on day $t$, $R_t$ is the current value of the reproduction number and $g_i$ is the probability of a secondary infection occurring $i$ days after the infector became infected themselves, with a maximum $g_\mathrm{max}$.
+Remembering the [session on convolutions](using-delay-distributions-to-model-the-data-generating-process-of-an-epidemic#delay-distributions-and-convolutions) you will be able to identify that the renewal equation represents a convolution of the infection time series with itself, with the delay distribution given by $g_i$ and $R_t$ representing a scaling that is being applied.
+
+::: {.callout-tip}
+## Discrete vs. continuous renewal equation
+The equation shown above represents the discrete version of the reproduction number.
+Similar to discussions in the [session on convolutions](using-delay-distributions-to-model-the-data-generating-process-of-an-epidemic#delay-distributions-and-convolutions) this can be interpreted as a discretised version of a continuous one where the sum is replaced by an integral and the generation time distribution is continuous.
+Note that in the discrete version we have started the sum at 1 and thus set $g_0=0$ which will make calculations easier.
+:::
+
+::: {.callout-tip}
+## Instantaneous vs. case reproduction number
+There are different definitions of the reproduction number that can be applied to the situation where it changes in time.
+As it is defined above it is also called the _instantaneous_ reproduction number because any change affects all currently infectious individuals instantaneously.
+Another example of a definition is the _case_ reproduction number, where changes affect individuals at the time that they are infected but then they have a constant reproduction number throughout their infectious period.
+:::
+
+::: {.callout-tip}
+## Stochastic vs. deterministic renewal equation
+The version of the discrete renewal equation we wrote above is deterministic, i.e. knowing the number of infections up to a certain time point and the reproduction number we can work out exactly how many new infections we will see.
+Sometimes stochasticity is added where the equation above gives the _expectation_ of $I_t$ but there exists random variation around it.
+In this course we will only deal with the deterministic renewal equation.
+:::
+
+# Simulating an epidemic using the renewal equation
+
+With the theory out of the way we now turn to simulating an epidemic using the renewal equation.
+We can use function included in the `nfidd` package to simulate the epidemic using the discrete renewal equation.
+
+```{r renewal_equation}
+renewal
+```
+
+::: {.callout-note}
+## Take 10 minutes
+Try to understand the `renewal()` function above.
+Compare it to the `convolve_with_delay()` function from the [session on convolutions](using-delay-distributions-to-model-the-data-generating-process-of-an-epidemic#delay-distributions-and-convolutions).
+How are they similar?
+Can you explain the key differences between the two?
+Try calling the function with a few different probability distributions and parameters.
+What kind of behaviours do you see depending on the values you put in?
+:::
+
+# Estimating $R_t$ from a time series of infections
+
+We now return to the time series of infections we used in the [session on convolutions](using-delay-distributions-to-model-the-data-generating-process-of-an-epidemic#delay-distributions-and-convolutions).
+
+```{r load_ts}
+inf_ts <- make_daily_infections(infection_times)
+head(inf_ts)
+```
+
+This creates the `inf_ts` data set which we can look at e.g. using
+
+```{r load_ts_inspect}
+head(inf_ts)
+```
+
+We use a renewal equation model in _stan_ to estimate the effective reproduction number throughout the outbreak.
+We assume that the generation time is gamma-distributed with mean 4 and standard deviation 2, with a maximum of 2 weeks (14 days).
+From this we can calculate that the parameters of the distribution are shape 4 and rate 1.
+We can use the `censored_delay_pmf()` function defined in the [session on convolutions](using-delay-distributions-to-model-the-data-generating-process-of-an-epidemic#delay-distributions-and-convolutions) to use this continuous distribution with the discrete renewal equation.
+
+To approximate the generation time PMF using random draws from the underlying continuous distribution use
+```{r gen_time_pmf}
+gen_time_pmf <- censored_delay_pmf(rgamma, max = 14, shape = 4, rate = 1)
+```
+
+The discrete renewal equation is only valid for generation times greater than 0 so we remove the first element of the pmf and re-normalise:
+
+```{r gen_time_renorm}
+gen_time_pmf <- gen_time_pmf[-1] ## remove first element
+gen_time_pmf <- gen_time_pmf / sum(gen_time_pmf) ## renormalise
+```
+
+As always we first load the stan model and spend some time trying to understand it.
+
+```{r stan_estimate_r}
+r_mod <- nfidd_cmdstan_model("estimate-r")
+r_mod
+```
+
+::: {.callout-tip}
+## Take 5 minutes
+Familiarise yourself with the model above.
+Again there is a `functions` block at the beginning of the model (lines 1-3), where we load a function called `renewal()` (line 2) from a file of the same name which can be found in the subdirectory `functions` of the `stan` directory or [viewed on the github repo](https://github.com/nfidd/nfidd/blob/main/stan/functions/renewal.stan).
+The functions correspond exactly to our earlier **R** function of the same name.
+Later, this function is called in the `model` block, to generate the time series of infections using the discretised renewal model (line 19).
+Which line defines priors, and which the likelihood?
+:::
+
+::: {.callout-note collapse="true"}
+## Solution
+Line 24 defines the prior distribution of $R_t$ at each time point, and Line 25 defines the likelihood using Poisson observation uncertainty.
+:::
+
+Once again we can generate estimates from this model:
+
+```{r r_fit, results = 'hide', message = FALSE}
+data <- list(
+ n = nrow(inf_ts) - 1,
+ obs = inf_ts$infections[-1],
+ I0 = inf_ts$infections[1],
+ gen_time_max = length(gen_time_pmf),
+ gen_time_pmf = gen_time_pmf
+)
+r_fit <- r_mod$sample(data = data, parallel_chains = 4)
+```
+
+```{r r_fit_summary}
+r_fit
+```
+
+Once stan has run its chains, we can visualise the posterior estimates.
+
+```{r r_plot}
+# Extract posterior draws
+r_posterior <- r_fit |>
+ gather_draws(R[infection_day]) |>
+ ungroup() |>
+ mutate(infection_day = infection_day - 1) |>
+ filter(.draw %in% sample(.draw, 100))
+
+ggplot(
+ data = r_posterior,
+ aes(x = infection_day, y = .value, group = .draw)) +
+ geom_line(alpha = 0.1) +
+ labs(title = "Estimated Rt",
+ subtitle = "Model 1: renewal equation from infections")
+```
+
+::: {.callout-tip}
+## Take 10 minutes
+Simulate from the renewal equation using the `renewal()` R function we defined above with a given $R_t$ trajectory.
+For example, you could look at $R_t$ increasing steadily, or suddenly, or having any other trajectory you might imagine.
+Use the stan model to infer the trajectory of the reproduction number from the resulting time series of infection.
+Does the model reproduce the simulated $R_t$ trajectories?
+:::
+
+# Estimating $R_t$ from a time series of symptom onsets
+
+Epidemiological data is rarely, perhaps never, available as a time series of infection events.
+Instead, we usually observe outcomes such as symptom onsets when individuals interact with the health system, e.g. by presenting to a hospital.
+In the [session on convolutions](using-delay-distributions-to-model-the-data-generating-process-of-an-epidemic#delay-distributions-and-convolutions) we simulated symptom onsets from a time series of infections by convolving with a delay and then sampling from a Poisson distribution:
+For this we used the `convolved_with_delay()` function.
+
+We then simulate observations again using:
+
+```{r generate_obs}
+ip_pmf <- censored_delay_pmf(rgamma, max = 14, shape = 5, rate = 1)
+onsets <- convolve_with_delay(inf_ts$infections, ip_pmf)
+obs <- rpois(length(onsets), onsets)
+```
+
+We now add this to our renewal equation model and use this to estimate infections as well as the reproduction number:
+
+```{r stan_estimate_inf_and_r}
+r_inf_mod <- nfidd_cmdstan_model("estimate-inf-and-r")
+r_inf_mod
+```
+::: {.callout-tip}
+## Take 5 minutes
+Familiarise yourself with the model above.
+Compare it to the model used earlier in this session, and the one used in the [session on convolutions](using-delay-distributions-to-model-the-data-generating-process-of-an-epidemic#estimating-a-time-series-of-infections).
+Does this model have more parameters?
+How do the assumptions about the infections time series differ between the models?
+:::
+
+We then generate estimates from this model:
+
+```{r r_inf_fit, results = 'hide', message = FALSE}
+data <- list(
+ n = length(obs) - 1,
+ obs = obs[-1],
+ I0 = inf_ts$infections[1],
+ gen_time_max = length(gen_time_pmf),
+ gen_time_pmf = gen_time_pmf,
+ ip_max = length(ip_pmf) - 1,
+ ip_pmf = ip_pmf
+)
+r_inf_fit <- r_inf_mod$sample(
+ data = data, parallel_chains = 4, init = \() list(init_R = 0)
+)
+```
+
+::: {.callout-note}
+Generally one should start MCMC samplers with multiple different starting values to make sure the whole posterior distribution is explored.
+When testing this model we noticed that sometimes the model failed to fit the data at all.
+Because of this we added an argument to start sampling with `init_R` set to 0.
+This makes sure the sampler starts fitting the model from a sensible value and avoids fitting failiures.
+:::
+
+```{r r_inf_fit_summary}
+r_inf_fit
+```
+
+This time we can extract both the `infections` and `R` variables by infection day.
+
+```{r r_inf_posterior}
+r_inf_posteriors <- r_inf_fit |>
+ gather_draws(infections[infection_day], R[infection_day]) |>
+ ungroup() |>
+ mutate(infection_day = infection_day - 1) |>
+ filter(.draw %in% sample(.draw, 100))
+```
+
+We can visualise infections compared to the data used to generate the time series of onsets
+
+```{r plot_infections}
+inf_posterior <- r_inf_posteriors |>
+ filter(.variable == "infections")
+ggplot(inf_posterior, mapping = aes(x = infection_day)) +
+ geom_line(mapping = aes(y = .value, group = .draw), alpha = 0.1) +
+ geom_line(
+ data = inf_ts, mapping = aes(y = infections), colour = "red"
+ ) +
+ labs(title = "Infections, estimated (grey) and observed (red)",
+ subtitle = "Model 2: renewal equation from symptom onsets")
+```
+
+and reproduction numbers
+
+```{r plot_rt}
+r_inf_posterior <- r_inf_posteriors |>
+ filter(.variable == "R")
+ggplot(
+ r_inf_posterior, mapping = aes(x = infection_day, y = .value, group = .draw)
+) +
+ geom_line(alpha = 0.1)
+```
+
+# Improving the generative model for the reproduction number
+
+In the model so far we have assumed that the reproduction number at any time point is independent of the reproduction number at any other time point. This assumption has resulted in the quite noisy estimates of the reproduction number that we have seen in the plots above.
+
+In reality, we might expect the reproduction number to change more smoothly over time (except in situations of drastic change such as a very effective intervention) and to be more similar at adjacent time points. We can model this by assuming that the reproduction number at time $t$ is a random draw from a normal distribution with mean equal to the reproduction number at time $t-1$ and some standard deviation $\sigma$. This can be described as a random walk model for the reproduction number. In fact, rather than using this model directly, a better choice might be to use a model where the logarithm of the reproduction number does a random walk, as this will ensure that the reproduction number is always positive and that changes are multiplicative rather than additive (i.e as otherwise the same absolute change in the reproduction number would have a larger effect when the reproduction number is small which likely doesn't match your intuition for how outbreaks evolve over time). We can write this model as
+
+$$
+\sigma \sim HalfNormal(0, 0.05) \\
+$$
+$$
+\log(R_0) \sim \mathcal{Lognormal}(-0.1, 0.5)
+$$
+$$
+\log(R_t) \sim \mathcal{N}(\log(R_{t-1}), \sigma)
+$$
+
+Here we have placed a prior on the standard deviation of the random walk, which we have assumed to be half-normal (i.e., normal but restricted to being non-negative) with a mean of 0 and a standard deviation of 0.05. This is a so-called _weakly informative prior_ that allows for some variation in the reproduction number over time but not an unrealistic amount. We have also placed a prior on the initial reproduction number, which we have assumed to be log-normally distributed with a mean of -0.1 and a standard deviation of 0.5. This is a weakly informative prior that allows for a wide range of initial reproduction numbers but has a mean of approximately 1.
+The last line is the geometric random walk.
+
+## Simulating a geometric random walk
+
+You can have a look at an R function for performing the geometric random walk:
+
+```{r geometric-random-walk}
+geometric_random_walk
+```
+
+::: {.callout-tip}
+## Take 5 minutes
+Look at this function and try to understand what it does.
+Note that we use the fact that we can generate a random normally distributed variable $X$ with mean 0 and standard deviation $\sigma$ by mutiplying a standard normally distributed variable (i.e., mean 0 and standard deviation 1) $Y$ with $\sigma$.
+Using this [non-centred parameterisation](https://mc-stan.org/docs/2_18/stan-users-guide/reparameterization-section.html) for efficiency) will improve our computational efficency later when using an equivalent function in stan
+:::
+
+We can use this function to simulate a random walk:
+
+```{r simulate-geometric-walk}
+R <- geometric_random_walk(init = 1, noise = rnorm(100), std = 0.1)
+data <- tibble(t = seq_along(R), R = exp(R))
+
+ggplot(data, aes(x = t, y = R)) +
+ geom_line() +
+ labs(title = "Simulated data from a random walk model",
+ x = "Time",
+ y = "R")
+```
+
+You can repeat this multiple times either with the same parameters or changing some to get a feeling for what this does.
+
+## Estimating $R_t$ with a geometric random walk prior
+
+We can now include this in a stan model,
+
+```{r stan_estimate_inf_and_r_rw}
+rw_mod <- nfidd_cmdstan_model("estimate-inf-and-r-rw")
+rw_mod
+```
+
+Note that the model is very similar to the one we used earlier, but with the addition of the random walk model for the reproduction number using a function in stan that does the same as our R function of the same name we defined.
+
+We can now generate estimates from this model:
+
+```{r r_inf_rw_fit, results = 'hide', message = FALSE}
+data <- list(
+ n = length(obs) - 1,
+ obs = obs[-1],
+ I0 = inf_ts$infections[1],
+ gen_time_max = length(gen_time_pmf),
+ gen_time_pmf = gen_time_pmf,
+ ip_max = length(ip_pmf) - 1,
+ ip_pmf = ip_pmf
+)
+r_rw_inf_fit <- rw_mod$sample(
+ data = data, parallel_chains = 4, max_treedepth = 12,
+ init = \() list(init_R = 0, rw_sd = 0.01)
+)
+```
+
+```{r r_inf_rw_fit_summary}
+r_rw_inf_fit
+```
+
+::: {.callout-note}
+As this is a more complex model we have increased the `max_treedepth` parameter to 12 to allow for more complex posterior distributions and we have also provided an initialisation for the `init_R` and `rw_sd` parameters to help the sampler find the right region of parameter space. This is a common technique when fitting more complex models and is needed as it is hard a priori to know where the sampler should start.
+:::
+
+We can again extract and visualise the posteriors in the same way as earlier.
+
+```{r r_rw_posterior}
+rw_posteriors <- r_rw_inf_fit |>
+ gather_draws(infections[infection_day], R[infection_day]) |>
+ ungroup() |>
+ mutate(infection_day = infection_day - 1) |>
+ filter(.draw %in% sample(.draw, 100))
+```
+
+```{r plot_infections_rw}
+rw_inf_posterior <- rw_posteriors |>
+ filter(.variable == "infections")
+ggplot(mapping = aes(x = infection_day)) +
+ geom_line(
+ data = rw_inf_posterior, mapping = aes(y = .value, group = .draw), alpha = 0.1
+ ) +
+ geom_line(data = inf_ts, mapping = aes(y = infections), colour = "red") +
+ labs(title = "Infections, estimated (grey) and observed (red)",
+ subtitle = "Model 3: renewal equation with random walk")
+```
+
+and reproduction numbers
+
+```{r plot_rt_rw}
+rw_r_inf_posterior <- rw_posteriors |>
+ filter(.variable == "R") |>
+ filter(.draw %in% sample(.draw, 100))
+ggplot(
+ data = rw_r_inf_posterior,
+ mapping = aes(x = infection_day, y = .value, group = .draw)
+) +
+ geom_line(alpha = 0.1) +
+ labs(title = "Estimated R",
+ subtitle = "Model 3: renewal equation with random walk")
+```
+
+::: {.callout-tip}
+## Take 10 minutes
+Compare the results across the models used in this session, and the one used in the [session on convolutions](using-delay-distributions-to-model-the-data-generating-process-of-an-epidemic).
+How do the models vary in the number of parameters that need to be estimated?
+How do the assumptions about the infections time series differ between the models?
+What do you notice about the level of uncertainty in the estimates of infections and $R_t$ over the course of the time series?
+If you have time you could try re-running the experiment with different $R_t$ trajectories and delay distributions to see whether results change.
+
+::: {.callout-note collapse="true"}
+## Solution
+We can see that using the renewal model as generative model we recover the time series of infections more accurately compared to previously when we assumed independent numbers of infections each day and that using a more believable model (i.e the geometric random walk) for the reproduction number improves things even more.
+Of course, this is helped by the fact that the data was generated by a model similar to the renewal model used for inference.
+
+# Comparing the $R_t$ trajectories
+
+```{r plot_r_posteriors}
+## earlier posteriors
+r_posterior <- r_posterior |>
+ mutate(data = "infections")
+r_inf_posterior <- r_inf_posterior |>
+ mutate(data = "onsets (normal)")
+rw_r_inf_posterior <- rw_r_inf_posterior |>
+ mutate(data = "onsets (random walk)")
+
+all_posteriors <- rbind(
+ r_inf_posterior,
+ rw_r_inf_posterior,
+ r_posterior
+)
+
+ggplot(
+ all_posteriors,
+ mapping = aes(x = infection_day, y = .value, group = .draw,
+ colour = data)
+) +
+ geom_line(alpha = 0.1) +
+ scale_fill_brewer(palette = "Set1") +
+ labs(
+ title = "Rt estimates from renewal equation models",
+ subtitle = paste(
+ "Estimates from infections, from symptom onsets, and from onsets with a",
+ "random walk"
+ )
+ ) +
+ guides(colour = guide_legend(override.aes = list(alpha = 1)))
+```
+
+We can see that the estimates are smoother when using the random walk model for the reproduction number, compared to the normal model. The model that fits directly to infections has the lowest uncertainty, which we would expect as it doesn't have to infer the number of infections from symptom onsets but even here the reproduction number estimates are unrealistically noisy due to the assumption of independence between infections each day.
+
+:::
+:::
+
+# Going further
+
+- We have used symptom onsets under the assumption that every infected person develops symptoms.
+Earlier we also created a time series of hospitalisation under the assumption that only a proportion (e.g., 30%) of symptomatic individuals get hospitalised.
+How would you change the model in this case?
+What are the implications for inference?
+- The [`EpiNow2`](https://epiforecasts.io/EpiNow2/) package implements a more complex version of the model we have used here. You will have the opportunity to try it in a later session.
+
+
+
+
diff --git a/sessions/biases-in-delay-distributions.qmd b/sessions/biases-in-delay-distributions.qmd
new file mode 100644
index 0000000..f4a6cf5
--- /dev/null
+++ b/sessions/biases-in-delay-distributions.qmd
@@ -0,0 +1,304 @@
+---
+title: "Biases in delay distributions"
+order: 3
+---
+
+# Introduction
+
+So far, we've looked at the uncertainty of the time delays between epidemiological events. The next challenge is that our information on these delays is usually biased, especially when we're analysing data in real time. We'll consider two types of biases that commonly occur in reported infectious disease data:
+
+- *Censoring*: when we know an event occurred at some time, but not exactly when.
+- *Truncation*: when not enough time has passed for all the relevant epidemiological events to occur or be observed.
+
+We can again handle these by including them as uncertain parameters in the modelling process.
+
+## Slides
+
+[Introduction to biases in epidemiological delays](slides/introduction-to-biases-in-epidemiological-delays)
+
+## Objectives
+
+In this session, we'll introduce censoring and right truncation as typical properties of infectious disease data sets, using the delay from symptom onset to hospitalisation as an example.
+
+::: {.callout-note collapse="true"}
+
+# Setup
+
+## Source file
+
+The source file of this session is located at `sessions/biases-in-delay-distributions.qmd`.
+
+## Libraries used
+
+In this session we will use the `nfidd` package to load a data set of infection times and access stan models and helper functions, the `ggplot2` package for plotting, the `dplyr` and `tidyr` packages to wrangle data, the `lubridate` package to deal with dates.
+
+```{r libraries, message = FALSE}
+library("nfidd")
+library("ggplot2")
+library("dplyr")
+library("tidyr")
+library("lubridate")
+```
+
+::: callout-tip
+The best way to interact with the material is via the [Visual Editor](https://docs.posit.co/ide/user/ide/guide/documents/visual-editor.html) of RStudio.
+:::
+
+## Initialisation
+
+We set a random seed for reproducibility.
+Setting this ensures that you should get exactly the same results on your computer as we do.
+We also set an option that makes `cmdstanr` show line numbers when printing model code.
+This is not strictly necessary but will help us talk about the models.
+
+```{r}
+set.seed(123)
+options(cmdstanr_print_line_numbers = TRUE)
+```
+
+:::
+
+# Load data
+
+We will use the same simulated data set as in the [session on delay distributions](delay-distributions#simulating-delayed-epidemiological-data).
+
+::: callout-note
+Remember, in this outbreak we are assuming:
+
+- the incubation period is **gamma**-distributed with **shape 5** and **rate 1**, i.e. a mean of 5 days
+- the time from onset to hospital admission is **lognormally**-distributed, with **meanlog 1.75** and **sdlog 0.5**, i.e. a mean delay of about a week
+:::
+
+We use the same function we used in that session to simulate symptom onset and hospitalisation data.
+
+```{r inf_hosp_solution}
+df <- add_delays(infection_times)
+```
+
+This creates the `df` data frame that we can inspect e.g. using
+
+```{r show_df}
+head(df)
+```
+
+# Dates, not days: censoring
+
+Data on health outcomes are usually not recorded in the way that we have used so far in this session: as a numeric time since a given start date.
+Instead, we usually deal with *dates*.
+
+We can make our simulated dataset a bit more realistic by rounding down the infection times to an integer number.
+
+```{r censored_times}
+# Use the floor() function to round down to integers
+df_dates <- df |>
+ mutate(
+ infection_time = floor(infection_time),
+ onset_time = floor(onset_time),
+ hosp_time = floor(hosp_time)
+ )
+head(df_dates)
+```
+
+::: {.callout-note}
+As before we are still not working with dates but numbers.
+This makes handling the data easier - we don't have to make any conversions before using the data in stan.
+:::
+
+Each of the numbers now represent the number of days that have passed since the start of the outbreak.
+That is, each of the numbers correspond to a day.
+In that sense, the data is more like typical data we get from infectious disease outbreaks, where we would usually have a line list with key events such as symptom onset or death reported by a *date*.
+In statistical terms, we call the delay *double interval censored*: "double" because the delays represent the time between two events that are both censored; and "interval" because all we know about the timings of the events is that they happened in a certain time interval (between 0:00 and 23:59 on the recorded day).
+
+## Estimating delay distributions accounting for censoring
+
+Let's estimate the time from symptom onset to hospitalisation with the censored data.
+
+A naïve approach to estimating the delay would be to ignore the fact that the data are censored.
+To estimate the delay from onset to hospitalisation, we could just use the difference between the censored times, which is an integer (the number of days).
+
+```{r integer-delays}
+df_dates <- df_dates |>
+ mutate(
+ incubation_period = onset_time - infection_time,
+ onset_to_hosp = hosp_time - onset_time
+ )
+```
+
+::: callout-tip
+## Take 5 minutes
+
+Fit the lognormal model used in the [session on delay distributions](delay-distributions#estimating-delay-distributions) to the estimates from the rounded data, i.e. using the `df_dates` data set.
+Do you still recover the parameters that we put in?
+:::
+
+::: {.callout-note collapse="true"}
+## Solution
+
+```{r df_dates_solution, results = 'hide', message = FALSE}
+mod <- nfidd_cmdstan_model("lognormal")
+res <- mod$sample(
+ data = list(
+ n = nrow(na.omit(df_dates)),
+ y = na.omit(df_dates)$onset_to_hosp
+ )
+)
+```
+
+```{r df_dates_solution_summary}
+res
+```
+
+Usually the estimates will be further from the "true" parameters than before when we worked with the unrounded data.
+:::
+
+To account for double interval censoring, we need to modify the model to include the fact that we don't know when exactly on any given day the event happened.
+For example, if we know that symptom onset of an individual occurred on 20 June, 2024, and they were admitted to hospital on 22 June, 2024, this could mean an onset-to-hospitalisation delay from 1 day (onset at 23:59 on the 20th, admitted at 0:01 on the 22nd) to 3 days (onset at 0:01 on the 20th, admitted at 23:59 on the 22nd).
+
+We can use this in our delay estimation by making the exact time of the events based on the dates given part of the estimation procedure:
+
+```{r censoring_adjusted_delay_model}
+cmod <- nfidd_cmdstan_model("censored-delay-model")
+cmod
+```
+
+::: callout-tip
+## Take 5 minutes
+
+Familiarise yourself with the model above.
+Do you understand all the lines?
+Which line(s) define the parameter prior distribution(s), which one(s) the likelihood, and which one(s) reflect that we have now provided the delay as the difference in integer days?
+:::
+
+::: {.callout-note collapse="true"}
+## Solution
+
+Lines 21-24 define the parametric prior distributions (for parameters meanlog and sdlog, and the estimates of exact times of events).
+Line 27 defines the likelihood.
+Lines 15-17 reflect the integer delays, adjusted by the estimated times of day.
+:::
+
+Now we can use this model to re-estimate the parameters of the delay distribution:
+
+```{r censored_estimate, results = 'hide', message = FALSE}
+cres <- cmod$sample(
+ data = list(
+ n = nrow(na.omit(df_dates)),
+ onset_to_hosp = na.omit(df_dates)$onset_to_hosp
+ )
+)
+```
+
+```{r censored_estimate_summary}
+cres
+```
+
+::: callout-tip
+## Take 10 minutes
+
+Try re-simulating the delays using different parameters of the delay distribution.
+Can you establish under which conditions the bias in estimation gets worse?
+:::
+
+# Real-time estimation: truncation
+
+The data set we have looked at so far in this session is a "final" data set representing an outbreak that has come and gone.
+However, information on delay distribution is often important during ongoing outbreaks as they can inform nowcasts and forecasts and help with broader interpretation of data.
+
+Estimating delays in real time comes with particular challenges, as the timing of the cut-off might introduce a bias.
+If, for example, infections are exponentially increasing then there will be disproportionately more people with recent symptom onset.
+Without adjustment, this would artificially decrease the estimate of the mean delay compared to its true value for all infections.
+This happens because most infections are recent (due to the exponential increase), but later symptom onsets amongst these have not had a chance to happen yet.
+
+Once again, we can simulate this effect, for example by imagining we would like to make an estimate on day 70 of our outbreak.
+Let us work with the original, un-censored data for the time from onset to hospitalisation so as to look at the issue of truncation in isolation:
+
+```{r truncated_df}
+df_realtime <- df |>
+ mutate(onset_to_hosp = hosp_time - onset_time) |>
+ filter(hosp_time <= 70)
+```
+
+## Estimating delay distributions accounting for truncation
+
+If we take the naïve mean of delays we get an underestimate as expected:
+
+```{r mean_truncated}
+# truncated mean delay
+mean(df_realtime$onset_to_hosp)
+# compare with the mean delay over the full outbreak
+mean(df$hosp_time - df$onset_time, na.rm=TRUE)
+```
+
+::: callout-tip
+## Take 5 minutes
+
+Fit the lognormal model used above to the estimates from the truncated data, i.e. using the `df_realtime` data set.
+How far away from the "true" parameters do you end up?
+:::
+
+::: {.callout-note collapse="true"}
+## Solution
+
+```{r df_realtime_solution, results = 'hide', message = FALSE}
+res <- mod$sample(
+ data = list(
+ n = nrow(na.omit(df_realtime)),
+ y = na.omit(df_realtime)$onset_to_hosp
+ )
+)
+```
+
+```{r df_realtime_solution_summary}
+res
+```
+:::
+
+Once again, we can write a model that adjusts for truncation, by re-creating the simulated truncation effect in the stan model:
+
+```{r truncation_adjusted_delay_model}
+tmod <- nfidd_cmdstan_model("truncated-delay-model")
+tmod
+```
+
+::: callout-tip
+## Take 5 minutes
+
+Familiarise yourself with the model above.
+Which line introduces the truncation, i.e. the fact that we have not been able to observe hospitalisation times beyond the cutoff of (here) 70 days?
+:::
+
+::: {.callout-note collapse="true"}
+## Solution
+
+Line 17 defines the upper limit of `onset_to_hosp` as `time_since_onset`.
+:::
+
+Now we can use this model to re-estimate the parameters of the delay distribution:
+
+```{r truncated_estimate, results = 'hide', message = FALSE}
+tres <- tmod$sample(
+ data = list(
+ n = nrow(df_realtime),
+ onset_to_hosp = df_realtime$onset_to_hosp,
+ time_since_onset = 70 - df_realtime$onset_time
+ )
+)
+```
+
+```{r truncated_estimate_summary}
+tres
+```
+
+::: callout-tip
+## Take 10 minutes
+
+Try re-simulating the delays using different parameters of the delay distribution.
+Can you establish under which conditions the bias in estimation gets worse?
+:::
+
+# Going further
+
+- We have looked at censoring and truncation separately, but in reality often both are present. Can you combine the two in a model?
+- The solutions we introduced for addressing censoring and truncation are only some possible ones for the censoring problem. There are other solutions that reduce the biases from estimation even further. For a full overview, the [review by Park et al.](https://doi.org/10.1101/2024.01.12.24301247) might be worth a read. If you are feeling adventurous, try to implement one or more of them in the stan model above - with a warning that this can get quite involved very quickly.
+
+# Wrap up
diff --git a/sessions/delay-distributions.qmd b/sessions/delay-distributions.qmd
new file mode 100644
index 0000000..0badf82
--- /dev/null
+++ b/sessions/delay-distributions.qmd
@@ -0,0 +1,220 @@
+---
+title: "Delay distributions"
+order: 2
+---
+
+# Introduction
+
+Every outbreak starts with an infection, but we rarely observe these directly. Instead, there are many types of epidemiological events that we might observe that occur after the time of infection: symptom onsets, hospitalisations and discharge, etc. The length of time between any of these events might be highly variable and uncertain. However, we often need to have some estimate for these delays in order to understand what's happening now and predict what might happen in the future. We capture this variability using probability distributions, which is the focus of this session.
+
+## Slides
+
+- [Introduction to epidemiological delays](slides/introduction-to-epidemiological-delays)
+
+## Objectives
+
+The aim of this session is for you to familiarise yourself with the concept of delay distributions used to describe reporting in infectious disease epidemiology.
+First we'll look at this working forwards from infections in an outbreak. We'll use `R` to probabilistically simulate delays from infection to reporting symptoms and hospitalisations. Then we'll work only from this set of outcome data and use a stan model to estimate the parameters of one specific delay (in this example, symptom onset to hospitalisation).
+
+::: {.callout-note collapse="true"}
+
+# Setup
+
+## Source file
+
+The source file of this session is located at `sessions/delay-distributions.qmd`.
+
+## Libraries used
+
+In this session we will use the `nfidd` package to load a data set of infection times and access stan models and helper functions, the `ggplot2` package for plotting, the `dplyr` and `tidyr` packages to wrangle data, the `lubridate` package to deal with dates, and the `posterior` packages for investigating the results of the inference conducted with stan.
+
+```{r libraries, message = FALSE}
+library("nfidd")
+library("ggplot2")
+library("dplyr")
+library("tidyr")
+library("lubridate")
+library("posterior")
+```
+
+::: {.callout-tip}
+The best way to interact with the material is via the [Visual Editor](https://docs.posit.co/ide/user/ide/guide/documents/visual-editor.html) of RStudio.
+:::
+
+## Initialisation
+
+We set a random seed for reproducibility.
+Setting this ensures that you should get exactly the same results on your computer as we do.
+We also set an option that makes `cmdstanr` show line numbers when printing model code.
+This is not strictly necessary but will help us talk about the models.
+
+```{r}
+set.seed(1234)
+options(cmdstanr_print_line_numbers = TRUE)
+```
+
+:::
+
+# Simulating delayed epidemiological data
+
+We will start this session by working with a simulated data set of infections from a disease that has caused an outbreak which subsequently ended.
+In our example outbreak, there were 6383 infections.
+The outbreak lasted 140 days after the first infection, with new infections peaking roughly around day 80.
+
+::: callout-note
+For now we will not concern ourselves with the model used to generate the epidemic.
+This represents a typical situation in the real world, where we may have a model of how an infection has spread, but we don't necessarily know how well this corresponds to what really happened.
+:::
+
+We will later deal with modelling the infectious process.
+For now, we will focus on modelling how infections get reported in data - the *observation* process.
+Using infectious disease data for analysis comes with three common challenges:
+
+1. We don't normally observe infections directly, but their *outcomes* as symptomatic cases, hospitalisations or other realisations.
+2. These observations are *incomplete* (e.g. not every infection leads to hospitalisation, and so focusing on hospitalisations will leave infections unobserved).
+3. These observations happen with a *delay* after the infection occurs (e.g. from infection to symptoms).
+
+## Load the outbreak data
+
+We will work with a data set that is included in the `nfidd` R package that you installed initially.
+The column `infection_time` is a linelist of infections from our example outbreak, given as a decimal number of days that have passed since the initial infection of the outbreak.
+It can be loaded with the `data` command.
+
+```{r}
+data(infection_times)
+head(infection_times)
+
+### visualise the infection curve
+ggplot(infection_times, aes(x = infection_time)) +
+ geom_histogram(binwidth = 1) +
+ scale_x_continuous(n.breaks = 10) +
+ labs(x = "Infection time (in days)", y = "Number of infections",
+ title = "Infections during an outbreak")
+```
+
+::: callout-note
+In reality, data from an outbreak will usually be given as dates, not decimals; and those will usually not represent infection, but an observed outcome such as symptom onset or hospital admission.
+For now we don't want to spend too much time manipulating dates in R, but we will get back to working with more realistic outbreak data later.
+:::
+
+## Working forwards: simulating a sequence of delays after infection
+
+We would now like to simulate hospitalisations arising from this outbreak.
+We will start with our data on infection times, and work forwards to symptom onsets and then hospitalisations. We'll make the following assumptions about the process from infection to hospital admission:
+
+- Infection to symptoms:
+ - We'll assume all infections cause symptoms.
+ - *Time from infection to symptom onset (incubation period):* We assume that the incubation period is **gamma**-distributed with **shape 5** and **rate 1**, i.e. a mean of 5 days.
+- Symptoms to hospital admission:
+ - We'll assume that 30% of symptomatic cases become hospitalised.
+ - *Time from symptom onset to hospital admission*: We assume that the onset-to-hospitalisation period is **lognormally** distributed, with **meanlog 1.75** and **sdlog 0.5**, corresponding to a mean delay of about a week.
+
+Let's put these assumptions into practice by simulating some data, adding onset and hospitalisation times (in decimal number of days after the first infection) to the infection times. We'll use random values for each infection from the probability distributions we've assumed above.
+
+::: {.callout-note}
+Throughout the course, we repeatedly use some small chunks of code.
+Instead of copying these between sessions, we've put them into functions in the `nfidd` R package that we can use when needed.
+To see exactly what the function does, just type the function name, e.g. `add_delays` for the function below.
+:::
+
+```{r inf_hosp_solution}
+df <- add_delays(infection_times)
+```
+
+::: {.callout-tip}
+## Take 2 minutes
+Have a look at the `add_delays()` function and try to understand its inner workings.
+You can also access a help file for this function, just like any other R function, using `?add_delays`.
+:::
+
+Now we can plot infections, hospitalisations and onsets.
+
+```{r plot_distributions, messages = FALSE}
+# convert our data frame to long format
+dfl <- df |>
+ pivot_longer(
+ cols = c(infection_time, onset_time, hosp_time),
+ names_to = "type", values_to = "time"
+ )
+# plot
+ggplot(dfl, aes(x = time)) +
+ geom_histogram(position = "dodge", binwidth = 1) +
+ facet_wrap(~ type, ncol = 1) +
+ xlab("Time (in days)") +
+ ylab("Count")
+```
+
+## Estimating delay distributions from outcome data
+
+As mentioned above, our data set of infection, symptom onset and hospitalisation times is not the typical data set we encounter in outbreaks.
+In reality, we don't have infection dates, and we also have to deal with missing data, incomplete observations, data entry errors etc.
+For now, let us just assume we have a data set only including symptom onset times and some hospitalisation times.
+
+Let's look at a specific problem: we would like to estimate how long it takes for people to become hospitalised after becoming symptomatic.
+This might be an important delay to know about, for example when modelling and forecasting hospitalisations, or more generally for estimating required hospital capacity.
+
+To do this we can use our data with *stan* to model the delay from symptom onset to hospitalisation, with the same assumption that it follows a lognormal distribution.
+
+```{r naive_delay_model}
+mod <- nfidd_cmdstan_model("lognormal")
+mod
+```
+
+Let's estimate the onset-to-hospitalisation delay using the simulated data set we created above. Do we recover the parameters used for simulation?
+
+We can do this by *sampling* from the model's posterior distribution by feeding it our simulated data set.
+
+```{r sample_naive_delay_model, results = 'hide', message = FALSE}
+## Specify the time from onset to hospitalisation
+df_onset_to_hosp <- df |>
+ mutate(onset_to_hosp = hosp_time - onset_time) |>
+ # exclude infections that didn't result in hospitalisation
+ drop_na(onset_to_hosp)
+## Use the data to sample from the model posterior
+res <- mod$sample(
+ data = list(
+ n = nrow(df_onset_to_hosp),
+ y = df_onset_to_hosp$onset_to_hosp
+ )
+)
+```
+
+To find out more about the `sample` function we used here, you can use `?cmdstanr::sample`.
+To see the estimates, we can use:
+
+```{r summarise_naive_delay_model}
+res$summary() ## could also simply type `res`
+```
+
+These estimates should look similar to what we used in the simulations.
+We can plot the resulting probability density functions.
+
+::: {.callout-note}
+Every time we sample from the model posterior, we create 4000 iterations. That is a lot of data to plot, so instead, when we produce plots we'll use a random sample of 100 iterations. You'll see this throughout the course.
+:::
+
+```{r plot_onset_hospitalisation}
+## get shape and rate samples from the posterior
+res_df <- res |>
+ as_draws_df() |>
+ filter(.draw %in% sample(.draw, 100)) # sample 100 draws
+
+## find the value (x) that includes 99% of the cumulative density
+max_x <- max(qlnorm(0.99, meanlog = res_df$meanlog, sdlog = res_df$sdlog))
+
+## calculate density on grid of x values
+x <- seq(0, max_x, length.out = 100)
+res_df <- res_df |>
+ crossing(x = x) |> ## add grid to data frame
+ mutate(density = dlnorm(x, meanlog, sdlog))
+
+## plot
+ggplot(res_df, aes(x = x, y = density, group = .draw)) +
+ geom_line(alpha = 0.3)
+```
+
+# Going further
+
+- In this session we were in the enviable situation of knowing which distribution was used to generate the data. With real data, of course, we don't have this information available. Try using a different distribution for inference (e.g. normal, or gamma). Do you get a good fit?
+
+# Wrap up
diff --git a/sessions/end-of-course-summary-and-discussion.qmd b/sessions/end-of-course-summary-and-discussion.qmd
new file mode 100644
index 0000000..ac56b1d
--- /dev/null
+++ b/sessions/end-of-course-summary-and-discussion.qmd
@@ -0,0 +1,40 @@
+---
+title: "End of course summary and discussion"
+order: 13
+---
+
+# Introduction
+
+We hope that you've enjoyed taking this course on nowcasting and forecasting infectious disease dynamics.
+We will be very happy to have your feedback, comments or any questions on the [course discussion page](https://github.com/nfidd/nfidd/discussions).
+
+
+## Slides
+
+[End of course summary](slides/closing)
+
+# Further reading
+
+The following is a highly subjective list of papers we would recommend to read for those interested in engaging further with the topics discussed here.
+
+## Delay estimation
+- Park et al., [Estimating epidemiological delay distributions for infectious diseases](https://doi.org/10.1101/2024.01.12.24301247) provides a comprehensive overview of challenges in estimating delay distribution and how to overcome them.
+- Charniga et al., [Best practices for estimating and reporting epidemiological delay distributions of infectious diseases using public health surveillance and healthcare data](https://doi.org/10.48550/arXiv.2405.08841) summarises challenges in estimating delay distributions into a set of best practices.
+
+## $R_t$ estimation
+- Gostic et al., [Practical considerations for measuring the effective reproductive number, $R_t$](https://doi.org/10.1371/journal.pcbi.1008409) provides an overview of some of the challenges in estimating reproduction numbers.
+- Brockhaus et al., [Why are different estimates of the effective reproductive number so different? A case study on COVID-19 in Germany](https://doi.org/10.1371/journal.pcbi.1011653) compares reproduction number estimates from different models and investigates their differences.
+
+## Nowcasting
+- Wolffram et al., [Collaborative nowcasting of COVID-19 hospitalization incidences in Germany](https://doi.org/10.1371/journal.pcbi.1011394) compares the performance of a range of methods that were used in a nowcasting hub and investigates what might explain performance differences.
+- Lison et al., [Generative Bayesian modeling to nowcast the effective reproduction number from line list data with missing symptom onset dates](https://doi.org/10.1371/journal.pcbi.1012021) develops a generative model for nowcasting and $R_t$ estimation and compares its performance to an approach where the steps for estimating incidence and reproduction number are separated.
+- Stoner et al., [A Powerful Modelling Framework for Nowcasting and Forecasting COVID-19 and Other Diseases](https://doi.org/10.48550/arXiv.1912.05965) contains a nice review of different methods for nowcasting evaluates a range of methods, in addition to introducing a new approach.
+
+## Forecasting
+- Funk et al., [Assessing the performance of real-time epidemic forecasts: A case study of Ebola in the Western Area region of Sierra Leone, 2014-15](https://doi.org/10.1371/journal.pcbi.1006785) evaluates the performance of a forecasting method that combines a mechanistic SEIR model with a random walk prior for the reproduction number.
+- Held et al, [Probabilistic forecasting in infectious disease epidemiology: the 13th Armitage lecture](https://doi.org/10.1002/sim.7363) makes a compelling argument for the use of probabilistic forecasts and evaluates spatial forecasts based on routine surveillance data.
+- Lopez et al., [Challenges of COVID-19 Case Forecasting in the US, 2020-2021](https://doi.org/10.1101/2023.05.30.23290732) describes the difficulty encountered in making accurate forecasts in COVID-19 cases in the US COVID-19 Forecast Hub.
+- Sherratt et al., [Predictive performance of multi-model ensemble forecasts of COVID-19 across European nations](https://doi.org/10.7554/eLife.81916) investigates the performance of different ensembles in the European COVID-19 Forecast Hub.
+- Asher, [Forecasting Ebola with a regression transmission model](10.1016/j.epidem.2017.02.009) describes model that implements an extension to the random walk with a drift term.
+- CDC, code repo for [Wastewater-informed COVID-19 forecasting](https://github.com/CDCgov/wastewater-informed-covid-forecasting).
+- Hyndman and Athanasopoulos, [Forecasting: Principles and Practice (free online text book)](https://otexts.com/fpp3/) is a textbook on forecasting with a range of time series models and a great resource for finding out more about them.
diff --git a/sessions/examples-of-available-tools.qmd b/sessions/examples-of-available-tools.qmd
new file mode 100644
index 0000000..ffb6f70
--- /dev/null
+++ b/sessions/examples-of-available-tools.qmd
@@ -0,0 +1,65 @@
+---
+title: "Examples of available tools"
+order: 11
+---
+
+# Introduction
+
+In the course so far we introduced key concepts and methods in nowcasting and forecasting of infectious disease dynamics, using a combination of R and (mostly) stan.
+Being able to understand these concepts and implement them in stan comes with the ability to create and adapt models that are tailor made to any given situation and specific characteristics of any given data set.
+At the same time, there is value in using and contributing to open-source **tools** that implement some or all of the methods we have encountered in the course.
+These tools have varying levels of flexibility to deal with different kinds of epidemiological problems and data.
+Whilst they will never have the flexibility of completely custom approaches, using and contributing to them avoids duplication, improves the chances of finding errors, and helps discussing and ultimately enforcing best practice.
+
+## Slides
+
+- [Examples of tools](slides/tools)
+
+## Objective
+
+The aim of this session is to try out some of the tools that are available that use some of the ideas we have discussed in this course.
+
+::: {.callout-note collapse="true"}
+
+# Setup
+
+## Source file
+
+The source file of this session is located at `sessions/examples-of-available-tools.qmd`.
+
+## Installing tools
+
+In order to install the tools mentioned below, refer to the installation instructions supplied with each of the packages.
+
+:::
+
+# Trying out available tools
+
+We are going to suggest trying three tools that implement several or all the concepts introduced in this course:
+
+## EpiEstim
+
+[EpiEstim](https://mrc-ide.github.io/EpiEstim/) implements the renewal equation on a time series of infections in a Bayesian framework, i.e. the model in `estimate-r.stan` in the [session on $R_t$ estimation](R-estimation-and-the-renewal-equation#estimating-R-from-a-time-series-of-infections).
+In combination with the [projections](https://www.repidemicsconsortium.org/projections/) package in can be used for forecasting.
+
+The [EpiEstim vignette](https://mrc-ide.github.io/EpiEstim/articles/full_EpiEstim_vignette.html) is a good starting point for a walkthrough of $R_t$ estimation and forecasting.
+
+## EpiNow2
+
+[EpiNow2](https://epiforecasts.io/EpiNow2/dev/) implements the renewal equation on a time series of delayed outcomes including nowcasts with a known reporting delay distribution, as well as forecasts using a Gaussian Process or random walk model, i.e. the models introduced here except the joint inference of nowcasts and delays and/or reproduction numbers.
+
+The [example of nowcasting, $R_t$ estimation and forecasting with EpiNow2](https://github.com/epiforecasts/nowcasting.example/blob/main/inst/reports/epinow2.md) is a good place to find out more about how to use EpiNow2 with linelist data.
+
+## Epinowcast
+
+[Epinowcast](https://package.epinowcast.org/) was created to replace EpiNow2.
+It implements all the models discussed in the course with a frontend to work with line lists, data by reference and report date or cumulative data snapshots.
+Whilst this model can also be used for forecasts (as we have seen) this does not currently have a user-facing interface in the package.
+
+The [vignette on estimating the effective reproduction number in real-time for a single timeseries with reporting delays](https://package.epinowcast.org/articles/single-timeseries-rt-estimation.html) is a good example of joint nowcasting and reproduction number estimation from an aggregated time series of reference (outcome) and reporting counts.
+This example does not start from a line list but does contain information about using the package for transforming between line list and appropriately aggregated data.
+
+## Going further
+
+There are many other R packages that can be used for $R_t$ estimation, nowcasting and forecasting.
+We invite readers to suggest further additions on our [discussion board](https://github.com/nfidd/nfidd/discussions).
diff --git a/sessions/forecast-ensembles.qmd b/sessions/forecast-ensembles.qmd
new file mode 100644
index 0000000..76f225e
--- /dev/null
+++ b/sessions/forecast-ensembles.qmd
@@ -0,0 +1,776 @@
+---
+title: "Forecast ensembles"
+order: 8
+---
+
+# Introduction
+
+We can classify models along a spectrum by how much they include an understanding of underlying processes, or mechanisms; or whether they emphasise drawing from the data using a statistical approach.
+These different approaches all have different strength and weaknesses, and it is not clear a prior which one produces the best forecast in any given situation.
+One way to attempt to draw strength from a diversity of approaches is the creation of so-called *forecast ensembles* from the forecasts produced by different models.
+
+In this session, we'll start with forecasts from the models we explored in the [forecasting models](forecasting-models) session and build ensembles of these models.
+We will then compare the performance of these ensembles to the individual models and to each other.
+Rather than using the forecast samples we have been using we will instead now use quantile-based forecasts.
+
+::: {.callout-note collapse="true"}
+## Representations of probabilistic forecasts
+
+Probabilistic predictions can be described as coming from a probabilistic probability distributions.
+In general and when using complex models such as the one we discuss in this course, these distributions can not be expressed in a simple analytical formal as we can do if, e.g. talking about common probability distributions such as the normal or gamma distributions.
+Instead, we typically use a limited number of samples generated from Monte-Carlo methods to represent the predictive distribution.
+However, this is not the only way to characterise distributions.
+
+A quantile is the value that corresponds to a given quantile level of a distribution.
+For example, the median is the 50th quantile of a distribution, meaning that 50% of the values in the distribution are less than the median and 50% are greater.
+Similarly, the 90th quantile is the value that corresponds to 90% of the distribution being less than this value.
+If we characterise a predictive distribution by its quantiles, we specify these values at a range of specific quantile levels, e.g. from 5% to 95% in 5% steps.
+:::
+
+## Slides
+
+- [Introduction to ensembles](slides/introduction-to-ensembles)
+
+## Objectives
+
+The aim of this session is to introduce the concept of ensembles of forecasts and to evaluate the performance of ensembles of the models we explored in the [forecasting models](forecasting-models) session.
+
+::: {.callout-note collapse="true"}
+
+# Setup
+
+## Source file
+
+The source file of this session is located at `sessions/forecast-ensembles.qmd`.
+
+## Libraries used
+
+In this session we will use the `nfidd` package to load a data set of infection times and access stan models and helper functions, the `dplyr` and `tidyr` packages for data wrangling, `ggplot2` library for plotting, the `tidybayes` package for extracting results of the inference and the `scoringutils` package for evaluating forecasts.
+We will also use `qra` for quantile regression averaging in the weighted ensemble section.
+
+```{r libraries, message = FALSE}
+library("nfidd")
+library("dplyr")
+library("tidyr")
+library("ggplot2")
+library("scoringutils")
+library("qra")
+```
+
+::: {.callout-tip}
+The best way to interact with the material is via the [Visual Editor](https://docs.posit.co/ide/user/ide/guide/documents/visual-editor.html) of RStudio.
+:::
+
+## Initialisation
+
+We set a random seed for reproducibility.
+Setting this ensures that you should get exactly the same results on your computer as we do.
+
+```{r}
+set.seed(123)
+```
+
+:::
+
+# Individual forecast models
+
+In this session we will use the forecasts from the models we explored in the session on [forecasting models](forecasting-models). There all shared the same basic renewal with delays structure but used different models for the evolution of the effective reproduction number over time. These were:
+
+- A random walk model
+- A differenced autoregressive model referred to as "More statistical"
+- A simple model of susceptible depeltion referred to as "More mechanistic"
+
+For the purposes of this session the precise details of the models are not critical to the concepts we are exploring.
+
+As in the session on [forecasting concepts](forecasting-concepts), we have fitted these models to a range of forecast dates so you don't have to wait for the models to fit.
+We will now evaluate the forecasts from the mechanistic and statistical models.
+
+```{r load_forecasts}
+data(rw_forecasts, stat_forecasts, mech_forecasts)
+forecasts <- bind_rows(
+ rw_forecasts,
+ mutate(stat_forecasts, model = "More statistical"),
+ mutate(mech_forecasts, model = "More mechanistic")
+) |>
+ ungroup()
+
+forecasts
+```
+
+::: {.callout-tip collapse="true"}
+## How did we generate these forecasts?
+We generated these forecasts using the code in `data-raw/generate-example-forecasts.r` which uses the same approach we just took before for a single forecast date but generalises it to many forecast dates.
+
+Some important things to note about these forecasts:
+
+ - We used a 14 day forecast horizon.
+ - Each forecast used all the data up to the forecast date.
+ - We generated 1000 predictive posterior samples for each forecast.
+ - We started forecasting 3 weeks into the outbreak and then forecast every 7 days until the end of the data (excluding the last 14 days to allow a full forecast).
+ - We use the same simulated outbreak data as before:
+
+```{r}
+gen_time_pmf <- make_gen_time_pmf()
+ip_pmf <- make_ip_pmf()
+onset_df <- simulate_onsets(
+ make_daily_infections(infection_times), gen_time_pmf, ip_pmf
+)
+head(onset_df)
+```
+
+:::
+
+# Converting sample-based forecasts to quantile-based forecasts
+
+As in this session we will be thinking about forecasts in terms quantiles of the predictive distributions, we will need to convert our sample based forecasts to quantile-based forecasts.
+We will do this by focusing at the *marginal distribution* at each predicted time point, that is we treat each time point as independent of all others and calculate quantiles based on the sample predictive trajectories at that time point.
+An easy way to do this is to use the `{scoringutils}` package.
+The steps to do this are to first declare the forecasts as `sample` forecasts.
+
+```{r convert-for-scoringutils}
+sample_forecasts <- forecasts |>
+ left_join(onset_df, by = "day") |>
+ filter(!is.na(.value)) |>
+ as_forecast_sample(
+ forecast_unit = c("target_day", "horizon", "model", "day"),
+ observed = "onsets",
+ predicted = ".value",
+ sample_id = ".draw"
+ )
+sample_forecasts
+```
+
+and then convert to `quantile` forecasts.
+
+```{r convert-to-quantile}
+quantile_forecasts <- sample_forecasts |>
+ as_forecast_quantile()
+quantile_forecasts
+```
+
+::: {.callout-tip collapse="true"}
+## What is happening here?
+
+- Internally `scoringutils` is calculating the quantiles of the sample-based forecasts.
+- It does this by using a set of default quantiles but different ones can be specified by the user to override the default.
+- It then calls the `quantile()` function from base R to calculate the quantiles.
+- This is estimating the value that corresponds to each given quantile level by ordering the samples and then taking the value at the appropriate position.
+:::
+
+# Simple unweighted ensembles
+
+A good place to start when building ensembles is to take the mean or median of the unweighted forecast at each quantile level, and treat these as quantiles of the ensemble predictive distribution.
+Typically, the median is preferred when outlier forecasts are likely to be present as it is less sensitive to these.
+However, the mean is preferred when forecasters have more faith in models that diverge from the median performance and want to represent this in the ensemble.
+
+::: {.callout-note collapse="true"}
+## Vincent average
+
+The procedure of calculating quantiles of a new distribution as a weighted average of quantiles of constituent distributions (e.g., different measurements) is called a *Vincent average*, after the biologist Stella Vincent who described this as early as 1912 when studying the function of whiskers in the behaviour of white rats.
+
+:::
+
+
+## Construction
+
+We can calculate the mean ensemble by taking the mean of the forecasts at each quantile level.
+
+```{r mean-ensemble}
+mean_ensemble <- quantile_forecasts |>
+ as_tibble() |>
+ summarise(
+ predicted = mean(predicted),
+ observed = unique(observed),
+ model = "Mean ensemble",
+ .by = c(target_day, horizon, quantile_level, day)
+ )
+```
+
+Similarly, we can calculate the median ensemble by taking the median of the forecasts at each quantile level.
+
+```{r median-ensemble}
+median_ensemble <- quantile_forecasts |>
+ as_tibble() |>
+ summarise(
+ predicted = median(predicted),
+ observed = unique(observed),
+ model = "Median ensemble",
+ .by = c(target_day, horizon, quantile_level, day)
+ )
+```
+
+We combine the ensembles into a single data frame along with the individual forecasts in order to make visualisation easier.
+
+```{r combine-ensembles}
+simple_ensembles <- bind_rows(
+ mean_ensemble,
+ median_ensemble,
+ quantile_forecasts
+)
+```
+
+## Visualisation
+
+How do these ensembles visually differ from the individual models? Lets start by plotting a single forecast from each model and comparing them.
+
+```{r plot-single-forecasts}
+plot_ensembles <- function(data, obs_data) {
+ data |>
+ pivot_wider(names_from = quantile_level, values_from = predicted) |>
+ ggplot(aes(x = day)) +
+ geom_ribbon(
+ aes(
+ ymin = .data[["0.05"]], ymax = .data[["0.95"]], fill = model,
+ group = target_day
+ ),
+ alpha = 0.2
+ ) +
+ geom_ribbon(
+ aes(
+ ymin = .data[["0.25"]], ymax = .data[["0.75"]], fill = model,
+ group = target_day
+ ),
+ alpha = 0.5
+ ) +
+ geom_point(
+ data = obs_data,
+ aes(x = day, y = onsets), color = "black"
+ ) +
+ scale_color_binned(type = "viridis") +
+ facet_wrap(~model) +
+ theme(legend.position = "none")
+}
+
+plot_single_forecasts <- simple_ensembles |>
+ filter(target_day == 57) |>
+ plot_ensembles(onset_df |> filter(day >= 57, day <= 57 + 14))
+
+plot_single_forecasts
+```
+
+Again we can get a different perspective by plotting the forecasts on the log scale.
+
+```{r plot-single-forecasts-log}
+plot_single_forecasts +
+ scale_y_log10()
+```
+
+::: {.callout-tip}
+## Take 2 minutes
+How do these ensembles compare to the individual models?
+How do they differ from each other?
+:::
+
+::: {.callout-note collapse="true"}
+## Solution
+How do these ensembles compare to the individual models?
+
+- Both of the simple ensembles appear to be less variable than the statistical models but have more variable than the mechanistic model.
+- Both ensembles are more like the statistical models than the mechanistic model.
+
+How do they differ from each other?
+
+- The mean ensemble has slightly tighter uncertainty bounds than the median ensemble.
+:::
+
+Now lets plot a range of forecasts from each model and ensemble.
+
+```{r plot-multiple-forecasts}
+plot_multiple_forecasts <- simple_ensembles |>
+ plot_ensembles(onset_df |> filter(day >= 21)) +
+ lims(y = c(0, 400))
+
+plot_multiple_forecasts
+```
+
+Again we can get a different perspective by plotting the forecasts on the log scale.
+
+```{r plot-multiple-forecasts-log}
+plot_multiple_forecasts +
+ scale_y_log10()
+```
+
+
+::: {.callout-tip}
+## Take 2 minutes
+How do these ensembles compare to the individual models?
+
+How do they differ from each other?
+
+Are there any differences across forecast dates?
+:::
+
+::: {.callout-note collapse="true"}
+## Solution
+How do these ensembles compare to the individual models?
+
+- As before, the ensembles appear to be less variable than the statistical models but more variable than the mechanistic model.
+
+How do they differ from each other?
+
+- The mean ensemble has marginally tighter uncertainty bounds than the median ensemble as for the single forecast.
+
+Are there any differences across forecast dates?
+
+- The mean ensemble appears to be more variable across forecast dates than the median ensemble with this being more pronounced after the peak of the outbreak.
+:::
+
+## Evaluation
+
+As in the [forecasting concepts session](forecasting-concepts), we can evaluate the accuracy of the ensembles using the `{scoringutils}` package and in particular the `score()` function.
+
+```{r score-ensembles}
+ensemble_scores <- simple_ensembles |>
+ as_forecast_quantile(forecast_unit = c("target_day", "horizon", "model")) |>
+ score()
+```
+
+Again we start with a high level overview of the scores by model.
+
+```{r score-overview}
+ensemble_scores |>
+ summarise_scores(by = c("model"))
+```
+
+::: {.callout-tip}
+## Take 5 minutes
+What do you think the scores are telling you? Which model do you think is best?
+What other scoring breakdowns might you want to look at?
+:::
+
+::: {.callout-note collapse="true"}
+## Solution
+What do you think the scores are telling you? Which model do you think is best?
+
+- The mean ensemble appears to be the best performing ensemble model overall.
+- However, the more mechanistic model appears to be the best performing model overall.
+
+What other scoring breakdowns might you want to look at?
+
+- There might be variation over forecast dates or horizons between the different ensemble methods
+:::
+
+# Unweighted ensembles of filtered models
+
+A simple method that is often used to improve ensemble performance is to prune out models that perform very poorly.
+Balancing this can be tricky however as it can be hard to know how much to prune.
+The key tradeoff to consider is how much to optimise for which models have performed well in the past (and what your definition of the past is, for example all time or only the last few weeks) versus how much you want to allow for the possibility that these models may not perform well in the future.
+
+## Construction
+
+As we just saw, the random walk model (our original baseline model) is performing poorly in comparison to the other models.
+We can remove this model from the ensemble and see if this improves the performance of the ensemble.
+
+::: {.callout-warning}
+## Warning
+
+Here we are technically cheating a little as we are using the test data to help select the models to include in the ensemble.
+In the real world you would not do this as you would not have access to the test data and so this is an idealised scenario.
+:::
+
+```{r remove-rw}
+filtered_models <- quantile_forecasts |>
+ filter(model != "Random walk")
+```
+
+We then need to recalculate the ensembles. First the mean ensemble,
+
+```{r recalculate-ensembles}
+filtered_mean_ensembles <- filtered_models |>
+ as_tibble() |>
+ summarise(
+ predicted = mean(predicted),
+ observed = unique(observed),
+ model = "Mean filtered ensemble",
+ .by = c(target_day, horizon, quantile_level, day)
+ )
+```
+
+and then the median ensemble.
+
+```{r recalculate-median-ensemble}
+filtered_median_ensembles <- filtered_models |>
+ as_tibble() |>
+ summarise(
+ predicted = median(predicted),
+ observed = unique(observed),
+ model = "Median filtered ensemble",
+ .by = c(target_day, horizon, quantile_level, day)
+ )
+```
+
+We combine these new ensembles with our previous ensembles in order to make visualisation easier.
+
+```{r combine-filtered-ensembles}
+filtered_ensembles <- bind_rows(
+ filtered_mean_ensembles,
+ filtered_median_ensembles,
+ simple_ensembles
+)
+```
+
+## Visualisation
+
+As for the simple ensembles, we can plot a single forecast from each model and ensemble.
+
+```{r plot-single-filtered-forecasts}
+filtered_ensembles |>
+ filter(target_day == 57) |>
+ plot_ensembles(onset_df |> filter(day >= 57, day <= 57 + 14))
+```
+
+and on the log scale.
+
+```{r plot-single-filtered-forecasts-log}
+filtered_ensembles |>
+ filter(target_day == 57) |>
+ plot_ensembles(onset_df |> filter(day >= 57, day <= 57 + 14)) +
+ scale_y_log10()
+```
+
+To get an overview we also plot a range of forecasts from each model and ensemble.
+
+```{r plot-multiple-filtered-forecasts}
+plot_multiple_filtered_forecasts <- filtered_ensembles |>
+ plot_ensembles(onset_df |> filter(day >= 21)) +
+ lims(y = c(0, 400))
+plot_multiple_filtered_forecasts
+```
+
+and on the log scale.
+
+```{r plot-multiple-filtered-forecasts-log}
+plot_multiple_filtered_forecasts +
+ scale_y_log10()
+```
+
+::: {.callout-tip}
+## Take 2 minutes
+How do the filtered ensembles compare to the simple ensembles?
+Which do you think is best?
+:::
+
+::: {.callout-note collapse="true"}
+## Solution
+How do the filtered ensembles compare to the simple ensembles?
+
+- The filtered ensembles appear to be less variable than the simple ensembles.
+- The filtered ensembles appear to be more like the mechanistic model than the simple ensembles.
+
+Which do you think is best?
+
+- Visually, the filtered ensembles appear very similar. This makes sense given we know there are only two models left in the ensemble.
+
+:::
+
+## Evaluation
+
+Let us score the filtered ensembles.
+
+```{r score-filtered-ensembles}
+filtered_ensemble_scores <- filtered_ensembles |>
+ as_forecast_quantile(
+ forecast_unit = c(
+ "target_day", "horizon", "model"
+ )
+ ) |>
+ score()
+```
+
+Again we can get a high level overview of the scores by model.
+
+```{r score-overview-filtered}
+filtered_ensemble_scores |>
+ summarise_scores(by = c("model"))
+```
+
+::: {.callout-tip}
+## Take 2 minutes
+How do the filtered ensembles compare to the simple ensembles?
+:::
+
+::: {.callout-note collapse="true"}
+## Solution
+How do the filtered ensembles compare to the simple ensembles?
+
+- The filtered ensembles appear to be more accurate than the simple ensembles.
+- As you would expect they are an average of the more mechanistic model and the more statistical model.
+- As there are only two models in the ensemble, the median and mean ensembles are identical.
+- For the first time there are features of the ensemble that outperform the more mechanistic model though it remains the best performing model overall.
+:::
+
+# Weighted quantile ensembles
+
+The simple mean and median we used to average quantiles earlier treats every model as the same.
+We could try to improve performance by replacing this with a weighted mean (or weighted median), for example given greater weight to models that have proven to make better forecasts.
+Here we will explore two common weighting methods:
+
+- Inverse WIS weighting
+- Quantile regression averaging
+
+Inverse WIS weighting is a simple method that weights the forecasts by the inverse of their WIS over some period (note that identifying what this period should be in order to produce the best forecasts is not straightforward as predictive performance may vary over time if models are good at different things).
+The main benefit of WIS weighting over other methods is that it is simple to understand and implement.
+However, it does not optimise the weights directly to produce the best forecasts.
+It relies on the hope that giving more weight to better performing models yields a better ensemble
+
+Quantile regression averaging on the other hand does optimise the weights directly in order to yield the best scores on past data.
+
+## Construction
+
+### Inverse WIS weighting
+
+In order to perform inverse WIS weighting we first need to calculate the WIS for each model. We already have this from the previous evaluation so we can reuse this.
+
+```{r calc-wis}
+weights_per_model <- ensemble_scores |>
+ dplyr::filter(
+ model %in% c("More mechanistic", "More statistical", "Random walk")
+ ) |>
+ summarise_scores(by = c("model", "target_day")) |>
+ select(model, target_day, wis) |>
+ mutate(inv_wis = 1 / wis) |>
+ mutate(
+ inv_wis_total_by_date = sum(inv_wis), .by = target_day
+ ) |>
+ mutate(weights = inv_wis / inv_wis_total_by_date)
+
+weights_per_model |>
+ select(model, target_day, weights) |>
+ pivot_wider(names_from = model, values_from = weights)
+```
+
+Now lets apply the weights to the forecast models. As we can only use information that was available at the time of the forecast to perform the weighting, we use weights from two weeks prior to the forecast date to inform each ensemble.
+
+```{r apply-weights}
+inverse_wis_ensemble <- quantile_forecasts |>
+ as_tibble() |>
+ left_join(
+ weights_per_model |>
+ mutate(target_day = target_day + 14),
+ by = c("model", "target_day")
+ ) |>
+ # assign equal weights if no weights are available
+ mutate(weights = ifelse(is.na(weights), 1/3, weights)) |>
+ summarise(
+ predicted = sum(predicted * weights),
+ observed = unique(observed),
+ model = "Inverse WIS ensemble",
+ .by = c(target_day, horizon, quantile_level, day)
+ )
+```
+
+### Quantile regression averaging
+
+We futher to perform quantile regression averaging (QRA) for each forecast date.
+Again we need to consider how many previous forecasts we wish to use to inform each ensemble forecast.
+Here we decide to use up to 3 weeks of previous forecasts to inform each QRA ensemble.
+
+```{r qra}
+forecast_dates <- quantile_forecasts |>
+ as_tibble() |>
+ pull(target_day) |>
+ unique()
+
+qra_by_forecast <- function(
+ quantile_forecasts,
+ forecast_dates,
+ group = c("target_end_date"),
+ ...
+) {
+ lapply(forecast_dates, \(x) {
+ quantile_forecasts |>
+ mutate(target_end_date = x) |>
+ dplyr::filter(target_day <= x) |>
+ dplyr::filter(target_day >= x - (3 * 7 + 1)) |>
+ dplyr::filter(target_day == x | day <= x) |>
+ qra(
+ group = group,
+ target = c(target_day = x),
+ ...
+ )
+ })
+}
+
+qra_ensembles_obj <- qra_by_forecast(
+ quantile_forecasts,
+ forecast_dates[-1],
+ group = c("target_end_date")
+)
+
+qra_weights <- seq_along(qra_ensembles_obj) |>
+ lapply(\(i) attr(qra_ensembles_obj[[i]], "weights") |>
+ mutate(target_day = forecast_dates[i + 1])
+ ) |>
+ bind_rows() |>
+ dplyr::filter(quantile == 0.5)
+
+qra_ensembles <- qra_ensembles_obj |>
+ bind_rows()
+```
+
+Instead of creating a single optimised ensemble and using this for all forecast horizons we might also want to consider a separate optimised QRA ensemble for each forecast horizon, reflecting that models might perform differently depending on how far ahead a forecast is produced.
+We can do this using `qra()` with the `group` argument.
+
+```{r qra-by-horizon}
+qra_ensembles_by_horizon <- qra_by_forecast(
+ quantile_forecasts,
+ forecast_dates[-c(1:2)],
+ group = c("horizon", "target_end_date"),
+ model = "QRA by horizon"
+)
+
+qra_weights_by_horizon <- seq_along(qra_ensembles_by_horizon) |>
+ lapply(\(i) attr(qra_ensembles_by_horizon[[i]], "weights") |>
+ mutate(target_day = forecast_dates[i + 2])
+ ) |>
+ bind_rows()
+```
+
+### Combine ensembles
+
+```{r combine-inverse-wis-ensemble}
+weighted_ensembles <- bind_rows(
+ inverse_wis_ensemble,
+ qra_ensembles,
+ qra_ensembles_by_horizon,
+ filtered_ensembles
+) |>
+ # remove the repeated filtered ensemble
+ filter(model != "Mean filtered ensemble")
+```
+
+## Visualisation
+
+### Single forecasts
+
+Again we start by plotting a single forecast from each model and ensemble.
+
+```{r plot-single-weighted-forecasts}
+weighted_ensembles |>
+ filter(target_day == 57) |>
+ plot_ensembles(onset_df |> filter(day >= 57, day <= 57 + 14))
+```
+
+and on the log scale.
+
+```{r plot-single-weighted-forecasts-log}
+weighted_ensembles |>
+ filter(target_day == 57) |>
+ plot_ensembles(onset_df |> filter(day >= 57, day <= 57 + 14)) +
+ scale_y_log10()
+```
+
+### Multiple forecasts
+
+As before we can plot a range of forecasts from each model and ensemble.
+
+```{r plot-multiple-weighted-forecasts}
+plot_multiple_weighted_forecasts <- weighted_ensembles |>
+ plot_ensembles(onset_df |> filter(day >= 21)) +
+ lims(y = c(0, 400))
+plot_multiple_weighted_forecasts
+```
+
+and on the log scale.
+
+```{r plot-multiple-weighted-forecasts-log}
+plot_multiple_weighted_forecasts +
+ scale_y_log10()
+```
+
+::: {.callout-tip}
+## Take 2 minutes
+How do the weighted ensembles compare to the simple ensembles?
+Which do you think is best?
+Are you surprised by the results? Can you think of any reasons that would explain them?
+:::
+
+::: {.callout-note collapse="true"}
+## Solution
+How do the weighted ensembles compare to the simple ensembles?
+
+:::
+
+### Models weights
+
+Now that we have a weighted ensemble, we can also look at the weights of the individual models. Here we do this for the quantile regression averaging ensemble but we could also do this for the inverse WIS ensemble or any other weighted ensemble (for an unweighted ensemble the weights are all equal).
+
+```{r plot-model-weights}
+qra_weights |>
+ ggplot(aes(x = target_day, y = weight, fill = model)) +
+ geom_col(position = "stack") +
+ theme(legend.position = "bottom")
+```
+
+::: {.callout-tip}
+## Take 2 minutes
+How do the weights change over time?
+Are you surprised by the results given what you know about the models performance?
+:::
+
+::: {.callout-note collapse="true"}
+## Solution
+How do the weights change over time?
+
+- Early on the more statistical models have higher weights
+- Gradually the random walk model gains weight and by the end of the forecast horizon it represents the entire ensemble.
+- Near the peak the mechanistic model also gains weight.
+
+Are you surprised by the results given what you know about the models performance?
+
+- As the random walk model is performing poorly, you would expect it to have low weights but actually it often doesn't.
+ This implies that its poor performance is restricted to certain parts of the outbreak.
+- Even though the mechanistic model performs really well overall it is only included in the ensemble around the peak.
+ This could be because the training data doesn't include changes in the epidemic dynamics and so the mechanistic model is not given sufficient weight.
+:::
+
+## Evaluation
+
+For a final evaluation we can look at the scores for each model and ensemble again.
+We remove the two weeks of forecasts as these do not have a quantile regression average forecasts as these require training data to estimate.
+
+```{r score-weighted-ensembles}
+weighted_ensemble_scores <- weighted_ensembles |>
+ filter(target_day >= 29) |>
+ as_forecast_quantile(forecast_unit = c("target_day", "horizon", "model")) |>
+ score()
+```
+
+Again we can get a high level overview of the scores by model.
+
+```{r score-overview-weighted}
+weighted_ensemble_scores |>
+ summarise_scores(by = c("model"))
+```
+
+Remembering the session on [forecasting concepts](forecasting-concepts), we should also check performance on the log scale.
+
+```{r score-overview-weighted-log}
+log_ensemble_scores <- weighted_ensembles |>
+ filter(target_day >= 29) |>
+ as_forecast_quantile(forecast_unit = c("target_day", "horizon", "model")) |>
+ transform_forecasts(
+ fun = log_shift,
+ offset = 1,
+ append = FALSE
+ ) |>
+ score()
+
+log_ensemble_scores |>
+ summarise_scores(by = c("model"))
+```
+
+::: {.callout-tip}
+## Take 2 minutes
+How do the weighted ensembles compare to the simple ensembles on the natural and log scale?
+:::
+
+::: {.callout-note collapse="true"}
+## Solution
+How do the weighted ensembles compare to the simple ensembles on the natural and log scale?
+:::
+
+# Going further
+
+
+# Wrap up
diff --git a/sessions/forecasting-concepts.qmd b/sessions/forecasting-concepts.qmd
new file mode 100644
index 0000000..998c199
--- /dev/null
+++ b/sessions/forecasting-concepts.qmd
@@ -0,0 +1,773 @@
+---
+title: "Forecasting concepts"
+order: 7
+---
+
+# Introduction
+
+So far we've looked at epidemiological processes and events up until the present. Now we'll start to forecast into the future, while considering what we might want from a "good" forecast. After enough time has passed to observe events, we can then retrospectively evaluate how well forecasts performed. We'll introduce evaluating forecasts qualitatively by visual inspection, and quantitatively using scoring metrics.
+
+## Slides
+
+- [Forecasting as an epidemiological problem](slides/forecasting-as-an-epidemiological-problem)
+
+## Objectives
+
+The aim of this session is to introduce the concept of forecasting, using a simple model, and forecasting evaluation.
+
+::: {.callout-note collapse="true"}
+
+# Setup
+
+## Source file
+
+The source file of this session is located at `sessions/forecasting-concepts.qmd`.
+
+## Libraries used
+
+In this session we will use the `nfidd` package to load a data set of infection times and access stan models and helper functions, the `dplyr` and `tidyr` packages for data wrangling, `ggplot2` library for plotting, the `tidybayes` package for extracting results of the inference and the `scoringutils` package for evaluating forecasts.
+
+```{r libraries, message = FALSE}
+library("nfidd")
+library("dplyr")
+library("tidyr")
+library("ggplot2")
+library("tidybayes")
+library("scoringutils")
+```
+
+::: {.callout-tip}
+The best way to interact with the material is via the [Visual Editor](https://docs.posit.co/ide/user/ide/guide/documents/visual-editor.html) of RStudio.
+:::
+
+## Initialisation
+
+We set a random seed for reproducibility.
+Setting this ensures that you should get exactly the same results on your computer as we do.
+We also set an option that makes `cmdstanr` show line numbers when printing model code.
+This is not strictly necessary but will help us talk about the models.
+
+```{r}
+set.seed(123)
+options(cmdstanr_print_line_numbers = TRUE)
+```
+
+:::
+
+# What is forecasting?
+
+Forecasting is the process of making predictions about the future based on past and present data.
+In the context of infectious disease epidemiology, forecasting is usually the process of predicting the future course of some metric of infectious disease incidence or prevalence based on past and present data.
+Here we focus on forecasting observed data (the number of individuals with new symptom onset) but forecasts can also be made for other quantities of interest such as the number of infections, the reproduction number, or the number of deaths.
+Epidemiological forecasting is closely related to nowcasting and, when using mechanistic approaches, estimation of the reproduction number. In fact, the model we will use for forecasting is the same as the model we used for nowcasting and estimation of the reproduction number.
+The only difference is that we will extend the model into the future.
+In order to make things simpler we will remove the nowcasting part of the model, but in principle all these approaches could be combined in a single model.
+
+::: {.callout-tip}
+## What do we look for in a good forecast? Some food for thought:
+1. **Calibration**: The forecast should be well calibrated. This means that the forecasted probabilities should match the observed frequencies. For example, if the model predicts a 50% probability of an event occurring, then the event should occur approximately 50% of the time.
+2. **Unbiasedness**: The forecast should be unbiased. This means that the average forecasted value should be equal to the average observed value. It shouldn't consistently over- or underpredict.
+3. **Accuracy**: The forecast should be accurate. This means that the forecasted values should be close to the observed values.
+4. **Sharpness**: As long as the other conditions are fulfilled we want prediction intervals to be as narrow as possible. Predicting that "anything can happen" might be correct but not very useful.
+:::
+
+
+# Extending a model into the future
+
+The model we introduced in the [renewal equation session](R-estimation-and-the-renewal-equation) the reproduction number using a random walk, then used a discrete renewal process to model the number of infections, and convolved these with a delay distribution to model the number of onsets with Poisson observation error.
+Based on what we found in the [nowcasting session](nowcasting) this seems like a reasonable model for the data and so we might want to use it to forecast into the future.
+
+We can do this by simulating the generative model forward in time.
+To do this we use the same model as in the renewal equation session but add a `generated quantities` block to create the forecasts.
+This is used for in a stan model for any calculations that only depend on other parameter estimates but are not themselves constrained by the data.
+So what does this look like in code? Lets load in the model again and take a look.
+
+```{r load_model}
+mod <- nfidd_cmdstan_model("estimate-inf-and-r-rw-forecast")
+mod
+```
+
+::: .callout-tip
+## Take 5 minutes
+What have we changed in the model to make it a forecasting model?
+Do you see any limitations of this approach?
+:::
+
+::: {.callout-note collapse="true"}
+## Solution
+- What have we changed in the model to make it a forecasting model?
+ - Added the `h` parameter to the data list to specify the number of days to forecast into the future.
+ - Added the `m` parameter as a piece of `transformed data` (i.e. a calculation that only uses data) that is the total number of days to include in the model (i.e. the number of days in the data plus the number of days to forecast).
+ - `m` is then used in all arrays in the model rather than `n`. This means that `rw_noise` is now `m - 1` long, and `R`, `onsets`, `infections` and `onsets` are `m` long.
+ - As there are only `n` observations in the data in the likelihood we only use the first `n` elements of `onsets`.
+ - To include observation error in the forecast a `generated quantities` block has been added which takes the last `h` onsets as the mean of a Poisson distribution and samples from this distribution to get the forecasted onsets.
+- Do you see any limitations of this approach?
+ - Including `h` in the `parameters` and `model` blocks increases the number of parameters and amount of work we have to do when fitting the model.
+ It would be more computationally efficient to have a separate model for forecasting.
+:::
+
+Before we can forecast we need some data to fit the model to.
+In order to assess the quality of the forecasts we will also need some future (or hold-out) data that we can compare the forecasts to.
+We will use the same simulated data as in the renewal and nowcasting sessions.
+We will try to make a forecast on day 71 (assuming we don't know what the data looks like after that day) as in the nowcasting session.
+
+```{r, load-simulated-onset}
+gen_time_pmf <- make_gen_time_pmf()
+ip_pmf <- make_ip_pmf()
+onset_df <- simulate_onsets(
+ make_daily_infections(infection_times), gen_time_pmf, ip_pmf
+)
+cutoff <- 71
+filtered_onset_df <- onset_df |>
+ filter(day <= cutoff)
+tail(onset_df)
+```
+
+## Fitting the model and forecast for 28 days into the future
+
+We can now fit the model to the data and then make a forecast.
+This should look very similar to the code we used in the renewal session but with the addition of a non-zero `h` in the data list.
+
+```{r fit_model, results = 'hide', message = FALSE}
+horizon <- 28
+
+data <- list(
+ n = nrow(filtered_onset_df),
+ I0 = 1,
+ obs = filtered_onset_df$onsets,
+ gen_time_max = length(gen_time_pmf),
+ gen_time_pmf = gen_time_pmf,
+ ip_max = length(ip_pmf) - 1,
+ ip_pmf = ip_pmf,
+ h = horizon # Here we set the number of days to forecast into the future
+)
+rw_forecast <- mod$sample(
+ data = data, parallel_chains = 4, adapt_delta = 0.95,
+ init = \() list(init_R = 0, rw_sd = 0.01)
+)
+```
+
+```{r rw_forecast_summary}
+rw_forecast
+```
+
+::: callout-note
+Because this model can struggle to fit to the data, we have increased the value of `adapt_delta` from its default value of 0.8.
+This is a tuning parameter that affects the step size of the sampler in exploring the posterior (higher `adapt_delta` leading to smaller step sizes meaning posterior exploration is slower but more careful).
+:::
+
+## Visualising the forecast
+
+We can now visualise the forecast.
+We will first extract the forecast and then plot the forecasted number of symptom onsets alongside the observed number of symptom onsets.
+
+```{r extract-forecast}
+forecast <- rw_forecast |>
+ gather_draws(forecast[day]) |>
+ ungroup() |>
+ mutate(day = day + cutoff)
+
+target_onsets <- onset_df |>
+ filter(day > cutoff) |>
+ filter(day <= cutoff + horizon)
+```
+
+```{r plot_forecast}
+forecast |>
+ filter(.draw %in% sample(.draw, 100)) |>
+ ggplot(aes(x = day)) +
+ geom_line(alpha = 0.1, aes(y = .value, group = .draw)) +
+ geom_point(data = target_onsets, aes(x = day, y = onsets), color = "black") +
+ labs(
+ title = "Symptom onsets",
+ subtitle = "Forecast (trajectories) and observed (points)"
+ )
+```
+
+::: {.callout-tip}
+## Take 5 minutes
+What do you think of this forecast?
+Did the model do a good job?
+Is there another way you could visualise the forecast that might be more informative?
+:::
+
+::: {.callout-note collapse="true"}
+## Solution
+- On the face of it the forecast looks very poor with some very high predictions compared to the data.
+- Based on this visualisation it is hard to tell if the model is doing a good job but it seems like it is not.
+- As outbreaks are generally considered to be exponential processes it might be more informative to plot the forecast on the log scale.
+
+```{r plot_forecast_log}
+forecast |>
+ filter(.draw %in% sample(.draw, 100)) |>
+ ggplot(aes(x = day)) +
+ geom_line(alpha = 0.1, aes(y = .value, group = .draw)) +
+ geom_point(data = target_onsets, aes(x = day, y = onsets), color = "black") +
+ scale_y_log10() +
+ labs(title = "Symptom onsets, log scale", subtitle = "Forecast and observed")
+```
+
+This should be a lot more informative.
+We see that for longer forecast horizons the model is not doing a great job of capturing the reduction in symptom onsets.
+However, we can now see that the model seems to be producing very reasonable forecasts for the first week or so of the forecast.
+This is a common pattern in forecasting where a model is good at capturing the short term dynamics but struggles with the longer term dynamics.
+:::
+
+As our forecasting model is based on the reproduction number, we can also visualise the forecast of the reproduction number.
+This can be helpful for understanding why our forecasts of symptom onsets look the way they do and for understanding the uncertainty in the forecasts.
+We can also compare this to the "true" reproduction number, estimated once all relevant data is available. To do this, we will fit the model again but with a later cutoff. Then we can compare the reproduction numbers produced as *forecasts* at the earlier time, with *estimates* at the later time that used more of the data.
+
+```{r fit_model_long, results = 'hide', message = FALSE}
+long_onset_df <- onset_df |>
+ filter(day <= cutoff + horizon)
+
+long_data <- list(
+ n = nrow(long_onset_df),
+ I0 = 1,
+ obs = long_onset_df$onsets,
+ gen_time_max = length(gen_time_pmf),
+ gen_time_pmf = gen_time_pmf,
+ ip_max = length(ip_pmf) - 1,
+ ip_pmf = ip_pmf,
+ h = 0
+)
+
+
+rw_long <- mod$sample(
+ data = long_data, parallel_chains = 4, adapt_delta = 0.95,
+ init = \() list(init_R = 0, rw_sd = 0.01)
+)
+```
+
+We first need to extract the forecast and estimated reproduction numbers.
+
+```{r extract-Rt}
+forecast_r <- rw_forecast |>
+ gather_draws(R[day]) |>
+ ungroup() |>
+ mutate(type = "forecast")
+
+long_r <- rw_long |>
+ gather_draws(R[day]) |>
+ ungroup() |>
+ mutate(type = "estimate")
+```
+
+We can now plot the forecast and estimated reproduction numbers.
+
+```{r plot-Rt}
+forecast_r |>
+ bind_rows(long_r) |>
+ filter(.draw %in% sample(.draw, 100)) |>
+ ggplot(aes(x = day)) +
+ geom_vline(xintercept = cutoff, linetype = "dashed") +
+ geom_hline(yintercept = 1, linetype = "dashed") +
+ geom_line(
+ aes(y = .value, group = interaction(.draw, type), color = type),
+ alpha = 0.1
+ ) +
+ labs(
+ title = "Estimated R",
+ subtitle = "Estimated over whole time series (red), and forecast (blue)"
+ ) +
+ guides(colour = guide_legend(override.aes = list(alpha = 1)))
+```
+
+::: {.callout-note}
+The horizontal dashed line at 1 represents the threshold for epidemic growth. If the reproduction number is above 1 then the epidemic is growing, if it is below 1 then the epidemic is shrinking.
+The vertical dashed line represents the point at which we started forecasting.
+:::
+
+::: {.callout-tip}
+## Take 5 minutes
+Can you use this plot to explain why the forecast of onsets looks the way it does?
+:::
+
+::: {.callout-note collapse="true"}
+## Solution
+- When both models are being fit to data (i.e before the vertical dashed line) the forecast and estimated reproduction numbers are very similar.
+- For short-term forecasts $R_t$ estimates continue to be fairly similar.
+- However, the estimates have a consistent downwards trend which is not captured by the forecast (which looks like it has a constant mean value with increasing uncertainty).
+- This explains the divergence between the forecast and the data as the horizon increases.
+- It looks like only a relatively small number of forecast $R_t$ trajectories grow to be very large but these are enough to visually dominate the forecast of onsets on the natural scale.
+- The performance we are seeing here makes sense given that random walks are defined to have a constant mean and increasing variance.
+:::
+
+We managed to learn quite a lot about our model's forecasting limitations just looking at a single forecast using visualisations. However, what if we wanted to quantify how well the model is doing? This is where forecast evaluation comes in which we will cover in the next section.
+
+# Introduction to forecast evaluation
+
+An important aspect of making forecasts is that we can later confront the forecasts with what really happened and use this to assess whether our forecast model makes good predictions, or which of multiple models work best in which situation.
+In this section you will get to know several ways of assessing different aspects of forecast performance.
+You will then use them to evaluate your forecasts.
+
+# Evaluate your forecast
+
+In order to properly evaluate forecasts from this model we really need to forecast over a period of time. Ideally, capturing different epidemic dynamics. This will also give us more to work with when using scoring metrics. We will now load in some forecasts we made earlier and evaluate them.
+
+```{r load_forecasts}
+data(rw_forecasts)
+rw_forecasts |>
+ ungroup()
+```
+
+
+::: {.callout-tip}
+We generated these forecasts using the code in `data-raw/generate-example-forecasts.r` which uses the same approach we just took for a single forecast date but generalises it to many forecasts dates.
+
+Some important things to note about these forecasts:
+
+- We used a 14 day forecast horizon.
+- Each forecast used all the data up to the forecast date.
+- We generated 1000 posterior samples for each forecast.
+- We started forecasting 3 weeks into the outbreak, and then forecast once a week (every 7 days), i.e., we created forecasts on day 22, day 29, ... to day 71. We excluded the last 14 days to allow a full forecast.
+- We used the outbreak data simulated in the same way as the one we've been using through the course:
+
+```{r}
+head(onset_df)
+```
+
+:::
+
+## Visualising your forecast
+
+As for a single forecast, our first step is to visualise the forecasts as this can give us a good idea of how well the model is doing without having to calculate any metrics.
+
+```{r plot-all-forecasts}
+rw_forecasts |>
+ filter(.draw %in% sample(.draw, 100)) |>
+ ggplot(aes(x = day)) +
+ geom_line(
+ aes(y = .value, group = interaction(.draw, target_day), col = target_day),
+ alpha = 0.1
+ ) +
+ geom_point(
+ data = onset_df |>
+ filter(day >= 21),
+ aes(x = day, y = onsets), color = "black") +
+ scale_color_binned(type = "viridis") +
+ labs(title = "Weekly forecasts of symptom onsets over an outbreak",
+ col = "Forecast start day")
+```
+
+
+As for the single forecast it may be helpful to also plot the forecast on the log scale.
+
+```{r plot-all-forecasts-log}
+rw_forecasts |>
+ filter(.draw %in% sample(.draw, 100)) |>
+ ggplot(aes(x = day)) +
+ geom_line(
+ aes(y = .value, group = interaction(.draw, target_day), col = target_day),
+ alpha = 0.1
+ ) +
+ geom_point(data = onset_df, aes(x = day, y = onsets), color = "black") +
+ scale_y_log10() +
+ scale_color_binned(type = "viridis") +
+ labs(title = "Weekly symptom onset forecasts: log scale",
+ col = "Forecast start day")
+```
+
+::: {.callout-tip}
+## Take 5 minutes
+What do you think of these forecasts?
+Are they any good?
+How well do they capture changes in trend?
+Does the uncertainty seem reasonable?
+Do they seem to under or over predict consistently?
+Would you visualise the forecast in a different way?
+:::
+
+::: {.callout-note collapse="true"}
+## Solution
+ - We think these forecasts are a reasonable place to start but there is definitely room for improvement.
+Are they any good?
+ - They seem to do a reasonable job of capturing the short term dynamics but struggle with the longer term dynamics.
+How well do they capture changes in trend?
+ - There is little evidence of the model capturing the reduction in onsets before it begins to show in the data.
+Does the uncertainty seem reasonable?
+ - On the natural scale it looks like the model often over predicts. Things seem more balanced on the log scale but the model still seems to be overly uncertain.
+Do they seem to under or over predict consistently?
+ - It looks like the model is consistently over predicting on the natural scale but this is less clear on the log scale.
+:::
+
+## Scoring your forecast
+
+On top of visualising the forecasts, we can also summarise performance quantitatively by transforming them using scoring metrics.
+Whilst some of these metrics are more useful for comparing models, many can be also be useful for understanding the performance of a single model.
+We will look at some of these metrics in the next section.
+
+::: {.callout-tip}
+In this session, we'll use "proper" scoring rules: these are scoring rules that make sure no model can get better scores than the *true* model, i.e. the model used to generate the data.
+Of course we usually don't know this (as we don't know the "true model" for real-world data) but proper scoring rules incentivise forecasters to make their best attempt at reproducing its behaviour.
+:::
+
+We will use the [`{scoringutils}`](https://epiforecasts.io/scoringutils/dev/) package to calculate these metrics.
+Our first step is to convert our forecasts into a format that the `{scoringutils}` package can use.
+We will use `as_forecast_sample()` to do this:
+
+```{r convert-forecasts}
+sc_forecasts <- rw_forecasts |>
+ left_join(onset_df, by = "day") |>
+ filter(!is.na(.value)) |>
+ as_forecast_sample(
+ forecast_unit = c(
+ "target_day", "horizon", "model"
+ ),
+ observed = "onsets",
+ predicted = ".value",
+ sample_id = ".draw"
+ )
+sc_forecasts
+```
+
+As you can see this has created a `forecast` object which has a print method that summarises the forecasts.
+
+
+::: {.callout-tip}
+## Take 2 minutes
+What important information is in the `forecast` object?
+:::
+
+::: {.callout-note collapse="true"}
+## Solution
+ - The forecast unit which is the target day, horizon, and model
+ - The type of forecast which is a sample forecast
+:::
+
+Everything seems to be in order. We can now use the `scoringutils` package to calculate some metrics. We will use the default sample metrics (as our forecasts are in sample format) and score our forecasts.
+
+```{r score-forecasts}
+sc_scores <- sc_forecasts |>
+ score()
+
+sc_scores
+```
+
+::: {.callout-note collapse="true"}
+## Learning more about the output of `score()`
+
+See the documentation for `?metrics_sample` for information on the default metrics for forecasts that are represented as samples (in our case the samples generated by the stan model).
+:::
+
+### At a glance
+
+Before we look in detail at the scores, we can use `summarise_scores` to get a quick overview of the scores. Don't worry if you don't understand all the scores yet, we will go some of them in more detail in the next section and you can find more information in the [`{scoringutils}` documentation](https://epiforecasts.io/scoringutils/dev).
+
+```{r}
+sc_scores |>
+ summarise_scores(by = "model")
+```
+
+::: {.callout-tip}
+## Take 2 minutes
+Before we look in detail at the scores, what do you think the scores are telling you?
+:::
+
+### Continuous ranked probability score
+
+#### What is the Continuous Ranked Probability Score (CRPS)?
+
+The Continuous Ranked Probability Score (CRPS) is a proper scoring rule used to evaluate the accuracy of probabilistic forecasts. It is a generalization of the Mean Absolute Error (MAE) to probabilistic forecasts, where the forecast is a distribution rather than a single point estimate (i.e. like ours).
+
+The CRPS can be thought about as the combination of two key aspects of forecasting:
+1. The accuracy of the forecast in terms of how close the predicted values are to the observed value.
+2. The confidence of the forecast in terms of the spread of the predicted values.
+
+By balancing these two aspects, the CRPS provides a comprehensive measure of the quality of probabilistic forecasts.
+
+::: {.callout-tip}
+## Key things to note about the CRPS
+ - Small values are better
+ - As it is an absolute scoring rule it can be difficult to use to compare forecasts across scales.
+:::
+
+
+::: {.callout-tip collapse="true"}
+#### Mathematical Definition (optional)
+For distributions with a finite first moment (a mean exists and it is finite), the CRPS can be expressed as:
+
+$$
+CRPS(D, y) = \mathbb{E}_{X \sim D}[|X - y|] - \frac{1}{2} \mathbb{E}_{X, X' \sim D}[|X - X'|]
+$$
+
+where $X$ and $X'$ are independent random variables sampled from the distribution $D$. To calculate this we simpley replace $X$ and $X'$ by samples from our posterior distribution and sum over all possible combinations.
+
+This equation can be broke down into the two components:
+
+##### Breakdown of the Components
+
+1. **Expected Absolute Error Between Forecast and Observation**: $\mathbb{E}_{X \sim D}[|X - y|]$
+ This term represents the average absolute difference between the values predicted by the forecasted distribution $D$ and the actual observed value $y$. It measures how far, on average, the forecasted values are from the observed value. A smaller value indicates that the forecasted distribution is closer to the observed value.
+
+2. **Expected Absolute Error Between Two Forecasted Values**: $\frac{1}{2} \mathbb{E}_{X, X' \sim D}[|X - X'|]$
+ This term represents the average absolute difference between two independent samples from the forecasted distribution $D$. It measures the internal variability or spread of the forecasted distribution. A larger value indicates a wider spread of the forecasted values.
+
+##### Interpretation
+
+- **First Term** ($\mathbb{E}_{X \sim D}[|X - y|]$): This term penalizes the forecast based on how far the predicted values are from the observed value. It ensures that the forecast is accurate in terms of proximity to the actual observation.
+
+- **Second Term** ($\frac{1}{2} \mathbb{E}_{X, X' \sim D}[|X - X'|]$): This term accounts for the spread of the forecasted distribution. It penalizes forecasts that are too uncertain or have a wide spread. By subtracting this term, the CRPS rewards forecasts that are not only accurate but also confident (i.e., have a narrow spread).
+:::
+
+Whilst the CRPS is a very useful metric it can be difficult to interpret in isolation. It is often useful to compare the CRPS of different models or to compare the CRPS of the same model under different conditions. For example, lets compare the CRPS across different forecast horizons.
+
+```{r}
+sc_scores |>
+ summarise_scores(by = "horizon") |>
+ ggplot(aes(x = horizon, y = crps)) +
+ geom_point() +
+ labs(title = "CRPS by daily forecast horizon",
+ subtitle = "Summarised across all forecasts")
+```
+
+and at different time points.
+
+```{r}
+sc_scores |>
+ summarise_scores(by = "target_day") |>
+ ggplot(aes(x = target_day, y = crps)) +
+ geom_point() +
+ labs(title = "CRPS by forecast start date",
+ subtitle = "Summarised across all forecasts", x = "forecast date")
+```
+
+::: {.callout-tip}
+## Take 5 minutes
+How do the CRPS scores change based on forecast date?
+How do the CRPS scores change with forecast horizon?
+What does this tell you about the model?
+:::
+
+::: {.callout-note collapse="true"}
+## Solution
+ - The CRPS scores increase for forecast dates where incidence is higher.
+ - The CRPS scores increase with forecast horizon.
+ - As the CRPS is an absolute measure it is hard to immediately know if the CRPS increasing with forecast date indicates that the model is performing worse.
+ - However, the CRPS increasing with forecast horizon is a sign that the model is struggling to capture the longer term dynamics of the epidemic.
+:::
+
+### PIT histograms
+
+As well as the CRPS we can also look at the calibration and bias of the model. Calibration is the agreement between the forecast probabilities and the observed frequencies. Bias is a measure of how likely the model is to over or under predict the observed values.
+
+There are many ways to assess calibration and bias but one common way is to use a probability integral transform (PIT) histogram. This is a histogram of the cumulative distribution of function of a forecast evaluated at the observed value.
+
+::: {.callout-tip}
+## Interpreting the PIT histogram
+- Ideally PIT histograms should be uniform.
+- If is a U shape then the model is overconfident and if it is an inverted U shape then the model is underconfident.
+- If it is skewed then the model is biased towards the direction of the skew.
+:::
+
+::: {.callout-tip collapse="true"}
+## Mathematical Definition (optional)
+
+### Continuous Case
+
+For a continuous random variable $X$ with cumulative distribution function (CDF) $F_X$, the PIT is defined as:
+
+$$
+Y = F_X(X)
+$$
+
+where $Y$ is uniformly distributed on $[0, 1]$.
+
+#### Integer Case
+
+When dealing with integer forecasts, the standard PIT does not yield a uniform distribution even if the forecasts are perfectly calibrated. To address this, a randomized version of the PIT is used. For an integer-valued random variable $X$ with CDF $F_X$, the randomized PIT is defined as:
+
+$$
+U = F_X(k) + v \cdot (F_X(k) - F_X(k-1))
+$$
+
+where:
+
+- $k$ is the observed integer value.
+- $F_X(k)$ is the CDF evaluated at $k$.
+- $v$ is a random variable uniformly distributed on $[0, 1]$.
+
+This transformation ensures that $U$ is uniformly distributed on $[0, 1]$ if the forecasted distribution $F_X$ is correctly specified.
+:::
+
+Let's first look at the overall PIT histogram.
+
+```{r pit-histogram}
+sc_forecasts |>
+ get_pit_histogram() |>
+ ggplot(aes(x = mid, y = density)) +
+ geom_col() +
+ labs(title = "PIT histogram", x = "Quantile", y = "Density")
+```
+
+As before lets look at the PIT histogram by forecast horizon. To save space we will group horizons into a few days each:
+
+```{r pit-histogram-horizon}
+sc_forecasts |>
+ mutate(group_horizon = case_when(
+ horizon <= 3 ~ "1-3",
+ horizon <= 7 ~ "4-7",
+ horizon <= 14 ~ "8-14"
+ )) |>
+ get_pit_histogram(by = "group_horizon") |>
+ ggplot(aes(x = mid, y = density)) +
+ geom_col() +
+ facet_wrap(~group_horizon) +
+ labs(title = "PIT by forecast horizon (days)")
+```
+
+and then for different forecast dates.
+
+```{r pit-histogram-date}
+sc_forecasts |>
+ get_pit_histogram(by = "target_day") |>
+ ggplot(aes(x = mid, y = density)) +
+ geom_col() +
+ facet_wrap(~target_day) +
+ labs(title = "PIT by forecast date")
+```
+
+::: {.callout-tip}
+## Take 5 minutes
+What do you think of the PIT histograms?
+Do they look well calibrated?
+Do they look biased?
+:::
+
+::: {.callout-note collapse="true"}
+## Solution
+- It looks like the model is biased towards overpredicting and that this bias gets worse at longer forecast horizons.
+- Looking over forecast dates it looks like much of this bias is coming from near the outbreak peak where the model is consistently overpredicting but the model is also over predicting at other times.
+:::
+
+## Scoring on the log scale
+
+We can also score on the logarithmic scale.
+This can be useful if we are interested in the relative performance of the model at different scales of the data, for example if we are interested in the model's performance at capturing the exponential growth phase of the epidemic.
+In some sense scoring in this way can be an approximation of scoring the effective reproduction number estimates.
+Doing this directly can be difficult as the effective reproduction number is a latent variable and so we cannot directly score it.
+
+We again use `scoringutils` but first transform both the forecasts and observations to the log scale.
+
+```{r log-convert-forecasts}
+log_sc_forecasts <- sc_forecasts |>
+ transform_forecasts(
+ fun = log_shift,
+ offset = 1,
+ append = FALSE
+ )
+
+log_scores <- log_sc_forecasts |>
+ score()
+```
+
+For more on scoring on the log scale see [this paper on scoring forecasts on transformed scales](https://journals.plos.org/ploscompbiol/article?id=10.1371/journal.pcbi.1011393).
+
+### At a glance
+
+```{r}
+log_scores |>
+ summarise_scores(by = "model")
+```
+
+::: {.callout-tip}
+## Take 2 minutes
+Before we look in detail at the scores, what do you think the scores are telling you? How do you think they will differ from the scores on the natural scale?
+:::
+
+### CRPS
+
+```{r}
+log_scores |>
+ summarise_scores(by = "horizon") |>
+ ggplot(aes(x = horizon, y = crps)) +
+ geom_point() +
+ labs(title = "CRPS by daily forecast horizon, scored on the log scale")
+```
+
+and across different forecast dates
+
+```{r}
+log_scores |>
+ summarise_scores(by = "target_day") |>
+ ggplot(aes(x = target_day, y = crps)) +
+ geom_point() +
+ labs(title = "CRPS by forecast date, scored on the log scale")
+```
+
+::: {.callout-tip}
+## Take 5 minutes
+How do the CRPS scores change based on forecast date?
+How do the CRPS scores change with forecast horizon?
+What does this tell you about the model?
+:::
+
+::: {.callout-note collapse="true"}
+## Solution
+- As for the natural scale CRPS scores increase with forecast horizon but now the increase appears to be linear vs exponential.
+- There has been a reduction in the CRPS scores for forecast dates near the outbreak peak compared to other forecast dates but this is still the period where the model is performing worst.
+:::
+
+### PIT histograms
+
+Let's first look at the overall PIT histogram.
+
+```{r log-pit-histogram}
+log_sc_forecasts |>
+ get_pit_histogram(by = "model") |>
+ ggplot(aes(x = mid, y = density)) +
+ geom_col() +
+ labs(title = "PIT histogram, scored on the log scale")
+```
+
+As before lets look at the PIT histogram by forecast horizon
+
+```{r log-pit-histogram-horizon}
+log_sc_forecasts |>
+ mutate(group_horizon = case_when(
+ horizon <= 3 ~ "1-3",
+ horizon <= 7 ~ "4-7",
+ horizon <= 14 ~ "8-14"
+ )) |>
+ get_pit_histogram(by = "group_horizon") |>
+ ggplot(aes(x = mid, y = density)) +
+ geom_col() +
+ facet_wrap(~group_horizon) +
+ labs(title = "PIT by forecast horizon, scored on the log scale")
+```
+
+and then for different forecast dates.
+
+```{r log-pit-histogram-date}
+log_sc_forecasts |>
+ get_pit_histogram(by = "target_day") |>
+ ggplot(aes(x = mid, y = density)) +
+ geom_col() +
+ facet_wrap(~target_day) +
+ labs(title = "PIT by forecast date, scored on the log scale")
+```
+
+::: {.callout-tip}
+## Take 5 minutes
+What do you think of the PIT histograms?
+Do they look well calibrated?
+Do they look biased?
+:::
+
+::: {.callout-note collapse="true"}
+## Solution
+- The overall PIT histograms suggest that the model is less biased to over predict when scored on the log scale than the natural scale, but it is still biased. This makes sense when we think back to the comparison of reproduction number estimates and forecasts we made earlier where the model was consistently over predicting on the reproduction number.
+- By forecast horizon the model is still biased towards over predicting but this bias is less pronounced than on the natural scale.
+- Towards the end and beginning of the forecast period the model appears to be well calibrated on the log scale but is biased towards over predicting in the middle of the forecast period.
+- This matches with our knowledge of the underlying reproduction number which were initially constant and then began to decrease only to stabilise towards the end of the outbreak.
+:::
+
+# Going further
+
+- In which other ways could we summarise the performance of the forecasts?
+- What other metrics could we use?
+- There is no one-size-fits-all approach to forecast evaluation, often you will need to use a combination of metrics to understand the performance of your model and typically the metrics you use will depend on the context of the forecast. What attributes of the forecast are most important to you?
+- There are many other metrics that can be used to evaluate forecasts. The [documentation](https://epiforecasts.io/scoringutils/dev/articles/metric-details.html) for the `{scoringutils}` package has a good overview of these metrics and how to use them.
+- One useful way to think about evaluating forecasts is to consider exploring the scores as a data analysis in its own right. For example, you could look at how the scores change over time, how they change with different forecast horizons, or how they change with different models. This can be a useful way to understand the strengths and weaknesses of your model. Explore some of these aspects using the scores from this session.
+
+# Wrap up
diff --git a/sessions/forecasting-models.qmd b/sessions/forecasting-models.qmd
new file mode 100644
index 0000000..5bea84d
--- /dev/null
+++ b/sessions/forecasting-models.qmd
@@ -0,0 +1,786 @@
+---
+title: "Forecasting models"
+order: 8
+---
+
+# Introduction
+
+We can classify models along a spectrum by how much they include an understanding of underlying processes, or mechanisms; or whether they emphasise drawing from the data using a statistical approach.
+In this session, we'll start with the renewal model that we've been using and explore adding both more mechanistic structure and then more statistical structure to the model. We'll again evaluate these models to see what effect these different approaches might have.
+
+## Slides
+
+- [Forecasting models](slides/forecasting-models)
+
+## Objectives
+
+The aim of this session is to introduce some common forecasting models and to evaluate them.
+
+::: {.callout-caution}
+None of the models introduced in this section are designed for real-world outbreak use!
+:::
+
+::: {.callout-note collapse="true"}
+
+# Setup
+
+## Source file
+
+The source file of this session is located at `sessions/forecasting-models.qmd`.
+
+## Libraries used
+
+In this session we will use the `nfidd` package to load a data set of infection times and access stan models and helper functions, the `dplyr` and `tidyr` packages for data wrangling, `ggplot2` library for plotting, the `tidybayes` package for extracting results of the inference and the `scoringutils` package for evaluating forecasts.
+
+```{r libraries, message = FALSE}
+library("nfidd")
+library("dplyr")
+library("tidyr")
+library("ggplot2")
+library("tidybayes")
+library("scoringutils")
+```
+
+::: {.callout-tip}
+The best way to interact with the material is via the [Visual Editor](https://docs.posit.co/ide/user/ide/guide/documents/visual-editor.html) of RStudio.
+:::
+
+## Initialisation
+
+We set a random seed for reproducibility.
+Setting this ensures that you should get exactly the same results on your computer as we do.
+We also set an option that makes `cmdstanr` show line numbers when printing model code.
+This is not strictly necessary but will help us talk about the models.
+
+```{r}
+set.seed(123)
+options(cmdstanr_print_line_numbers = TRUE)
+```
+
+:::
+
+# What did we learn about the random walk model from the [forecasting concepts session](forecasting-concepts)?
+
+- It was unbiased when the reproduction number was approximately constant but when the reproduction number was reducing due to susceptible depletion, the random walk model overestimated the number of cases systematically.
+- It did relatively well at short-term forecasting indicating that it was capturing some of the underlying dynamics of the data generating process.
+
+::: {.callout-note collapse="true"}
+## What did a geometric random walk model look like again?
+
+```{r geometric-random-walk-sim}
+R <- geometric_random_walk(init = 1, noise = rnorm(100), std = 0.1)
+data <- tibble(t = seq_along(R), R = exp(R))
+
+ggplot(data, aes(x = t, y = R)) +
+ geom_line() +
+ labs(title = "Simulated data from a random walk model",
+ x = "Time",
+ y = "R")
+```
+
+:::
+
+# Forecasting models as a spectrum
+
+A large part of this course has been about showing the importance of understanding and modelling the underlying mechanisms of the data generating process.
+However, in many forecasting scenarios, we may not have a good understanding of the underlying mechanisms, or the data may be too noisy to make accurate predictions.
+The worst case is that we have mistaken beliefs about the underlying mechanisms and use these to make predictions which are systematically wrong and misleading.
+In these cases, forecasters often use _statistical models_ to make predictions which have little or no mechanistic basis.
+In our work, we have found that a combination of mechanistic and statistical models can be very powerful but that identifying the best model for a given forecasting task can be challenging.
+
+## Adding more mechanistic structure to the renewal model
+
+One way to potentially improve the renewal model is to add more mechanistic structure. In the [forecasting concepts session](forecasting-concepts), we saw that the renewal model was making unbiased forecasts when the reproduction number was constant but that it overestimated the number of cases when the reproduction number was reducing due to susceptible depletion.
+
+::: {.callout-warning}
+This is slightly cheating as we know the future of this outbreak and so can make a model to match. This is easy to do and important to watch for if wanting to make generalisable methods.
+:::
+
+This suggests that we should add a term to the renewal model which captures the depletion of susceptibles. One way to do this is to add a term which is proportional to the number of susceptibles in the population. This is the idea behind the _SIR model_ which is a simple compartmental model of infectious disease transmission. If we assume that susceptible depletion is the only mechanism which is causing the reproduction number to change, we can write the reproduction model as:
+
+$$
+R_t = \frac{S_{t-1}}{N} R_0
+$$
+
+::: {.callout-note}
+This approximates susceptible depletion as a linear function of the number of susceptibles in the population. This is a simplification but it is a good starting point.
+:::
+
+::: {.callout-note collapse="true"}
+
+## What behaviour would we expect from this model?
+
+```{r}
+n <- 100
+N <- 1000
+R0 <- 1.5
+S <- rep(NA, n)
+S[1] <- N
+Rt <- rep(NA, n) ## reproduction number
+Rt[1] <- R0
+I <- rep(NA, n)
+I[1] <- 1
+for (i in 2:n) {
+ Rt[i] <- (S[i-1]) / N * R0
+ I[i] <- I[i-1] * Rt[i]
+ S[i] <- S[i-1] - I[i]
+}
+
+data <- tibble(t = 1:n, Rt = Rt)
+
+ggplot(data, aes(x = t, y = Rt)) +
+ geom_line() +
+ labs(title = "Simulated data from an SIR model",
+ x = "Time",
+ y = "Rt")
+```
+:::
+
+The key assumptions we are making here are:
+
+- The population is constant and we roughly know the size of the population.
+- The reproduction number only changes due to susceptible depletion
+- The number of new cases at each time is proportional to the number of susceptibles in the population.
+
+We've coded this up as a stan model in `stan/mechanistic-r.stan`. See `stan/functions/pop_bounded_renewal.stan` for the function which calculates the reproduction number. Let's load the model:
+
+```{r}
+mech_mod <- nfidd_cmdstan_model("mechanistic-r")
+mech_mod
+```
+
+## Adding more statistical structure to the renewal model
+
+Adding more mechanistic structure is not always possible and, if we don't specify mechanisms correctly, might make forecasts worse.
+Rather than adding more mechanistic structure to the renewal model, we could add more statistical structure with the aim of improving performance.
+Before we do this, we need to think about what we want from a forecasting model.
+As we identified above, we want a model which is unbiased and which has good short-term forecasting properties.
+We know that we want it to be able to adapt to trends in the reproduction number and that we want it to be able to capture the noise in the data.
+A statistical term that can be used to describe a time series with a trend is saying that the time series is _non-stationary_.
+More specifically, a _stationary_ time series is defined as one whose statistical properties, such as mean and variance, do not change over time.
+In infectious disease epidemiology, this would only be expected for endemic diseases without external seasonal influence.
+
+The random walk model we used in the [forecasting concept](forecasting-concepts) session is a special case of a more general class of models called _autoregressive (AR) models_.
+AR models are a class of models which predict the next value in a time series as a linear combination of the previous values in the time series.
+The random walk model is specifically a special case of an AR(1) model where the next value in the time series is predicted as the previous value, multiplied by a value between 1 and -1 , plus some noise. This becomes a random walk when the multipled value is 0.
+
+ For the log-transformed reproduction number ($log(R_t)$), the model is:
+
+$$
+log(R_t) = \phi log(R_{t-1}) + \epsilon_t
+$$
+
+where $\epsilon_t$ is a normally distributed error term with mean 0 and standard deviation $\sigma$ and $\phi$ is a parameter between -1 and 1. If we restrict $\phi$ to be between 0 and 1, we get a model which is biased towards a reproduction number of 1 but which can still capture trends in the data that decay over time.
+
+::: {.callout-note collapse="true"}
+## What behaviour would we expect from this model?
+
+```{r}
+n <- 100
+phi <- 0.4
+sigma <- 0.1
+log_R <- rep(NA, n)
+log_R[1] <- rnorm(1, 0, sigma)
+for (i in 2:n) {
+ log_R[i] <- phi * log_R[i-1] + rnorm(1, 0, sigma)
+}
+data <- tibble(t = 1:n, R = exp(log_R))
+
+ggplot(data, aes(x = t, y = R)) +
+ geom_line() +
+ labs(title = "Simulated data from an exponentiated AR(1) model",
+ x = "Time",
+ y = "R")
+```
+:::
+
+However, we probably don't want a model which is biased towards a reproduction number of 1 (unless we have good reason to believe that is the expected behaviour). So what should we do?
+
+Returning to the idea that the reproduction number is a _non-stationary_ time series, as we expect to have a trend in the reproduction numbers we want to capture, we can use a method from the field of time series analysis called _differencing_ to make the time series stationary. This involves taking the difference between consecutive values in the time series. For the log-transformed reproduction number, this would be:
+
+$$
+log(R_t) - log(R_{t-1}) = \phi (log(R_{t-1}) - log(R_{t-2})) + \epsilon_t
+$$
+
+
+::: {.callout-note collapse="true"}
+## What behaviour would we expect from this model?
+
+Again we look at an R function that implements this model:
+
+```{r geometric-diff-ar}
+geometric_diff_ar
+```
+
+We can use this function to simulate a differenced AR process:
+
+```{r}
+log_R <- geometric_diff_ar(init = 1, noise = rnorm(100), std = 0.1, damp = 0.1)
+
+data <- tibble(t = seq_along(log_R), R = exp(log_R))
+
+ggplot(data, aes(x = t, y = R)) +
+ geom_line() +
+ labs(title = "Simulated data from an exponentiated AR(1) model with differencing",
+ x = "Time",
+ y = "R")
+```
+
+:::
+
+We've coded up a model that uses this differenced AR process as a stan model in `stan/statistical-r.stan`. See `stan/functions/geometic_diff_ar.stan` for the function which calculates the reproduction number. Lets load the model:
+
+```{r}
+stat_mod <- nfidd_cmdstan_model("statistical-r")
+stat_mod
+```
+
+
+# Forecasting with the mechanistic and statistical models
+
+We will now use the mechanistic and statistical models to forecast the number of cases in the future using data simulated in the same way as we did in the [forecasting concepts session](forecasting-concepts). We will first load in the data and filter for a target forecast date.
+
+```{r, load-simulated-onset}
+gen_time_pmf <- make_gen_time_pmf()
+ip_pmf <- make_ip_pmf()
+onset_df <- simulate_onsets(
+ make_daily_infections(infection_times), gen_time_pmf, ip_pmf
+)
+onset_df
+
+# we'll make a forecast on day non day 41, pretending we haven't seen the later
+# data
+cutoff <- 41
+
+filtered_onset_df <- onset_df |>
+ filter(day <= cutoff)
+```
+
+We will now fit the more mechanistic model to the data.
+```{r fit_mech_model, results = 'hide', message = FALSE}
+horizon <- 28
+
+data <- list(
+ n = nrow(filtered_onset_df),
+ I0 = 1,
+ obs = filtered_onset_df$onsets,
+ gen_time_max = length(gen_time_pmf),
+ gen_time_pmf = gen_time_pmf,
+ ip_max = length(ip_pmf) - 1,
+ ip_pmf = ip_pmf,
+ h = horizon, # Here we set the number of days to forecast into the future
+ N_prior = c(10000, 2000) # the prior for the population size
+)
+mech_forecast_fit <- mech_mod$sample(
+ data = data, parallel_chains = 4
+)
+```
+
+```{r mech-forcast-print}
+mech_forecast_fit
+```
+
+We will now fit the more statistical model to the data.
+
+```{r fit_stat_model, results = 'hide', message = FALSE}
+data <- list(
+ n = nrow(filtered_onset_df),
+ I0 = 1,
+ obs = filtered_onset_df$onsets,
+ gen_time_max = length(gen_time_pmf),
+ gen_time_pmf = gen_time_pmf,
+ ip_max = length(ip_pmf) - 1,
+ ip_pmf = ip_pmf,
+ h = horizon # Here we set the number of days to forecast into the future
+)
+stat_forecast_fit <- stat_mod$sample(
+ data = data, parallel_chains = 4,
+ # again set the initial values to make fitting more numerically stable
+ init = \() list(init_R = 0, rw_sd = 0.01)
+)
+```
+
+Finally, we can extract the forecasts from the models and plot them.
+
+```{r extract-forecast}
+mech_forecast <- mech_forecast_fit |>
+ gather_draws(forecast[day]) |>
+ ungroup() |>
+ mutate(day = day + cutoff)
+
+stat_forecast <- stat_forecast_fit |>
+ gather_draws(forecast[day]) |>
+ ungroup() |>
+ mutate(day = day + cutoff)
+
+forecast <- bind_rows(
+ mutate(mech_forecast, model = "more mechanistic"),
+ mutate(stat_forecast, model = "more statistical")
+) |>
+ ungroup()
+
+target_onsets <- onset_df |>
+ filter(day > cutoff) |>
+ filter(day <= cutoff + horizon)
+```
+
+
+```{r plot_forecast}
+forecast |>
+ filter(.draw %in% sample(.draw, 100)) |>
+ ggplot(aes(x = day)) +
+ geom_line(
+ alpha = 0.1,
+ aes(y = .value, group = interaction(.draw, model), colour = model)
+ ) +
+ geom_point(data = target_onsets, aes(x = day, y = onsets), color = "black") +
+ guides(colour = guide_legend(override.aes = list(alpha = 1))) +
+ lims(y = c(0, 500))
+```
+
+and on the log scale
+
+```{r plot_log_forecast}
+forecast |>
+ filter(.draw %in% sample(.draw, 100)) |>
+ ggplot(aes(x = day)) +
+ geom_line(
+ alpha = 0.1,
+ aes(y = .value, group = interaction(.draw, model), colour = model)
+ ) +
+ geom_point(data = target_onsets, aes(x = day, y = onsets), color = "black") +
+ scale_y_log10() +
+ guides(colour = guide_legend(override.aes = list(alpha = 1)))
+```
+
+::: {.callout-tip}
+## Take 2 minutes
+What do you notice about the forecasts from the more mechanistic and more statistical models?
+:::
+
+::: {.callout-note collapse="true"}
+## Solution
+- The more mechanistic model captures the downturn in the data very well.
+- However it appears to be somewhat biased as it consistently overpredicts.
+- The more statistical model produces some very large forecasts but also has significant probability mass on a a more rapid reduction that ultimately observed.
+- Neither model simply extend the trend in the way the random walk model did in the [forecasting concepts session](forecasting-concepts).
+:::
+
+::: {.callout-note collapse="true"}
+As these models are still renewal processes we can still plot the time-varying reproduction number which can be a helpful way of reasoning about how the models are performing.
+
+```{r plot-stat-rt}
+stat_forecast_fit |>
+ gather_draws(R[day]) |>
+ ungroup() |>
+ filter(.draw %in% sample(.draw, 100)) |>
+ ggplot(aes(y = .value, x = day)) +
+ geom_hline(yintercept = 1, linetype = "dashed") +
+ geom_line(aes(group = .draw), alpha = 0.1)
+```
+:::
+
+::: {.callout-tip}
+## What happens when you are very wrong about the population size? (optional)
+
+In the above we assumed that we knew the population size roughly. In practice, we may not. Refit the more mechanistic model with different priors for the population size and see how the forecasts change.
+
+:::
+
+::: {.callout-note collapse="true"}
+## Solution
+
+```{r very-wrong, results = 'hide', message = FALSE}
+data <- list(
+ n = nrow(filtered_onset_df),
+ I0 = 1,
+ obs = filtered_onset_df$onsets,
+ gen_time_max = length(gen_time_pmf),
+ gen_time_pmf = gen_time_pmf,
+ ip_max = length(ip_pmf) - 1,
+ ip_pmf = ip_pmf,
+ h = horizon, # Here we set the number of days to forecast into the future
+ N_prior = c(100, 20) # the prior for the population size
+)
+
+mech_forecast_fit_diff <- mech_mod$sample(
+ data = data, parallel_chains = 4
+)
+
+mech_forecast_diff <- mech_forecast_fit_diff |>
+ gather_draws(forecast[day]) |>
+ ungroup() |>
+ mutate(day = day + cutoff)
+```
+
+```{r very-wrong-plot}
+mech_forecast_diff |>
+ filter(.draw %in% sample(.draw, 100)) |>
+ ggplot(aes(y = .value, x = day)) +
+ geom_line(alpha = 0.1, aes(group = .draw)) +
+ geom_point(
+ data = target_onsets, aes(x = day, y = onsets),
+ color = "black"
+ )
+```
+:::
+
+# Evaluating forecasts from our models
+
+As in the [forecasting concepts session](forecasting-concepts), we have fit these models to a range of forecast dates so you don't have to wait for the models to fit. We will now evaluate the forecasts from the mechanistic and statistical models.
+
+```{r load_forecasts}
+data(rw_forecasts, stat_forecasts, mech_forecasts)
+forecasts <- bind_rows(
+ rw_forecasts,
+ mutate(stat_forecasts, model = "More statistical"),
+ mutate(mech_forecasts, model = "More mechanistic")
+) |>
+ ungroup()
+```
+
+::: {.callout-tip, collapse="true"}
+## How did we estimate these forecasts?
+We generated these forecasts using the code in `data-raw/generate-example-forecasts.r` which uses the same approach we just took for a single forecast date but generalises it to many forecast dates.
+
+Some important things to note about these forecasts:
+
+ - We used a 14 day forecast horizon.
+ - Each forecast used all the data up to the forecast date.
+ - We generated 1000 posterior samples for each forecast.
+ - We started forecasting 3 weeks into the outbreak and then forecast every 7 days until the end of the data (excluding the last 14 days to allow a full forecast).
+ - We use the same simulated outbreak data:
+
+```{r}
+head(onset_df)
+```
+
+:::
+
+## Visualising your forecast
+
+
+```{r plot-all-forecasts}
+forecasts |>
+ filter(.draw %in% sample(.draw, 100)) |>
+ ggplot(aes(x = day)) +
+ geom_line(aes(y = .value, group = interaction(.draw, target_day), col = target_day), alpha = 0.1) +
+ geom_point(data = onset_df |>
+ filter(day >= 21),
+ aes(x = day, y = onsets), color = "black") +
+ scale_color_binned(type = "viridis") +
+ facet_wrap(~model) +
+ lims(y = c(0, 500))
+```
+
+
+As for the single forecast it is helpful to also plot the forecast on the log scale.
+
+```{r plot-all-forecasts-log}
+forecasts |>
+ filter(.draw %in% sample(.draw, 100)) |>
+ ggplot(aes(x = day)) +
+ geom_line(
+ aes(y = .value, group = interaction(.draw, target_day), col = target_day),
+ alpha = 0.1
+ ) +
+ geom_point(data = onset_df, aes(x = day, y = onsets), color = "black") +
+
+ scale_color_binned(type = "viridis") +
+ facet_wrap(~model)
+```
+
+::: {.callout-tip}
+## Take 5 minutes
+How do these forecasts compare?
+Which do you prefer?
+:::
+
+::: {.callout-note collapse="true"}
+## Solution
+How do these forecasts compare?
+
+- The more mechanistic model captures the downturn in the data very well.
+- Past the peak all models were comparable.
+- The more statistical model captures the downturn faster than the random walk but less fast than the more mechanistic mode.
+- The more statistical model sporadically predicts a more rapidly growing outbreak than occurred early on.
+- The more statistical model is more uncertain than the mechanistic model but less uncertain than the random walk.
+
+Which do you prefer?
+
+- The more mechanistic model seems to be the best at capturing the downturn in the data and the uncertainty in the forecasts seems reasonable.
+- If we weren't confident in the effective susceptible population the AR model might be preferable.
+:::
+
+## Scoring your forecast
+
+
+```{r convert-forecasts}
+sc_forecasts <- forecasts |>
+ left_join(onset_df, by = "day") |>
+ filter(!is.na(.value)) |>
+ as_forecast_sample(
+ forecast_unit = c(
+ "target_day", "horizon", "model"
+ ),
+ observed = "onsets",
+ predicted = ".value",
+ sample_id = ".draw"
+ )
+sc_forecasts
+```
+
+
+Everything seems to be in order. We can now use the `scoringutils` package to calculate some metrics as we did in the [forecasting concepts session](forecasting-concepts).
+
+```{r score-forecasts}
+sc_scores <- sc_forecasts |>
+ score()
+
+sc_scores
+```
+ scale_y_log10(limits = c(NA, 500)) +
+::: {.callout-note collapse="true"}
+## Learning more about the output of `score()`
+
+See the documentation for `?metrics_sample` for information on the default sample metrics.
+:::
+
+### At a glance
+
+Let's summarise the scores by model first.
+
+```{r}
+sc_scores |>
+ summarise_scores(by = "model")
+```
+
+::: {.callout-tip}
+## Take 2 minutes
+Before we look in detail at the scores, what do you think the scores are telling you? Which model do you think is best?
+:::
+
+### Continuous ranked probability score
+
+As in the [forecasting concepts session](forecasting-concepts), we will start by looking at the CRPS by horizon and forecast date.
+
+::: {.callout-tip}
+## Reminder: Key things to note about the CRPS
+ - Small values are better
+ - As it is an absolute scoring rule it can be difficult to use to compare forecasts across scales.
+:::
+
+First by forecast horizon.
+
+```{r}
+sc_scores |>
+ summarise_scores(by = c("model", "horizon")) |>
+ ggplot(aes(x = horizon, y = crps, col = model)) +
+ geom_point()
+```
+
+and across different forecast dates
+
+```{r}
+sc_scores |>
+ summarise_scores(by = c("target_day", "model")) |>
+ ggplot(aes(x = target_day, y = crps, col = model)) +
+ geom_point()
+```
+
+::: {.callout-tip}
+## Take 5 minutes
+How do the CRPS scores change based on forecast date?
+How do the CRPS scores change with forecast horizon?
+:::
+
+::: {.callout-note collapse="true"}
+## Solution
+How do the CRPS scores change based on forecast horizon?
+
+- All models have increasing CRPS with forecast horizon.
+- The more mechanistic model has the lowest CRPS at all forecast horizon.
+- The more stastical model starts to outperform the random walk model at longer time horizons.
+
+How do the CRPS scores change with forecast date?
+
+- The more statistical model does particularly poorly around the peak of the outbreak but outperforms the random walk model.
+- The more mechanistic model does particularly well around the peak of the outbreak versus all other models
+- The more statistical model starts to outperfrom the other models towards the end of the outbreak.
+
+:::
+
+### PIT histograms
+
+::: {.callout-tip}
+## Reminder: Interpreting the PIT histogram
+- Ideally PIT histograms should be uniform.
+- If is a U shape then the model is overconfident and if it is an inverted U shape then the model is underconfident.
+- If it is skewed then the model is biased towards the direction of the skew.
+:::
+
+
+Let's first look at the overall PIT histogram.
+
+```{r pit-histogram}
+sc_forecasts |>
+ get_pit_histogram(by = "model") |>
+ ggplot(aes(x = mid, y = density)) +
+ geom_col() +
+ facet_wrap(~model)
+```
+
+As before let's look at the PIT histogram by forecast horizon (to save space we will group horizons)
+
+```{r pit-histogram-horizon}
+sc_forecasts |>
+ mutate(group_horizon = case_when(
+ horizon <= 3 ~ "1-3",
+ horizon <= 7 ~ "4-7",
+ horizon <= 14 ~ "8-14"
+ )) |>
+ get_pit_histogram(by = c("model", "group_horizon")) |>
+ ggplot(aes(x = mid, y = density)) +
+ geom_col() +
+ facet_grid(vars(model), vars(group_horizon))
+```
+
+and then for different forecast dates.
+
+```{r pit-histogram-date, fig.width = 10}
+sc_forecasts |>
+ get_pit_histogram(by = c("model", "target_day")) |>
+ ggplot(aes(x = mid, y = density)) +
+ geom_col() +
+ facet_grid(vars(model), vars(target_day))
+```
+
+::: {.callout-tip}
+## Take 5 minutes
+What do you think of the PIT histograms?
+:::
+
+::: {.callout-note collapse="true"}
+## Solution
+What do you think of the PIT histograms?
+
+- The more mechanistic model is reasonably well calibrated but has a slight tendency to be overconfident.
+- The random walk is biased towards overpredicting.
+- The more statistical model is underconfident.
+- Across horizons the more mechanistic model is only liable to underpredict at the longest horizons.
+- The random walk model is initially relatively unbiased and well calibrated but becomes increasingly likely to overpredict as the horizon increases.
+- The forecast date stratified PIT histograms are hard to interpret. We may need to find other ways to visualise bias and calibration at this level of stratification (see the `{scoringutils}` documentation for some ideas).
+
+:::
+
+## Scoring on the log scale
+
+Again as in the [forecasting concepts session](forecasting-concepts), we will also score the forecasts on the log scale.
+
+```{r log-convert-forecasts}
+log_sc_forecasts <- sc_forecasts |>
+ transform_forecasts(
+ fun = log_shift,
+ offset = 1,
+ append = FALSE
+ )
+
+log_sc_scores <- log_sc_forecasts |>
+ score()
+```
+
+
+::: {.callout-tip}
+Reminder: For more on scoring on the log scale see [this paper on scoring forecasts on transformed scales](https://journals.plos.org/ploscompbiol/article?id=10.1371/journal.pcbi.1011393).
+:::
+
+### At a glance
+
+```{r}
+log_sc_scores |>
+ summarise_scores(by = "model")
+```
+
+::: {.callout-tip}
+## Take 2 minutes
+Before we look in detail at the scores, what do you think the scores are telling you? Which model do you think is best?
+:::
+
+### CRPS
+
+```{r}
+log_sc_scores |>
+ summarise_scores(by = c("model", "horizon")) |>
+ ggplot(aes(x = horizon, y = crps, col = model)) +
+ geom_point()
+```
+
+```{r}
+log_sc_scores |>
+ summarise_scores(by = c("target_day", "model")) |>
+ ggplot(aes(x = target_day, y = crps, col = model)) +
+ geom_point()
+```
+
+::: {.callout-tip}
+## Take 5 minutes
+How do the CRPS scores on the log scale compare to the scores on the original scale?
+:::
+
+::: {.callout-note collapse="true"}
+## Solution
+- The performance of the mechanistic model is more variable across forecast horizon than on the natural scale.
+- On the log scale the by horizon performance of the random walk and more statistical mdoel is more comparable than on the log scale.
+- The period of high incidence dominates the target day stratified scores less on the log scale. We see that all models performed less well early and late on.
+:::
+
+### PIT histograms
+
+```{r pit-histogram-log}
+log_sc_forecasts |>
+ get_pit_histogram(by = "model") |>
+ ggplot(aes(x = mid, y = density)) +
+ geom_col() +
+ facet_wrap(~model)
+```
+
+
+```{r pit-histogram-horizon-log}
+log_sc_forecasts |>
+ mutate(group_horizon = case_when(
+ horizon <= 3 ~ "1-3",
+ horizon <= 7 ~ "4-7",
+ horizon <= 14 ~ "8-14"
+ )) |>
+ get_pit_histogram(by = c("model", "group_horizon")) |>
+ ggplot(aes(x = mid, y = density)) +
+ geom_col() +
+ facet_grid(vars(model), vars(group_horizon))
+```
+
+
+```{r pit-histogram-date-log, fig.width = 10}
+log_sc_forecasts |>
+ get_pit_histogram(by = c("model", "target_day")) |>
+ ggplot(aes(x = mid, y = density)) +
+ geom_col() +
+ facet_grid(vars(model), vars(target_day))
+```
+
+::: {.callout-tip}
+## Take 5 minutes
+What do you think of the PIT histograms?
+:::
+
+::: {.callout-note collapse="true"}
+## Solution
+The PIT histograms are similar to the original scale PIT histograms but the mechanistic model appears better calibrated.
+:::
+
+# Going further
+
+- We have only looked at three forecasting models here. There are many more models that could be used. For example, we could use a more complex mechanistic model which captures more of the underlying dynamics of the data generating process. We could also use a more complex statistical model which captures more of the underlying structure of the data.
+- We could also combine the more mechanistic and more statistical models to create a hybrid model which captures the best of both worlds (maybe).
+- We could also use a more complex scoring rule to evaluate the forecasts. For example, we could use a multivariate scoring rule which captures more of the structure of the data.
+
+# Wrap up
diff --git a/sessions/introduction-and-course-overview.qmd b/sessions/introduction-and-course-overview.qmd
new file mode 100644
index 0000000..becbb23
--- /dev/null
+++ b/sessions/introduction-and-course-overview.qmd
@@ -0,0 +1,84 @@
+---
+title: "Introduction and course overview"
+order: 0
+---
+
+[From an epidemiological line list to informing decisions in real-time](slides/from-line-list-to-decisions)
+
+### Aim of the course
+
+In this course we will address how can we use data typically collected in an outbreak to answer questions like
+
+- What is the number of cases now (*nowcasting*)
+- Is the outbreak rising/falling and by how much (*$R_t$ estimation*)
+- What does this mean for the near future (*forecasting*)
+
+To answer these questions, we need to understand the epidemiological processes that create the kinds of data that we typically have available for outbreak analysis.
+
+There are particular challenges when trying to do these analyses *in real time* (i.e. whilst transmission and data collection is ongoing) rather than retrospectively, which we will address in turn.
+
+::: callout
+Let's look at outbreak data from the perspective of an individual infection.
+There are two types of processes happening:
+
+- upwards, from an individual infection through to being recorded in outbreak data; and
+
+- outwards, from each infection spreading to cause new infections in the outbreak.
+
+Both of these processes involve time delays which makes analysing data in real time especially tricky.
+:::
+
+In this course, we first focus on making sense of the delays and biases in the data we are able to access in real time during an outbreak.
+We'll combine this with the process of infectious disease transmission, with the reproduction number as a key component.
+With that, we can start to interpret the present (nowcasting) and predict the future (forecasting).
+
+### Why this course?
+
+- These are common questions in outbreak response
+- Accounting for underlying processes can get surprisingly complicated quickly[^1], and it's easy to make mistakes
+- There's currently (at the time of devising this course) no comprehensive training resource that links these common questions and challenges
+
+### Approach
+
+Throughout the course we will
+
+1. simulate typical infectious disease data in **R** (thus introducing the *generative model*)
+2. apply the generative model to data in the probabilistic programming language **stan**, to
+ - learn about the system (conduct inference)
+ - make predictions (nowcasting/forecasting)
+
+Each session in the course:
+
+- builds on the previous one so that participants will have an overview of the real-time analysis workflow by the end of the course;
+- starts with a short introductory talk;
+- mainly consists of interactive content that participants will work through;
+- has optional/additional material that can be skipped or completed after the course ends;
+
+For those attending the in-person version the course also:
+
+- has multiple instructors ready to answer questions about this content; if several people have a similar question we may pause the session and discuss it with the group;
+- ends with a wrap-up and discussion where we review the sessions material.
+
+### Timeline for the course
+
+The course was created to be taught in-person for 2.5 days but of course if you are studying this on your own using the web site you can go through the material at your own pace and in your own time.
+Broadly, the intended timeline is:
+
+- delay distributions and how to estimate them (day 1)
+- $R_t$ estimation and the generation interval (day 1)
+- nowcasting (day 2)
+- forecasting and evaluation, ensemble methods (day 2)
+- applications (day 3)
+
+Let's get started!
+
+- Have a more detailed look at the [learning objectives](/learning_objectives)
+
+- [Introduction to the course and the instructors](slides/introduction-to-the-course-and-the-instructors)
+
+- If you haven't already, start with [getting set up for the course](/getting-set-up)
+
+- Once you're all set up, let's introduce some concepts and methods we'll rely heavily in this course in the [session on delay distributions](delay-distributions).
+
+
+[^1]: Time travel is [messy stuff](https://youtu.be/q2nNzNo_Xps)
diff --git a/sessions/joint-nowcasting.qmd b/sessions/joint-nowcasting.qmd
new file mode 100644
index 0000000..76ad573
--- /dev/null
+++ b/sessions/joint-nowcasting.qmd
@@ -0,0 +1,365 @@
+---
+title: "Nowcasting with an unknown reporting delay"
+order: 6.5
+---
+
+# Introduction
+
+In the last session we introduced the idea of nowcasting using a simple model. However, this approach had problems: we didn't fully account for uncertainty, or for example observation error in the primary events, and it's not a fully generative model of the data reporting process. And as we saw, if we get the delay distribution wrong, we can get the nowcast very wrong.
+
+A better approach is to jointly estimate the delay distribution together with the nowcast. We can do this by using information from multiple snapshots of the data as it changes over time (using a data structure called the "reporting triangle"). In this session, we'll introduce this approach to joint estimation in nowcasting. At the end we'll then demonstrate a way to combine this with our previous work estimating the reproduction number, steadily improving our real time outbreak model.
+
+## Slides
+
+- [Introduction to joint estimation of delays and nowcasts](slides/introduction-to-joint-estimation-of-nowcasting-and-reporting-delays)
+
+## Objectives
+
+This session aims to introduce how to do nowcasting if the reporting delay distribution is unknown.
+
+::: {.callout-note collapse="true"}
+
+# Setup
+
+## Source file
+
+The source file of this session is located at `sessions/joint-nowcasting.qmd`.
+
+## Libraries used
+
+In this session we will use the `nfidd` package to load a data set of infection times and access stan models and helper functions, the `dplyr` and `tidyr` packages for data wrangling, `ggplot2` library for plotting, and the `tidybayes` package for extracting results of the inference.
+
+```{r libraries, message = FALSE}
+library("nfidd")
+library("dplyr")
+library("tidyr")
+library("ggplot2")
+library("tidybayes")
+```
+
+::: {.callout-tip}
+The best way to interact with the material is via the [Visual Editor](https://docs.posit.co/ide/user/ide/guide/documents/visual-editor.html) of RStudio.
+:::
+
+## Initialisation
+
+We set a random seed for reproducibility.
+Setting this ensures that you should get exactly the same results on your computer as we do.
+We also set an option that makes `cmdstanr` show line numbers when printing model code.
+This is not strictly necessary but will help us talk about the models.
+
+```{r}
+set.seed(123)
+options(cmdstanr_print_line_numbers = TRUE)
+```
+
+:::
+
+# Joint estimation of delay distributions and nowcasting
+
+## Motivation
+
+So far we have assumed that the delay distribution is known. In practice, this is often not the case and we need to estimate it from the data.
+As we discussed in the [session on biases in delay distributions](sessions/biases-in-delay-distributions), this can be done using individual data and then passing this estimate to a simple nowcasting model like those above.
+However, this has the disadvantage that the nowcasting model does not take into account the uncertainty in the delay distribution or observation error of the primary events.
+We can instead estimate the delay distribution and nowcast the data jointly.
+
+## The reporting triangle
+
+To jointly estimate we need to decompose observations into what is known as the reporting triangle.
+This is a matrix where the rows are the days of onset and the columns are the days of report.
+The entries are the number of onsets on day $i$ that are reported on day $j$.
+We can then use this matrix to estimate the delay distribution and nowcast the data.
+It is referred to as a triangle because the data for the more recent data entries are incomplete which gives the matrix a triangular shape.
+
+We can construct the reporting triangle from onsets ($N_{t}$) as follows:
+$$
+N_{t} = \sum_{d=0}^{D} n_{t,d}
+$$
+
+Where $n_{t,d}$ is the number of onsets on day $t$ that are reported on day $t-d$ and $D$ represents the maximum delay between date of reference and time of report which in theory could be infinite but in practice we set to a finite value to make the model identifiable and computationally feasible.
+We can now construct a model to estimate $n_{t,d}$,
+
+$$
+ n_{t,d} \mid \lambda_{t},p_{t,d} \sim \text{Poisson} \left(\lambda_{t} \times p_{t,d} \right),\ t=1,...,T.
+$$
+
+where $\lambda_{t}$ is the expected number of onsets on day $t$ and $p_{t,d}$ is the probability that an onset on day $t$ is reported on day $t-d$.
+Here $\lambda_{t}$ is the same as the expected number of onsets on day $t$ in the simple nowcasting model above so we again modelled it using a geometric random walk for now.
+We model $p_{t,d}$ as a [Dirichlet distribution](https://distribution-explorer.github.io/multivariate_continuous/dirichlet.html) as it is a distribution over probabilities.
+$p_{t,d}$ is equivalent to the reporting delays we have been using as fixed quantities so far but now estimated within the model.
+In most real-world settings we would want to use our domain expertise to inform the prior distribution of $p_{t,d}$.
+
+## Simulating the reporting triangle
+
+Now that we are aiming to jointly estimate the delay distribution we need additional data.
+We can simulate this data by using the same generative process as above but now also simulating the reporting delays.
+
+Once again we generate our simulated onset dataset:
+
+```{r, load-simulated-onset}
+gen_time_pmf <- make_gen_time_pmf()
+ip_pmf <- make_ip_pmf()
+onset_df <- simulate_onsets(
+ make_daily_infections(infection_times), gen_time_pmf, ip_pmf
+)
+head(onset_df)
+cutoff <- 71
+```
+
+We also need to simulate the reporting delays:
+
+```{r simulate-reporting-delays}
+reporting_delay_pmf <- censored_delay_pmf(
+ rlnorm, max = 15, meanlog = 1, sdlog = 0.5
+)
+plot(reporting_delay_pmf)
+```
+
+We can then simulate the reporting triangle:
+
+```{r simulate-reporting-triangle}
+reporting_triangle <- onset_df |>
+ filter(day < cutoff) |>
+ mutate(
+ reporting_delay = list(
+ tibble(d = 0:15, reporting_delay = reporting_delay_pmf)
+ )
+ ) |>
+ unnest(reporting_delay) |>
+ mutate(
+ reported_onsets = rpois(n(), onsets * reporting_delay)
+ ) |>
+ mutate(reported_day = day + d)
+```
+
+We also need to update our simulated truth data to include the Poisson observation error we are assuming is part of the observation process.
+
+```{r update-simulated-onset}
+noisy_onsets_df <- reporting_triangle |>
+ summarise(noisy_onsets = sum(reported_onsets), .by = day)
+```
+
+As we only partially observe the reporting triangle we need to filter it to only include the data we have observed:
+
+```{r filter-reporting-triangle}
+filtered_reporting_triangle <- reporting_triangle |>
+ filter(reported_day <= max(day))
+```
+
+Finally, we sum the filtered reporting triangle to get the counts we actually observe.
+
+```{r counts-observed}
+available_onsets <- filtered_reporting_triangle |>
+ summarise(available_onsets = sum(reported_onsets), .by = day)
+```
+
+## Fitting the joint model
+
+As usual we start by loading the model:
+
+```{r stan-joint-nowcast}
+joint_mod <- nfidd_cmdstan_model("joint-nowcast")
+joint_mod
+```
+
+::: {.callout-tip collapse="true"}
+## Model details
+This time we won't go into details of the model.
+For now, it is important that you understand the concept but as the models get more complex we hope that you trust us that the model does what we describe above.
+
+Once thing to note is that we are now fitting the initial number of symptom onsets (`init_onsets`).
+This is different from earlier when we had to pass the initial number of infections (`I0`) as data.
+In most situations this number would be unknown so what we do here is closer to what one would do in the real world.
+:::
+
+We then fit it do data:
+
+```{r joint-nowcast-fit, results = 'hide', message = FALSE}
+joint_data <- list(
+ n = length(unique(filtered_reporting_triangle$day)), # number of days
+ m = nrow(filtered_reporting_triangle), # number of reports
+ p = filtered_reporting_triangle |>
+ group_by(day) |>
+ filter(d == max(d)) |>
+ mutate(d = d + 1) |>
+ pull(d), # number of observations per day
+ obs = filtered_reporting_triangle$reported_onsets, # observed symptom onsets
+ d = 16 # number of reporting delays
+)
+joint_nowcast_fit <- joint_mod$sample(data = joint_data, parallel_chains = 4)
+```
+
+```{r joint-nowcast-fit-summary}
+joint_nowcast_fit
+```
+
+::: {.callout-important}
+One benefit of this model is that because we have decomposed the data into the reporting triangle we can make a nowcast that uses the data we have available augmented with predictions from the model.
+This should give us far more accurate uncertainty estimates than the simple nowcasting models above (see `stan/functions/combine_obs_with_predicted_obs_rng.stan` but note the code is fairly involved).
+:::
+
+We now extract this nowcast:
+
+```{r joint-nowcast}
+joint_nowcast_onsets <- joint_nowcast_fit |>
+ gather_draws(nowcast[day]) |>
+ ungroup() |>
+ filter(.draw %in% sample(.draw, 100))
+```
+
+Finally, we can plot the nowcast alongside the observed data:
+
+```{r plot-joint-nowcast}
+ggplot(joint_nowcast_onsets, aes(x = day)) +
+ geom_col(
+ data = noisy_onsets_df, mapping = aes(y = noisy_onsets), alpha = 0.6
+ ) +
+ geom_line(mapping = aes(y = .value, group = .draw), alpha = 0.1) +
+ geom_point(data = available_onsets, mapping = aes(y = available_onsets))
+```
+
+:::{.callout-tip}
+Reminder: The points in this plot represent the data available when the nowcast was made (and so are truncated) whilst the bars represent the finally reported data (a perfect nowcast would exactly reproduce these).
+:::
+
+::: {.callout-tip}
+## Take 5 minutes
+Look back at the last three nowcasts. How do they compare? What are the advantages and disadvantages of each? Could we improve the nowcasts further?
+:::
+
+::: {.callout-note collapse="true"}
+## Solution
+- The simple nowcast struggled to capture the generative process of the data and so produced poor nowcasts.
+The nowcast with the geometric random walk was better but still struggled to capture the generative process of the data.
+The joint nowcast was the best of the three as it properly handled the uncertainty and allowed us to fit the delay distribution versus relying on known delays.
+- However, the joint nowcast is still quite simple (in the sense that no detailed mechanism or reporting process is being modelled) and so may struggle to capture more complex patterns in the data.
+In particular the prior model for the geometric random walk assumes that onsets are the same as the previous day with some statistical noise.
+This may not be a good assumption in a rapidly changing epidemic (where the reproduction number is not near 1).
+- In addition, whilst we say it is "quite simple" as should be clear from the code it is quite complex and computationally intensive.
+This is because we are fitting a model to the reporting triangle which is a much larger data set and so the model is relatively quite slow to fit.
+:::
+
+
+# Putting it all together: Estimating the reproduction number, nowcasting, and joint estimation of delay distributions
+
+::: {.callout-note}
+This section contains a lot of code and is quite complex. It is not necessary to understand all of it to get the main points of the session. We recommend reading through it to get a sense of how all the pieces fit together but don't worry if you don't understand all of it.
+:::
+
+In the previous sessions, we have seen how to estimate the reproduction number and how to nowcast the data.
+We can now put these two pieces together to estimate the reproduction number and nowcast the data jointly.
+This should allow us to produce more accurate nowcasts as we can use the information from the reproduction number to inform the nowcast and vice versa.
+
+As in the [renewal sesssion](R-estimation-and-the-renewal-equation) we need to define the generation time distribution and a incubation period distribution.
+We will use the same distributions as in the [renewal session](R-estimation-and-the-renewal-equation) for simplicity.
+These are:
+
+```{r gt}
+plot(gen_time_pmf)
+```
+
+and
+
+```{r ip}
+plot(ip_pmf)
+```
+
+We now load in the model:
+
+```{r stan-joint-nowcast-rt}
+joint_rt_mod <- nfidd_cmdstan_model("joint-nowcast-with-r")
+joint_rt_mod
+```
+
+::: {.callout-tip}
+## Take 2 minutes
+Familiarise yourself with the model above.
+Can you see how it combines the nowcasting and the estimation of the reproduction number?
+Can you suggest how you swap in the simple nowcasting model whilst keeping the estimation of the reproduction number?
+:::
+
+::: {.callout-note collapse="true"}
+## Solution
+Essentially rather than using `observe_onsets_with_delay.stan` we would use `condition_onsets_by_report.stan` and pass in the proportion reported as a data.
+This would allow us to use the simple nowcasting model whilst still estimating the reproduction number.
+We would also remove the `generated quantities` block as we are not nowcasting the data and simplify the observations to just the number of onsets.
+:::
+
+Now let's fit the final model for this session!
+
+```{r joint-nowcast-rt-fit, results = 'hide', message = FALSE}
+joint_rt_data <- c(joint_data,
+ list(
+ gen_time_max = length(gen_time_pmf),
+ gen_time_pmf = gen_time_pmf,
+ ip_max = length(ip_pmf) - 1,
+ ip_pmf = ip_pmf,
+ h = 0 # this is a small easter egg for the attentive reader
+ )
+)
+joint_rt_fit <- joint_rt_mod$sample(
+ data = joint_rt_data, parallel_chains = 4,
+ adapt_delta = 0.95,
+ init = \() list(init_R = 0, rw_sd = 0.01)
+)
+```
+
+::: {.callout-tip}
+We use `adapt_delta = 0.95` here as this is a relatively complex model with a difficult to explore posterior. Increasing this setting decreases the step size of the sampler and so makes it easier for it to explore the posterior. The downside is that fitting then takes longer.
+:::
+
+```{r joint-nowcast-rt-fit-summary}
+joint_rt_fit
+```
+
+First we can extract the nowcast and plot the nowcast alongside the observed data:
+```{r joint-nowcast-with-r}
+joint_nowcast_with_r_onsets <- joint_rt_fit |>
+ gather_draws(nowcast[day]) |>
+ ungroup() |>
+ filter(.draw %in% sample(.draw, 100))
+```
+
+```{r plot-joint-nowcast-with-r}
+ggplot(joint_nowcast_with_r_onsets, aes(x = day)) +
+ geom_col(
+ data = noisy_onsets_df, mapping = aes(y = noisy_onsets), alpha = 0.6
+ ) +
+ geom_line(mapping = aes(y = .value, group = .draw), alpha = 0.1) +
+ geom_point(data = available_onsets, mapping = aes(y = available_onsets))
+```
+
+We can also extract the reproduction number and plot it:
+
+```{r joint-rt}
+joint_rt <- joint_rt_fit |>
+ gather_draws(R[day]) |>
+ ungroup() |>
+ filter(.draw %in% sample(.draw, 100))
+
+ggplot(joint_rt, aes(x = day, y = .value, group = .draw)) +
+ geom_line(alpha = 0.1)
+```
+
+::: {.callout-tip}
+## Take 2 minutes
+What do you think of the nowcast now?
+Does it look better than the previous one?
+What about the reproduction number?
+:::
+
+::: {.callout-note collapse="true"}
+## Solution
+- Whilst the majority of the nowcast is similar we see that the nowcast for days nearer to the present is more accurate as this model can capture the trend in infections and account for delays from infection to onset and onset to report.
+- The key takeaway from the reproduction number plot is that it looks similar to the one we estimated in the [renewal session](R-estimation-and-the-renewal-equation).
+This is because we have accounted for the truncation (otherwise it would be spuriously decreasing towards the end of the timeseries).
+:::
+
+# Going further
+
+- The simple nowcast models we showed here assumed perfect knowledge of the delay distribution. What happens when you instead use an estimate of the delay distribution from the data? Try and do this using methods from [session on biases in delay distributions](sessions/biases-in-delay-distributions) and see how it affects the simple nowcast models.
+- Despite being fairly involved the joint nowcast model we used here is still quite simple and may struggle to capture more complex patterns in the data.
+In practice more complex methods are often needed to account for structure in the reporting process, time-varying delay distributions, or delays that vary by other factors (such as the age of cases).
+- The [`epinowcast`](https://package.epinowcast.org/) package implements a more complex version of the model we have used here. It is designed to be highly flexible and so can be used to model a wide range of different data sets. You will have the opportunity to try it in a later session.
+- This session focussed on the role of the generative process in nowcasting. This is an area of active research but [this paper](https://journals.plos.org/ploscompbiol/article?id=10.1371/journal.pcbi.1012021) gives a good overview of the current state of the art.
diff --git a/sessions/methods-in-the-real-world.qmd b/sessions/methods-in-the-real-world.qmd
new file mode 100644
index 0000000..bd7bf5a
--- /dev/null
+++ b/sessions/methods-in-the-real-world.qmd
@@ -0,0 +1,8 @@
+---
+title: "Methods in the real world"
+order: 12
+---
+
+# Objectives
+
+The aim of this session is to provide an overview of the different methods that are used in the real world from the perspective of the particpants and course instructors.
diff --git a/sessions/nowcasting.qmd b/sessions/nowcasting.qmd
new file mode 100644
index 0000000..5bbe93a
--- /dev/null
+++ b/sessions/nowcasting.qmd
@@ -0,0 +1,397 @@
+---
+title: "Nowcasting concepts"
+order: 6
+---
+
+# Introduction
+
+So far we've explored the delays and biases of real-time outbreak data, started to correct for these, and considered the underlying process that drives the evolution of an outbreak (looking at the reproduction number and renewal equation). Next, we'll focus on predicting new information about how an outbreak is evolving in the present and future.
+
+We know that we have incomplete information in the present because of delays in the observation process (reporting delays). The aim of nowcasting is to predict what an epidemiological time series will look like *after all delayed reports* are in, for which we need to account for the delays and biases we've already considered.
+
+## Slides
+
+- [Introduction to nowcasting](slides/introduction-to-nowcasting)
+
+## Objectives
+
+This session aims to introduce the concept of _nowcasting_, and see how we can perform a nowcast if we know the underlying delay distribution.
+
+::: {.callout-note collapse="true"}
+
+# Setup
+
+## Source file
+
+The source file of this session is located at `sessions/nowcasting.qmd`.
+
+## Libraries used
+
+In this session we will use the `nfidd` package to load a data set of infection times and access stan models and helper functions, the `dplyr` and `tidyr` packages for data wrangling, `ggplot2` library for plotting, and the `tidybayes` package for extracting results of the inference.
+
+```{r libraries, message = FALSE}
+library("nfidd")
+library("dplyr")
+library("tidyr")
+library("ggplot2")
+library("tidybayes")
+```
+
+::: {.callout-tip}
+The best way to interact with the material is via the [Visual Editor](https://docs.posit.co/ide/user/ide/guide/documents/visual-editor.html) of RStudio.
+:::
+
+## Initialisation
+
+We set a random seed for reproducibility.
+Setting this ensures that you should get exactly the same results on your computer as we do.
+We also set an option that makes `cmdstanr` show line numbers when printing model code.
+This is not strictly necessary but will help us talk about the models.
+
+```{r}
+set.seed(123)
+options(cmdstanr_print_line_numbers = TRUE)
+```
+
+:::
+
+# Simulating delayed reporting
+
+Epidemiological data is not usually available immediately for analysis.
+Instead, data usually gets collated at different levels of a healthcare or health surveillance system, cleaned, checked before being aggregated and/or anonymised and ultimately shared with an analyst.
+We call the _reporting time_ the time a data point (e.g. a time or day of symptom onset or a time or day of hospitalisation) has entered the data set used for some analysis.
+Similar to the data discussed in the preceding session, this time is often only available as a date, i.e. censored at the scale of a day.
+
+We can simulate this reporting process.
+Let us assume that the symptom onsets are reported with a delay and that this delay is characterised by a lognormal distribution with meanlog 1 and sdlog 0.5:
+To do so, we perform a very similar simulation to what we did in the [session on delay distributions](delay-distributions#simulating-delayed-epidemiological-data), except now we don't simulate hospitalisations but reports of symptom onsets:
+
+```{r onset_report}
+data(infection_times)
+df <- infection_times |>
+ mutate(
+ onset_time = infection_time + rgamma(n(), shape = 5, rate = 1),
+ report_time = onset_time + rlnorm(n(), meanlog = 1, sdlog = 0.5)
+ )
+```
+
+We then assume that we're 70 days into the outbreak, i.e. we only consider observations with a reporting time less than 71 - other symptom onset may have already happened, but we have not observed them yet.
+
+
+```{r truncate_reports}
+cutoff <- 71
+df_co <- df |>
+ filter(report_time < cutoff)
+```
+
+We can now convert this to a time series of symptom onsets and reports:
+
+```{r aggregate}
+## create time series of infections, onsets, and reports
+df_co <- df_co |>
+ transmute(
+ infection_day = floor(infection_time),
+ onset_day = floor(onset_time),
+ report_day = floor(report_time)
+ )
+
+infection_ts <- df_co |>
+ count(day = infection_day, name = "infections")
+onset_ts <- df_co |>
+ count(day = onset_day, name = "onsets")
+reports_ts <- df_co |>
+ count(day = report_day, name = "reports")
+
+all_days <- expand_grid(day = seq(0, cutoff - 1)) |>
+ full_join(infection_ts, by = "day") |>
+ full_join(onset_ts, by = "day") |>
+ full_join(reports_ts, by = "day") |>
+ replace_na(list(onsets = 0, reports = 0))
+```
+
+Plotting these, we get
+
+```{r ts_plot, fig.height = 10}
+combined <- all_days |>
+ pivot_longer(c(onsets, reports, infections), names_to = "variable")
+ ggplot(combined, aes(x = day, y = value)) +
+ facet_grid(variable ~ .) +
+ geom_col()
+```
+
+Looking at the three plots in isolation we would conclude very different things about the epidemic: symptom onsets seem to have flattened off and perhaps are going down, whereas reports are increasing rapidly.
+
+This apparent contradiction appears because onsets are reported with a delay.
+By cutting off at a certain _reporting_ date, we do not observe many of the recent symptom onsets that are yet to be be reported.
+We can see that if we plot the final data set alongside the cut-off one:
+
+```{r plot_cut_final}
+# Use full outbreak dataset
+final <- df |>
+ transmute(onset_day = floor(onset_time))
+final_onset_ts <- final |>
+ count(day = onset_day, name = "onsets")
+final_all_days <- expand_grid(day = seq(0, max(final_onset_ts$day))) |>
+ full_join(final_onset_ts, by = "day") |>
+ replace_na(list(onsets = 0)) |>
+ mutate(cutoff = "final")
+intermediate <- combined |>
+ filter(variable == "onsets") |>
+ select(-variable) |>
+ rename(onsets = value) |>
+ mutate(cutoff = "70 days")
+combined_cutoffs <- rbind(
+ intermediate,
+ final_all_days
+)
+ggplot(combined_cutoffs, aes(x = day, y = onsets, colour = cutoff)) +
+ geom_line() +
+ scale_colour_brewer(palette = "Dark2") +
+ geom_vline(xintercept = cutoff, linetype = "dashed")
+```
+
+As we can see, even though it may much seem like the epidemic curve is going down, in fact in the final data set one can see that at the time symptom onsets were still increasing.
+The apparent decline towards the present (indicated by a dashed vertical line) was caused by the delay in reporting.
+
+::: {.callout-note}
+## Plotting and analysing data by report date
+Looking at the plots one might suggest plotting and analysing the data by date of reporting which correctly showed the data to be still increasing and which should, by definition, not be subject to future changes.
+
+This can sometimes be a sensible way to visualise the data.
+However, reporting might itself be subject to biases such as breaks during the weekend, holidays etc or reporting delays may vary over time.
+At the same time, when it comes to capacity or intervention planning we may need to know how many people e.g. become sick on any given day and will thus present to the healthcare system rather than how many will be reported.
+:::
+
+::: {.callout-tip}
+Estimating the "true curve" (i.e. what we expect to see once the data are complete at a future date) of the time series of _epidemiologically relevant events_ from a potentially truncated epidemiological curve and information about the delays is what is usually called "nowcasting".
+:::
+
+# Nowcasting with a known delay
+
+## The simplest possible nowcasting model
+
+Here we assume that the delay distribution is known and that we can use it to nowcast the most recent data. In practice, the delay distribution is often not known and needs to be estimated from the data. We could do this using methods from [the session on biases in delay distributions](sessions/biases-in-delay-distributions).
+
+In the [session on convolutions](using-delay-distributions-to-model-the-data-generating-process-of-an-epidemic#estimating-a-time-series-of-infections) we used delay distributions convolved with the infection times to estimate the time series of symptom onsets. A simple way to nowcast is to use the same approach but using the cumulative distribution function of the delay distribution rather than the probability density function and only apply it to the most recent data as this is the only data that can be subject to change (due to delays in reporting).
+
+We will build intuition for this as usual using simulation. First we define the proportion reported using a delay distribution up to 15 days, again using a lognormal distribution with meanlog 1 and sdlog 0.5:
+
+```{r}
+proportion_reported <- plnorm(1:15, 1, 0.5)
+plot(proportion_reported)
+```
+
+::: {.callout-tip}
+## The `plnorm` function
+The `plnorm()` function is related to the `rlnorm()` function we used earlier to simulate the individual level reporting delay, but instead it gives the cumulative distribution function rather than random samples. That is, it gives us the probability that a report is made on day 1 or earlier, day 2 or earlier, etc.
+:::
+
+We can now use this delay distribution to nowcast the most recent data. Here we use the same simulation approach as in the [renewal session](R-estimation-and-the-renewal-equation) (here we have created helper functions `make_gen_time_pmf()` and `make_ip_pmf()` to make it easier to re-use; we recommend to have a look at what these functions do), and apply the `reporting_delay` to the last 15 days of data.
+
+```{r, load-simulated-onset}
+gen_time_pmf <- make_gen_time_pmf()
+ip_pmf <- make_ip_pmf()
+onset_df <- simulate_onsets(
+ make_daily_infections(infection_times), gen_time_pmf, ip_pmf
+)
+reported_onset_df <- onset_df |>
+ filter(day < cutoff) |>
+ mutate(proportion_reported = c(rep(1, n() - 15), rev(proportion_reported)),
+ reported_onsets = rpois(n(), onsets * proportion_reported)
+ )
+tail(reported_onset_df)
+```
+
+::: {.callout-tip}
+## Take 5 minutes
+Spend a few minutes trying to understand the code above. What is the `proportion_reported`? What is the `reported_onsets`?
+:::
+
+::: {.callout-note collapse="true"}
+## Solution
+- The `proportion_reported` is the cumulative distribution function of the delay distribution. It gives the probability that a report is made on day 1 or earlier, day 2 or earlier, etc. Note that for days more that 15 days into the past
+- The `reported_onsets` are the number of onsets that are reported on each day. This is calculated by multiplying the number of onsets by the proportion of onsets that are reported on each day. It has Poisson noise added to it to simulate the stochasticity in the reporting process.
+
+```{r plot-proportion-reported}
+reported_onset_df |>
+ ggplot(aes(x = day, y = reported_onsets)) +
+ geom_col()
+```
+:::
+
+We can now fit our first nowcasting model. Here we assume exactly the same generative process as we used for simulation and model the number of onsets as independent draws from a normal distribution.
+
+```{r stan-simple-nowcast}
+mod <- nfidd_cmdstan_model("simple-nowcast")
+mod
+```
+
+::: {.callout-tip}
+## Take 5 minutes
+Familiarise yourself with the model above. What does it do?
+:::
+
+::: {.callout-note collapse="true"}
+## Solution
+- On line 2 we define a new function `condition_onsets_by_report.stan` which takes the number of onsets and reports and the delay distribution as input and returns the nowcasted number of onsets.
+- On line 17, this function is used to calculate the nowcasted number of onsets and this is then used in the likelihood.
+- On line 21, we define the generative process for the number of onsets. Here we assume that onsets are independent with each drawn from a normal distribution.
+:::
+
+Once again we can generate estimates from this model:
+
+```{r nowcast_fit, results = 'hide', message = FALSE}
+data <- list(
+ n = nrow(reported_onset_df) - 1,
+ obs = reported_onset_df$reported_onsets[-1],
+ report_max = length(proportion_reported) - 1,
+ report_cdf = proportion_reported
+)
+simple_nowcast_fit <- mod$sample(data = data, parallel_chains = 4)
+```
+
+```{r nowcast_fit_summary}
+simple_nowcast_fit
+```
+
+We can now plot onsets alongside those nowcasted by the model:
+
+```{r simple-nowcast-onsets}
+nowcast_onsets <- simple_nowcast_fit |>
+ gather_draws(onsets[day]) |>
+ ungroup() |>
+ filter(.draw %in% sample(.draw, 100)) |>
+ mutate(day = day + 1)
+```
+
+```{r plot_nowcast}
+ggplot(nowcast_onsets, aes(x = day)) +
+ geom_line(mapping = aes(y = .value, group = .draw), alpha = 0.1) +
+ geom_col(data = reported_onset_df, mapping = aes(y = onsets), alpha = 0.6) +
+ geom_point(data = reported_onset_df, mapping = aes(y = reported_onsets))
+```
+
+:::{.callout-tip}
+The points in this plot represent the data available when the nowcast was made (and so are truncated) whilst the bars represent the finally reported data (a perfect nowcast would exactly reproduce these).
+:::
+
+::: {.callout-tip}
+As we found in the [using delay distributions to model the data generating process of an epidemic session](using-delay-distributions-to-model-the-data-generating-process-of-an-epidemic#estimating-a-time-series-of-infections), this simple model struggles to recreate the true number of onsets. This is because it does not capture the generative process of the data (i.e. the transmission process and delays from infection to onset). In the next section we will see how we can use a model that does capture this generative process to improve our nowcasts.
+:::
+
+## Adding in a geometric random walk to the nowcasting model
+
+As we saw in the [session on the renewal equation](R-estimation-and-the-renewal-equation), a geometric random walk is a simple way to model multiplicative growth. Adding this into our simple nowcasting model may help us to better capture the generative process of the data and so produce a better nowcast.
+
+We first load the model
+
+```{r stan-nowcast-with-rw}
+rw_mod <- nfidd_cmdstan_model("simple-nowcast-rw")
+rw_mod
+```
+
+and then fit it
+
+```{r rw-nowcast-fit, results = 'hide', message = FALSE}
+rw_nowcast_fit <- rw_mod$sample(data = data, parallel_chains = 4)
+```
+
+```{r rw-nowcast-fit-summary}
+rw_nowcast_fit
+```
+
+
+Again we can extract the nowcasted onsets and plot them alongside the observed data:
+
+```{r rw-nowcast-onsets}
+rw_nowcast_onsets <- rw_nowcast_fit |>
+ gather_draws(onsets[day]) |>
+ ungroup() |>
+ filter(.draw %in% sample(.draw, 100)) |> ## sample 100 iterations randomly
+ mutate(day = day + 1)
+```
+
+```{r rw-plot_nowcast}
+ggplot(rw_nowcast_onsets, aes(x = day)) +
+ geom_col(data = reported_onset_df, mapping = aes(y = onsets), alpha = 0.6) +
+ geom_line(mapping = aes(y = .value, group = .draw), alpha = 0.1) +
+ geom_point(data = reported_onset_df, mapping = aes(y = reported_onsets))
+```
+
+::: {.callout-tip}
+## Take 2 minutes
+What do you think of the nowcast now? Does it look better than the previous one?
+:::
+
+::: {.callout-note collapse="true"}
+## Solution
+- The nowcast better matches the ultimately observed data.
+The geometric random walk allows the model to capture the multiplicative growth in the data and so better capture that current indidence is related to past incidence.
+- This should be particularly true when the data is more truncated (i.e nearer to the date of the nowcast) as the geometric random walk allows the model to extrapolate incidence based on previous incidence rather than relying on the prior distribution as the simpler model did.
+- However, the model is still quite simple and so may struggle to capture more complex patterns in the data.
+In particular, the prior model for the geometric random walk assumes that onsets are the same as the previous day with statistical noise.
+This may not be a good assumption in a rapidly changing epidemic (where the reproduction number is not near 1).
+:::
+
+## What happens if we get the delay distribution wrong?
+
+In practice, we often do not know the delay distribution and so need to estimate it using the data at hand.
+In the [session on biases in delay distributions](sessions/biases-in-delay-distributions) we saw how we could do this using individual-level records.
+We will now look at what happens if we get the delay distribution wrong.
+
+We use the same data as before but now assume that the delay distribution is a gamma distribution with shape 2 and rate 3.
+This is a very different distribution to the lognormal distribution we used to simulate the data.
+
+```{r gamma-dist}
+wrong_proportion_reported <- pgamma(1:15, 2, 3)
+plot(wrong_proportion_reported)
+```
+
+We first need to update the data to use this new delay distribution:
+
+```{r wrong-delay-data}
+wrong_delay_data <- data
+wrong_delay_data$report_cdf <- wrong_proportion_reported
+```
+
+We now fit the nowcasting model with the wrong delay distribution:
+
+```{r gamma-nowcast-fit, results = 'hide', message = FALSE}
+gamma_nowcast_fit <- rw_mod$sample(data = wrong_delay_data, parallel_chains = 4)
+```
+
+```{r gamma-nowcast-fit-summary}
+gamma_nowcast_fit
+```
+
+
+Again we can extract the nowcast of symptom onsets and plot it alongside the observed data:
+
+```{r gamma-nowcast-onsets}
+gamma_nowcast_onsets <- gamma_nowcast_fit |>
+ gather_draws(onsets[day]) |>
+ ungroup() |>
+ filter(.draw %in% sample(.draw, 100)) |>
+ mutate(day = day + 1)
+```
+
+```{r gamma-plot_nowcast}
+ggplot(gamma_nowcast_onsets, aes(x = day)) +
+ geom_col(data = reported_onset_df, mapping = aes(y = onsets), alpha = 0.6) +
+ geom_line(mapping = aes(y = .value, group = .draw), alpha = 0.1) +
+ geom_point(data = reported_onset_df, mapping = aes(y = reported_onsets))
+```
+
+::: {.callout-tip}
+## Take 2 minutes
+What do you think of the nowcast now? How would you know you had the wrong delay if you didn't have the true delay distribution?
+:::
+
+::: {.callout-note collapse="true"}
+## Solution
+- The nowcast now looks very different to the observed data. This is because the model is using the wrong delay distribution to nowcast the data.
+- If you didn't have the true delay distribution you would not know that you had the wrong delay distribution. This is why it is important to estimate the delay distribution from the data.
+- In practice, you would likely compare the nowcast to the observed data and if they did not match you would consider whether the delay distribution was the cause.
+:::
+
+# Wrap up
diff --git a/sessions/slides/closing.qmd b/sessions/slides/closing.qmd
new file mode 100644
index 0000000..ac33655
--- /dev/null
+++ b/sessions/slides/closing.qmd
@@ -0,0 +1,90 @@
+---
+title: "End of course summary"
+author: "Nowcasting and forecasting of infectious disease dynamics"
+engine: knitr
+format:
+ revealjs:
+ output: slides/closing.html
+ footer: "End of course summary"
+ slide-level: 3
+---
+
+### Aim of this course:
+
+How can we use data typically collected in an outbreak to answer questions like
+
+- what **is** the number of cases now? (*nowcasting*)
+- is it rising/falling and by how much? (*$R_t$ estimation*)
+- what does this mean for the near future (*forecasting*)
+
+in real time.
+
+### Timeline
+
+- delay distributions and how to estimate them (day 1)
+- $R_t$ estimation and the generation interval (day 1)
+- nowcasting (day 2)
+- forecasting and evaluation, ensemble methods (day 2)
+- applications (day 3)
+
+# Key takeaways
+
+### Day 1: Delay distributions {.smaller}
+
+- delays play a fundamental role in nowcasting/forecasting
+- we characterise them with probability distributions
+- estimating delays requires correction for biases due to
+ - **double interval censoring** (daily data)
+ - **right truncation** (real-time analysis)
+
+
+
+### Day 1 (cont.): Convolutions {.smaller}
+
+- we can use **convolutions** to model delays at the population scale
+- when doing so we need to make sure to account for **double interval censoring**
+- **$R_t$ estimation** using the renewal equation is a convolution problem
+- improving the generative model leads to improvements in estimation (**geometric random walk** vs. independent priors)
+
+
+
+### Day 2: Nowcasting {.smaller}
+
+- **nowcasting** is the task of predicting what data will look once delays have resolved
+- it is a **right truncation problem** (same as discussed before)
+- a **joint generative model** can combine delay estimation, nowcasting and $R_t$ estimation
+
+
+
+### Day 2 (cont.): Forecasting {.smaller}
+
+- **forecasting** is the task of making unconditional statements about the future
+- meaningful forecasts are **probabilistic**
+- we can assess forecasts using **proper scoring rules**
+- a wide range of methods are available for making forecasts
+- we can use visualisation and scoring to understand the predictive performance of different models
+
+
+
+### Day 3: Applications {.smaller}
+
+- the methods introduced here have **wide applications** in infectious disease epidemiology
+- **open-source tools** are available to make this task easier in practice
+
+### Outlook {.smaller}
+
+- it is worth trying some of these methods here in practice to learn more about typical nowcast/forecast performance and intricacies of infectious disease data
+- one way of doing so is by contributing to forecast hubs
+
+
+
+[https://respicast.ecdc.europa.eu/](https://respicast.ecdc.europa.eu/)
+
+### Feedback {.smaller}
+
+- please tell us if you enjoyed the course, what worked / didn't work etc.
+- we will send out a survey for feedback
+
+# Thank you for attending!
+
+[Return to the session](../end-of-course-summary-and-discussion)
diff --git a/sessions/slides/convolutions.qmd b/sessions/slides/convolutions.qmd
new file mode 100644
index 0000000..89ae3d6
--- /dev/null
+++ b/sessions/slides/convolutions.qmd
@@ -0,0 +1,75 @@
+---
+title: "Delay distributions at the population level"
+author: "Nowcasting and forecasting of infectious disease dynamics"
+engine: knitr
+format:
+ revealjs:
+ output: slides/introduction-to-biases-in-epidemiological-delays.html
+ footer: "Delay distributions at the population level"
+---
+
+## Individual delays
+
+If $f(t)$ is our delay distribution then
+
+$$
+p(y_i) = f(y_i - x_i)
+$$
+
+is the probability that *secondary* event of individual $i$ happens at time $y_i$ given its primary event happened at $x_i$.
+
+## Population level counts
+
+The expected number of individuals $S_t$ that have their secondary event at time $t$ can then be calculated as the sum of these probabilities
+
+$$
+S_t = \sum_i f_{t - x_i}
+$$
+
+. . .
+
+**Note:** If $S_t$ is in discrete time steps then $f_t$ needs to be a discrete probability distribution.
+
+## Population level counts
+
+If the number of individuals $P_{t'}$ that have their primary event at time $t'$ then we can rewrite this as
+
+$$
+S_t = \sum_{t'} P_{t'} f_{t - t'}
+$$
+
+This operation is called a (discrete) **convolution** of $P$ with $f$.
+
+We can use convolutions with the delay distribution that applies at the *individual* level to determine *population-level* counts.
+
+## Example: infections to symptom onsets
+
+
+
+## Why use a convolution, not individual delays?
+
+- we don't always have individual data available
+- we often model other processes at the population level (such as transmission) and so being able to model delays on the same scale is useful
+- doing the computation at the population level requires fewer calculations (i.e. is faster)
+
+::: {.fragment .fade-in}
+- **however, a downside is that we won't have realistic uncertainty, especially if the number of individuals is small**
+:::
+
+## What if $f$ is continuous?
+
+Having moved to the population level, we can't estimate individual-level event times any more.
+
+Instead, we *discretise* the distribution (remembering that it is **double censored** - as both events are censored).
+
+This can be solved mathematically but in the session we will use simulation.
+
+## `r fontawesome::fa("laptop-code", "white")` Your Turn {background-color="#447099" transition="fade-in"}
+
+- Simulate convolutions with infection counts
+- Discretise continuous distributions
+- Estimate parameters numbers of infections from number of symptom onsets, using a convolution model
+
+#
+
+[Return to the session](../biases-in-delay-distributions)
diff --git a/sessions/slides/figures/bayesian_model.png b/sessions/slides/figures/bayesian_model.png
new file mode 100644
index 0000000..5a78d11
Binary files /dev/null and b/sessions/slides/figures/bayesian_model.png differ
diff --git a/sessions/slides/figures/bayesian_model_without_distributions.png b/sessions/slides/figures/bayesian_model_without_distributions.png
new file mode 100644
index 0000000..e0a0786
Binary files /dev/null and b/sessions/slides/figures/bayesian_model_without_distributions.png differ
diff --git a/sessions/slides/figures/cdc_flu_forecast_use.png b/sessions/slides/figures/cdc_flu_forecast_use.png
new file mode 100644
index 0000000..2820eb6
Binary files /dev/null and b/sessions/slides/figures/cdc_flu_forecast_use.png differ
diff --git a/sessions/slides/figures/censoring_1.png b/sessions/slides/figures/censoring_1.png
new file mode 100644
index 0000000..93dded6
Binary files /dev/null and b/sessions/slides/figures/censoring_1.png differ
diff --git a/sessions/slides/figures/censoring_2.png b/sessions/slides/figures/censoring_2.png
new file mode 100644
index 0000000..09b801f
Binary files /dev/null and b/sessions/slides/figures/censoring_2.png differ
diff --git a/sessions/slides/figures/censoring_final.png b/sessions/slides/figures/censoring_final.png
new file mode 100644
index 0000000..05f6b9d
Binary files /dev/null and b/sessions/slides/figures/censoring_final.png differ
diff --git a/sessions/slides/figures/complete_reporting_triangle.png b/sessions/slides/figures/complete_reporting_triangle.png
new file mode 100644
index 0000000..6e3483c
Binary files /dev/null and b/sessions/slides/figures/complete_reporting_triangle.png differ
diff --git a/sessions/slides/figures/crowdforecastr.png b/sessions/slides/figures/crowdforecastr.png
new file mode 100644
index 0000000..fc7e4c1
Binary files /dev/null and b/sessions/slides/figures/crowdforecastr.png differ
diff --git a/sessions/slides/figures/ferguson2020.png b/sessions/slides/figures/ferguson2020.png
new file mode 100644
index 0000000..5367af3
Binary files /dev/null and b/sessions/slides/figures/ferguson2020.png differ
diff --git a/sessions/slides/figures/germany_early.png b/sessions/slides/figures/germany_early.png
new file mode 100644
index 0000000..4bf1539
Binary files /dev/null and b/sessions/slides/figures/germany_early.png differ
diff --git a/sessions/slides/figures/germany_historical.png b/sessions/slides/figures/germany_historical.png
new file mode 100644
index 0000000..763c3c2
Binary files /dev/null and b/sessions/slides/figures/germany_historical.png differ
diff --git a/sessions/slides/figures/hub-ensemble-good.png b/sessions/slides/figures/hub-ensemble-good.png
new file mode 100644
index 0000000..a97824b
Binary files /dev/null and b/sessions/slides/figures/hub-ensemble-good.png differ
diff --git a/sessions/slides/figures/hub-ensemble-less-good.png b/sessions/slides/figures/hub-ensemble-less-good.png
new file mode 100644
index 0000000..f5d3dce
Binary files /dev/null and b/sessions/slides/figures/hub-ensemble-less-good.png differ
diff --git a/sessions/slides/figures/hub-metadata.png b/sessions/slides/figures/hub-metadata.png
new file mode 100644
index 0000000..b5ddbd3
Binary files /dev/null and b/sessions/slides/figures/hub-metadata.png differ
diff --git a/sessions/slides/figures/infections_onset_sketch.png b/sessions/slides/figures/infections_onset_sketch.png
new file mode 100644
index 0000000..0166243
Binary files /dev/null and b/sessions/slides/figures/infections_onset_sketch.png differ
diff --git a/sessions/slides/figures/linelist.png b/sessions/slides/figures/linelist.png
new file mode 100644
index 0000000..eb84d93
Binary files /dev/null and b/sessions/slides/figures/linelist.png differ
diff --git a/sessions/slides/figures/mechanism.png b/sessions/slides/figures/mechanism.png
new file mode 100644
index 0000000..1e2090f
Binary files /dev/null and b/sessions/slides/figures/mechanism.png differ
diff --git a/sessions/slides/figures/mechanism1.png b/sessions/slides/figures/mechanism1.png
new file mode 100644
index 0000000..cd4ff2e
Binary files /dev/null and b/sessions/slides/figures/mechanism1.png differ
diff --git a/sessions/slides/figures/mechanism2.png b/sessions/slides/figures/mechanism2.png
new file mode 100644
index 0000000..c08197c
Binary files /dev/null and b/sessions/slides/figures/mechanism2.png differ
diff --git a/sessions/slides/figures/mechanism3.png b/sessions/slides/figures/mechanism3.png
new file mode 100644
index 0000000..f5a121f
Binary files /dev/null and b/sessions/slides/figures/mechanism3.png differ
diff --git a/sessions/slides/figures/mechanism4.png b/sessions/slides/figures/mechanism4.png
new file mode 100644
index 0000000..ac5cae2
Binary files /dev/null and b/sessions/slides/figures/mechanism4.png differ
diff --git a/sessions/slides/figures/metcalf_lessler_fraught.png b/sessions/slides/figures/metcalf_lessler_fraught.png
new file mode 100644
index 0000000..efcbfce
Binary files /dev/null and b/sessions/slides/figures/metcalf_lessler_fraught.png differ
diff --git a/sessions/slides/figures/monkeypox_delayed.png b/sessions/slides/figures/monkeypox_delayed.png
new file mode 100644
index 0000000..6f7df5f
Binary files /dev/null and b/sessions/slides/figures/monkeypox_delayed.png differ
diff --git a/sessions/slides/figures/muni_model.png b/sessions/slides/figures/muni_model.png
new file mode 100644
index 0000000..99ee7fb
Binary files /dev/null and b/sessions/slides/figures/muni_model.png differ
diff --git a/sessions/slides/figures/nishiura2007.png b/sessions/slides/figures/nishiura2007.png
new file mode 100644
index 0000000..4349d8e
Binary files /dev/null and b/sessions/slides/figures/nishiura2007.png differ
diff --git a/sessions/slides/figures/nowcasting.png b/sessions/slides/figures/nowcasting.png
new file mode 100644
index 0000000..24d5689
Binary files /dev/null and b/sessions/slides/figures/nowcasting.png differ
diff --git a/sessions/slides/figures/pit-log-scale.png b/sessions/slides/figures/pit-log-scale.png
new file mode 100644
index 0000000..2914021
Binary files /dev/null and b/sessions/slides/figures/pit-log-scale.png differ
diff --git a/sessions/slides/figures/r_convolution_sketch.png b/sessions/slides/figures/r_convolution_sketch.png
new file mode 100644
index 0000000..b6739e6
Binary files /dev/null and b/sessions/slides/figures/r_convolution_sketch.png differ
diff --git a/sessions/slides/figures/reporting_triangle.png b/sessions/slides/figures/reporting_triangle.png
new file mode 100644
index 0000000..d8bb509
Binary files /dev/null and b/sessions/slides/figures/reporting_triangle.png differ
diff --git a/sessions/slides/figures/respicast-comparison.png b/sessions/slides/figures/respicast-comparison.png
new file mode 100644
index 0000000..ea0d0a5
Binary files /dev/null and b/sessions/slides/figures/respicast-comparison.png differ
diff --git a/sessions/slides/figures/respicast-forecasts.png b/sessions/slides/figures/respicast-forecasts.png
new file mode 100644
index 0000000..193b6e2
Binary files /dev/null and b/sessions/slides/figures/respicast-forecasts.png differ
diff --git a/sessions/slides/figures/respicast-multi.png b/sessions/slides/figures/respicast-multi.png
new file mode 100644
index 0000000..0a17ca2
Binary files /dev/null and b/sessions/slides/figures/respicast-multi.png differ
diff --git a/sessions/slides/figures/respicast-single.png b/sessions/slides/figures/respicast-single.png
new file mode 100644
index 0000000..8f99c3b
Binary files /dev/null and b/sessions/slides/figures/respicast-single.png differ
diff --git a/sessions/slides/figures/respicast.png b/sessions/slides/figures/respicast.png
new file mode 100644
index 0000000..ec7896f
Binary files /dev/null and b/sessions/slides/figures/respicast.png differ
diff --git a/sessions/slides/figures/truncation_1.png b/sessions/slides/figures/truncation_1.png
new file mode 100644
index 0000000..e018358
Binary files /dev/null and b/sessions/slides/figures/truncation_1.png differ
diff --git a/sessions/slides/figures/truncation_final.png b/sessions/slides/figures/truncation_final.png
new file mode 100644
index 0000000..db21ad1
Binary files /dev/null and b/sessions/slides/figures/truncation_final.png differ
diff --git a/sessions/slides/figures/truncation_prefinal.png b/sessions/slides/figures/truncation_prefinal.png
new file mode 100644
index 0000000..39529e3
Binary files /dev/null and b/sessions/slides/figures/truncation_prefinal.png differ
diff --git a/sessions/slides/figures/ward2022.png b/sessions/slides/figures/ward2022.png
new file mode 100644
index 0000000..84ea75a
Binary files /dev/null and b/sessions/slides/figures/ward2022.png differ
diff --git a/sessions/slides/figures/weather_forecast.png b/sessions/slides/figures/weather_forecast.png
new file mode 100644
index 0000000..8f0ad97
Binary files /dev/null and b/sessions/slides/figures/weather_forecast.png differ
diff --git a/sessions/slides/forecasting-as-an-epidemiological-problem.qmd b/sessions/slides/forecasting-as-an-epidemiological-problem.qmd
new file mode 100644
index 0000000..d5bfda7
--- /dev/null
+++ b/sessions/slides/forecasting-as-an-epidemiological-problem.qmd
@@ -0,0 +1,93 @@
+---
+title: "Forecasting as an epidemiological problem"
+author: "Nowcasting and forecasting of infectious disease dynamics"
+engine: knitr
+format:
+ revealjs:
+ output: slides/forecasting-as-an-epidemiological-problem.html
+ footer: "Forecasting as an epidemiological problem"
+ slide-level: 3
+---
+
+### Forecasting in infectious disease epidemiology
+
+{width=50%}
+
+[Metcalf & Lessler, *Science*, 2017](https://doi.org/10.1126/science.aam8335)
+
+### Using models for statements about the future {.smaller}
+
+Modelling the future can help decision making:
+
+- how many beds to we need?
+- where should we allocate vaccines?
+- where should we trial vaccines?
+
+However:
+
+- not all modelling is prediction
+- not all modelling of the future is forecasting
+
+### Different ways of modelling the future {.smaller}
+
+- **Nowcasts** make statements about current trends based on partial data
+
+- **Forecasts** are *unconditional* statements about the future: what *will happen*
+
+- **Scenarios** state what *would* happen under certain conditions
+
+### Why nowcast/forecast? {.smaller}
+
+- to create **situational awareness**
+ - nowcast: where are we now?
+ - forecast: where are we heading?
+
+### CDC use of influenza forecasts
+
+
+
+[CDC: About Flu Forecasting](https://www.cdc.gov/flu/weekly/flusight/how-flu-forecasting.htm)
+
+### Relationship with $R_t$ estimation {.smaller}
+
+- In infectious disease epidemiology, many relevant interventions and other changes affect the strength of **transmission**
+- Things that affect transmission don't affect the predicted outcomes directly, but via $R_t$
+- In that sense, predicting infections comes down to **predicting $R_t$**
+- Commonly, forecast models assume **no change** in $R_t$. Is this a good assumption?
+
+### Relationship with nowcasting {.smaller}
+
+- Nowcast: we have **some** data from the dates we're predicting
+- Forecast: we have **no** data from the dates we're predicting (usually: because they're in the future)
+
+### Forecasts are usually *probabilistic* {.smaller}
+
+- Because the future is uncertain, it is natural to express predictions in probabilities, e.g. there is a X% chance of exceeding Y hospital admissions.
+
+
+
+### Importance of evaluation {.smaller}
+
+- Because forecasts are unconditional (what will happen) we can compare them to data and see how well they did
+- Doing this allows us to answer question like
+ - Are our forecasts any good?
+ - How far ahead can we trust forecasts?
+ - Which model works best for making forecasts?
+- So-called **proper scoring rules** incentivise forecasters to express an honest belief about the future
+- Many proper scoring rules (and other metrics) are available to assess probabilistic forecasts
+
+### The forecasting paradigm {.smaller}
+
+#### Maximise *sharpness* subject to *calibration*
+
+- Statements about the future should be **correct** ("calibration")
+- Statements about the future should aim to have **narrow uncertainty** ("sharpness")
+
+## `r fontawesome::fa("laptop-code", "white")` Your Turn {background-color="#447099" transition="fade-in"}
+
+1. Start with a model we used before and use it to make a forecast (using stan)
+2. Evaluate the forecasts using proper scoring rules
+
+#
+
+[Return to the session](../forecasting-concepts)
diff --git a/sessions/slides/forecasting-models.qmd b/sessions/slides/forecasting-models.qmd
new file mode 100644
index 0000000..e868dba
--- /dev/null
+++ b/sessions/slides/forecasting-models.qmd
@@ -0,0 +1,170 @@
+---
+title: "Forecasting models"
+author: "Nowcasting and forecasting of infectious disease dynamics"
+engine: knitr
+format:
+ revealjs:
+ output: slides/forecasting-as-an-epidemiological-problem.html
+ footer: "Forecasting as an epidemiological problem"
+ slide-level: 3
+---
+
+### {.smaller}
+
+{width="70%"}
+
+:::: {.columns}
+
+::: {.column}
+
+- We can classify models by the level of mechanism they include
+- All of the model types we will introduce in the next few slides have been used for COVID-19 forecasting (the US and/or European COVID-19 forecast hub)
+
+**NOTE:** level of mechanism $\neq$ model complexity
+
+:::
+
+::: {.column}
+
+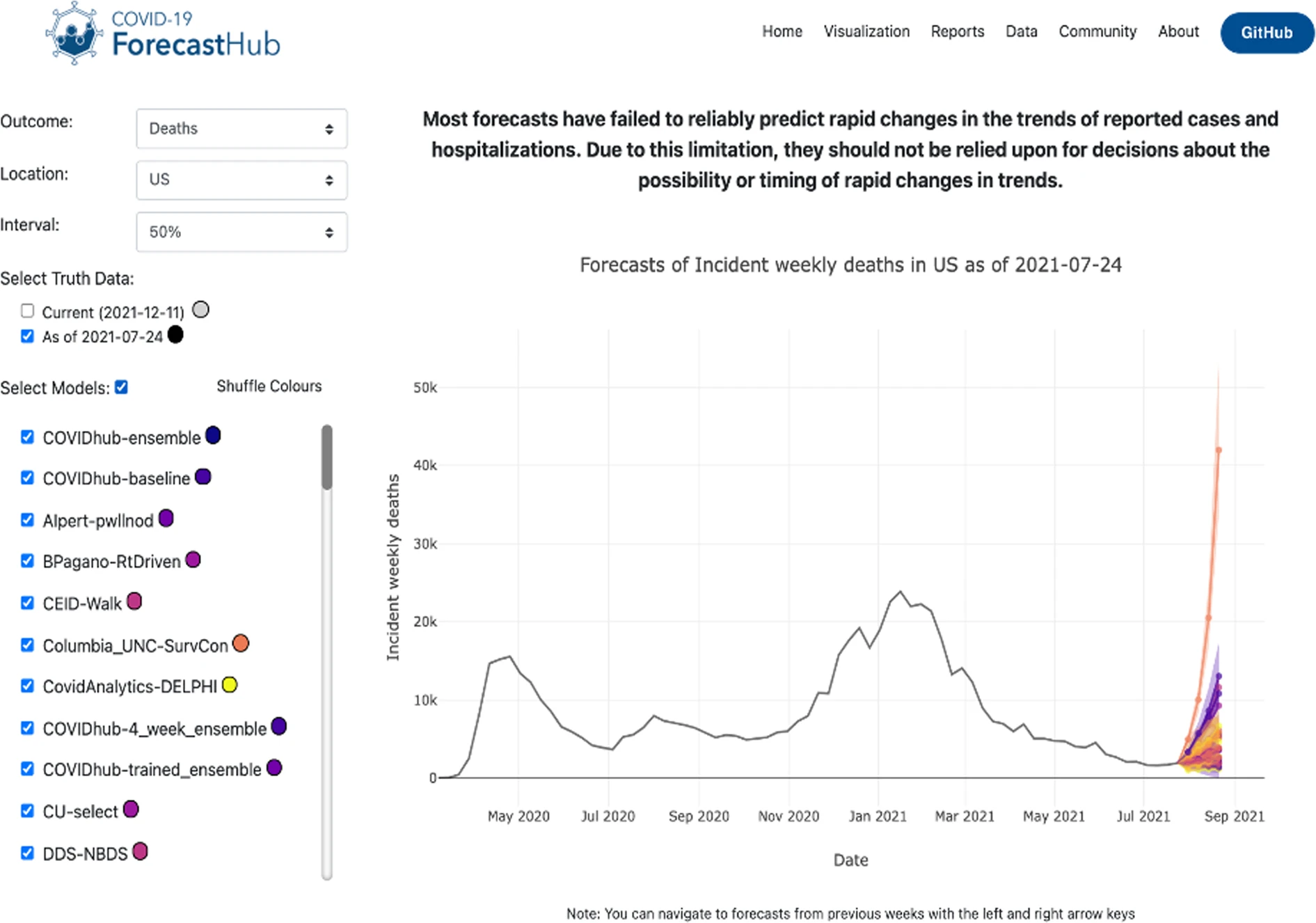
+
+[Cramer *et al.*, *Scientific Data*, 2022](https://doi.org/10.1038/s41597-022-01517-w)
+
+:::
+
+::::
+
+### {.smaller}
+
+{width="70%"}
+
+:::: {.columns}
+
+::: {.column}
+
+**Complex agent-based models**
+
+Conceptually probably the closest to metereological forecasting, if with much less real-time data.
+
+:::
+
+::: {.column}
+
+
+
+[Venkatramanan *et al.*, *Epidemics*, 2018](https://doi.org/10.1016/j.epidem.2017.02.010)
+
+:::
+
+::::
+
+### {.smaller}
+
+{width="70%"}
+
+:::: {.columns}
+
+::: {.column}
+
+**Compartmental models**
+
+Aim to capture relevant mechanisms but without going to the individual level.
+
+:::
+
+::: {.column}
+
+
+
+[Keeling *et al.*, *PLOS Comp Biol*, 2021](https://doi.org/10.1371/journal.pcbi.1008619)
+
+:::
+
+::::
+
+### {.smaller}
+
+{width="70%"}
+
+:::: {.columns}
+
+::: {.column}
+
+**Semi-mechanistic models**
+
+- Include some epidemiological mechanism (e.g. SIR or the renewal equation)
+- Add a nonmechanistic time component inspired by statistical models (e.g. random walk)
+- e.g., the model we have worked with so far
+
+:::
+
+::: {.column}
+
+
+
+[Dureau *et al.*, *Biostatistics*, 2013](https://doi.org/10.1093/biostatistics/kxs052)
+
+:::
+
+::::
+
+### {.smaller}
+
+{width="70%"}
+
+:::: {.columns}
+
+::: {.column}
+
+**Statistical models**
+
+- Models that don't include any epidemiological background e.g. ARIMA; also called *time-series models*
+- The random walk model when used on its own (without going via $R_t$) is called a [stochastic volatility model](https://mc-stan.org/docs/stan-users-guide/time-series.html#stochastic-volatility-models)
+
+:::
+
+::: {.column}
+
+
+
+:::
+
+::::
+
+### {.smaller}
+
+{width="70%"}
+
+:::: {.columns}
+
+::: {.column}
+
+**Other models**
+
+- Expert or crowd opinion
+- Machine learning
+- ...
+
+:::
+
+::: {.column}
+
+
+
+[Bosse *et al.*, *Wellcome Open Res*, 2024](https://doi.org/10.12688/wellcomeopenres.19380.2)
+
+:::
+
+::::
+
+## `r fontawesome::fa("laptop-code", "white")` Your Turn {.smaller background-color="#447099" transition="fade-in"}
+
+1. Review the performance of the random walk model
+2. Motivate a mechanism to order to address some of the issues
+3. Motivate a statistical approach aiming to address the same issues
+4. Compare the performance of these models for a single forecast
+5. Evaluate many forecast from these models and compare their performance
+
+#
+
+[Return to the session](../forecasting-concepts)
diff --git a/sessions/slides/from-line-list-to-decisions.qmd b/sessions/slides/from-line-list-to-decisions.qmd
new file mode 100644
index 0000000..5ff0ab3
--- /dev/null
+++ b/sessions/slides/from-line-list-to-decisions.qmd
@@ -0,0 +1,71 @@
+---
+title: "From an epidemiological line list to informing decisions in real-time"
+author: "Nowcasting and forecasting of infectious disease dynamics"
+engine: knitr
+format:
+ revealjs:
+ output: slides/from-line-list-to-decisions.html
+ footer: "From an epidemiological line list to informing decisions in real-time"
+ slide-level: 3
+---
+
+### "We were losing ourselves in details [...] all we needed to know is, are the number of cases rising, falling or levelling off?"
+
+Hans Rosling, Liberia, 2014
+
+. . .
+
+- what **is** the number of cases now?
+- is it rising/falling and by how much?
+- what does this mean for the near future?
+
+### Data usually looks like this
+
+
+
+### Aggregated data can look like this {.smaller}
+
+
+
+[UKHSA, 2022](https://www.gov.uk/government/publications/monkeypox-outbreak-technical-briefings/investigation-into-monkeypox-outbreak-in-england-technical-briefing-1)
+[Overton et al., *PLOS Comp Biol*, 2023](https://doi.org/10.1371/journal.pcbi.1011463)
+
+### Aim of this course:
+
+How can we use data typically collected in an outbreak to answer questions like
+
+- what **is** the number of cases now? (*nowcasting*)
+- is it rising/falling and by how much? (*$R_t$ estimation*)
+- what does this mean for the near future (*forecasting*)
+
+in real time.
+
+### Approach
+
+Throughout the course we will
+
+1. simulate typical infectious disease data in **R**
+(the *generative model*)
+2. apply the generative model to data in **stan** to
+ - learn about the system (conduct inference)
+ - make predictions (nowcasting/forecasting)
+
+### Timeline
+
+::: {.incremental}
+- delay distributions and how to estimate them (day 1)
+- $R_t$ estimation and the generation interval (day 1)
+- nowcasting (day 2)
+- forecasting and evaluation, ensemble methods (day 2)
+- applications (day 3)
+:::
+
+#
+
+To start the course go to:
+[https://nfidd.github.io/nfidd/](https://nfidd.github.io/nfidd/)
+and get started on the first sesion (*Probability distributions and parameter estimation*)
+
+#
+
+[Return to the session](../introduction-and-course-overview)
diff --git a/sessions/slides/introduction-to-biases-in-epidemiological-delays.qmd b/sessions/slides/introduction-to-biases-in-epidemiological-delays.qmd
new file mode 100644
index 0000000..2429bfb
--- /dev/null
+++ b/sessions/slides/introduction-to-biases-in-epidemiological-delays.qmd
@@ -0,0 +1,102 @@
+---
+title: "Introduction to biases in epidemiological delays"
+author: "Nowcasting and forecasting of infectious disease dynamics"
+engine: knitr
+format:
+ revealjs:
+ output: slides/introduction-to-biases-in-epidemiological-delays.html
+ footer: "Introduction to biases in epidemiological delays"
+---
+
+```{r seed}
+set.seed(123)
+```
+## Biases in epidemiological delays
+
+Why might our estimates of epidemiological delays be biased?
+
+. . .
+
+::: {.fragment .fade-out}
+- data reliability and representativeness
+:::
+- intrinsic issues with data collection and recording
+
+## Issue #1: Double censoring
+
+- reporting of events usually as a **date** (not date + precise time)
+- for short delays this can make quite a difference
+- accounting for it incorrectly can introduce more bias than doing nothing
+
+## Double censoring: example {.smaller}
+
+We are trying to estimate an incubation period. For person A we know exposure happened on day 1 and symptom onset on day 3.
+
+
+
+## Double censoring: example {.smaller}
+
+We are trying to estimate an incubation period. For person A we know exposure happened on day 1 and symptom onset on day 3.
+
+
+
+## Double censoring: example {.smaller}
+
+We are trying to estimate an incubation period. For person A we know exposure happened on day 1 and symptom onset on day 3.
+
+
+
+## Double censoring: example {.smaller}
+
+We are trying to estimate an incubation period. For person A we know exposure happened on day 1 and symptom onset on day 3.
+
+
+
+The *true* incubation period of A could be anywhere between 1 and 3 days (but not all equally likely).
+
+## Issue #2: right truncation
+
+- reporting of events can be triggered by the **secondary** event
+- in that case, longer delays might be missing because whilst the *primary events* have occurred the *secondary events* **have not occurred yet**
+
+## Example: right truncation {.smaller}
+
+We are trying to estimate an incubation period. Each arrow represents one person with an associated pair of events (infection and symptom onset).
+
+
+
+## Example: right truncation {.smaller}
+
+We are trying to estimate an incubation period. Each arrow represents one person with an associated pair of events (infection and symptom onset).
+
+
+
+## Example: right truncation {.smaller}
+
+We are trying to estimate an incubation period. Each arrow represents one person with an associated pair of events (infection and symptom onset).
+
+
+
+## Example: right truncation {.smaller}
+
+We are trying to estimate an incubation period. Each arrow represents one person with an associated pair of events (infection and symptom onset)
+
+
+
+On the day of analysis we have not observed some delays yet, and these tended to be **longer**. This is made worse during periods of **exponential growth**.
+
+## Censoring and right truncation {.smaller}
+
+- When analysing data from an outbreak in real time, we are likely to have double censoring **and** right truncation, making things worse
+- In the practical we will only look at the two separately to keep things simple
+
+## `r fontawesome::fa("laptop-code", "white")` Your Turn {background-color="#447099" transition="fade-in"}
+
+1. Simulate epidemiological delays with biases
+2. Estimate parameters of a delay distribution, correcting for biases
+
+
+#
+
+[Return to the session](../biases-in-delay-distributions)
+
diff --git a/sessions/slides/introduction-to-ensembles.qmd b/sessions/slides/introduction-to-ensembles.qmd
new file mode 100644
index 0000000..0709d6c
--- /dev/null
+++ b/sessions/slides/introduction-to-ensembles.qmd
@@ -0,0 +1,154 @@
+---
+title: "Multi-model ensembles"
+author: "Nowcasting and forecasting of infectious disease dynamics"
+format:
+ revealjs:
+ output: slides/forecasting-as-an-epidemiological-problem.html
+ footer: "Multi-model ensembles"
+---
+
+## Ensembles
+
+- Combine many different models' forecasts into a single prediction
+
+## Why ensemble?
+
+::: {.fragment .fade-in}
+Many uncertainties, many approaches: many models
+
+ - Layers of uncertainty
+
+ - Model parameters
+ - e.g. parameterising delay distributions
+
+ - Model structure
+ - e.g. more mechanistic or more statistical approaches
+ - Want to use all available information
+:::
+
+## Why ensemble?
+
+"Whole is greater than sum of parts"
+
+ - Average of multiple predictions is often more performant than any individual model
+
+ - History in weather & economic forecasting
+
+ - Seen this in infectious disease forecasting
+
+ - "Forecast challenges": Ebola, dengue, flu, COVID-19...
+
+## Ensemble methods
+
+::: {.fragment .fade-in}
+
+- Summarising across models to create single (probabilistic) prediction
+
+ - e.g. average at each models' probabilistic quantiles
+
+ - Mean
+
+ - Median - trims the outliers, so narrows the uncertainty
+:::
+
+## Ensemble methods
+
+- Equal or weighted combination
+
+ - Weight models by past forecast performance
+
+ - e.g. using forecast scores
+
+ - Rarely better than equal average
+
+## Collaborative modelling
+
+### "Forecast hubs"
+
+- Crowdsourcing forecasts
+
+ - Open source collaborative projects
+
+ - Anyone able to contribute a forecast
+
+- Forecasts ensembled into a single projection
+
+- Also enables consistent evaluation
+
+## Collaborative modelling
+
+### "Forecast hubs"
+
+- Outbreak modelling
+
+ - Since 2013 for US influenza
+
+ - Ebola, dengue, chikungunya, COVID-19, WNV
+
+##
+
+... European [Respicast](https://respicast.ecdc.europa.eu/)
+
+
+
+## Single model {.smaller}
+
+
+## ... Multiple models {.smaller}
+
+
+## ... ... Multi-model ensemble {.smaller}
+
+
+## Evaluation: European COVID-19 Hub
+
+- March 2021 - now
+
+- ~40 teams, ~50 models
+
+- Weekly ensemble & evaluation
+
+
+## Evaluation: European COVID-19 Hub {.smaller}
+
+
+
+
+## Evaluation: European COVID-19 Hub {.smaller}
+
+
+
+
+## Evaluating ensembles
+
+::: {.fragment .fade-in}
+
+ - Ensemble reduces variance
+
+ - (+) Stable
+
+ - (-) Can't explore extremes
+:::
+
+::: {.fragment .fade-in}
+
+ - Dependent on components
+
+ - (+) Ensemble typically more accurate than any individual component
+
+ - (-) Obscures mechanisms
+:::
+
+## Ensembles: reflections
+
+- Comparing uncertainties
+
+ - Which aspects of uncertainty do we want to keep (compare), or combine?
+
+
+
+- Collaborative modelling
+
+ - Opportunity for exchange & evaluation
+
+ - Consensus - at the cost of context?
diff --git a/sessions/slides/introduction-to-epidemiological-delays.qmd b/sessions/slides/introduction-to-epidemiological-delays.qmd
new file mode 100644
index 0000000..ecbf3e6
--- /dev/null
+++ b/sessions/slides/introduction-to-epidemiological-delays.qmd
@@ -0,0 +1,171 @@
+---
+title: "Introduction to epidemiological delays"
+author: "Nowcasting and forecasting of infectious disease dynamics"
+engine: knitr
+format:
+ revealjs:
+ output: slides/introduction-to-epidemiological-delay-distributions.html
+ footer: "Introduction to epidemiological delays"
+---
+
+```{r seed}
+set.seed(123)
+library("dplyr")
+library("tidyr")
+library("ggplot2")
+```
+# Epidemiological delay
+
+Time between two epidemiological events
+
+## Epidemiological events: disease progression
+- infection
+- symptom onset
+- becoming infectious
+- hospital admission
+- death
+
+## Epidemiological events: recovery
+- pathogen clearance
+- symptoms clearance
+- end of infectiousness
+- discharge from hospital
+
+## Epidemiological events: control
+
+- quarantine
+- isolation
+- treatment
+
+## Epidemiological events: reporting
+
+- specimen taken
+- report added to database
+
+## Some delays have names
+
+*infection* to *symptom onset*
+
+. . .
+
+**Incubation period**
+
+## Some delays have names
+
+*infection* to *becoming infectious*
+
+. . .
+
+**Latent period**
+
+## Some delays have names
+
+*becoming infectious* to *end of infectiousness*
+
+. . .
+
+**Infectious period**
+
+## Some delays have names
+
+hospital *admission* to *discharge*
+
+. . .
+
+**Length of stay**
+
+## Some delays have names
+
+*symptom onset* (person A) to *symptom onset* (person B, infected by A)
+
+. . .
+
+**Serial interval**
+
+## Some delays have names
+
+*infection* (person A) to *infection* (person B, infected by A)
+
+. . .
+
+**Generation interval**
+
+## Why do we want to know these?
+
+## Key parameters in mathematical models {.smaller}
+
+
+
+[Ferguson et al., 2020](https://doi.org/10.25561/77482)
+
+## Key parameters in mathematical models {.smaller}
+
+
+
+[Ward et al., 2020](https://doi.org/10.25561/77482)
+
+## Key elements of infectious disease epidemiology {.smaller}
+
+
+[Nishiura et al., 2007](https://doi.org/10.1186/1742-7622-4-2)
+
+## Why do we want to know these?
+
+- Key elements of infectious disease epidemiology
+- Intricate relationship with nowcasting/forecasting
+
+## Quantifying delays
+
+- Epidemiological delays are *variable*
+- We can capture their variability using probability distributions
+
+## Warning: Two levels of uncertainty {background-color="#000000" transition="fade-in" .smaller}
+
+- Probability distributions characterise variability in the delays *between individuals*
+- *Parameters* of the probability distribution can be uncertain
+
+:::: {.columns}
+
+::: {.column width="40%"}
+$$
+\alpha \sim \mathrm{Normal}(mean = 5, sd = 0.1) \\
+\beta \sim \mathrm{Normal}(mean = 1, sd = 0.1) \\
+$$
+
+Showing probability density functions of lognormal distributions with shape $\alpha$ and rate $\beta$.
+
+:::
+
+::: {.column width="60%"}
+```{r plot_multiples, fig.width=5, fig.height=3}
+n <- 20
+df <- tibble(
+ id = seq_len(n),
+ shape = rnorm(n, mean = 5, sd = 0.1),
+ rate = rlnorm(n, meanlog = 0, sdlog = 0.1)
+)
+
+## plot 99% of PMF
+max_x <- max(qgamma(0.99, shape = df$shape, rate = df$rate))
+
+## calculate density on grid of x values
+x <- seq(0.1, max_x, length.out = 100)
+df <- df |>
+ crossing(x = x) |> ## add grid to data frame
+ mutate(density = dgamma(x, shape, rate))
+
+ggplot(df, aes(x = x, y = density, group = id)) +
+ geom_line(alpha = 0.3)
+```
+:::
+
+::::
+
+## `r fontawesome::fa("laptop-code", "white")` Your Turn {background-color="#447099" transition="fade-in"}
+
+- Simulate epidemiological delays
+- Estimate parameters of a delay distribution
+
+#
+
+[Return to the session](../delay-distributions)
diff --git a/sessions/slides/introduction-to-joint-estimation-of-nowcasting-and-reporting-delays.qmd b/sessions/slides/introduction-to-joint-estimation-of-nowcasting-and-reporting-delays.qmd
new file mode 100644
index 0000000..48404da
--- /dev/null
+++ b/sessions/slides/introduction-to-joint-estimation-of-nowcasting-and-reporting-delays.qmd
@@ -0,0 +1,71 @@
+---
+title: "Introduction to joint estimation of nowcasting and reporting delays"
+author: "Nowcasting and forecasting of infectious disease dynamics"
+engine: knitr
+format:
+ revealjs:
+ output: slides/introduction-to-joint-estimation-of-nowcasting-and-reporting-delays.html
+ footer: "Introduction to joint estimation of nowcasting and reporting delays"
+ slide-level: 3
+ chalkboard: true
+---
+
+### Motivating example
+
+Often we see data like this
+
+
+
+
+(all figures in this deck are courtesy of Johannes Bracher)
+
+### The aim of nowcasting
+
+Predict what an epidemiological time series will look like *after all delayed reports* are in.
+
+
+
+
+### The limitations of simple nowcasting methods
+
+ - Hard to propagate uncertainty
+ - Doesn't account for observation error in the primary events
+ - Not a generative model of the data reporting process (hard to add complex reporting patterns)
+ - If we get the delay wrong, we can get the nowcast wrong
+
+### Jointly estimating reporting delays and nowcasting
+
+One potential solution is to jointly estimate the reporting delays and the nowcast. We can do this if we have multiple snapshots of the data.
+
+
+
+### The reporting triangle
+
+The reporting triangle is the name given to the data structure that arises when we have multiple snapshots of the data.
+
+
+
+### The reporting triangle
+
+Here the rows represent the time of the primary event, and the columns represent the time of the report.
+Some events are missing because they are yet to happen.
+
+
+
+### Completing the reporting triangle
+
+The aim of nowcasting is to complete the reporting triangle. This means filling in the missing entries in the lower triangle and then summing the rows to get the nowcast.
+
+
+
+## `r fontawesome::fa("laptop-code", "white")` Your Turn {background-color="#447099" transition="fade-in"}
+
+1. Simulate the reporting triangle
+2. Perform a joint estimation of the delay and nowcast
+3. Understand the limitations of the data generating process
+4. Perform a joint estimation of the delay, nowcast, and reproduction number
+
+###
+
+[Return to the session](../joint-nowcasting)
+
diff --git a/sessions/slides/introduction-to-nowcasting.qmd b/sessions/slides/introduction-to-nowcasting.qmd
new file mode 100644
index 0000000..b5a9ba8
--- /dev/null
+++ b/sessions/slides/introduction-to-nowcasting.qmd
@@ -0,0 +1,80 @@
+---
+title: "Introduction to nowcasting"
+author: "Nowcasting and forecasting of infectious disease dynamics"
+engine: knitr
+format:
+ revealjs:
+ output: slides/introduction-to-nowcasting.html
+ footer: "Introduction to nowcasting"
+ chalkboard: true
+ slide-level: 3
+---
+
+### Motivating example {.smaller}
+
+Often we see data like this
+
+
+
+Data after the dashed line are marked as uncertain. What, if anything, do they tell us about current trends?
+
+### second example {.smaller}
+
+... or like this
+
+
+
+### second example {.smaller}
+
+... or like this
+
+
+
+[RKI COVID-19 Situation Report, 8 March 2020](https://www.rki.de/DE/Content/InfAZ/N/Neuartiges_Coronavirus/Situationsberichte/2020-03-08-en.pdf?__blob=publicationFile)
+
+###
+
+These patterns arise because:
+
+- Epidemiological time series are aggregated by the *epidemiologically meaningful date*
+ (e.g. symptom onset, hospital admission, death)
+- There is a *delay* between this date, and the events showing up in the data
+- This leads to an "articifial" *dip* in the most recent data
+
+### The aim of nowcasting
+
+Predict what an epidemiological time series will look like *after all delayed reports* are in.
+
+
+
+(slide courtesy of Johannes Bracher)
+
+### Nowcasting as right truncation
+
+Remember from [biases in delay estimation](../biases-in-delay-distributions):
+
+**Right truncation**
+
+- reporting of events can be triggered by the **secondary** event
+- in that case, longer delays might be missing because whilst the *primary events* have occurred the *secondary events* **have not occurred yet**
+
+Nowcasting is exactly this!.
+
+### A simple approach to nowcasting
+
+1. Estimate the delay distribution from other data
+2. Specify a model for the epidemic dynamics
+3. Use the estimated delay distribution to model the expected right truncation in the data
+4. Fit the model to the truncated data
+5. Use the untruncated estimates from the model as the nowcast
+
+## `r fontawesome::fa("laptop-code", "white")` Your Turn {background-color="#447099" transition="fade-in"}
+
+1. Perform nowcast with a known reporting delay distribution
+2. Perform a nowcast using a more realistic data generating process
+3. Explore the impact of getting the delay distribution wrong
+
+#
+
+[Return to the session](../nowcasting)
+
diff --git a/sessions/slides/introduction-to-reproduction-number.qmd b/sessions/slides/introduction-to-reproduction-number.qmd
new file mode 100644
index 0000000..6fbf2c5
--- /dev/null
+++ b/sessions/slides/introduction-to-reproduction-number.qmd
@@ -0,0 +1,78 @@
+---
+title: "Introduction to the time-varying reproduction number"
+author: "Nowcasting and forecasting of infectious disease dynamics"
+engine: knitr
+format:
+ revealjs:
+ output: slides/introduction-to-reproduction-number.html
+ footer: "Introduction to the time-varying reproduction number"
+execute:
+ echo: true
+---
+
+```{r seed, echo = FALSE}
+set.seed(123)
+```
+
+## Convolution session {.smaller}
+
+```{stan, file = system.file("stan", "estimate-infections.stan", package = "nfidd"), eval = FALSE, output.var = "mod"}
+#| code-line-numbers: "|23"
+```
+
+. . .
+
+Prior for infections at time $t$ is independent from infections at all other time points.
+Is this reasonable?
+
+## Infections depend on previous infections
+
+Remember the definition of the generation time distribution $g(t)$:
+
+*infection* (person A) to *infection* (person B, infected by A)
+
+Through this, infections depend on previous infections:
+
+$$
+I_t = \mathrm{scaling} \times \sum_{t' < t} I_t' g(t - t')
+$$
+
+What is this scaling?
+
+## Scaling of infections with previous infections
+
+Let's assume we have $I_0$ infections at time 0, and the scaling doesn't change in time.
+
+How many people will they go on to infect?
+
+. . .
+
+$$
+I = \mathrm{scaling} \times \sum_{t=0}^\infty I_0 g(t) = \mathrm{scaling} * I_0
+$$
+
+The scaling can be interpreted as the (time-varying) reproduction number $R_t$.
+
+## The renewal equation
+
+If $R_t$ can change over time, it can still be interpreted as the ("instantaneous") reproduction number:
+
+$$
+I_t = R_t \times \sum_{t' < t} I_t' g(t - t')
+$$
+
+We can estimate $R_t$ from a time series of infections using the renewal equation.
+
+## The renewal equation as convolution
+
+
+
+## `r fontawesome::fa("laptop-code", "white")` Your Turn {background-color="#447099" transition="fade-in"}
+
+- Simulate infections using the renewal equation
+- Estimate reproduction numbers using a time series of infections
+- Combine with delay distributions to jointly infer infections and R from a time series of outcomes
+
+#
+
+[Return to the session](../R-estimation-and-the-renewal-equation)
diff --git a/sessions/slides/introduction-to-stan.qmd b/sessions/slides/introduction-to-stan.qmd
new file mode 100644
index 0000000..2e8de81
--- /dev/null
+++ b/sessions/slides/introduction-to-stan.qmd
@@ -0,0 +1,104 @@
+---
+title: "Introduction to stan"
+author: "Nowcasting and forecasting of infectious disease dynamics"
+format:
+ revealjs:
+ output: slides/introduction-to-stan.html
+ footer: "Introduction to stan"
+---
+
+```r
+set.seed(12345)
+```
+
+## What is stan and why do we use it?
+
+- a *Probabilistic Programming Language* for Bayesian inference
+ (i.e., a way to write down models)
+
+- models are written in a text file (often ending `.stan`) and then loaded into an R/python/etc interface
+
+- once a model is written down, stan can be used to **generate samples** from the **posterior distribution** (using a variety of methods)
+
+## How to write a model in stan {.smaller}
+
+:::: {.columns}
+
+::: {.column width="40%"}
+In a stan model file we specify:
+
+- Data (types and names)
+
+- Parameters (types and names)
+
+- Model (prior and likelihood)
+:::
+
+::: {.column width="60%"}
+
+
+```{stan empty_model, output.var = "empty", eval = FALSE, echo = TRUE}
+data {
+
+}
+
+parameters {
+
+}
+
+model {
+
+}
+```
+:::
+::::
+
+## Example: fairness of a coin {.smaller}
+
+:::: {.columns}
+
+::: {.column width="40%"}
+
+Data:
+
+- $N$ coin flips
+
+- $x$ times heads
+
+Parameters
+
+- $\theta$, probability of getting heads; uniform prior in $[0, 1]$
+
+:::
+
+::: {.column width="60%"}
+
+
+```{stan coin_model, output.var = "coin", eval = FALSE, echo = TRUE, file = nfidd::nfidd_cmdstan_model("coin")
+```
+:::
+::::
+
+## Using stan from R {.smaller}
+
+There are two packages for using stan from R. We will use the `cmdstanr` package:
+
+```{r load_coin_model, echo = TRUE}
+library("cmdstanr")
+mod <- nfidd::nfidd_cmdstan_model("coin")
+mod
+```
+
+## Sampling from the posterior {.xmaller}
+
+```{r sample_from_coin_model, echo = TRUE}
+data <- list(
+ N = 10, ## 10 coin flips
+ x = 6 ## 6 times heads
+)
+mod$sample(data = data)
+```
+
+#
+
+[Return to the session](../R-Stan-and-statistical-concepts)
diff --git a/sessions/slides/introduction-to-statistical-concepts.qmd b/sessions/slides/introduction-to-statistical-concepts.qmd
new file mode 100644
index 0000000..9a42970
--- /dev/null
+++ b/sessions/slides/introduction-to-statistical-concepts.qmd
@@ -0,0 +1,268 @@
+---
+title: "Introduction to statistical concepts used in the course"
+author: "Nowcasting and forecasting of infectious disease dynamics"
+format:
+ revealjs:
+ output: slides/introduction-to-statistical-concepts.html
+ footer: "Introduction to statistical concepts used in the course"
+ slide-level: 3
+---
+
+```{r echo = FALSE, message = FALSE}
+library("ggplot2")
+set.seed(123)
+```
+
+### Why statistical concepts?
+
+- We'll need to estimate things (delays, reproduction numbers, case numbers now and in the future)
+
+- We'll want to correctly specify uncertainty
+
+- We'll want to incorporate our domain expertise
+
+- We'll do this using *Bayesian inference*
+
+## Bayesian inference in 15 minutes
+
+
+
+### Interlude: probabilities
+
+::: {.callout-tip}
+#### [Laplace, 1812](https://archive.org/details/thorieanalytiqu01laplgoog)
+
+*Probability theory is nothing but common sense reduced to calculation.*
+:::
+
+### Interlude: probabilities (1/3) {.smaller}
+
+- If $A$ is a random variable, we write
+ $$ p(A = a)$$
+ for the *probability* that $A$ takes value $a$.
+- We often write
+ $$ p(A = a) = p(a)$$
+- Example: The probability that it rains tomorrow
+ $$ p(\mathrm{tomorrow} = \mathrm{rain}) = p(\mathrm{rain})$$
+- Normalisation
+ $$ \sum_{a} p(a) = 1 $$
+
+### Interlude: probabilities (2/3) {.smaller}
+
+- If $A$ and $B$ are random variables, we write
+ $$ p(A = a, B = b) = p(a, b)$$ for the *joint probability* that $A$ takes value $a$ and $B$ takes value $b$
+- Example: The probability that it rains today and tomorrow
+ $$ p(\mathrm{tomorrow} = \mathrm{rain}, \mathrm{today} = \mathrm{rain}) = p(\mathrm{rain}, \mathrm{rain})$$
+- We can obtain a *marginal probability* from joint probabilities by summing
+ $$ p(a) = \sum_{b} p(a, b)$$
+
+### Interlude: probabilities (3/3) {.smaller}
+
+- The *conditional probability* of getting outcome $a$ from random variable $A$, given that the outcome of random variable $B$ was $b$, is written as
+ $$ p(A = a | B = b) = p(a| b) $$
+- Example: the probability that it rains tomorrow given that it is sunny today
+ $$ p(\mathrm{tomorrow} = \mathrm{rain} | \mathrm{today} = \mathrm{rain}) = p(\mathrm{rain} | \mathrm{rain})$$
+- Conditional probabilities are related to joint probabilities as
+ $$ p(a | b) = \frac{p(a, b)}{p(b)}$$
+- We can combine conditional probabilities in the *chain rule*
+ $$ p(a, b, c) = p(a | b, c) p(b | c) p (c) $$
+
+### Probability distributions (discrete) {.smaller}
+
+```{r create_poisson_plot}
+kicks <- seq(0, 6)
+prob <- dpois(kicks, lambda = 0.61)
+df <- data.frame(kicks = kicks, prob = prob)
+pp <- ggplot(df, aes(x = kicks, y = prob)) +
+ theme_bw(20) +
+ geom_point(size = 3) +
+ ylab("Probability") +
+ xlab("Number of kicks")
+```
+
+- E.g., how many people die of horse kicks if there are 0.61 kicks per year
+- Described by the *Poisson* distribution
+
+:::: {.columns}
+
+::: {.column width="50%"}
+```{r plot_poisson1}
+pp
+```
+:::
+
+::: {.column width="50%"}
+:::
+
+#### Two directions
+1. Calculate the probability
+2. Randomly sample
+
+
+::::
+
+### Calculate discrete probability {.smaller}
+
+- E.g., how many people die of horse kicks if there are 0.61 kicks per year
+- Described by the *Poisson* distribution
+
+:::: {.columns}
+
+::: {.column width="50%"}
+```{r plot_poisson2}
+pp
+```
+:::
+
+::: {.column width="50%"}
+What is the probability of 2 deaths in a year?
+```{r dpois, echo = TRUE}
+ dpois(x = 2, lambda = 0.61)
+```
+:::
+
+::::
+
+#### Two directions
+
+1. **Calculate the probability**
+2. Randomly sample
+
+### Generate a random (Poisson) sample {.smaller}
+- E.g., how many people die of horse kicks if there are 0.61 kicks per year
+- Described by the *Poisson* distribution
+
+:::: {.columns}
+
+::: {.column width="50%"}
+```{r plot_poisson3}
+pp
+```
+:::
+
+::: {.column width="50%"}
+Generate one random sample from the probability distribution
+```{r rpois, echo = TRUE}
+ rpois(n = 1, lambda = 0.61)
+```
+:::
+
+::::
+
+#### Two directions
+
+1. Calculate the probability
+2. **Randomly sample**
+
+### Probability distributions (continuous) {.smaller}
+- Extension of probabilities to *continuous* variables
+- E.g., the temperature in Stockholm tomorrow
+
+Normalisation:
+$$ \int p(a) da = 1 $$
+
+Marginal probabilities:
+$$ p(a) = \int_{} p(a, b) db$$
+
+#### Two directions
+1. Calculate the probability (density)
+2. Randomly sample
+
+### Calculate probability density {.smaller}
+- Extension of probabilities to *continuous* variables
+- E.g., the temperature in Stockholm tomorrow
+
+```{r create_normal_plot}
+temp_mean <- 23
+temp_sd <- 2
+temp <- seq(15, 31, by = 0.01)
+prob <- dnorm(temp,mean = temp_mean, sd = temp_sd)
+df <- data.frame(temp = temp, prob = prob)
+pn <- ggplot(df, aes(x = temp, y = prob)) +
+ theme_bw(20) +
+ geom_line(lwd = 2) +
+ xlab("Temperature") +
+ ylab("Probability density")
+```
+
+:::: {.columns}
+
+::: {.column width="50%"}
+```{r plot_normal1}
+pn
+```
+:::
+
+::: {.column width="50%"}
+What is the probability density of $30^\circ C$ tomorrow, if the mean temperature on the day is $23^\circ C$ (standard deviation $2^\circ C$) ? A naïve model could be:
+```{r dnorm, echo = TRUE}
+ dnorm(x = 30,
+ mean = 23,
+ sd = 2)
+```
+:::
+
+::::
+
+#### Two directions
+
+1. **Calculate the probability**
+2. Randomly sample
+
+### Generate a random (normal) sample {.smaller}
+
+:::: {.columns}
+
+::: {.column width="50%"}
+```{r plot_normal2}
+pn
+```
+:::
+
+::: {.column width="50%"}
+Generate one random sample from the normal probability distribution with mean 23 and standard deviation 2:
+```{r rnorm, echo = TRUE}
+ rnorm(n = 1,
+ mean = 23,
+ sd = 2)
+```
+:::
+
+::::
+
+#### Two directions
+
+1. Calculate the probability
+2. **Randomly sample**
+
+## Bayesian inference in 15 minutes {.smaller}
+
+
+
+Idea of Bayesian inference: treat $\theta$ as **random variables** (with a probability distribution) and **condition on data**: posterior probability $p(\theta | \mathrm{data})$ as target of inference.
+
+### Bayes' rule {.smaller}
+
+- We treat the parameters of the a $\theta$ as random with *prior probabilities* given by a distribution $p(\theta)$. Confronting the model with data we obtain *posterior probabilities* $p(\theta | \mathrm{data})$, our target of inference. Applying the rule of conditional probabilities, we can write this as
+
+$$ p(\theta | \textrm{data}) = \frac{p(\textrm{data} | \theta) p(\theta)}{p(\textrm{data})}$$
+
+- $p(\textrm{data} | \theta)$ is the /likelihood/
+- $p(\textrm{data})$ is a /normalisation constant/
+
+- In words,
+ $$\textrm{(posterior)} \propto \textrm{(normalised likelihood)} \times \textrm{(prior)}$$
+
+### Bayesian inference
+
+
+
+### MCMC
+
+- Markov-chain Monte Carlo (MCMC) is a method to generate *samples* from the *posterior distribution*, the **target** of inference
+
+- [stan](https://mc-stan.org/) is a probabilistic programming language that helps you to write down probabilistic models and to fit them using MCMC samplers and other methods.
+
+#
+
+[Return to the session](../R-Stan-and-statistical-concepts)
diff --git a/sessions/slides/introduction-to-the-course-and-the-instructors.qmd b/sessions/slides/introduction-to-the-course-and-the-instructors.qmd
new file mode 100644
index 0000000..1a6714b
--- /dev/null
+++ b/sessions/slides/introduction-to-the-course-and-the-instructors.qmd
@@ -0,0 +1,59 @@
+---
+title: "Introduction to the course and the instructors"
+author: "Nowcasting and forecasting of infectious disease dynamics"
+format:
+ revealjs:
+ output: slides/introduction-to-the-course-and-the-instructors.html
+ footer: "Introduction to the course and the instructors"
+---
+
+```{r instructors}
+instructors <- c("sbfnk", "seabbs", "kathsherratt")
+```
+
+```{r get_contributors}
+library("dplyr")
+library("gh")
+library("jsonlite")
+whoarewe <- lapply(sort(instructors), \(instructor) {
+ gh(paste0("GET /users/{username}"), username = instructor)[
+ c("login", "avatar_url", "html_url", "name")
+ ]
+})
+```
+
+# Why this course?
+
+::: {.incremental}
+- common question in outbreak response
+- easy to make mistakes
+- no comprehensive training resource
+:::
+
+# Who are we?
+
+```{r whoarewe, results='asis'}
+cat(":::: {.columns}\n")
+for (instructor in seq_along(whoarewe)) {
+ cat(
+ "::: {.column width=\"", floor(100 / length(whoarewe)), "%\"}\n",
+ "\n",
+ "[", whoarewe[[instructor]]$name, "](",
+ whoarewe[[instructor]]$html_url, ")\n",
+ ":::\n", sep = ""
+ )
+}
+cat("::::\n")
+```
+
+# Who are you?
+
+Introduce yourself with:
+
+- name
+- where you travelled from
+- why you're here
+
+#
+
+[Return to the session](../introduction-and-course-overview)
diff --git a/sessions/slides/tools.qmd b/sessions/slides/tools.qmd
new file mode 100644
index 0000000..056f3ff
--- /dev/null
+++ b/sessions/slides/tools.qmd
@@ -0,0 +1,50 @@
+---
+title: "Examples of tools"
+author: "Nowcasting and forecasting of infectious disease dynamics"
+engine: knitr
+format:
+ revealjs:
+ output: slides/tools.html
+ footer: "Examples of tools"
+ slide-level: 3
+---
+
+### Timeline so far
+
+- delay distributions and how to estimate them (day 1)
+- $R_t$ estimation and the generation interval (day 1)
+- nowcasting (day 2)
+- forecasting and evaluation, ensemble methods (day 2)
+- applications (day 3)
+
+### Available tools or custom implementation?
+
+A few open-source tools implement some or all of the methods we have encountered in the course.
+
+### Available tools or custom implementation?
+
+- Loses flexibility of custom approaches
+
+ - different epidemiological problems, different data - different methods?
+
+### Available tools or custom implementation?
+
+- Value of using - and contributing
+
+ - avoids duplication
+
+ - improves the chances of finding errors
+
+ - helps discussing and ultimately enforcing best practice
+
+## `r fontawesome::fa("laptop-code", "white")` Your Turn {background-color="#447099" transition="fade-in"}
+
+How does each tool relate to key concepts in the course?
+
+ - EpiEstim
+ - EpiNow2
+ - Epinowcast
+
+#
+
+[Return to the session](../examples-of-available-tools)
diff --git a/sessions/using-delay-distributions-to-model-the-data-generating-process-of-an-epidemic.qmd b/sessions/using-delay-distributions-to-model-the-data-generating-process-of-an-epidemic.qmd
new file mode 100644
index 0000000..77a59c4
--- /dev/null
+++ b/sessions/using-delay-distributions-to-model-the-data-generating-process-of-an-epidemic.qmd
@@ -0,0 +1,318 @@
+---
+title: "Using delay distributions to model the data generating process"
+order: 4
+---
+
+# Introduction
+
+We've now developed a good idea of how epidemiological time delays affect our understanding of an evolving outbreak. So far, we've been working with individual line-list data. However, we usually want to model how an outbreak evolves through a whole population. The aim of this session is to introduce how delay distributions can be used to model population-level data generating process during an epidemic.
+
+This means working with aggregated count data. This creates some new issues in correctly accounting for uncertainty. We handle population-level data using *convolutions* as a way of combining count data with a distribution of individual probabilities. We will have to adjust our continuous probability distributions to work with this using *discretisation*. We'll then need to re-introduce additional uncertainty to account for the observation process at a population level.
+
+## Slides
+
+- [Delay distributions at the population level](slides/convolutions)
+
+## Objectives
+
+In this session, we'll focus on the delay from infection to symptom onset at a population level.
+First, we will introduce the techniques of convolution and discretisation. We'll apply these to an aggregated time series of infections in order to simulate observed symptom onsets.
+Then, we'll use those simulated symptom onsets to try and reconstruct a time series of infections.
+
+::: {.callout-note collapse="true"}
+
+# Setup
+
+## Source file
+
+The source file of this session is located at `sessions/using-delay-distributions-to-model-the-data-generating-process-of-an-epidemic.qmd`.
+
+## Libraries used
+
+In this session we will use the `nfidd` package to load a data set of infection times and access stan models and helper functions, the `dplyr` and `tidyr` packages for data wrangling, the `ggplot2` library for plotting and the `tidybayes` package for extracting results of the inference.
+
+```{r libraries, message = FALSE}
+library("nfidd")
+library("dplyr")
+library("tidyr")
+library("ggplot2")
+library("tidybayes")
+```
+
+::: callout-tip
+The best way to interact with the material is via the [Visual Editor](https://docs.posit.co/ide/user/ide/guide/documents/visual-editor.html) of RStudio.
+:::
+
+## Initialisation
+
+We set a random seed for reproducibility.
+Setting this ensures that you should get exactly the same results on your computer as we do.
+We also set an option that makes `cmdstanr` show line numbers when printing model code.
+This is not strictly necessary but will help us talk about the models.
+
+```{r}
+set.seed(123)
+options(cmdstanr_print_line_numbers = TRUE)
+```
+
+:::
+
+# Simulating observations from a time series of infections
+
+As before we first simulate the process that generates the data we typically observe.
+In this session, we'll focus on the process from infection to symptom onset.
+Then we'll use the same model to conduct inference.
+
+## Delay distributions and convolutions
+
+In the last session we simulated *individual outcomes* from a delay distribution, and then re-estimated the corresponding parameters.
+However, sometimes we do not have data on these individual-level outcomes, either because they are not recorded or because they cannot be shared, for example due to privacy concerns.
+At the population level, individual-level delays translate into *convolutions*.
+
+If we have a time series of infections $I_t$ ($t=1, 2, 3, \ldots, t_\mathrm{max}$), where $t$ denotes the day on which the infections occur, and observable outcomes occur with a delay given by a delay distribution $p_i$ ($i=0, 1, 2, \dots, p_\mathrm{max}$), where $i$ is the number of days after infection that the observation happens, then the number of observable outcomes $C_t$ on day $t$ is given by
+
+$$
+C_t = \sum_{i=0}^{i=p_\mathrm{max}} I_{t-i} p_i
+$$
+
+In other words, the number of observable outcomes on day $t$ is given by the sum of infections on all previous days multiplied by the probability that those infections are observed on day $t$.
+For example, the observable outcomes $C_t$ could be the number of symptom onsets on day $t$ and $p_i$ is the incubation period.
+
+We can use the same data as in the [session on biases in delay distributions](biases-in-delay-distributions#create-data-set), but this time we first aggregate this into a daily time series of infections.
+You can do this using:
+
+```{r aggregate}
+inf_ts <- make_daily_infections(infection_times)
+```
+
+::: callout-note
+As before you can look at the code of the function by typing `make_daily_infections`.
+The second part of this function is used to add days without infections with a zero count.
+This will make our calculations easier later (as otherwise we would have to try and detect these in any models that used this data which could be complicated).
+:::
+
+And look at the first few rows of the daily aggregated data:
+
+```{r display-aggregate}
+head(inf_ts)
+```
+
+Now we can convolve the time series with a delay distribution to get a time series of outcomes as suggested above.
+
+#### Discretising a delay distribution
+
+In our first session, we decided to assume the delay from infection to symptom onset had a gamma distribution.
+However, if we want to use the gamma distribution with shape 5 and rate 1 as before, we face a familiar issue.
+The gamma distribution is a *continuous* distribution, but now our delay data are in days which are *discrete* entities.
+We will assume that both events that make up the delay (here infection and symptom onset) are observed as daily counts (e.g. as number of infections/symptom onsets by calendar date).
+Therefore, both observations are **censored** (as events are rounded to the nearest date).
+This means that our distribution is **double interval censored** which we encountered in the [the biases in delay distribution session](sessions/biases-in-delay-distributions), so we need to use the same ideas introduced in that session.
+
+::: {.callout-note collapse=true}
+#### Mathematical Definition (optional): Discretising a delay distribution subject to double interval censoring
+
+The cumulative distribution function (CDF) ($F(t)$) of a distribution that has a daily censored primary event can be expressed as,
+
+$$
+F^*(t) = \int_0^1 F(t - u) du
+$$
+
+In effect, this is saying that the daily censored CDF is the average of the continuous distributions CDF over all possible event times (here between 0 and 1).
+
+The probability mass function (PMF) of this distribution when observed as a daily process (i.e. the secondary event is also daily censored) is then
+
+$$
+f_t \propto F^*(t + 1) - F^*(t - 1)
+$$
+
+The important point is that the ultimately observed PMF is a combination of a primary event daily censoring process and a secondary event daily censoring process.
+:::
+
+We can think about this via simulation.
+We do so by generating many replicates of the corresponding random delay, taking into account that we have already rounded down our infection times to infection days.
+This means that discretising a delay in this context is **double censoring** as we discussed in the [the biases in delay distribution session](sessions/biases-in-delay-distributions).
+In the absence of any other information or model, we assume for our simulation that infection occurred at some random time during the day, with each time equally likely.
+We can then apply the incubation period using a continuous probability distribution, before once again rounding down to get the day of symptom onset (mimicking daily reporting).
+We repeat this many times to get the probability mass function that allows us to go from infection days to symptom onset days.
+
+You can view the function we have written do this using:
+
+```{r discretise_2_day_window}
+censored_delay_pmf
+```
+
+::: callout-note
+## Take 5 minutes
+
+Try to understand the `censored_delay_pmf()` function above.
+Try it with a few different probability distributions and parameters, e.g. for the parameters given above and a maximum delay of 2 weeks (14 days) it would be:
+
+```{r discretised_gamma}
+gamma_pmf <- censored_delay_pmf(rgamma, max = 14, shape = 5, rate = 1)
+gamma_pmf
+
+plot(gamma_pmf)
+```
+:::
+
+#### Applying a convolution
+
+Next we apply a convolution with the discretised incubation period distribution to the time series of infections, to generate a time series of symptom onsets.
+The function we have written to do this is called `convolve_with_delay`
+
+```{r convolution}
+convolve_with_delay
+```
+
+::: callout-tip
+## Take 5 minutes
+
+Try to understand the `convolve_with_delay()` function above.
+Try it with a few different time series and delay distributions.
+How would you create the time series of symptom onsets from infections, using the discretised gamma distribution created above (saved in `gamma_pmf`)?
+:::
+
+::: {.callout-note collapse="true"}
+## Solution
+
+```{r applied_convolution}
+onsets <- convolve_with_delay(inf_ts$infections, gamma_pmf)
+```
+:::
+
+We can plot these symptom onsets:
+
+```{r convolution_plot}
+combined <- inf_ts |>
+ rename(time = infection_day) |>
+ mutate(onsets = onsets)
+ggplot(combined, aes(x = time, y = onsets)) +
+ geom_bar(stat = "identity")
+```
+
+Do they look similar to the plot of symptom onsets in the [session on delay distributions](delay-distributions#simulating-delayed-epidemiological-data)?
+
+## Observation uncertainty
+
+Usually not all data are perfectly observed.
+Also, the convolution we applied is a *deterministic* operation that brushes over the fact that individual delays are random.
+We should therefore find another way to model the variation these processes introduce.
+
+Given that we are now dealing with count data a natural choice is the Poisson distribution.
+We can use this to generate uncertainty around our convolved data.
+
+```{r uncertain}
+combined <- combined |>
+ mutate(observed = rpois(n(), onsets))
+```
+
+::: callout-tip
+## Take 5 minutes
+
+Does a plot of these observations look more like the plots from the [session on delay distributions](delay-distributions#simulating-delayed-epidemiological-data) than the convolution plotted above?
+:::
+
+::: {.callout-note collapse="true"}
+## Solution
+
+```{r plot-with-poisson}
+ggplot(combined, aes(x = time, y = observed)) +
+ geom_bar(stat = "identity")
+```
+:::
+
+# Estimating a time series of infections
+
+As in previous sessions, we don't usually have data on infections. We now create a model that uses symptom onsets to estimate the number of infections over time. We'll base the model on an uninformed prior and work forward from what we know about the observation process.
+
+```{r stan_estimate_infections}
+mod <- nfidd_cmdstan_model("estimate-infections")
+mod
+```
+
+::: callout-tip
+## Take 10 minutes
+
+Familiarise yourself with the model above.
+Unlike before there is now a `functions` block at the beginning of the model (lines 1-3), where we load a function called `convolve_with_delay()` (line 2) from a file of the same name which can be found in the subdirectory `functions` of the `stan` directory or [viewed on the github repo](https://github.com/nfidd/nfidd/blob/main/stan/functions/convolve_with_delay.stan).
+The functions correspond exactly to our earlier **R** function of the same name.
+Later, this functions is called in the `model` block, to generate the time series of symptom onsets (line 18).
+
+What is the prior assumption on the daily number of infections?
+Which line defines the likelihood, and how does it relate to the section about observation uncertainty above?
+:::
+
+::: {.callout-note collapse="true"}
+## Solution
+
+The model assumes that infections every day are independent from infections on any other day (line 23) and determined only by the number of symptom onsets that they result in (line 18).
+Line 24 defines the likelihood, and it does so using the Poisson observation uncertainty we used above.
+:::
+
+We can now use this model to conduct inference, i.e. to try to reconstruct the time series of infections from the time series of onsets that we generated earlier.
+
+```{r inf_fit, results = 'hide', message = FALSE}
+data <- list(
+ n = nrow(combined),
+ obs = combined$observed,
+ ip_max = length(gamma_pmf) - 1,
+ ip_pmf = gamma_pmf
+)
+inf_fit <- mod$sample(data = data, parallel_chains = 4)
+```
+
+::: callout-caution
+Note that this code might take a few minutes to run.
+We have added the `parallel_chains` option to make use of any paralle hardware you may ave.
+If you don't have 4 computing cores and/or the code runs very slowly, you could try only running 2 chains (`chains = 2`) and/or fewer samples (`iter_warmup = 500, iter_sampling = 500`).
+:::
+
+::: callout-tip
+In this model, we have estimated many more parameters than in the previous models: instead of e.g. 2 parameters of a probability distribution, we now have a total of `r nrow(onsets)` time points for which we estimate the number of infections.
+This is because we don't have a model for the *process* that generates infections.
+How would this be different if we e.g. used an SIR model here?
+:::
+
+We can see the first few estimates of the number of infections using:
+
+```{r inf_summary}
+inf_fit
+```
+
+Again, we can do a posterior predictive check by plotting the modelled estimates of the time series of infections (with uncertainty) against our original data.
+Does it look like a good fit?
+
+```{r inf_ppc}
+# Extract posterior draws
+inf_posterior <- inf_fit |>
+ gather_draws(infections[infection_day]) |>
+ ungroup() |>
+ mutate(infection_day = infection_day - 1) |>
+ filter(.draw %in% sample(.draw, 100))
+
+ggplot(mapping = aes(x = infection_day)) +
+ geom_line(
+ data = inf_posterior, mapping = aes(y = .value, group = .draw), alpha = 0.1
+ ) +
+ geom_line(data = inf_ts, mapping = aes(y = infections), colour = "red") +
+ labs(title = "Infections per day",
+ subtitle = "True data (red), and sample of estimated trajectories (black)")
+```
+
+::: callout-tip
+This time we used the `gather_draws()` function included in `tidybayes` to extract the inference results.
+This is particularly useful when dealing with arrays such as `inference` because it allows to extract them with a given index (here: `[day]`).
+:::
+
+# Going further
+
+- Above, we used a Poisson distribution to characterise uncertainty.
+ In the Poisson distribution, the variance is the same as the mean.
+ Another common choice is the negative binomial distribution, which has a more flexible relationship between variance and mean.
+ If you re-did the analysis above with the negative binomial distribution, what would be the difference?
+
+- We could have used the individual-level model of the previous section to try to estimate the number of infections with a known delay distribution by estimating each individual infection time.
+ How would this look in stan code?
+ Would you expect it to yield a different result?
+
+# Wrap up
diff --git a/styles.css b/styles.css
new file mode 100644
index 0000000..4179ec1
--- /dev/null
+++ b/styles.css
@@ -0,0 +1,3 @@
+element.style {
+ margin-bottom: 1em;
+}