You signed in with another tab or window. Reload to refresh your session.You signed out in another tab or window. Reload to refresh your session.You switched accounts on another tab or window. Reload to refresh your session.Dismiss alert
I tried to extract features and got very interesting results.
As you can see level 6 features was constant. I used different pictures and checked all of 196 feature maps and always got the same result. I also checked different weights for different realization of pwc-net. Can you please describe this result.
The text was updated successfully, but these errors were encountered:
Doing some gradient analysis I found gradient vanishing phenomena to the last correlation layer or encoding layer is quite strong....
My intuition is the correlation output usually has a much smaller scale than the feature tensor, thus the activation could diminish, so do the gradient to the last decoder and encoder layer.
(note that there is no direct connection from flow map to 6th layer feature tensor, all their gradient comes through the correlation layer, which seems like a correlation bottleneck )
I tried to extract features and got very interesting results.
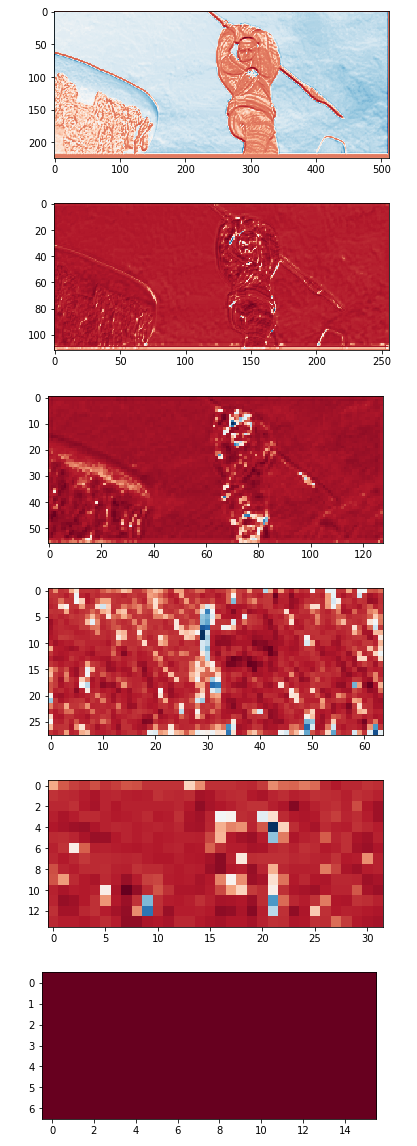
As you can see level 6 features was constant. I used different pictures and checked all of 196 feature maps and always got the same result. I also checked different weights for different realization of pwc-net. Can you please describe this result.
The text was updated successfully, but these errors were encountered: