forked from CjTouzi/edx-Scalable-Machine-Learning
-
Notifications
You must be signed in to change notification settings - Fork 0
/
Copy pathML_lab1_review_student.py
666 lines (473 loc) · 36.5 KB
/
ML_lab1_review_student.py
1
2
3
4
5
6
7
8
9
10
11
12
13
14
15
16
17
18
19
20
21
22
23
24
25
26
27
28
29
30
31
32
33
34
35
36
37
38
39
40
41
42
43
44
45
46
47
48
49
50
51
52
53
54
55
56
57
58
59
60
61
62
63
64
65
66
67
68
69
70
71
72
73
74
75
76
77
78
79
80
81
82
83
84
85
86
87
88
89
90
91
92
93
94
95
96
97
98
99
100
101
102
103
104
105
106
107
108
109
110
111
112
113
114
115
116
117
118
119
120
121
122
123
124
125
126
127
128
129
130
131
132
133
134
135
136
137
138
139
140
141
142
143
144
145
146
147
148
149
150
151
152
153
154
155
156
157
158
159
160
161
162
163
164
165
166
167
168
169
170
171
172
173
174
175
176
177
178
179
180
181
182
183
184
185
186
187
188
189
190
191
192
193
194
195
196
197
198
199
200
201
202
203
204
205
206
207
208
209
210
211
212
213
214
215
216
217
218
219
220
221
222
223
224
225
226
227
228
229
230
231
232
233
234
235
236
237
238
239
240
241
242
243
244
245
246
247
248
249
250
251
252
253
254
255
256
257
258
259
260
261
262
263
264
265
266
267
268
269
270
271
272
273
274
275
276
277
278
279
280
281
282
283
284
285
286
287
288
289
290
291
292
293
294
295
296
297
298
299
300
301
302
303
304
305
306
307
308
309
310
311
312
313
314
315
316
317
318
319
320
321
322
323
324
325
326
327
328
329
330
331
332
333
334
335
336
337
338
339
340
341
342
343
344
345
346
347
348
349
350
351
352
353
354
355
356
357
358
359
360
361
362
363
364
365
366
367
368
369
370
371
372
373
374
375
376
377
378
379
380
381
382
383
384
385
386
387
388
389
390
391
392
393
394
395
396
397
398
399
400
401
402
403
404
405
406
407
408
409
410
411
412
413
414
415
416
417
418
419
420
421
422
423
424
425
426
427
428
429
430
431
432
433
434
435
436
437
438
439
440
441
442
443
444
445
446
447
448
449
450
451
452
453
454
455
456
457
458
459
460
461
462
463
464
465
466
467
468
469
470
471
472
473
474
475
476
477
478
479
480
481
482
483
484
485
486
487
488
489
490
491
492
493
494
495
496
497
498
499
500
501
502
503
504
505
506
507
508
509
510
511
512
513
514
515
516
517
518
519
520
521
522
523
524
525
526
527
528
529
530
531
532
533
534
535
536
537
538
539
540
541
542
543
544
545
546
547
548
549
550
551
552
553
554
555
556
557
558
559
560
561
562
563
564
565
566
567
568
569
570
571
572
573
574
575
576
577
578
579
580
581
582
583
584
585
586
587
588
589
590
591
592
593
594
595
596
597
598
599
600
601
602
603
604
605
606
607
608
609
610
611
612
613
614
615
616
617
618
619
620
621
622
623
624
625
626
627
628
629
630
631
632
633
634
635
636
637
638
639
640
641
642
643
644
645
646
647
648
649
650
651
652
653
654
655
656
657
658
659
660
661
662
663
664
665
# coding: utf-8
# 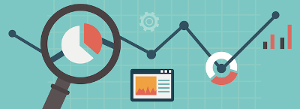
# # **Math and Python review and CTR data download**
# #### This notebook reviews vector and matrix math, the [NumPy](http://www.numpy.org/) Python package, and Python lambda expressions. It also covers downloading the data required for Lab 4, where you will analyze website click-through rates. Part 1 covers vector and matrix math, and you'll do a few exercises by hand. In Part 2, you'll learn about NumPy and use `ndarray` objects to solve the math exercises. Part 3 provides additional information about NumPy and how it relates to array usage in Spark's [MLlib](https://spark.apache.org/mllib/). Part 4 provides an overview of lambda expressions, and you'll wrap up by downloading the dataset for Lab 4.
# #### To move through the notebook just run each of the cells. You can run a cell by pressing "shift-enter", which will compute the current cell and advance to the next cell, or by clicking in a cell and pressing "control-enter", which will compute the current cell and remain in that cell. You should move through the notebook from top to bottom and run all of the cells. If you skip some cells, later cells might not work as expected.
# #### Note that there are several exercises within this notebook. You will need to provide solutions for cells that start with: `# TODO: Replace <FILL IN> with appropriate code`.
#
# #### ** This notebook covers: **
# #### *Part 1:* Math review
# #### *Part 2:* NumPy
# #### *Part 3:* Additional NumPy and Spark linear algebra
# #### *Part 4:* Python lambda expressions
# #### *Part 5:* CTR data download
# In[1]:
labVersion = 'cs190_week1_v_1_2'
# ### ** Part 1: Math review **
# #### ** (1a) Scalar multiplication: vectors **
# #### In this exercise, you will calculate the product of a scalar and a vector by hand and enter the result in the code cell below. Scalar multiplication is straightforward. The resulting vector equals the product of the scalar, which is a single value, and each item in the original vector. In the example below, $ a $ is the scalar (constant) and $ \mathbf{v} $ is the vector. $$ a \mathbf{v} = \begin{bmatrix} a v_1 \\\ a v_2 \\\ \vdots \\\ a v_n \end{bmatrix} $$
# #### Calculate the value of $ \mathbf{x} $: $$ \mathbf{x} = 3 \begin{bmatrix} 1 \\\ -2 \\\ 0 \end{bmatrix} $$
# #### Calculate the value of $ \mathbf{y} $: $$ \mathbf{y} = 2 \begin{bmatrix} 2 \\\ 4 \\\ 8 \end{bmatrix} $$
# In[2]:
# TODO: Replace <FILL IN> with appropriate code
# Manually calculate your answer and represent the vector as a list of integers values.
# For example, [2, 4, 8].
x = [3, -6, 0]
y = [4, 8, 16]
# In[3]:
# TEST Scalar multiplication: vectors (1a)
# Import test library
from test_helper import Test
Test.assertEqualsHashed(x, 'e460f5b87531a2b60e0f55c31b2e49914f779981',
'incorrect value for vector x')
Test.assertEqualsHashed(y, 'e2d37ff11427dbac7f833a5a7039c0de5a740b1e',
'incorrect value for vector y')
# #### ** (1b) Element-wise multiplication: vectors **
# #### In this exercise, you will calculate the element-wise multiplication of two vectors by hand and enter the result in the code cell below. You'll later see that element-wise multiplication is the default method when two NumPy arrays are multiplied together. Note we won't be performing element-wise multiplication in future labs, but we are introducing it here to distinguish it from other vector operators, and to because it is a common operations in NumPy, as we will discuss in Part (2b).
# #### The element-wise calculation is as follows: $$ \mathbf{x} \odot \mathbf{y} = \begin{bmatrix} x_1 y_1 \\\ x_2 y_2 \\\ \vdots \\\ x_n y_n \end{bmatrix} $$
# #### Calculate the value of $ \mathbf{z} $: $$ \mathbf{z} = \begin{bmatrix} 1 \\\ 2 \\\ 3 \end{bmatrix} \odot \begin{bmatrix} 4 \\\ 5 \\\ 6 \end{bmatrix} $$
# In[5]:
# TODO: Replace <FILL IN> with appropriate code
# Manually calculate your answer and represent the vector as a list of integers values.
z = [4, 10 ,18]
# In[6]:
# TEST Element-wise multiplication: vectors (1b)
Test.assertEqualsHashed(z, '4b5fe28ee2d274d7e0378bf993e28400f66205c2',
'incorrect value for vector z')
# #### ** (1c) Dot product **
# #### In this exercise, you will calculate the dot product of two vectors by hand and enter the result in the code cell below. Note that the dot product is equivalent to performing element-wise multiplication and then summing the result.
# #### Below, you'll find the calculation for the dot product of two vectors, where each vector has length $ n $: $$ \mathbf{w} \cdot \mathbf{x} = \sum_{i=1}^n w_i x_i $$
# #### Note that you may also see $ \mathbf{w} \cdot \mathbf{x} $ represented as $ \mathbf{w}^\top \mathbf{x} $
# #### Calculate the value for $ c_1 $ based on the dot product of the following two vectors:
# #### $$ c_1 = \begin{bmatrix} 1 \\\ -3 \end{bmatrix} \cdot \begin{bmatrix} 4 \\\ 5 \end{bmatrix}$$
# #### Calculate the value for $ c_2 $ based on the dot product of the following two vectors:
# #### $$ c_2 = \begin{bmatrix} 3 \\\ 4 \\\ 5 \end{bmatrix} \cdot \begin{bmatrix} 1 \\\ 2 \\\ 3 \end{bmatrix}$$
# In[7]:
# TODO: Replace <FILL IN> with appropriate code
# Manually calculate your answer and set the variables to their appropriate integer values.
c1 = -11
c2 = 26
# In[8]:
# TEST Dot product (1c)
Test.assertEqualsHashed(c1, '8d7a9046b6a6e21d66409ad0849d6ab8aa51007c', 'incorrect value for c1')
Test.assertEqualsHashed(c2, '887309d048beef83ad3eabf2a79a64a389ab1c9f', 'incorrect value for c2')
# #### ** (1d) Matrix multiplication **
# #### In this exercise, you will calculate the result of multiplying two matrices together by hand and enter the result in the code cell below.
# #### Below, you'll find the calculation for multiplying two matrices together. Note that the number of columns for the first matrix and the number of rows for the second matrix have to be equal and are are represented by $ n $:
# #### $$ [\mathbf{X} \mathbf{Y}]_{i,j} = \sum_{r=1}^n \mathbf{X}_{i,r} \mathbf{Y}_{r,j} $$
# #### First, you'll calculate the value for $ \mathbf{X} $.
# #### $$ \mathbf{X} = \begin{bmatrix} 1 & 2 & 3 \\\ 4 & 5 & 6 \end{bmatrix} \begin{bmatrix} 1 & 2 \\\ 3 & 4 \\\ 5 & 6 \end{bmatrix} $$
# #### Next, you'll perform an outer product and calculate the value for $ \mathbf{Y} $. Note that outer product is just a special case of general matrix multiplication and follows the same rules as normal matrix multiplication.
# #### $$ \mathbf{Y} = \begin{bmatrix} 1 \\\ 2 \\\ 3 \end{bmatrix} \begin{bmatrix} 1 & 2 & 3 \end{bmatrix} $$
# In[16]:
# TODO: Replace <FILL IN> with appropriate code
# Represent matrices as lists within lists. For example, [[1,2,3], [4,5,6]] represents a matrix with
# two rows and three columns. Use integer values.
X = [[22, 28],
[49, 64]]
Y = [[1, 2, 3],
[2, 4, 6],
[3, 6, 9]]
# In[17]:
# TEST Matrix multiplication (1d)
Test.assertEqualsHashed(X, 'c2ada2598d8a499e5dfb66f27a24f444483cba13',
'incorrect value for matrix X')
Test.assertEqualsHashed(Y, 'f985daf651531b7d776523836f3068d4c12e4519',
'incorrect value for matrix Y')
# ### ** Part 2: NumPy **
# #### ** (2a) Scalar multiplication **
# #### [NumPy](http://docs.scipy.org/doc/numpy/reference/) is a Python library for working with arrays. NumPy provides abstractions that make it easy to treat these underlying arrays as vectors and matrices. The library is optimized to be fast and memory efficient, and we'll be using it throughout the course. The building block for NumPy is the [ndarray](http://docs.scipy.org/doc/numpy/reference/generated/numpy.ndarray.html), which is a multidimensional array of fixed-size that contains elements of one type (e.g. array of floats).
# #### For this exercise, you'll create a `ndarray` consisting of the elements \[1, 2, 3\] and multiply this array by 5. Use [np.array()](http://docs.scipy.org/doc/numpy/reference/generated/numpy.array.html) to create the array. Note that you can pass a Python list into `np.array()`. To perform scalar multiplication with an `ndarray` just use `*`.
# #### Note that if you create an array from a Python list of integers you will obtain a one-dimensional array, *which is equivalent to a vector for our purposes*.
# In[18]:
# It is convention to import NumPy with the alias np
import numpy as np
# In[19]:
# TODO: Replace <FILL IN> with appropriate code
# Create a numpy array with the values 1, 2, 3
simpleArray = np.array([1,2,3])
# Perform the scalar product of 5 and the numpy array
timesFive = 5* simpleArray
print simpleArray
print timesFive
# In[20]:
# TEST Scalar multiplication (2a)
Test.assertTrue(np.all(timesFive == [5, 10, 15]), 'incorrect value for timesFive')
# #### ** (2b) Element-wise multiplication and dot product **
# #### NumPy arrays support both element-wise multiplication and dot product. Element-wise multiplication occurs automatically when you use the `*` operator to multiply two `ndarray` objects of the same length.
# #### To perform the dot product you can use either [np.dot()](http://docs.scipy.org/doc/numpy/reference/generated/numpy.dot.html#numpy.dot) or [np.ndarray.dot()](http://docs.scipy.org/doc/numpy/reference/generated/numpy.ndarray.dot.html). For example, if you had NumPy arrays `x` and `y`, you could compute their dot product four ways: `np.dot(x, y)`, `np.dot(y, x)`, `x.dot(y)`, or `y.dot(x)`.
# #### For this exercise, multiply the arrays `u` and `v` element-wise and compute their dot product.
# In[23]:
# TODO: Replace <FILL IN> with appropriate code
# Create a ndarray based on a range and step size.
u = np.arange(0, 5, .5)
v = np.arange(5, 10, .5)
elementWise = u * v
dotProduct = np.dot(u, v)
print 'u: {0}'.format(u)
print 'v: {0}'.format(v)
print '\nelementWise\n{0}'.format(elementWise)
print '\ndotProduct\n{0}'.format(dotProduct)
# In[24]:
# TEST Element-wise multiplication and dot product (2b)
Test.assertTrue(np.all(elementWise == [ 0., 2.75, 6., 9.75, 14., 18.75, 24., 29.75, 36., 42.75]),
'incorrect value for elementWise')
Test.assertEquals(dotProduct, 183.75, 'incorrect value for dotProduct')
# #### ** (2c) Matrix math **
# #### With NumPy it is very easy to perform matrix math. You can use [np.matrix()](http://docs.scipy.org/doc/numpy/reference/generated/numpy.matrix.html) to generate a NumPy matrix. Just pass a two-dimensional `ndarray` or a list of lists to the function. You can perform matrix math on NumPy matrices using `*`.
# #### You can transpose a matrix by calling [numpy.matrix.transpose()](http://docs.scipy.org/doc/numpy/reference/generated/numpy.matrix.transpose.html) or by using `.T` on the matrix object (e.g. `myMatrix.T`). Transposing a matrix produces a matrix where the new rows are the columns from the old matrix. For example: $$ \begin{bmatrix} 1 & 2 & 3 \\\ 4 & 5 & 6 \end{bmatrix}^\mathbf{\top} = \begin{bmatrix} 1 & 4 \\\ 2 & 5 \\\ 3 & 6 \end{bmatrix} $$
#
# #### Inverting a matrix can be done using [numpy.linalg.inv()](http://docs.scipy.org/doc/numpy/reference/generated/numpy.linalg.inv.html). Note that only square matrices can be inverted, and square matrices are not guaranteed to have an inverse. If the inverse exists, then multiplying a matrix by its inverse will produce the identity matrix. $ \scriptsize ( \mathbf{A}^{-1} \mathbf{A} = \mathbf{I_n} ) $ The identity matrix $ \scriptsize \mathbf{I_n} $ has ones along its diagonal and zero elsewhere. $$ \mathbf{I_n} = \begin{bmatrix} 1 & 0 & 0 & \dots & 0 \\\ 0 & 1 & 0 & \dots & 0 \\\ 0 & 0 & 1 & \dots & 0 \\\ \vdots & \vdots & \vdots & \ddots & \vdots \\\ 0 & 0 & 0 & \dots & 1 \end{bmatrix} $$
# #### For this exercise, multiply $ \mathbf{A} $ times its transpose $ ( \mathbf{A}^\top ) $ and then calculate the inverse of the result $ ( [ \mathbf{A} \mathbf{A}^\top ]^{-1} ) $.
# In[26]:
# TODO: Replace <FILL IN> with appropriate code
from numpy.linalg import inv
A = np.matrix([[1,2,3,4],[5,6,7,8]])
print 'A:\n{0}'.format(A)
# Print A transpose
print '\nA transpose:\n{0}'.format(A.T)
# Multiply A by A transpose
AAt = A * A.T
print '\nAAt:\n{0}'.format(AAt)
# Invert AAt with np.linalg.inv()
AAtInv =np.linalg.inv(AAt)
print '\nAAtInv:\n{0}'.format(AAtInv)
# Show inverse times matrix equals identity
# We round due to numerical precision
print '\nAAtInv * AAt:\n{0}'.format((AAtInv * AAt).round(4))
# In[27]:
# TEST Matrix math (2c)
Test.assertTrue(np.all(AAt == np.matrix([[30, 70], [70, 174]])), 'incorrect value for AAt')
Test.assertTrue(np.allclose(AAtInv, np.matrix([[0.54375, -0.21875], [-0.21875, 0.09375]])),
'incorrect value for AAtInv')
# ### ** Part 3: Additional NumPy and Spark linear algebra **
# #### ** (3a) Slices **
# #### You can select a subset of a one-dimensional NumPy `ndarray`'s elements by using slices. These slices operate the same way as slices for Python lists. For example, `[0, 1, 2, 3][:2]` returns the first two elements `[0, 1]`. NumPy, additionally, has more sophisticated slicing that allows slicing across multiple dimensions; however, you'll only need to use basic slices in future labs for this course.
# #### Note that if no index is placed to the left of a `:`, it is equivalent to starting at 0, and hence `[0, 1, 2, 3][:2]` and `[0, 1, 2, 3][0:2]` yield the same result. Similarly, if no index is placed to the right of a `:`, it is equivalent to slicing to the end of the object. Also, you can use negative indices to index relative to the end of the object, so `[-2:]` would return the last two elements of the object.
# #### For this exercise, return the last 3 elements of the array `features`.
# In[28]:
# TODO: Replace <FILL IN> with appropriate code
features = np.array([1, 2, 3, 4])
print 'features:\n{0}'.format(features)
# The last three elements of features
lastThree = features[-3:]
print '\nlastThree:\n{0}'.format(lastThree)
# In[29]:
# TEST Slices (3a)
Test.assertTrue(np.all(lastThree == [2, 3, 4]), 'incorrect value for lastThree')
# #### ** (3b) Combining `ndarray` objects **
# #### NumPy provides many functions for creating new arrays from existing arrays. We'll explore two functions: [np.hstack()](http://docs.scipy.org/doc/numpy/reference/generated/numpy.hstack.html), which allows you to combine arrays column-wise, and [np.vstack()](http://docs.scipy.org/doc/numpy/reference/generated/numpy.vstack.html), which allows you to combine arrays row-wise. Note that both `np.hstack()` and `np.vstack()` take in a tuple of arrays as their first argument. To horizontally combine three arrays `a`, `b`, and `c`, you would run `np.hstack((a, b, c))`.
# #### If we had two arrays: `a = [1, 2, 3, 4]` and `b = [5, 6, 7, 8]`, we could use `np.vstack((a, b))` to produce the two-dimensional array: $$ \begin{bmatrix} 1 & 2 & 3 & 4 \\\ 5 & 6 & 7 & 8 \end{bmatrix} $$
# #### For this exercise, you'll combine the `zeros` and `ones` arrays both horizontally (column-wise) and vertically (row-wise).
# #### Note that the result of stacking two arrays is an `ndarray`. If you need the result to be a matrix, you can call `np.matrix()` on the result, which will return a NumPy matrix.
# In[30]:
# TODO: Replace <FILL IN> with appropriate code
zeros = np.zeros(8)
ones = np.ones(8)
print 'zeros:\n{0}'.format(zeros)
print '\nones:\n{0}'.format(ones)
zerosThenOnes = np.hstack((zeros, ones)) # A 1 by 16 array
zerosAboveOnes = np.vstack((zeros, ones)) # A 2 by 8 array
print '\nzerosThenOnes:\n{0}'.format(zerosThenOnes)
print '\nzerosAboveOnes:\n{0}'.format(zerosAboveOnes)
# In[31]:
# TEST Combining ndarray objects (3b)
Test.assertTrue(np.all(zerosThenOnes == [0,0,0,0,0,0,0,0,1,1,1,1,1,1,1,1]),
'incorrect value for zerosThenOnes')
Test.assertTrue(np.all(zerosAboveOnes == [[0,0,0,0,0,0,0,0],[1,1,1,1,1,1,1,1]]),
'incorrect value for zerosAboveOnes')
# #### ** (3c) PySpark's DenseVector **
# #### PySpark provides a [DenseVector](https://spark.apache.org/docs/latest/api/python/pyspark.mllib.html#pyspark.mllib.linalg.DenseVector) class within the module [pyspark.mllib.linalg](https://spark.apache.org/docs/latest/api/python/pyspark.mllib.html#module-pyspark.mllib.linalg). `DenseVector` is used to store arrays of values for use in PySpark. `DenseVector` actually stores values in a NumPy array and delegates calculations to that object. You can create a new `DenseVector` using `DenseVector()` and passing in an NumPy array or a Python list.
# #### `DenseVector` implements several functions. The only function needed for this course is `DenseVector.dot()`, which operates just like `np.ndarray.dot()`.
# #### Note that `DenseVector` stores all values as `np.float64`, so even if you pass in an NumPy array of integers, the resulting `DenseVector` will contain floating-point numbers. Also, `DenseVector` objects exist locally and are not inherently distributed. `DenseVector` objects can be used in the distributed setting by either passing functions that contain them to resilient distributed dataset (RDD) transformations or by distributing them directly as RDDs. You'll learn more about RDDs in the spark tutorial.
# #### For this exercise, create a `DenseVector` consisting of the values `[3.0, 4.0, 5.0]` and compute the dot product of this vector with `numpyVector`.
# In[33]:
from pyspark.mllib.linalg import DenseVector
# In[36]:
# TODO: Replace <FILL IN> with appropriate code
numpyVector = np.array([-3, -4, 5])
print '\nnumpyVector:\n{0}'.format(numpyVector)
# Create a DenseVector consisting of the values [3.0, 4.0, 5.0]
myDenseVector = DenseVector([3.0, 4.0, 5.0])
# Calculate the dot product between the two vectors.
denseDotProduct = DenseVector.dot(myDenseVector, numpyVector)
print 'myDenseVector:\n{0}'.format(myDenseVector)
print '\ndenseDotProduct:\n{0}'.format(denseDotProduct)
# In[37]:
# TEST PySpark's DenseVector (3c)
Test.assertTrue(isinstance(myDenseVector, DenseVector), 'myDenseVector is not a DenseVector')
Test.assertTrue(np.allclose(myDenseVector, np.array([3., 4., 5.])),
'incorrect value for myDenseVector')
Test.assertTrue(np.allclose(denseDotProduct, 0.0), 'incorrect value for denseDotProduct')
# ### ** Part 4: Python lambda expressions **
# #### ** (4a) Lambda is an anonymous function **
# #### We can use a lambda expression to create a function. To do this, you type `lambda` followed by the names of the function's parameters separated by commas, followed by a `:`, and then the expression statement that the function will evaluate. For example, `lambda x, y: x + y` is an anonymous function that computes the sum of its two inputs.
# #### Lambda expressions return a function when evaluated. The function is not bound to any variable, which is why lambdas are associated with anonymous functions. However, it is possible to assign the function to a variable. Lambda expressions are particularly useful when you need to pass a simple function into another function. In that case, the lambda expression generates a function that is bound to the parameter being passed into the function.
# #### Below, we'll see an example of how we can bind the function returned by a lambda expression to a variable named `addSLambda`. From this example, we can see that `lambda` provides a shortcut for creating a simple function. Note that the behavior of the function created using `def` and the function created using `lambda` is equivalent. Both functions have the same type and return the same results. The only differences are the names and the way they were created.
# #### For this exercise, first run the two cells below to compare a function created using `def` with a corresponding anonymous function. Next, write your own lambda expression that creates a function that multiplies its input (a single parameter) by 10.
# #### Here are some additional references that explain lambdas: [Lambda Functions](http://www.secnetix.de/olli/Python/lambda_functions.hawk), [Lambda Tutorial](https://pythonconquerstheuniverse.wordpress.com/2011/08/29/lambda_tutorial/), and [Python Functions](http://www.bogotobogo.com/python/python_functions_lambda.php).
# In[38]:
# Example function
def addS(x):
return x + 's'
print type(addS)
print addS
print addS('cat')
# In[39]:
# As a lambda
addSLambda = lambda x: x + 's'
print type(addSLambda)
print addSLambda
print addSLambda('cat')
# In[40]:
# TODO: Replace <FILL IN> with appropriate code
# Recall that: "lambda x, y: x + y" creates a function that adds together two numbers
multiplyByTen = lambda x: x*10
print multiplyByTen(5)
# Note that the function still shows its name as <lambda>
print '\n', multiplyByTen
# In[41]:
# TEST Python lambda expressions (4a)
Test.assertEquals(multiplyByTen(10), 100, 'incorrect definition for multiplyByTen')
# #### ** (4b) `lambda` fewer steps than `def` **
# #### `lambda` generates a function and returns it, while `def` generates a function and assigns it to a name. The function returned by `lambda` also automatically returns the value of its expression statement, which reduces the amount of code that needs to be written.
# #### For this exercise, recreate the `def` behavior using `lambda`. Note that since a lambda expression returns a function, it can be used anywhere an object is expected. For example, you can create a list of functions where each function in the list was generated by a lambda expression.
# In[42]:
# Code using def that we will recreate with lambdas
def plus(x, y):
return x + y
def minus(x, y):
return x - y
functions = [plus, minus]
print functions[0](4, 5)
print functions[1](4, 5)
# In[43]:
# TODO: Replace <FILL IN> with appropriate code
# The first function should add two values, while the second function should subtract the second
# value from the first value.
lambdaFunctions = [lambda x, y: x+y, lambda x, y: x-y]
print lambdaFunctions[0](4, 5)
print lambdaFunctions[1](4, 5)
# In[44]:
# TEST lambda fewer steps than def (4b)
Test.assertEquals(lambdaFunctions[0](10, 10), 20, 'incorrect first lambdaFunction')
Test.assertEquals(lambdaFunctions[1](10, 10), 0, 'incorrect second lambdaFunction')
# #### ** (4c) Lambda expression arguments **
# #### Lambda expressions can be used to generate functions that take in zero or more parameters. The syntax for `lambda` allows for multiple ways to define the same function. For example, we might want to create a function that takes in a single parameter, where the parameter is a tuple consisting of two values, and the function adds the two values together. The syntax could be either: `lambda x: x[0] + x[1]` or `lambda (x0, x1): x0 + x1`. If we called either function on the tuple `(3, 4)` it would return `7`. Note that the second `lambda` relies on the tuple `(3, 4)` being unpacked automatically, which means that `x0` is assigned the value `3` and `x1` is assigned the value `4`.
# #### As an other example, consider the following parameter lambda expressions: `lambda x, y: (x[0] + y[0], x[1] + y[1])` and `lambda (x0, x1), (y0, y1): (x0 + y0, x1 + y1)`. The result of applying either of these functions to tuples `(1, 2)` and `(3, 4)` would be the tuple `(4, 6)`.
# #### For this exercise: you'll create one-parameter functions `swap1` and `swap2` that swap the order of a tuple; a one-parameter function `swapOrder` that takes in a tuple with three values and changes the order to: second element, third element, first element; and finally, a three-parameter function `sumThree` that takes in three tuples, each with two values, and returns a tuple containing two values: the sum of the first element of each tuple and the sum of second element of each tuple.
# In[45]:
# Examples. Note that the spacing has been modified to distinguish parameters from tuples.
# One-parameter function
a1 = lambda x: x[0] + x[1]
a2 = lambda (x0, x1): x0 + x1
print 'a1( (3,4) ) = {0}'.format( a1( (3,4) ) )
print 'a2( (3,4) ) = {0}'.format( a2( (3,4) ) )
# Two-parameter function
b1 = lambda x, y: (x[0] + y[0], x[1] + y[1])
b2 = lambda (x0, x1), (y0, y1): (x0 + y0, x1 + y1)
print '\nb1( (1,2), (3,4) ) = {0}'.format( b1( (1,2), (3,4) ) )
print 'b2( (1,2), (3,4) ) = {0}'.format( b2( (1,2), (3,4) ) )
# In[46]:
# TODO: Replace <FILL IN> with appropriate code
# Use both syntaxes to create a function that takes in a tuple of two values and swaps their order
# E.g. (1, 2) => (2, 1)
swap1 = lambda x: (x[1],x[0])
swap2 = lambda (x0, x1): (x1, x0)
print 'swap1((1, 2)) = {0}'.format(swap1((1, 2)))
print 'swap2((1, 2)) = {0}'.format(swap2((1, 2)))
# Using either syntax, create a function that takes in a tuple with three values and returns a tuple
# of (2nd value, 3rd value, 1st value). E.g. (1, 2, 3) => (2, 3, 1)
swapOrder = lambda x: (x[1],x[2], x[0])
print 'swapOrder((1, 2, 3)) = {0}'.format(swapOrder((1, 2, 3)))
# Using either syntax, create a function that takes in three tuples each with two values. The
# function should return a tuple with the values in the first position summed and the values in the
# second position summed. E.g. (1, 2), (3, 4), (5, 6) => (1 + 3 + 5, 2 + 4 + 6) => (9, 12)
sumThree =lambda x, y, z:(x[0]+y[0]+z[0],x[1]+y[1]+z[1] )
print 'sumThree((1, 2), (3, 4), (5, 6)) = {0}'.format(sumThree((1, 2), (3, 4), (5, 6)))
# In[47]:
# TEST Lambda expression arguments (4c)
Test.assertEquals(swap1((1, 2)), (2, 1), 'incorrect definition for swap1')
Test.assertEquals(swap2((1, 2)), (2, 1), 'incorrect definition for swap2')
Test.assertEquals(swapOrder((1, 2, 3)), (2, 3, 1), 'incorrect definition fo swapOrder')
Test.assertEquals(sumThree((1, 2), (3, 4), (5, 6)), (9, 12), 'incorrect definition for sumThree')
# #### ** (4d) Restrictions on lambda expressions **
# #### [Lambda expressions](https://docs.python.org/2/reference/expressions.html#lambda) consist of a single [expression statement](https://docs.python.org/2/reference/simple_stmts.html#expression-statements) and cannot contain other [simple statements](https://docs.python.org/2/reference/simple_stmts.html). In short, this means that the lambda expression needs to evaluate to a value and exist on a single logical line. If more complex logic is necessary, use `def` in place of `lambda`.
# #### Expression statements evaluate to a value (sometimes that value is None). Lambda expressions automatically return the value of their expression statement. In fact, a `return` statement in a `lambda` would raise a `SyntaxError`.
# #### The following Python keywords refer to simple statements that cannot be used in a lambda expression: `assert`, `pass`, `del`, `print`, `return`, `yield`, `raise`, `break`, `continue`, `import`, `global`, and `exec`. Also, note that assignment statements (`=`) and augmented assignment statements (e.g. `+=`) cannot be used either.
# In[48]:
# Just run this code
# This code will fail with a syntax error, as we can't use print in a lambda expression
import traceback
try:
exec "lambda x: print x"
except:
traceback.print_exc()
# #### ** (4e) Functional programming **
# #### The `lambda` examples we have shown so far have been somewhat contrived. This is because they were created to demonstrate the differences and similarities between `lambda` and `def`. An excellent use case for lambda expressions is functional programming. In functional programming, you will often pass functions to other functions as parameters, and `lambda` can be used to reduce the amount of code necessary and to make the code more readable.
# #### Some commonly used functions in functional programming are map, filter, and reduce. Map transforms a series of elements by applying a function individually to each element in the series. It then returns the series of transformed elements. Filter also applies a function individually to each element in a series; however, with filter, this function evaluates to `True` or `False` and only elements that evaluate to `True` are retained. Finally, reduce operates on pairs of elements in a series. It applies a function that takes in two values and returns a single value. Using this function, reduce is able to, iteratively, "reduce" a series to a single value.
# #### For this exercise, you'll create three simple `lambda` functions, one each for use in map, filter, and reduce. The map `lambda` will multiply its input by 5, the filter `lambda` will evaluate to `True` for even numbers, and the reduce `lambda` will add two numbers. Note that we have created a class called `FunctionalWrapper` so that the syntax for this exercise matches the syntax you'll see in PySpark.
# #### Note that map requires a one parameter function that returns a new value, filter requires a one parameter function that returns `True` or `False`, and reduce requires a two parameter function that combines the two parameters and returns a new value.
# In[49]:
# Create a class to give our examples the same syntax as PySpark
class FunctionalWrapper(object):
def __init__(self, data):
self.data = data
def map(self, function):
"""Call `map` on the items in `data` using the provided `function`"""
return FunctionalWrapper(map(function, self.data))
def reduce(self, function):
"""Call `reduce` on the items in `data` using the provided `function`"""
return reduce(function, self.data)
def filter(self, function):
"""Call `filter` on the items in `data` using the provided `function`"""
return FunctionalWrapper(filter(function, self.data))
def __eq__(self, other):
return (isinstance(other, self.__class__)
and self.__dict__ == other.__dict__)
def __getattr__(self, name): return getattr(self.data, name)
def __getitem__(self, k): return self.data.__getitem__(k)
def __repr__(self): return 'FunctionalWrapper({0})'.format(repr(self.data))
def __str__(self): return 'FunctionalWrapper({0})'.format(str(self.data))
# In[50]:
# Map example
# Create some data
mapData = FunctionalWrapper(range(5))
# Define a function to be applied to each element
f = lambda x: x + 3
# Imperative programming: loop through and create a new object by applying f
mapResult = FunctionalWrapper([]) # Initialize the result
for element in mapData:
mapResult.append(f(element)) # Apply f and save the new value
print 'Result from for loop: {0}'.format(mapResult)
# Functional programming: use map rather than a for loop
print 'Result from map call: {0}'.format(mapData.map(f))
# Note that the results are the same but that the map function abstracts away the implementation
# and requires less code
# In[51]:
# TODO: Replace <FILL IN> with appropriate code
dataset = FunctionalWrapper(range(10))
# Multiply each element by 5
mapResult = dataset.map(lambda x: x*5)
# Keep the even elements
# Note that "x % 2" evaluates to the remainder of x divided by 2
filterResult = dataset.filter(lambda x: x%2==0)
# Sum the elements
reduceResult = dataset.reduce(lambda x,y: x+y)
print 'mapResult: {0}'.format(mapResult)
print '\nfilterResult: {0}'.format(filterResult)
print '\nreduceResult: {0}'.format(reduceResult)
# In[52]:
# TEST Functional programming (4e)
Test.assertEquals(mapResult, FunctionalWrapper([0, 5, 10, 15, 20, 25, 30, 35, 40, 45]),
'incorrect value for mapResult')
Test.assertEquals(filterResult, FunctionalWrapper([0, 2, 4, 6, 8]),
'incorrect value for filterResult')
Test.assertEquals(reduceResult, 45, 'incorrect value for reduceResult')
# #### ** (4f) Composability **
# #### Since our methods for map and filter in the `FunctionalWrapper` class return `FunctionalWrapper` objects, we can compose (or chain) together our function calls. For example, `dataset.map(f1).filter(f2).reduce(f3)`, where `f1`, `f2`, and `f3` are functions or lambda expressions, first applies a map operation to `dataset`, then filters the result from map, and finally reduces the result from the first two operations.
# #### Note that when we compose (chain) an operation, the output of one operation becomes the input for the next operation, and operations are applied from left to right. It's likely you've seen chaining used with Python strings. For example, `'Split this'.lower().split(' ')` first returns a new string object `'split this'` and then `split(' ')` is called on that string to produce `['split', 'this']`.
# #### For this exercise, reuse your lambda expressions from (4e) but apply them to `dataset` in the sequence: map, filter, reduce. Note that since we are composing the operations our result will be different than in (4e). Also, we can write our operations on separate lines to improve readability.
# In[53]:
# Example of a mult-line expression statement
# Note that placing parentheses around the expression allow it to exist on multiple lines without
# causing a syntax error.
(dataset
.map(lambda x: x + 2)
.reduce(lambda x, y: x * y))
# In[54]:
# TODO: Replace <FILL IN> with appropriate code
# Multiply the elements in dataset by five, keep just the even values, and sum those values
finalSum = (dataset
.map(lambda x: x*5)
.filter(lambda x: x%2==0)
.reduce(lambda x,y: x+y))
print finalSum
# In[55]:
# TEST Composability (4f)
Test.assertEquals(finalSum, 100, 'incorrect value for finalSum')
# ### ** Part 5: CTR data download **
# #### Lab four will explore website click-through data provided by Criteo. To obtain the data, you must first accept Criteo's data sharing agreement. Below is the agreement from Criteo. After you accept the agreement, you can obtain the download URL by right-clicking on the "Download Sample" button and clicking "Copy link address" or "Copy Link Location", depending on your browser. Paste the URL into the `# TODO` cell below. The file is 8.4 MB compressed. The script below will download the file to the virtual machine (VM) and then extract the data.
# #### If running the cell below does not render a webpage, open the [Criteo agreement](http://labs.criteo.com/downloads/2014-kaggle-display-advertising-challenge-dataset/) in a separate browser tab. After you accept the agreement, you can obtain the download URL by right-clicking on the "Download Sample" button and clicking "Copy link address" or "Copy Link Location", depending on your browser. Paste the URL into the `# TODO` cell below.
# #### Note that the download could take a few minutes, depending upon your connection speed.
# In[56]:
# Run this code to view Criteo's agreement
# Note that some ad blocker software will prevent this IFrame from loading.
# If this happens, open the webpage in a separate tab and follow the instructions from above.
from IPython.lib.display import IFrame
IFrame("http://labs.criteo.com/downloads/2014-kaggle-display-advertising-challenge-dataset/",
600, 350)
# In[64]:
# TODO: Replace <FILL IN> with appropriate code
# Just replace <FILL IN> with the url for dac_sample.tar.gz
import glob
import os.path
import tarfile
import urllib
import urlparse
# Paste url, url should end with: dac_sample.tar.gz
url = 'https://s3-eu-west-1.amazonaws.com/criteo-labs/dac.tar.gz'
url = url.strip()
baseDir = os.path.join('data')
inputPath = os.path.join('cs190', 'dac_sample.txt')
fileName = os.path.join(baseDir, inputPath)
inputDir = os.path.split(fileName)[0]
def extractTar(check = False):
# Find the zipped archive and extract the dataset
tars = glob.glob('dac_sample*.tar.gz*')
if check and len(tars) == 0:
return False
if len(tars) > 0:
try:
tarFile = tarfile.open(tars[0])
except tarfile.ReadError:
if not check:
print 'Unable to open tar.gz file. Check your URL.'
return False
tarFile.extract('dac_sample.txt', path=inputDir)
print 'Successfully extracted: dac_sample.txt'
return True
else:
print 'You need to retry the download with the correct url.'
print ('Alternatively, you can upload the dac_sample.tar.gz file to your Jupyter root ' +
'directory')
return False
if os.path.isfile(fileName):
print 'File is already available. Nothing to do.'
elif extractTar(check = True):
print 'tar.gz file was already available.'
elif not url.endswith('dac_sample.tar.gz'):
print 'Check your download url. Are you downloading the Sample dataset?'
else:
# Download the file and store it in the same directory as this notebook
try:
urllib.urlretrieve(url, os.path.basename(urlparse.urlsplit(url).path))
except IOError:
print 'Unable to download and store: {0}'.format(url)
extractTar()
# In[65]:
import os.path
baseDir = os.path.join('data')
inputPath = os.path.join('cs190', 'dac_sample.txt')
fileName = os.path.join(baseDir, inputPath)
if os.path.isfile(fileName):
rawData = (sc
.textFile(fileName, 2)
.map(lambda x: x.replace('\t', ','))) # work with either ',' or '\t' separated data
print rawData.take(1)
rawDataCount = rawData.count()
print rawDataCount
# This line tests that the correct number of observations have been loaded
assert rawDataCount == 100000, 'incorrect count for rawData'
if rawDataCount == 100000:
print 'Criteo data loaded successfully!'