-
Notifications
You must be signed in to change notification settings - Fork 0
Commit
This commit does not belong to any branch on this repository, and may belong to a fork outside of the repository.
add Least squares algorithm in opt_alg.md
- Loading branch information
Showing
3 changed files
with
203 additions
and
18 deletions.
There are no files selected for viewing
This file was deleted.
Oops, something went wrong.
This file contains bidirectional Unicode text that may be interpreted or compiled differently than what appears below. To review, open the file in an editor that reveals hidden Unicode characters.
Learn more about bidirectional Unicode characters
This file contains bidirectional Unicode text that may be interpreted or compiled differently than what appears below. To review, open the file in an editor that reveals hidden Unicode characters.
Learn more about bidirectional Unicode characters
Original file line number | Diff line number | Diff line change |
---|---|---|
@@ -0,0 +1,202 @@ | ||
--- | ||
draft: true | ||
comments: true | ||
slug: opt_alg | ||
title: "nndl-优化算法" | ||
date: 2025-01-17 | ||
categories: | ||
- 算法 | ||
tags: | ||
- Neural Network | ||
- Deep Learning | ||
- Algorithm | ||
--- | ||
|
||
## 随机梯度下降法 | ||
<figure markdown="span" class="hover-zoom"> | ||
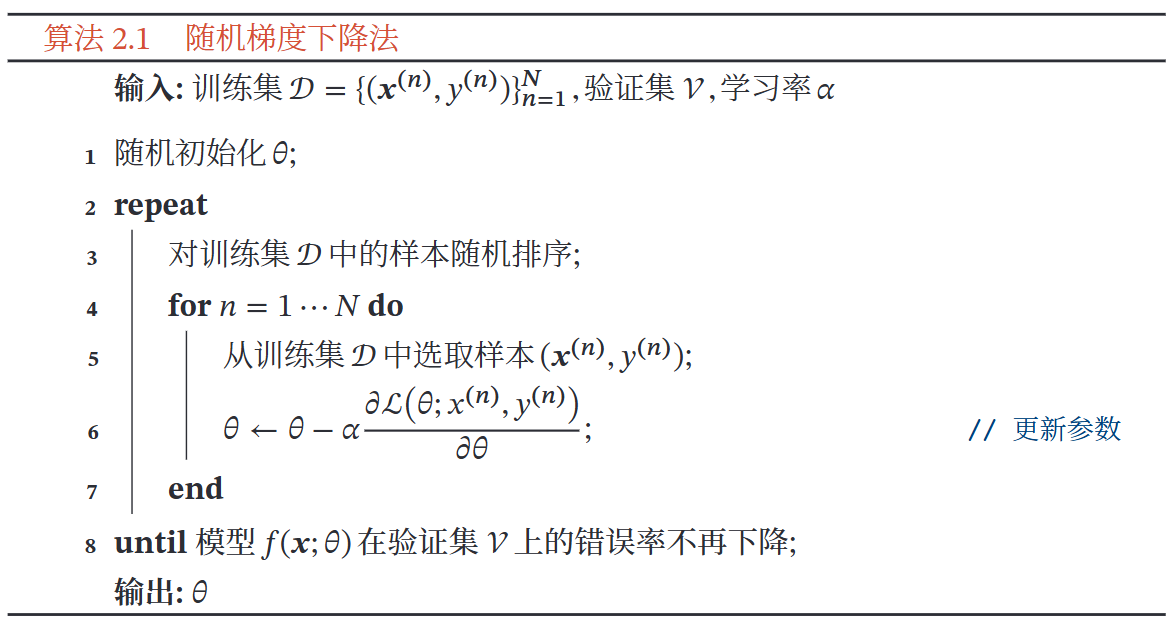{width=500} | ||
</figure> | ||
=== "生成数据点" | ||
``` python | ||
import numpy as np | ||
n = 500 | ||
m = 5 | ||
w = np.array([[3,2,6,1,7]]).T | ||
b = 5 | ||
print('shape of w:',w.shape) | ||
np.random.seed(42) | ||
x = np.random.rand(m, n) | ||
x_aug = np.pad(x, ((0, 1), (0, 0)), 'constant', constant_values=1) | ||
# print(x_aug) | ||
w_aug = np.pad(w, ((0, 1), (0, 0)), 'constant', constant_values=b) | ||
y = np.dot(w_aug.T,x_aug) | ||
print(y.shape) | ||
# print(augmented_matrix) | ||
# 添加高斯噪声 | ||
mu, sigma = 0, 1 # 均值和标准差 | ||
noise = np.random.normal(mu, sigma, y.shape) | ||
print(noise.shape) | ||
y_noisy = y + noise | ||
from sklearn.model_selection import train_test_split | ||
|
||
# 转置 x_aug 和 y_noisy 以进行打乱和分割 | ||
x_aug = x_aug.T | ||
y_noisy = y_noisy.T | ||
|
||
# 将数据打乱并分成训练集和测试集 | ||
x_train, x_test, y_train, y_test = train_test_split(x_aug, y_noisy, test_size=0.2, random_state=42) | ||
|
||
# 输出结果 | ||
print("训练集 X 的形状:", x_train[:,:-1].shape) | ||
print("测试集 X 的形状:", x_test.shape) | ||
print("训练集 Y 的形状:", y_train.shape) | ||
print("测试集 Y 的形状:", y_test.shape) | ||
|
||
``` | ||
=== "线性模型,损失函数和梯度" | ||
``` python | ||
# 线性模型函数 | ||
def line_fun(x, w, b): | ||
return np.dot(w.T, x)+b | ||
|
||
|
||
# 设定一个平方损失函数的梯度优化 | ||
def gradient_optimization(x, y, w, b): | ||
y_pre = line_fun(x, w, b) | ||
grad_w = -(y - y_pre) * x | ||
grad_b = -(y - y_pre) | ||
# print(f"grad_w {grad_w.shape}, grad_b {grad_b.shape}") | ||
return grad_w, grad_b | ||
# 损失函数 | ||
def loss_function(x, y, k, b): | ||
y_pre = line_fun(x, k, b) | ||
return np.mean((y - y_pre) ** 2) | ||
``` | ||
=== "优化:随机梯度下降法" | ||
``` python | ||
# 设定学习变量w和b的初始值 | ||
w = np.ones((5, 1)) # 初始权重向量,维度为5*1 | ||
b = 0.0 | ||
learning_rate = 0.001 | ||
decay_rate = 0.5 | ||
|
||
tolerance = 1e-6 | ||
max_epochs = 1000 | ||
min_improvement = 1e-6 | ||
patience = 10 | ||
|
||
# 训练过程 | ||
previous_loss = float('inf') | ||
improvement_streak = 0 | ||
|
||
# 训练集循环 | ||
for i in range(max_epochs): | ||
learning_rate = 0.01 / (1 + decay_rate * i) | ||
current_loss = 0 | ||
for n in range(x_train.shape[0]): | ||
x_n = x_train[n, :-1].reshape(5,1) # 输入特征向量 | ||
y_n = y_train[n] # 目标值 | ||
grad_w, grad_b = gradient_optimization(x_n, y_n, w, b) | ||
# print(f"grad_w {grad_w.T}, grad_b {grad_b}") | ||
w = w - learning_rate * grad_w | ||
b = b - learning_rate * grad_b | ||
current_loss += loss_function(x_n, y_n, w, b) | ||
|
||
current_loss /= x_train.shape[0] | ||
if abs(previous_loss - current_loss) < min_improvement: | ||
improvement_streak += 1 | ||
else: | ||
improvement_streak = 0 | ||
|
||
if improvement_streak >= patience: | ||
print(f"Training stopped after {i} epochs due to no significant improvement.") | ||
break | ||
|
||
previous_loss = current_loss | ||
|
||
print(f"Final values: w={w.T},\n b={b}, loss={previous_loss}") | ||
|
||
|
||
``` | ||
|
||
## 最小二乘法 | ||
|
||
=== "生成数据点" | ||
``` python | ||
import numpy as np | ||
from sklearn.model_selection import train_test_split | ||
|
||
n = 500 | ||
m = 5 | ||
w = np.array([[3,2,6,1,7]]).T | ||
b = 5 | ||
np.random.seed(42) | ||
x = np.random.rand(m, n) | ||
x_aug = np.pad(x, ((0, 1), (0, 0)), 'constant', constant_values=1) | ||
w_aug = np.pad(w, ((0, 1), (0, 0)), 'constant', constant_values=b) | ||
y = np.dot(w_aug.T,x_aug) | ||
|
||
# 添加高斯噪声 | ||
mu, sigma = 0, 1 # 均值和标准差 | ||
noise = np.random.normal(mu, sigma, y.shape) | ||
y_noisy = y + noise | ||
|
||
|
||
# 转置 x_aug 和 y_noisy 以进行打乱和分割 | ||
x_aug = x_aug.T | ||
y_noisy = y_noisy.T | ||
|
||
# 将数据打乱并分成训练集和测试集 | ||
x_train, x_test, y_train, y_test = train_test_split(x_aug, y_noisy, test_size=0.2, random_state=42) | ||
|
||
# 输出结果 | ||
print("训练集 X 的形状:", x_train[:,:-1].shape) | ||
print("测试集 X 的形状:", x_test.shape) | ||
print("训练集 Y 的形状:", y_train.shape) | ||
print("测试集 Y 的形状:", y_test.shape) | ||
|
||
|
||
``` | ||
=== "线性模型,损失函数和梯度" | ||
``` python | ||
# 线性模型函数 | ||
class Linear(): | ||
def __init__(self, input_dim, output_dim): | ||
self.params = {} | ||
self.params['w'] = np.random.randn(input_dim, output_dim).astype(np.float32) | ||
self.params['b'] = np.random.randn(output_dim).astype(np.float32) | ||
``` | ||
=== "优化:随机梯度下降法" | ||
``` python | ||
def inverse_matrix(matrix): | ||
try: | ||
# 判断矩阵是否可逆 | ||
if np.linalg.det(matrix) == 0: | ||
return None, "The matrix is not invertible" | ||
else: | ||
# 计算矩阵的逆 | ||
inv_matrix = np.linalg.inv(matrix) | ||
return inv_matrix | ||
except np.linalg.LinAlgError as e: | ||
return None, f"An error occurred: {str(e)}" | ||
|
||
# 优化算法: | ||
def optimizer_RLS(X, y, model, reg_lambda=0.001): | ||
x_mean_T = np.mean(X, axis=0).T | ||
y_mean = np.mean(y) | ||
x_sub = np.subtract(X,x_mean_T) | ||
# 计算w_star的第一项 | ||
temp1 = inverse_matrix(np.dot(x_sub.T,x_sub)+reg_lambda*np.eye(x_sub.shape[1])) | ||
# 计算w_star的第二项 | ||
temp2 = np.dot(x_sub.T,(y-y_mean)) | ||
w_star = np.dot(temp1,temp2) | ||
b_star = y_mean - np.dot(x_mean_T, w_star) | ||
model.params['w'] = w_star | ||
model.params['b'] = b_star | ||
|
||
model = Linear(5, 1) | ||
optimizer_RLS(x_train[:,:-1], y_train, model) | ||
print("w_pred:",model.params['w'], "b_pred: ", model.params['b']) # print model parameters | ||
``` | ||
|